- 1Academic Unit of Ophthalmology, Institute of Inflammation and Ageing, University of Birmingham, Birmingham, United Kingdom
- 2Birmingham and Midland Eye Centre, Birmingham, United Kingdom
- 3Academic Ophthalmology, School of Medicine, University of Nottingham, Nottingham, United Kingdom
- 4Department of Cornea and Refractive Surgery, LV Prasad Eye Institute, Hyderabad, India
- 5Singapore National Eye Centre, Singapore Eye Research Institute, Singapore, Singapore
- 6Department of Ophthalmology and Visual Sciences, Duke-National University of Singapore (NUS) Medical School, Singapore, Singapore
The accelerated growth in electronic health records (EHR), Internet-of-Things, mHealth, telemedicine, and artificial intelligence (AI) in the recent years have significantly fuelled the interest and development in big data research. Big data refer to complex datasets that are characterized by the attributes of “5 Vs”—variety, volume, velocity, veracity, and value. Big data analytics research has so far benefitted many fields of medicine, including ophthalmology. The availability of these big data not only allow for comprehensive and timely examinations of the epidemiology, trends, characteristics, outcomes, and prognostic factors of many diseases, but also enable the development of highly accurate AI algorithms in diagnosing a wide range of medical diseases as well as discovering new patterns or associations of diseases that are previously unknown to clinicians and researchers. Within the field of ophthalmology, there is a rapidly expanding pool of large clinical registries, epidemiological studies, omics studies, and biobanks through which big data can be accessed. National corneal transplant registries, genome-wide association studies, national cataract databases, and large ophthalmology-related EHR-based registries (e.g., AAO IRIS Registry) are some of the key resources. In this review, we aim to provide a succinct overview of the availability and clinical applicability of big data in ophthalmology, particularly from the perspective of corneal diseases and cataract, the synergistic potential of big data, AI technologies, internet of things, mHealth, and wearable smart devices, and the potential barriers for realizing the clinical and research potential of big data in this field.
1. Introduction
The concept of big data was first introduced in 1990s to capture dataset that are too complex to be stored and analyzed using traditional computer software (Mallappallil et al., 2020). It was previously defined as data that display the characteristics of “3 Vs”—volume, velocity and variety (Mooney et al., 2015). Additional attributes such as veracity and value have also been suggested to fully capture the true nature and values of big data (known as the “5 Vs”).1
In the recent years, the accelerated growth in electronic health records (EHR), disease registries, biobanks, mHealth, Internet-of-Things (IoT), telemedicine, and artificial intelligence (AI) have helped unlock the multi-faceted potential of big data (Chiang et al., 2018; Li et al., 2021; Sahu et al., 2021). Compared to traditional dataset, the wealth of information provided by big data (which are often derived from large-scale or nationwide studies) can facilitate comprehensive and timely examination of the epidemiology, trends, characteristics, outcomes, and prognostic factors of the diseases (Roski et al., 2014; Mallappallil et al., 2020). In addition, the findings help to guide public health policies in terms of risk factors modulation, disease prevention and control, optimization of healthcare service provision, and allocation of research funding targeting more prevalent diseases (Roski et al., 2014).
The multi-dimensional values of big data have been increasingly capitalized in many branches of medicine, including ophthalmology (Cheng et al., 2020; Li et al., 2021). One of the best examples relates to the recent use of big data in understanding the trends and spread of COVID-19, risk factors, treatment outcomes, and morbidity/mortality, which helped inform the clinical practice and public health policies (Haleem et al., 2020; Ting et al., 2020c; Villanustre et al., 2021). Furthermore, big data have enabled the development of highly accurate AI algorithms (which are often data-hungry) in diagnosing a wide range of medical diseases as well as discovering new patterns or associations of diseases that are previously unknown to us (Figure 1) (Ting D. S. W. et al., 2017; Milea et al., 2020; Mehta et al., 2021; Rim et al., 2021; Ting et al., 2021b).
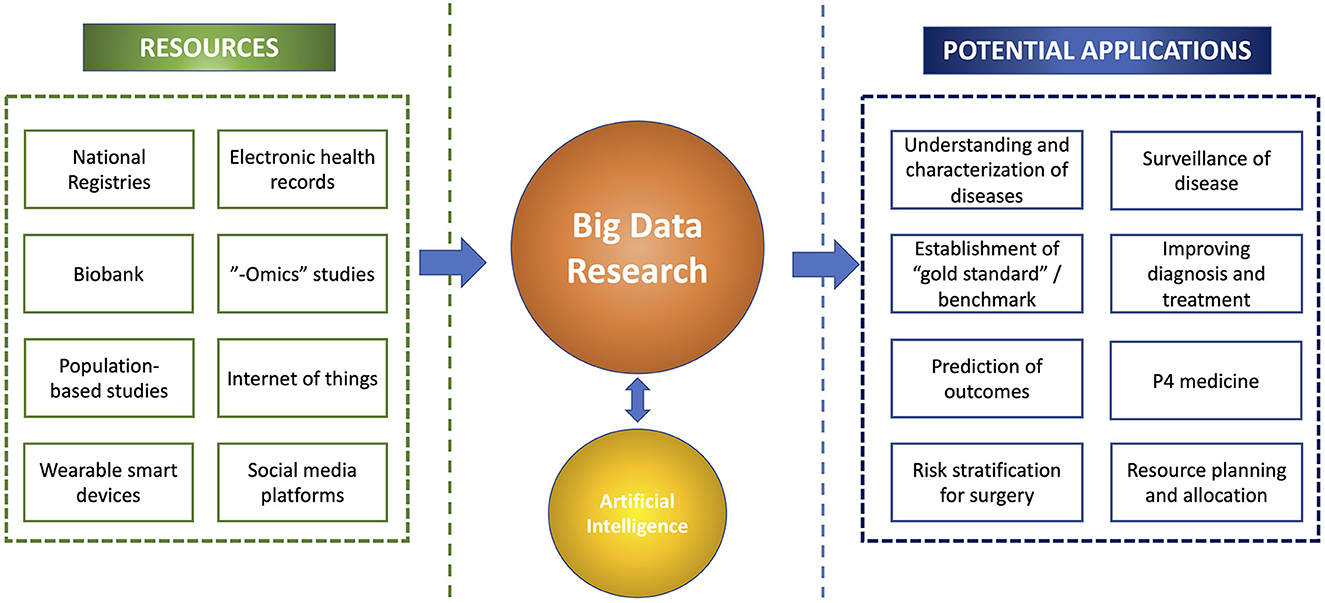
Figure 1. An overview of the big data research, including the resources, potential synergism with artificial intelligence, and clinical applications.
Within the field of ophthalmology, there is a rapidly expanding pool of large clinical registries, epidemiological studies, omics studies, and biobanks through which big data can be accessed (Chua et al., 2019; Tan et al., 2019). In view of the increased availability and accessibility of big data and recent technological advancements, this paper aimed to provide a succinct overview of the availability, clinical applicability and future potentials of big data in ophthalmology, particularly from the perspective of corneal diseases and cataract.
