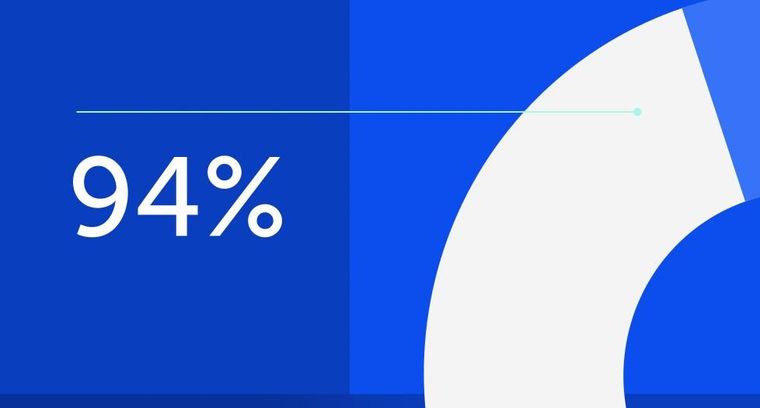
94% of researchers rate our articles as excellent or good
Learn more about the work of our research integrity team to safeguard the quality of each article we publish.
Find out more
CORRECTION article
Front. Big Data, 16 October 2023
Sec. Big Data and AI in High Energy Physics
Volume 6 - 2023 | https://doi.org/10.3389/fdata.2023.1301942
This article is a correction to:
Applications and Techniques for Fast Machine Learning in Science
A corrigendum on
Applications and techniques for fast machine learning in science
by Deiana, A. M., Tran, N., Agar, J., Blott, M., Di Guglielmo, G., Duarte, J., Harris, P., Hauck, S., Liu, M., Neubauer, M. S., Ngadiuba, J., Ogrenci-Memik, S., Pierini, M., Aarrestad, T., Bähr, S., Becker, J., Berthold, A.-S., Bonventre, R. J., Müller Bravo, T. E., Diefenthaler, M., Dong, Z., Fritzsche, N., Gholami, A., Govorkova, E., Guo, D., Hazelwood, K. J., Herwig, C., Khan, B., Kim, S., Klijnsma, T., Liu, Y., Lo, K. H., Nguyen, T., Pezzullo, G., Rasoulinezhad, S., Rivera, R. A., Scholberg, K., Selig, J., Sen, S., Strukov, D., Tang, W., Thais, S., Unger, K. L., Vilalta, R., von Krosigk, B., Wang, S., and Warburton, T. K. (2022). Front. Big Data 5:787421. doi: 10.3389/fdata.2022.787421
In the published article, there was an error regarding the affiliation for author Anne-Sophie Berthold. Instead of having affiliation 25 (Cerebras Systems, Sunnyvale, CA, United States) they should have 14 (Institute of Nuclear and Particle Physics, Technische Universität Dresden, Dresden, Germany).
The authors apologize for this error and state that this does not change the scientific conclusions of the article in any way. The original article has been updated.
All claims expressed in this article are solely those of the authors and do not necessarily represent those of their affiliated organizations, or those of the publisher, the editors and the reviewers. Any product that may be evaluated in this article, or claim that may be made by its manufacturer, is not guaranteed or endorsed by the publisher.
Keywords: machine learning for science, big data, particle physics, codesign, coprocessors, heterogeneous computing, fast machine learning
Citation: Deiana AM, Tran N, Agar J, Blott M, Di Guglielmo G, Duarte J, Harris P, Hauck S, Liu M, Neubauer MS, Ngadiuba J, Ogrenci-Memik S, Pierini M, Aarrestad T, Bähr S, Becker J, Berthold A-S, Bonventre RJ, Müller Bravo TE, Diefenthaler M, Dong Z, Fritzsche N, Gholami A, Govorkova E, Guo D, Hazelwood KJ, Herwig C, Khan B, Kim S, Klijnsma T, Liu Y, Lo KH, Nguyen T, Pezzullo G, Rasoulinezhad S, Rivera RA, Scholberg K, Selig J, Sen S, Strukov D, Tang W, Thais S, Unger KL, Vilalta R, von Krosigk B, Wang S and Warburton TK (2023) Corrigendum: Applications and techniques for fast machine learning in science. Front. Big Data 6:1301942. doi: 10.3389/fdata.2023.1301942
Received: 25 September 2023; Accepted: 26 September 2023;
Published: 16 October 2023.
Approved by:
Frontiers Editorial Office, Frontiers Media SA, SwitzerlandCopyright © 2023 Deiana, Tran, Agar, Blott, Di Guglielmo, Duarte, Harris, Hauck, Liu, Neubauer, Ngadiuba, Ogrenci-Memik, Pierini, Aarrestad, Bähr, Becker, Berthold, Bonventre, Müller Bravo, Diefenthaler, Dong, Fritzsche, Gholami, Govorkova, Guo, Hazelwood, Herwig, Khan, Kim, Klijnsma, Liu, Lo, Nguyen, Pezzullo, Rasoulinezhad, Rivera, Scholberg, Selig, Sen, Strukov, Tang, Thais, Unger, Vilalta, von Krosigk, Wang and Warburton. This is an open-access article distributed under the terms of the Creative Commons Attribution License (CC BY). The use, distribution or reproduction in other forums is permitted, provided the original author(s) and the copyright owner(s) are credited and that the original publication in this journal is cited, in accordance with accepted academic practice. No use, distribution or reproduction is permitted which does not comply with these terms.
*Correspondence: Allison McCarn Deiana, YWRlaWFuYUBzbXUuZWR1; Nhan Tran, bnRyYW5AZm5hbC5nb3Y=
Disclaimer: All claims expressed in this article are solely those of the authors and do not necessarily represent those of their affiliated organizations, or those of the publisher, the editors and the reviewers. Any product that may be evaluated in this article or claim that may be made by its manufacturer is not guaranteed or endorsed by the publisher.
Research integrity at Frontiers
Learn more about the work of our research integrity team to safeguard the quality of each article we publish.