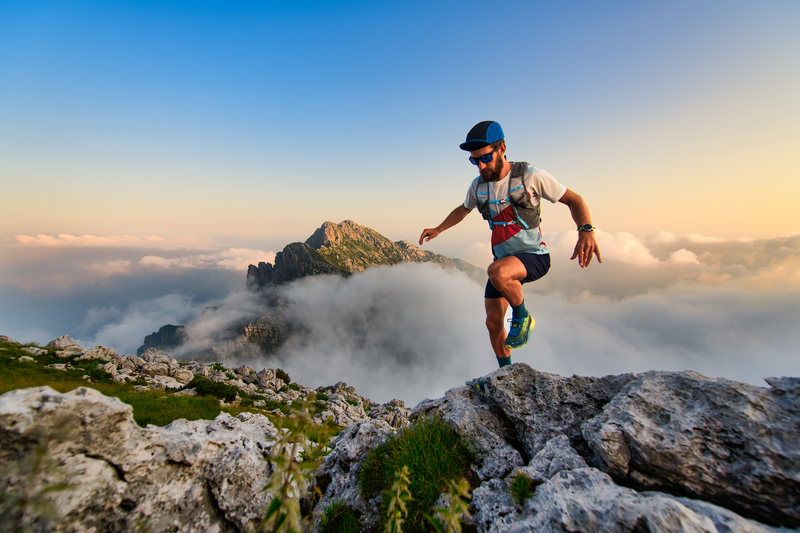
94% of researchers rate our articles as excellent or good
Learn more about the work of our research integrity team to safeguard the quality of each article we publish.
Find out more
ORIGINAL RESEARCH article
Front. Big Data
Sec. Data Analytics for Social Impact
Volume 8 - 2025 | doi: 10.3389/fdata.2025.1440816
This article is part of the Research Topic Intelligence and Big Data for Sustainable Urban Built Environment View all 4 articles
The final, formatted version of the article will be published soon.
You have multiple emails registered with Frontiers:
Please enter your email address:
If you already have an account, please login
You don't have a Frontiers account ? You can register here
As environmental awareness increased due to the surge in greenhouse gases, green travel modes such as bicycles and walking have gradually became popular choices. However, the current traffic environment has many hidden problems that endanger the personal safety of traffic participants and hinder the development of green travel. Traditional methods, such as identifying risky locations after traffic accidents, suffer from the disadvantages of delayed response and lack of foresight. Against this background, we proposed a mobile edge crowdsensing framework to dynamically assess urban traffic green travel safety risks. Specifically, a large number of mobile devices were used to sense the road environment, from which a semantic detection framework detected the traffic high-risk behaviors of traffic participants. Then multi-source and heterogeneous urban crowdsensing data were used to model the travel safety risk to achieve a comprehensive and real-time assessment of urban green travel safety. We evaluated our method by leveraging real-world datasets collected from Xiamen Island. Results showed that our framework could accurately detect traffic high-risk behaviors with average F1-scores of 86.5% and assessed the travel safety risk with R² of 0.85 outperforming various baseline methods.
Keywords: Urban traffic, Traffic data, mobile crowdsensing, Urban computing, Computer Vision
Received: 30 May 2024; Accepted: 24 Feb 2025.
Copyright: © 2025 Wang, Tang, Wei, Yang, Yang, Wang and Chen. This is an open-access article distributed under the terms of the Creative Commons Attribution License (CC BY). The use, distribution or reproduction in other forums is permitted, provided the original author(s) or licensor are credited and that the original publication in this journal is cited, in accordance with accepted academic practice. No use, distribution or reproduction is permitted which does not comply with these terms.
* Correspondence:
Longbiao Chen, Xiamen University, Xiamen, China
Disclaimer: All claims expressed in this article are solely those of the authors and do not necessarily represent those of their affiliated organizations, or those of the publisher, the editors and the reviewers. Any product that may be evaluated in this article or claim that may be made by its manufacturer is not guaranteed or endorsed by the publisher.
Research integrity at Frontiers
Learn more about the work of our research integrity team to safeguard the quality of each article we publish.