2. Big data in corneal diseases
According to a recent report by the World Health Organization (WHO), corneal opacity represents the 5th leading cause of blindness globally (Flaxman et al., 2017).2 It is also estimated that ~6 million people suffer from moderate to severe visual impairment secondary to corneal opacity, including non-trachomatous and trachomatous-related cases (see text footnote 2). More importantly, corneal blindness has been shown to be significantly more prevalent in low- and middle-income countries (LMICs), mainly due to limited healthcare resources, higher rate of ocular trauma, poor environmental and personal hygiene, malnutrition, and lower educational level, amongst others (Flaxman et al., 2017; Porth et al., 2019; Ting et al., 2021e). Given the enormity of corneal blindness globally and the significant mismatch between the disease burden and the availability of healthcare resources and workforce, strategic measures are urgently needed.
Within the field of cornea, there is an increasing pool of large corneal registries and epidemiological studies that contains rich resources of big data. These include corneal transplant registries, infectious keratitis studies, genomic studies, large ophthalmology-related registries, EMR-based platforms, and biobanks (Keenan et al., 2012; Chiang et al., 2018; Donthineni et al., 2019). These cornea-related big data enable a better grasp of the prevalence, risk factors, outcomes, and impact of various corneal diseases, which in turn allow for more effective formulations of various therapeutic and preventative strategies in tackling the diseases. In this section, we summarize the main cornea-related big data in various countries and their impact on clinical practice, research and public policies.
2.1. Corneal transplant registries
Corneal transplantation or keratoplasty is the most common type of transplantation performed worldwide (Tan et al., 2012). Currently it remains the main method for restoring corneal clarity and vision in patients with visually debilitating corneal diseases (Tan et al., 2012). However, the persistent shortage of donor corneas has posed significant barriers to successful corneal transplantations (Gain et al., 2016). This has also led to the implementation of various innovative measures, with an aim to improve the eye donation rate (Rithalia et al., 2009; Ting et al., 2016a), utilization of donor corneas (Ting et al., 2016b; Gupta et al., 2018), and reduction of the need for donor corneas (Kinoshita et al., 2018; Ting et al., 2022). In order to tackle this persistent barrier, a wide range of national corneal graft registries and eye banks have been established across the world, including the US, the UK, Europe, India, Australia, and Singapore, amongst others (Table 1) (Tan et al., 2015, 2019; Sharma et al., 2019; Dunker et al., 2021).3
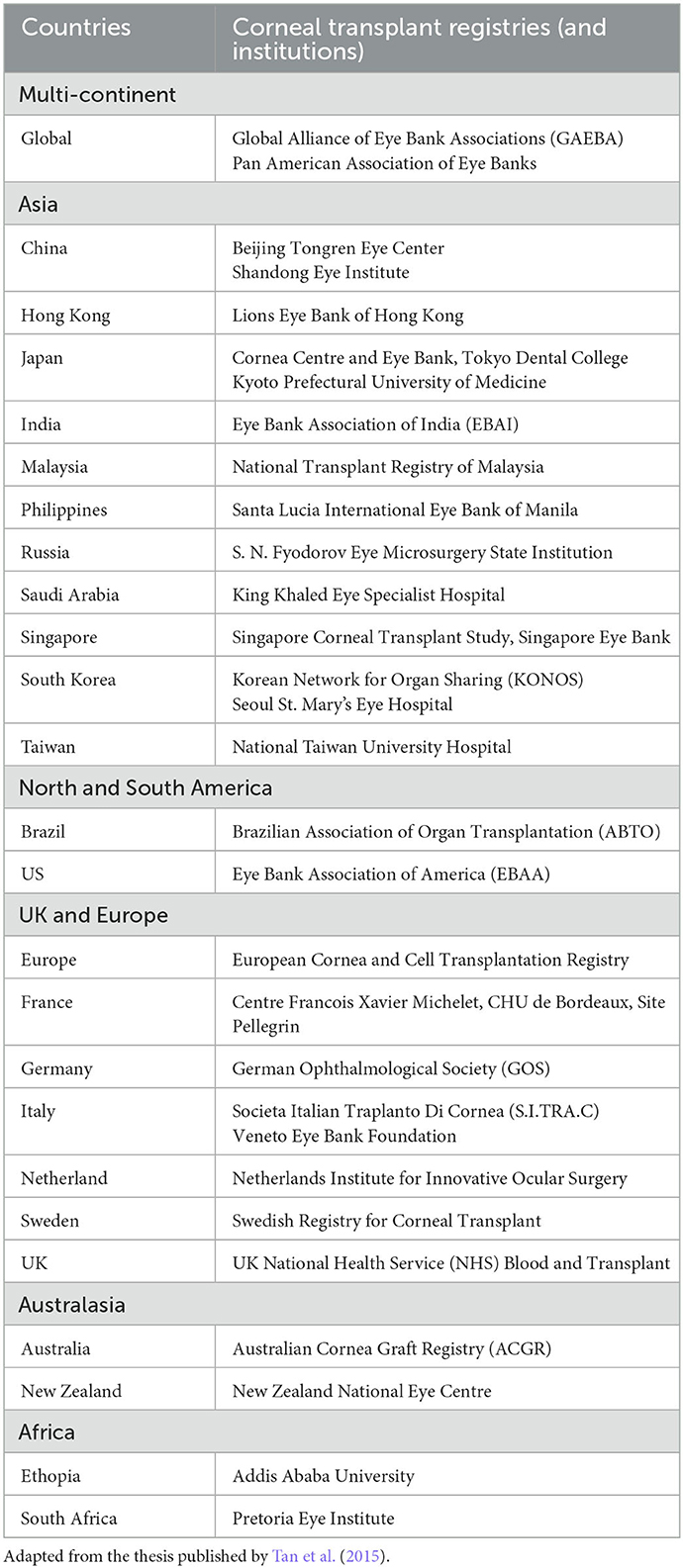
Table 1. Summary of main corneal transplant registries and institutions in the world, categorized by continents.
The purposes of these national registries and eye banks are manifold. Firstly, it helps standardize the corneal donation-to-transplantation pathway nationally and identify any potential limiting factors, enabling more effective interventions to improve the conversion rate of eye donation and the utilization rate of the donated corneas (Gogia et al., 2015; Ting et al., 2016b; Sharma et al., 2019). Secondly, the prospective database can facilitate examination of the ongoing availability of donor corneas to allow for equal and fair distribution of the donor corneas across the country (Ting et al., 2016b; Gupta et al., 2018) (see text footnote 3).4 It also helps inform the policymakers and relevant stakeholders on the need for importation (or exportation) depending on the local supply of donor corneas. Thirdly, they provide up-to-date examination of the trends in the types and indications of keratoplasty (Keenan et al., 2012; Park et al., 2015). For instance, various studies have demonstrated a paradigm shift from penetrating keratoplasty (PK) to lamellar keratoplasty [including anterior lamellar keratoplasty (ALK) and endothelial keratoplasty (EK)] over the past decade in many countries. A recent European Cornea and Cell Transplantation Registry study of 10 centers from the Europe, the UK and Switzerland (n = 12913 keratoplasty) demonstrated that Descemet stripping automated endothelial keratoplasty (DSAEK) was the most commonly performed technique (46%), followed by PK (30%) and Descemet membrane endothelial keratoplasty (9%) (Dunker et al., 2021). In addition, the study demonstrated that Fuchs endothelial corneal dystrophy (FECD), regraft, pseudophakic bullous keratopathy (PBK), and keratoconus were the main indications for keratoplasty. These common indications were consistently reported in many other national studies conducted in other countries (Keenan et al., 2012; Ting et al., 2012; Park et al., 2015; Tan et al., 2015; Ang et al., 2016c; Fuest et al., 2017). Understanding of the common indications for keratoplasty provides the clinicians and researchers with a clearer picture of how the limited resources (i.e., donor corneas) are being utilized. In addition, more targeted research effort can be channeled toward these higher prevalent corneal diseases to search for alternative therapeutic strategies and reduce the need for donor corneas.
