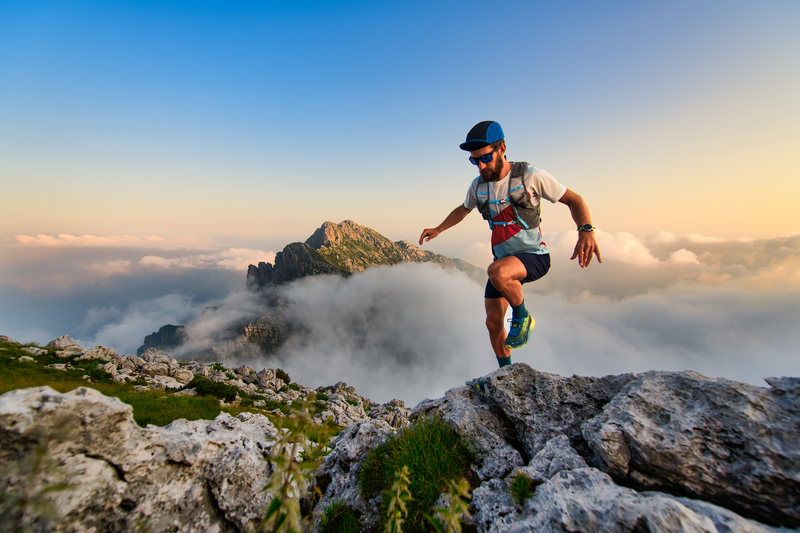
94% of researchers rate our articles as excellent or good
Learn more about the work of our research integrity team to safeguard the quality of each article we publish.
Find out more
EDITORIAL article
Front. Big Data , 07 January 2025
Sec. Cybersecurity and Privacy
Volume 7 - 2024 | https://doi.org/10.3389/fdata.2024.1537878
This article is part of the Research Topic Cybersecurity and Artificial Intelligence: Advances, Challenges, Opportunities, Threats View all 6 articles
Editorial on the Research Topic
Cybersecurity and artificial intelligence: advances, challenges, opportunities, threats
Cybersecurity and AI have become increasingly important in today's interconnected world. Developments in them and because of them find their way regularly not only to scientific literature but to the media as well. Despite its benefits, the increasing use of and reliance on AI, including Machine Learning (ML), have created a complex threat landscape. Simultaneously, cybercriminals have become more sophisticated and organized, targeting not only individuals but also organizations and even nations. These threat actors use advanced tactics, techniques, and procedures to exploit vulnerabilities to attack AI-powered systems. To mitigate these threats, it is essential to enhance our understanding of the risks involved and to develop effective, specially targeted cybersecurity strategies and robust defensive mechanisms. The increasing pervasiveness of AI and related technologies, coupled with the growing sophistication of cybercriminals, has created a challenging threat landscape that requires innovative solutions to mitigate.
Five articles are published in this Research Topic. They present novel research results in three areas, namely on ML-based clustering algorithms, on the trustworthiness of AI-based systems, and on risks emerging from the malicious use of AI.
Streaming services are highly popular today. The clustering of users of online or offline services is frequently used for fraud detection. Density-based spatial clustering of applications with noise (DBSCAN) is a clustering algorithm used in machine learning to partition data into clusters based on their distance to other points. Mochurad et al., in their article entitled “A fast parallelized DBSCAN algorithm based on OpenMp for detection of criminals on streaming services,” propose a parallel algorithm for fast clustering of users of a streaming service. The proposed algorithm maintains DBSCAN's distinctive features while exhibiting significant speed-up.
Within a Security Information and Event Management (SIEM) system, the User and Entity Behavior Analytics (UEBA) engine aims to analyze the behavior of employees, third-party contractors, and collaborators of the organization to detect misbehavior in user activities. In their article entitled “A comprehensive investigation of clustering algorithms for User and Entity Behavior Analytics,” Artioli et al. conduct a comprehensive investigation of multiple clustering algorithms, encompassing a wide range of clustering techniques, including traditional and more modern advances, and they evaluate their applicability for UEBA.