Furthermore, useful information can be obtained from these national corneal transplant registries to understand the risk factors and prognostic factors of keratoplasty, ultimately improving the clinical outcomes (Ang et al., 2011, 2012a, 2016a; Bose et al., 2013). Ang et al. (2016b) observed that patients with FECD and bullous keratopathy achieved a better long-term graft survival following Descemet membrane endothelial keratoplasty (DMEK) when compared to Descemet stripping automated endothelial keratoplasty (DSAEK) and PK. On the other hand, an Australian national study of >15,000 cases of keratoplasty demonstrated that the survival of lamellar keratoplasty (i.e., DALK and EK) fared worse than PK, with some evidence showing the influence of learning curve on the outcome of EK (Coster et al., 2014). Important prognostic factors for graft survival rate, including the indication for graft, number of previous grafts, history of ocular surface inflammation or glaucoma, corneal neovascularization, and postoperative events such as graft rejection or infection, were also identified via these national corneal transplantation studies (Williams et al., 2008; Ang et al., 2012b, 2014, 2020; Sibley et al., 2020). Indications such as PBK and infectious keratitis (IK) have been shown to be associated with a worse outcome compared to “low-risk” conditions such as keratoconus and FECD following keratoplasty (Tan et al., 2012), highlighting the need for improvement in the treatment strategy for certain indications (Ang and Sng, 2018).
More importantly, the registries enable examination and monitoring for any significant postoperative adverse events such as infection and endophthalmitis (Chen et al., 2015; Gauthier et al., 2017; Song et al., 2021). Edelstein et al. (2016) previously conducted a study of 354,390 keratoplasty based on the data from the Eye Bank Association of America, analyzing all adverse events following all types of keratoplasty. They observed a higher rate of fungal infection in their study compared to non-US studies and postulated that this might be due to the lack of antifungal agent used in the corneal storage medium in the US (Chen et al., 2015; Edelstein et al., 2016). It was also found that fungal keratitis and endophthalmitis were more common following EK (1.5–3 times higher risk) than PK and ALK, potentially attributed to the increased warming time associated with the preparation of EK tissues in the eye bank (Edelstein et al., 2016). These findings will allow for the refinement of the eye bank protocol in terms of processing and storage of donor corneas, ultimately leading to improved clinical outcome and safety.
2.2. Infectious keratitis databases
Corneal opacity is the 5th leading cause of blindness globally, with IK being the main culprit. IK was previously recognized as a “silent epidemic”, and recently, a “neglected tropical disease” status was proposed (Ung et al., 2019). The incidence is estimated to range between 2.5–799 per 100,000 population per year (Ting et al., 2021e). It can be caused by a wide range of organisms, including bacteria, fungi, viruses, and parasites, and polymicrobial infection (Ting et al., 2019b, 2021d; Khoo et al., 2020). In view of its significant impact on human health, healthcare systems and economy, it is therefore not surprising to observe a vast amount of literature on IK, encompassing the epidemiology, risk factors, clinical characteristics, causative organisms, management, and outcomes of the disease. Large IK studies published in the recent years are summarized in Table 2 (Lin et al., 2017, 2019; Tan et al., 2017; Khor et al., 2018; Peng et al., 2018; Green et al., 2019; Tavassoli et al., 2019; Asbell et al., 2020; Khoo et al., 2020; Kowalski et al., 2020; Somerville et al., 2021; Ting et al., 2021d).
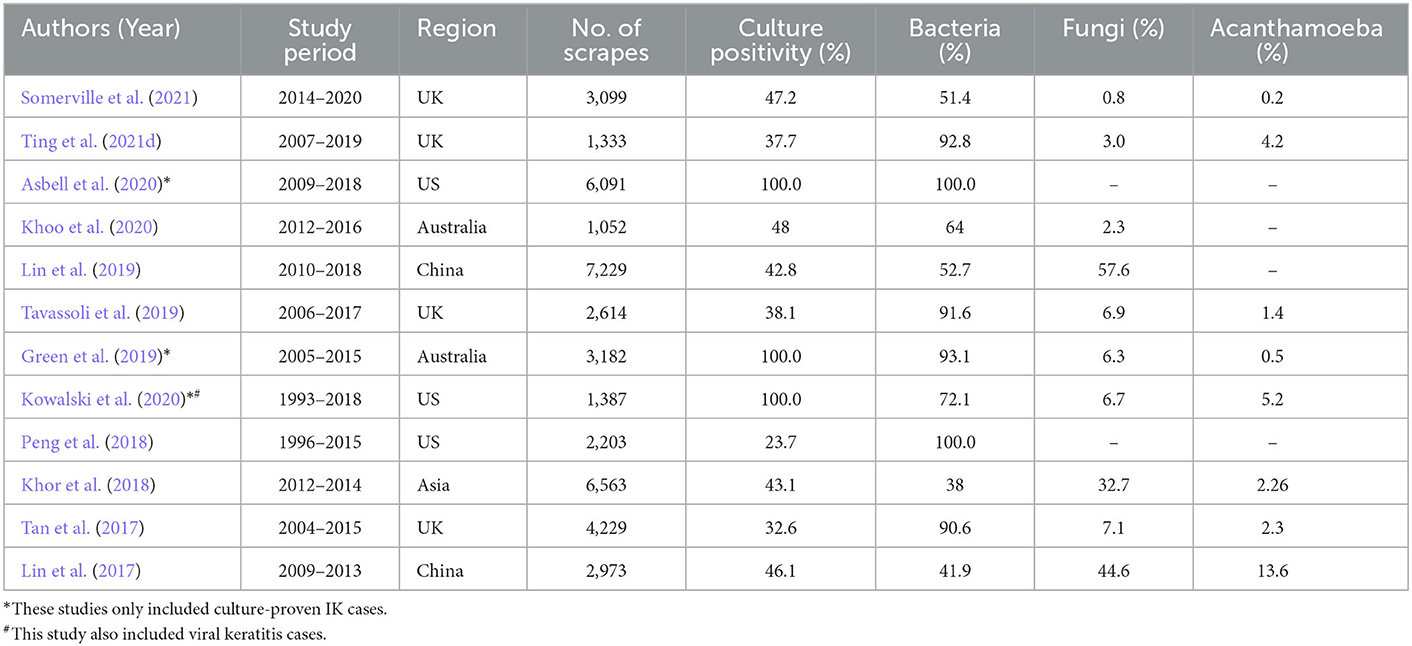
Table 2. Summary of large-scale infectious keratitis study (>1,000 cases) in the world published between 2016 and 2021, in chronological order.
The clinical and laboratory data captured by these large-scale IK studies enables a better grasp of the microbiological profiles, risk factors, disease impact, and treatment response. So far, from the epidemiological standpoint, these studies have helped unveil the considerable geographical, temporal and seasonal variations in IK, which provide useful guidance to the choice of antimicrobial treatment. For example, Staphylococci spp. and Pseudomonas spp. were shown to be the most common organisms in the UK, the US and Australia (Tan et al., 2017; Tavassoli et al., 2019; Khoo et al., 2020; Kowalski et al., 2020; Ting et al., 2021d). In addition, several studies (Tan et al., 2017; Ting et al., 2018, 2021d) have identified an increasing trend of moraxella keratitis in the UK over the past decade. In contrast, a recent Asia Cornea Society Infectious Keratitis Study (ACSIKS) of more than 6,000 patients showed that fungal and bacterial infections were the main causes of IK in developing and developed countries, respectively (Khor et al., 2018). More importantly. the study observed ~50% of the eyes developed moderate visual loss (< 6/18 vision), with 46% of the performed therapeutic keratoplasty failed by 6 months' follow-up, highlighting the significant impact on the affected patients.
Studies have shown that the initial severity of the ulcer and presenting visual acuity serve as important prognostic factors for IK (Khoo et al., 2020; Ting et al., 2021a,c). Therefore understanding the risk factors via big data research allows for effective implantation of various preventative strategies in reducing the incidence of IK. Contact lens (CL) has been consistently identified as one of the most common risk factors for IK (Cariello et al., 2011; Keay et al., 2011; Ting et al., 2021a). In particular, the risk of CL-related IK was shown to be associated with use of expired CL and overnight CL wear (Sauer et al., 2020). Understanding of these underlying factors allow for better education among the patients and CL wearers. Trauma serves as another important risk factor for IK, particularly in the developing countries (Ganguly et al., 2011; Kaliamurthy et al., 2013). In addition, based on a population-based, cross-section sectional study, [Cornea Opacity Rural Epidemiological (CORE) study] (Gupta et al., 2017), it was found that the use of traditional eye medicine and self-medication was prevalent in the rural regions of India, which could lead to delay in seeking appropriate medical care and exacerbation of corneal diseases and/or infection. These epidemiological studies have helped improve the public awareness and call for new regulatory legislations to address these issues.
Broad-spectrum topical antimicrobial therapy serves as the current mainstay of treatment for IK, though their efficacy is being challenged by the emergence of AMR, observed in several large-scale IK studies (Lalitha et al., 2017; Asbell et al., 2020; Ting et al., 2021e). Clinically, AMR-related pathogens has been shown to negatively affect the outcome and healing time of IK (Kaye et al., 2010). In the Antibiotic Resistance Among Ocular Microorganisms (ARMOR) with data from >6,000 ocular isolates, Asbell et al. (2020) observed that ~40% of the Staphylococci spp. were methicillin-resistant, and many of them were multidrug resistant. On the other hand, various studies in the UK demonstrated a low rate of AMR (< 5–10%) against the commonly employed antibiotic regimens used for IK, including fluoroquinolone, cephalosporin and aminoglycoside (Tan et al., 2017; Tavassoli et al., 2019; Ting et al., 2021d). These findings emphasize the wide geographical and temporal variations in AMR for IK and the importance of updated examination in specific regions. Better knowledge of the AMR pattern could also help guide the most appropriate initial treatment for IK in each region.
2.3. Corneal genomic studies
The increase in large-scale genetic studies, particularly genome-wide association study (GWAS) and genome-wide linkage study (GWLS), has significantly advanced our understanding of many diseases, including corneal diseases, and offer potential novel targets for gene therapy (Riazuddin et al., 2009, 2010, 2013; Baratz et al., 2010; Burdon et al., 2011; Bykhovskaya et al., 2012; Czugala et al., 2012; Li et al., 2013; Lu et al., 2013; Sahebjada et al., 2013; Dudakova et al., 2015; Afshari et al., 2017; McComish et al., 2019). GWAS is an invaluable methodology designed to analyze common genetic variations across the whole genome, particularly single nucleotide polymorphisms (SNPs), by analyzing the genotype-phenotype associations of a disease in case-control cohorts with a large number of individuals. On the other hand, GWLS is a useful tool used to genotype a particular disease by examining families with affected and unaffected individuals (Karolak and Gajecka, 2017; Tam et al., 2019).
FECD and keratoconus are by far the two most commonly investigated corneal diseases. The research focus on these two conditions is primarily driven by the high burden and prevalence of the diseases. Moreover, they represent the most common indications for keratoplasty in many countries (Ting et al., 2012; Park et al., 2015), placing significant burden on the donor corneas. Over the years, GWAS has increasingly been used to identify genetic susceptibility regions in FECD and keratoconus (Iliff et al., 2012; Karolak and Gajecka, 2017). For instance, Hardcastle et al. (2021) recently conducted a multi-ethnic GWAS of keratoconus, including >100,000 individuals, and identified 36 significant genomic loci that were associated with keratoconus. McComish et al. (2019) discovered a novel genetic locus in PNPLA2 at chromosome 11 for keratoconus based on over 6 million genetic variants. Several novel genetic loci for FECD, including TCF4, LAMC1 rs3768617, LINC00970/ATP1B1 rs1200114, and KANK4 rs79742895, have also been identified (Afshari et al., 2017). GWLS have also facilitated the identification of a number of important genetic mutations linked to keratoconus, including TGFBI, TCEB1, CAST, COL8A1, and LOX genes (Karolak and Gajecka, 2017). Next-generation sequencing, which enables extensive and deep sequencing of the DNA (Londin et al., 2013), has recently been employed to detect novel mutations associated with many other types of corneal dystrophy (Zhang et al., 2019).
In view of the rapid proliferation of the genomic studies, many genetic banks, databases and web-based resources such as https://www.ncbi.nlm.nih.gov/gtr/ and https://www.omim.org/ have been created to capture and summarize the genomic association of a wide array of human diseases, including ocular diseases. The availability of these results not only help reduce unnecessary duplication of any previously conducted research, which often involves extensive time, effort and funding, but also expedite the discovery and development of new therapeutic targets via knowledge- and data-sharing.