Current AI risk management frameworks neglect human factors, and metrics for socially related or human threats are missing. Polemi et al., in their article entitled “Challenges and efforts in managing AI trustworthiness risks: a state of knowledge,” provide a comprehensive approach to AI trustworthiness, combining technical and social mitigation measures, standards, and ongoing research initiatives by exploring various dimensions of trustworthiness of AI-based systems, covering legislation, AI cyber threat intelligence, and characteristics of AI adversaries. Furthermore, the socio-psychological threats associated with AI integration into society are examined, addressing issues such as bias, misinformation, and privacy erosion. As a result, the importance of collaboration between cybersecurity engineers, AI experts, and social-psychology-behavior-ethics professionals is highlighted.
AI is increasingly used to support the development of software. Even though this practice can improve coding proficiency, security of the developed code cannot be ensured. In fact, recent research has demonstrated that some AI models produce software with vulnerabilities. Negri-Ribalta et al., in their article entitled “A systematic literature review on the impact of AI models on the security of code generation”, conduct a systematic literature review to establish and systematize the state of the art on how AI models impact software security.
Recent advancements in AI, especially deep learning, have contributed to a significant increase in the creation of new realistic-looking synthetic media (video, image, and audio) and manipulation of existing media; this has resulted in what is currently called “deepfake” material. Altuncu et al., in their article entitled “Deepfake: definitions, performance metrics and standards, datasets, and a meta-review”, provide a comprehensive overview of deepfake, covering multiple important aspects of this emerging concept. Such aspects include different definitions of the concept, commonly used performance metrics and standards, and deepfake-related datasets. The paper also provides a meta-review of 15 deepfake-related survey papers published since 2020, focusing not only on the above aspects but also on the analysis of key challenges and recommendations.
We hope that the reader will find useful information in this Research Topic on some aspects of the emerging field of the intersection between AI and cybersecurity. Further research in this area is vital if we are to better understand the nature of AI as a “double-edged sword” and mitigate the accordant emerging risks.
PP: Project administration, Writing – review & editing. SK: Project administration, Writing – original draft, Writing – review & editing. NP: Project administration, Writing – review & editing.
The authors declare that the research was conducted in the absence of any commercial or financial relationships that could be construed as a potential conflict of interest.
The author(s) declared that they were an editorial board member of Frontiers, at the time of submission. This had no impact on the peer review process and the final decision.
All claims expressed in this article are solely those of the authors and do not necessarily represent those of their affiliated organizations, or those of the publisher, the editors and the reviewers. Any product that may be evaluated in this article, or claim that may be made by its manufacturer, is not guaranteed or endorsed by the publisher.
Keywords: cybersecurity (CS), artificial intelligence, adversarial machine learning, advances, challenges, opportunities, threats
Citation: Papadopoulos P, Katsikas S and Pitropakis N (2025) Editorial: Cybersecurity and artificial intelligence: advances, challenges, opportunities, threats. Front. Big Data 7:1537878. doi: 10.3389/fdata.2024.1537878
Received: 01 December 2024; Accepted: 17 December 2024;
Published: 07 January 2025.
Edited and reviewed by: Elena Ferrari, University of Insubria, Italy
Copyright © 2025 Papadopoulos, Katsikas and Pitropakis. This is an open-access article distributed under the terms of the Creative Commons Attribution License (CC BY). The use, distribution or reproduction in other forums is permitted, provided the original author(s) and the copyright owner(s) are credited and that the original publication in this journal is cited, in accordance with accepted academic practice. No use, distribution or reproduction is permitted which does not comply with these terms.
*Correspondence: Pavlos Papadopoulos, UC5QYXBhZG9wb3Vsb3NAbmFwaWVyLmFjLnVr
Disclaimer: All claims expressed in this article are solely those of the authors and do not necessarily represent those of their affiliated organizations, or those of the publisher, the editors and the reviewers. Any product that may be evaluated in this article or claim that may be made by its manufacturer is not guaranteed or endorsed by the publisher.
Research integrity at Frontiers
Learn more about the work of our research integrity team to safeguard the quality of each article we publish.