2.4. Electronic health record-based registries and biobanks
The rapid emergence of EHR in healthcare systems in the recent years has allowed the capture and analysis of big data by the clinicians, researchers and relevant stakeholders (DesRoches et al., 2008; Day et al., 2015; Evans, 2016). One of the most notable examples in the field of ophthalmology is the Intelligent Research in Sight (IRIS). Registry, which is a US-based ophthalmic EHR registry established by the American Academy of Ophthalmology (Parke Ii et al., 2017; Chiang et al., 2018). In 2016, the IRIS Registry had already captured data from >17 million eye patients, including over a million of patients with dry eye disease (DED), and >35 million ophthalmic visits from 7,200 US-based ophthalmologists, providing valuable information on prevalence, demographic factors, risk factors, management and outcome of a wide range of ocular diseases (Chiang et al., 2018). So far, the IRIS Registry has enabled research in many fields of ophthalmology, including cornea (Anchouche et al., 2021), cataract (Pershing et al., 2020; Owen et al., 2021; Lacy et al., 2022), glaucoma (Chang et al., 2021; Olivier et al., 2021), and retina (Malhotra et al., 2021; Khanani et al., 2022). For instance, Anchouche et al. (2021) included >60,000 patients of chemical and thermal ocular injuries in the US (using the IRIS Registry data) and demonstrated a significant increase in the incidence by 20% from 2013 to 2017 in the US. In a similar vein, Donthineni et al. (2019) demonstrated the value of utilizing EHR-derived big data to estimate the incidence of DED in India as well as the predisposing factors such as age, gender, socio-economic status, and profession. Using the same EHR database of >2 million patients, Das and Basu (2022) were able to identify >20,000 patients who presented with epidemic keratoconjunctivitis and characterized the clinical features and outcomes, enabling more accurate and timely diagnosis and treatment.
Furthermore, there are nationwide databases such as the UK Biobank which also contain extensive clinical, imaging and genomic data related to the eye, including the cornea (Chua et al., 2019). UK Biobank is a large-scale biomedical database and research resource, which contains extensive health, genetic and bioimaging information from >500,000 people in the UK, with regularly update on additional follow-up data.5 Corneal hysteresis serves as an important biomechanical property of cornea, and it has been shown to influence the measurement of intraocular pressure and risk of glaucoma (Deol et al., 2015). Based on the data of >90,000 participants obtained from the UK Biobank, significant associations between corneal hysteresis and various demographic factors such as age, sex, and ethnicity were detected (Zhang et al., 2019). Khawaja et al. (2019) similarly identified five novel loci that are associated with corneal biomechanical properties, including corneal hysteresis and corneal resistance, which may have important implication on the pathogenesis of keratoconus. In addition, GWAS based on the UK Biobank data enabled the discovery of four novel genetic loci, including HERC2, LINC00340, NPLOC4, and ZC3H11B genes, for corneal astigmatism (Shah and Guggenheim, 2018).
3. Big data in cataract
Cataract is the leading cause of blindness and visual impairment globally, affecting around 94 million of the world population, particularly in the low- and middle-income countries (LMICs) (see text footnote 2) (Flaxman et al., 2017). Currently, ~20 million cases of cataract surgery are being performed each year (Wang et al., 2016), making it the most commonly performed surgery worldwide. In view of the continuous advancement in the phacoemulsification technology, surgical techniques, biometry calculation for IOL power, and IOL technology, the demand for perfect vision and no/minimal risk of surgical complication continues to rise (Erie, 2014; Ting D. S. J. et al., 2017; Sudhir et al., 2019; Day et al., 2020; Ting et al., 2020a). Furthermore, as cataract surgery is the most commonly performed ophthalmic surgery, it often used as the benchmark for assessing an ophthalmologist's surgical competence, especially during the specialist training or residency program.
To date, many national cataract databases have been established across the world (Table 3). One of the primary aims of these databases is to examine and audit the outcomes of the cataract surgery performed by the surgeons. Secondly, it also helps provide a benchmark for the visual outcome and safety of the surgery for all the cataract surgeons, with adjustment of the experience and complexity of the case-mix. In addition, these big data may also identify important factors that can predict the risk of intraoperative and postoperative complications, including posterior capsular rupture (PCR), retinal detachment, cystoid macular edema (CMO), endophthalmitis, and many others.
One of the most well-known examples is the Swedish National Cataract Register, which is the oldest nationwide cataract registry established in 1992 (Lundström et al., 2002). So far, it has produced >60 publications in the literature, covering many aspects of cataract surgery such as visual and refractive outcomes, posterior capsular rupture, endophthalmitis, postoperative practice pattern, and development of a composite risk-stratification scoring system, amongst others (Farhoudi et al., 2018; Zetterberg et al., 2021; Friling et al., 2022; Ridderskär et al., 2022). The European Registry of Quality Outcome for Cataract and Refractive Surgery (EUREQUO), which is supported by the ESCRS, represents another large-scale database that has so far captured more than 3 million cases of cataract surgery in Europe.6 This database provides pertinent surgical outcomes as well as the patient-reported outcomes following cataract and refractive surgeries, allowing the operating surgeons to audit their results and implement changes to their surgery (if required) to further improve the clinical outcomes. In addition, the big data obtained from this database (which included >2 million cases) has enabled effective analysis of risk factors for PCR and dropped nucleus during cataract surgery (Lundström et al., 2020; Segers et al., 2022).
In the UK, the Royal College of Ophthalmologists (RCOphth), UK, established the DHR-based National Ophthalmology Databases (NOD) in 2009, with an aim to monitor and improve the outcomes in cataract surgery and various ophthalmic conditions, including diabetic eye disease, age-related macular degeneration, glaucoma, and retinal detachment.7 In 2020, the NOD published the annual report on cataract surgery, which included >200,000 cataract surgery performed by >2,000 surgeons (web). The report highlighted that 86% of the eyes achieved at least 1 Snellen-line improvement in vision postoperatively. The overall PCR rate was 1.1%, with a higher rate (2.4%) in less experienced trainee surgeons and lower rate (0.9%) in consultant surgeons. Within the UK, all ophthalmic surgeons, including consultants and trainees, are required to record the number of cataract surgery performed and the rate of complications (particularly the rate of PCR), The findings from the NOD not only set important benchmarks for all the UK cataract surgeons but also help identify surgeons and trainees who require additional support and training on cataract surgery, particularly if the PCR rate is considerably higher than the national benchmark. These data have also been utilized to stratify the risk of PCR and vitreous loss, enabling the development of effective risk-stratification system to optimize patient selection and safety (Narendran et al., 2009; Day et al., 2015).
As mentioned above, IRIS Registry has showcased its clinical and research values in many fields of ophthalmology. Within the context of cataract surgery, Pershing et al. (2020) demonstrated a 0.04% rate of postoperative endophthalmitis among >8 million cases of cataract surgery and identified important predictive factors such as younger age, need for anterior vitrectomy, and when cataract surgery is combined with other ophthalmic surgeries. In addition, researchers were also able to utilize the IRIS Registry in analyzing and comparing the refractive outcomes and risk of endophthalmitis between immediate sequential and delayed sequential bilateral cataract surgery, which helps inform the clinical practice (Owen et al., 2021; Lacy et al., 2022).
In addition, the recent COVID-19 pandemic has caused an unprecedented surge in the service backlog and number of cases on the waiting list, particularly for cataract surgery (Ting et al., 2020b). With the availability of big data obtained from the EHR, it enables a comprehensive and systematic analysis of the utilization of the clinical and theater space, workflow efficiency (e.g., turnaround time between each cataract surgery), and supply-and-demand matching in terms of available workforce/resources and service backlog, which are useful for strategic planning and allocation of the resources within healthcare services. Big data from large-scale population-based studies also provide invaluable information on the service coverage and health equity (or inequity). For instance, effective cataract surgical coverage (eCSC) is often used as a measure to evaluate the service access to cataract surgery and the outcome of the surgery. A recent population-based study, based on 148 Rapid Assessment of Avoidable Blindness (RAAB) survey data from 55 countries involving ~500,000 adults aged 50 years and older, reported that eCSC varied considerably between countries, with higher rate in countries with greater income level, highlighting the need for increased efforts to improve access and quality of the surgery in under-resourced countries (McCormick et al., 2022).
4. Future directions
4.1. Integration of big data and artificial intelligence
The relationship between big data and AI-assisted technologies is highly synergistic and inextricably linked. The enormity and nature of big data usually require advanced computing power, software and algorithms (e.g., machine learning and deep learning-based AI algorithms) to process and analyze the data. On the other hand, development of highly accurate and generalizable AI algorithms often requires the input of big data that satisfy the attributed of “5 Vs”. With the rapid development of big data research and digital technologies in the recent years, it is anticipated that AI-power big data analytic platforms, coupled with telemedicine, will shape the future landscape of medicine (Sim et al., 2016; Ting et al., 2019a; Wu et al., 2019). The need for these innovative digital technologies in clinical practice was further heightened by the recent COVID-19 pandemic where all branches of healthcare services, including ophthalmology, have been severely impacted (Babu et al., 2020; Ting et al., 2020b,c, 2021f; Whitelaw et al., 2020; Ho et al., 2021; Kim et al., 2021).
So far, big data-driven AI technologies have demonstrated its clinical potential in many areas of corneal diseases and cataract. These encompass screening and diagnosing a wide array of conditions (e.g., keratoconus, IK, corneal opacity) and cataract, and preoperative planning for refractive surgery, to making automated clinical decisions for various diseases (Rampat et al., 2021). Studies have shown that the diagnostic accuracy of several AI algorithms can be as high as 92–97% in detecting keratoconus and preclinical keratoconus or forme fruste keratoconus (Arbelaez et al., 2012; Smadja et al., 2013; Hidalgo et al., 2016; Issarti et al., 2019; Lavric and Valentin, 2019; Ting et al., 2021b). Automated assessment of the corneal endothelial cell density in normal and diseased eyes as well as corneal guttata, based on AI-assisted algorithms using specular microscopy images and/or retroillumination slit-lamp photographs, have been developed to improve the management and follow-up in patients with corneal endothelial diseases and post-endothelial keratoplasty (Joseph et al., 2020; Vigueras-Guillén et al., 2020; Shilpashree et al., 2021; Soh et al., 2021; Karmakar et al., 2022). A recent study also reported the potential of machine learning algorithms in predicting the 10-year graft survival of PK and DSAEK using random survival forests analysis with highest variable importance factors (Ang et al., 2022). Understanding of the predictive factors allows the clinicians to address any modifiable preoperative factors, select the most appropriate type of keratoplasty for each individual patient, and optimize the long-term graft survival. This will also help reduce the need for regrafting, which has been shown as one of the most common indications for keratoplasty (Ting et al., 2012; Aboshiha et al., 2018).
In addition, several studies have demonstrated the ability of AI algorithms in diagnosing and differentiating the types of IK, and differentiating active IK from healed corneal scar (Liu et al., 2020; Lv et al., 2020; Koyama et al., 2021; Tiwari et al., 2022). These technologies are particularly useful in regions where resources and expertise are lacking. More recently, Li et al. (2020) reported the superior performance of a DL-based AI algorithm in diagnosing a wide range of corneal and conjunctival diseases, including IK, pterygium and conjunctivitis, and cataract based on using slit-lamp photographs. More importantly, the algorithm was able to provide automated clinical recommendation for further management, including clinical observation, medical treatment and surgical interventions. This can greatly reduce the diagnostic and referral time and improve the workflow efficiency within the healthcare system. For acute ocular conditions such as IK, timely diagnosis is crucial in achieving a good outcome; hence an effective AI-powered platform may serve as a novel diagnostic solution, particularly in LMICs.
Automated detection and grading of cataract as well as diagnosis of posterior capsular opacification by AI algorithms have also been described (Xu et al., 2020; Gutierrez et al., 2022). Furthermore, newer generations of IOL calculation formulae, based on big data-powered AI algorithms, have also been developed to enhance the predict accuracy of the IOL power (Ladas et al., 2021; Gutierrez et al., 2022). By using a dataset of ~5,000 patients, Li T. et al. (2022) recently demonstrated the ability of AI in enhancing the prediction of effective lens position and improving the accuracy of existing IOL formulas, including Haigis, Hoffer Q, Holladay, and SRK/T. Therefore, it is anticipated that the millions of data housed within several national cataract registries can be utilized to train and develop effect AI-based IOL calculation formulae and optimize the visual and refractive outcomes of cataract surgery in the near future.
4.2. Empowerment of big data research by internet of things, mHeath, and wearable devices
The potential of big data research has further been fueled by the recent rapid expansion and development in Internet of Things (IoT) technologies, mobile health (mHealth; a branch of medical and public health practice powered by mobile devices), and wearable devices (Kelly et al., 2020). So far, it has been estimated that more than 2 billion people own a mobile phone globally, with >50 million people utilizing app-based, interactive health self-care and self-triage (Millenson et al., 2018). The scopes and applications of mHealth range considerably from delivering health education, digital therapy, supporting clinical decision making for diagnosis and treatment, to improving clinical outcomes via behavioral modification (Rowland et al., 2020). Within the field of cornea, Inomata et al. (2020b) utilized crowdsourced big data, obtained from a mobile app (DryEyeRhythm) of around 3,000 participants, to identify participants with diagnosed and undiagnosed symptomatic DED and determine their associated risk factors. In addition, it was found that depressive symptoms are more common in individuals with DED, enabling an earlier detection and intervention for depression in this cohort of individuals (Inomata et al., 2020a).
Recent advances in wearable devices have also enabled real-time collection of millions of health datapoints (e.g., heart rate, blood pulse, step count, daily activity, etc.) for non-invasive diagnosis and monitoring of various diseases, including cardiovascular diseases, pulmonary diseases, hypertension, and diabetes, amongst others (Guk et al., 2019; Lu et al., 2020). Studies have shown that smart contact lenses (CLs) with biosensing technology are able to detect tear content and metabolites, including glucose and exosomes, which enable real-time non-invasive detection and monitoring of diabetes and cancer (Park et al., 2018; Li S. et al., 2022). The big data obtained from these biosensing technologies may be useful for CL wearers in the future where these smart CLs may help detect any early changes in the tear metabolites and inflammatory cytokines, which may help predict the risk of development of CL-induced DED, inflammatory and infectious keratitis.
In addition, Chen et al. (2021) recently developed a blink-sensing glasses to detect the blinking pattern between DED subjects and health controls. It has been recognized in the recent years that increased digital screen use (either for occupational, recreational or educational purpose) is a significant predisposing risk factor for DED (Mehra and Galor, 2020). Several mechanisms have been proposed, including reduced/abnormal blinking (which can lead to increased tear evaporation and ocular surface inflammation) and damage from the emitting light from the digital screen devices. Therefore, the big data generated from these blink-sensing glasses have the potential of monitoring the blinking patterns and behaviors of the digital screen users (or individuals with DED), which allows for an effective modification of the lifestyle and improves the management of DED.
4.3. Role of big data in predictive, preventive, personalized, and precision (P4) medicine
With the exponential increase in the availability of multi-omics data, large-scale population-based studies, EHR, and digital technologies, it is becoming possible to harness the power of these big data for implementing predictive, preventive, personalized, and participatory (P4) medicine. Instead of treating the patients reactively based on the presenting symptoms and signs, P4 medicine advocates personalizing the care to each individual at an individual molecular, cellular and organ levels, making the treatment more effective and cost-effective (Flores et al., 2013). Furthermore, the combination of real-time health data collected from these wearable smart devices with clinical and “multi-omics” data can potentially improve the understanding and management of certain multifactorial diseases (for instance, DED) where lifestyle and environmental factors play a vital role in the pathogenesis and phenotypic features of the disease (Inomata et al., 2020c).
Recently, Inomata et al. (2021) highlighted the potential of mHealth by using a data-driven multidimensional smartphone-based digital phenotyping strategy to assess and classify DED, which is a highly heterogeneous and multifactorial disease. A wide range of data, including demographics, medical history, lifestyle questions, blink sensing (via smartphone cameras and CIFaceFeature for facial detection), and daily subjective symptoms [using the Japanese version of the Ocular Surface Disease Index (J-OSDI)], were collected through the DryEyeRhythm mobile app. Subsequently, through hierarchical clustering heatmap, the authors were able to visualize and classify DED patients into several categories with distinct DED characteristics, illustrating the potential of P4 medicine in managing DED.
The potential of big data in shaping P4 medicine in ophthalmology is huge. For instance, with the increased availability of big data, it will be possible to personalize the choice of IOL for patients who are undergoing cataract surgery and predict those who are most likely to benefit from a certain type of IOL implant (e.g., multifocal vs. monofocal) in the future. One may also leverage the power of big data (and AI) to predict of the risk of postoperative complications following cataract surgery, which helps distinguish the group of patients who are suitable for community follow-up vs. hospital follow-up, thereby relieving the burden on the over-stretched healthcare services. Furthermore, with the combination of phenotypic and genotypic data as well as lifestyle factors (e.g., tendency for eye rubbing), it may be possible to predict the group of patients with keratoconus who are most likely to progress, thereby enabling early prophylactic intervention such as corneal cross-linking to prevent progression and maintain good vision.
4.4. Potential barriers for enabling big data research and its clinical potential
The potential values of big data, EHR, telehealth and mHealth in healthcare have long been recognized by the WHO, which was published as a report by the Global Observatory for eHealth in 2016 (World Health Organization, 2016). However, several barriers exist for translating their potential to real clinical values (Hulsen et al., 2019; Uslu and Stausberg, 2021). One of the main barriers is cost. Establishment and maintenance of these large-scale platforms, registries and EHR often requires substantial financial resources and workforce, which explains the scarcity of big data in under-resourced LMICs. Depending on the scale of the registries and EHR, they can cost the healthcare service from tens of thousands to multimillion dollars to set up and maintain the platforms within the healthcare services (Menachemi and Collum, 2011). Processing and analysis of big data also necessitates advanced computing power, facilities and expertise in order to arrive at clinically meaningful findings and conclusions. Furthermore, considerable expertise, workforce, resources, and facilities, all of which are associated with a high cost, need to be in place to prevent or reduce the risk of EHR system failure, which can significantly disrupt and paralyze the delivery of the healthcare services and cause harm to patients.
In addition to the cost and workforce, there remains a number of significant challenges associated with big data research, including data completeness, accuracy, heterogeneous data sources/platforms, data security, sharing, and visualization (Househ et al., 2017; Dash et al., 2019). Most large-scale EHR systems are designed for delivery of clinical services but not for evidence generation; therefore, capitalization of the EHR-derived big data for clinical research purposes is considerably challenged by the consistency, accuracy, and completeness of data. In addition, many of the systems are usually created for general medical and surgical services, which leads to inaccurate or incomplete data collection for ophthalmic diseases. For instance, many of the ophthalmic diagnoses/codes are not available in the general EHR systems, inhibiting an accurate assessment and analysis of the incidence, characteristics, causes, and impact of ophthalmic diseases presenting to the health services.
Another potential barrier for big data research is data privacy and sharing. Although there has been an increased availability of big data captured through various sources (Figure 1), processing of these data for research purposes are prohibited, unless: (1) the data are fully anonymized; (2) the data owner (i.e., the patients, healthy volunteers, etc.) provide “explicit consent”; or (3) the processing of data are necessary for provision of healthcare services or for public interest (Hulsen et al., 2019). In 2018, the European Union introduced a new set of regulations—the General Data Protection Regulation (GDPR)—to safeguard data privacy.8 It also places constraints on data sharing where appropriate consent needs to be obtained from the patient before the data can be shared with another organization. In addition, data sharing and management is further guided by the FAIR principles, which include “Findability,” “Accessibility,” “Interoperability,” and “Reusability” (Wilkinson et al., 2016). Therefore, to realize the potential of big data research in ophthalmology, all these highlighted barriers will need to be fully considered and addressed.
5. Conclusions
The continuous growth of IoT technologies, increased acceptability of mHealth, accessibility and affordability of mobile and wearable smart devices, and advancement in AI technologies in the coming years are likely to further expand the potential and applications of big data research in medicine and surgery, including ophthalmology (Wang et al., 2020; Rono et al., 2021; Dow et al., 2022). The establishment of big data resources such as corneal transplant registries, genomic studies, biobanks, and large scale EHR-based registries has so far provided a vast amount of valuable clinical and research information on cataract and a wide range of corneal diseases, ranging from non-sight threatening but functionally debilitating (e.g., DED) to sight threatening conditions (e.g., IK, PBK, keratoconus, etc.). Big data has advanced the understanding of many diseases, provided important benchmark for treatment and surgery, improved treatment outcome, and informed public policies. It is also anticipated that big data research will help propel the field of P4 medicine. However, there is currently a significant deficit and mismatch in the availability and demand for big data in LMICs, highlighting the need for increased effort and work to be invested in the under-resourced countries where blindness secondary to corneal opacity and cataract predominates (Pineda, 2015; Tan et al., 2015).
Author contributions
Study conceptualization and design: DSJT and MA. Data collection and drafting of initial manuscript: DSJT. Critical revision of manuscript: RD, DSWT, and MA. All authors contributed to the data interpretation, analysis, and final approval of manuscript.
Funding
DSJT acknowledges support from the Medical Research Council/Fight for Sight Clinical Research Fellowship (MR/T001674/1).
Conflict of interest
The authors declare that the research was conducted in the absence of any commercial or financial relationships that could be construed as a potential conflict of interest.
Publisher's note
All claims expressed in this article are solely those of the authors and do not necessarily represent those of their affiliated organizations, or those of the publisher, the editors and the reviewers. Any product that may be evaluated in this article, or claim that may be made by its manufacturer, is not guaranteed or endorsed by the publisher.
Footnotes
1. ^https://www.ibm.com/blogs/watson-health/the-5-vs-of-big-data/
2. ^https://www.who.int/news-room/fact-sheets/detail/blindness-and-visual-impairment
4. ^https://www.odt.nhs.uk/statistics-and-reports/
5. ^https://www.ukbiobank.ac.uk/
6. ^https://www.escrs.org/about-escrs/registries/eurequo/
7. ^https://www.nodaudit.org.uk/resources/publications-annual-report
References
Aboshiha, J., Jones, M. N. A., Hopkinson, C. L., and Larkin, D. F. P. (2018). Differential survival of penetrating and lamellar transplants in management of failed corneal grafts. JAMA Ophthalmol. 136, 859–865. doi: 10.1001/jamaophthalmol.2018.1515
Afshari, N. A., Igo, R. P. Jr., Morris, N. J., Stambolian, D., Sharma, S., Pulagam, V. L., et al. (2017). Genome-wide association study identifies three novel loci in Fuchs endothelial corneal dystrophy. Nat. Commun. 8, 14898. doi: 10.1038/ncomms14898
Anchouche, S., Hall, N., Bal, S., Dana, R., Elze, T., Miller, J. W., et al. (2021). Chemical and thermal ocular burns in the United States: an IRIS registry analysis. Ocul. Surf. 21, 345–347. doi: 10.1016/j.jtos.2021.03.008
Ang, M., He, F., Lang, S., Sabanayagam, C., Cheng, C. Y., Arundhati, A., et al. (2022). Machine learning to analyze factors associated with ten-year graft survival of keratoplasty for cornea endothelial disease. Front. Med. 9, 831352. doi: 10.3389/fmed.2022.831352
Ang, M., Htoon, H. M., Cajucom-Uy, H. Y., Tan, D., and Mehta, J. S. (2011). Donor and surgical risk factors for primary graft failure following Descemet's stripping automated endothelial keratoplasty in Asian eyes. Clin. Ophthalmol. 5, 1503–1508. doi: 10.2147/OPTH.S25973
Ang, M., Li, L., Chua, D., Wong, C., Htoon, H. M., Mehta, J. S., et al. (2014). Descemet's stripping automated endothelial keratoplasty with anterior chamber intraocular lenses: complications and 3-year outcomes. Br. J. Ophthalmol. 98, 1028–1032. doi: 10.1136/bjophthalmol-2013-304622
Ang, M., Lim, F., Htoon, H. M., Tan, D., and Mehta, J. S. (2016a). Visual acuity and contrast sensitivity following Descemet stripping automated endothelial keratoplasty. Br. J. Ophthalmol. 100, 307–311. doi: 10.1136/bjophthalmol-2015-306975
Ang, M., Mehta, J. S., Lim, F., Bose, S., Htoon, H. M., Tan, D., et al. (2012a). Endothelial cell loss and graft survival after Descemet's stripping automated endothelial keratoplasty and penetrating keratoplasty. Ophthalmology 119, 2239–2244. doi: 10.1016/j.ophtha.2012.06.012
Ang, M., Mehta, J. S., Sng, C. C., Htoon, H. M., and Tan, D. T. (2012b). Indications, outcomes, and risk factors for failure in tectonic keratoplasty. Ophthalmology 119, 1311–1319. doi: 10.1016/j.ophtha.2012.01.021
Ang, M., and Sng, C. C. A. (2018). Descemet membrane endothelial keratoplasty and glaucoma. Curr. Opin. Ophthalmol. 29, 178–184. doi: 10.1097/ICU.0000000000000454
Ang, M., Soh, Y., Htoon, H. M., Mehta, J. S., and Tan, D. (2016b). Five-year graft survival comparing descemet stripping automated endothelial keratoplasty and penetrating keratoplasty. Ophthalmology 123, 1646–1652. doi: 10.1016/j.ophtha.2016.04.049
Ang, M., Ting, D. S. J., Kumar, A., May, K. O., Htoon, H. M., Mehta, J. S., et al. (2020). Descemet membrane endothelial keratoplasty in asian eyes: intraoperative and postoperative complications. Cornea 39, 940–945. doi: 10.1097/ICO.0000000000002302
Ang, M., Wilkins, M. R., Mehta, J. S., and Tan, D. (2016c). Descemet membrane endothelial keratoplasty. Br. J. Ophthalmol. 100, 15–21. doi: 10.1136/bjophthalmol-2015-306837
Arbelaez, M. C., Versaci, F., Vestri, G., Barboni, P., and Savini, G. (2012). Use of a support vector machine for keratoconus and subclinical keratoconus detection by topographic and tomographic data. Ophthalmology 119, 2231–2238. doi: 10.1016/j.ophtha.2012.06.005
Asbell, P. A., Sanfilippo, C. M., Sahm, D. F., and DeCory, H. H. (2020). Trends in antibiotic resistance among ocular microorganisms in the United States from 2009 to 2018. JAMA Ophthalmol. 138, 439–450. doi: 10.1001/jamaophthalmol.2020.0155
Babu, N., Kohli, P., Mishra, C., Sen, S., Arthur, D., Chhablani, D., et al. (2020). To evaluate the effect of COVID-19 pandemic and national lockdown on patient care at a tertiary-care ophthalmology institute. Indian J. Ophthalmol. 68, 1540–1544. doi: 10.4103/ijo.IJO_1673_20
Baratz, K. H., Tosakulwong, N., Ryu, E., Brown, W. L., Branham, K., Chen, W., et al. (2010). E2-2 protein and Fuchs's corneal dystrophy. N. Engl. J. Med. 363, 1016–1024. doi: 10.1056/NEJMoa1007064
Bose, S., Ang, M., Mehta, J. S., Tan, D. T., and Finkelstein, E. (2013). Cost-effectiveness of descemet's stripping endothelial keratoplasty versus penetrating keratoplasty. Ophthalmology 120, 464–470. doi: 10.1016/j.ophtha.2012.08.024
Burdon, K. P., Macgregor, S., Bykhovskaya, Y., Javadiyan, S., Li, X., Laurie, K. J., et al. (2011). Association of polymorphisms in the hepatocyte growth factor gene promoter with keratoconus. Invest. Ophthalmol. Vis. Sci. 52, 8514–8519. doi: 10.1167/iovs.11-8261
Bykhovskaya, Y., Li, X., Epifantseva, I., Haritunians, T., Siscovick, D., Aldave, A., et al. (2012). Variation in the lysyl oxidase (LOX) gene is associated with keratoconus in family-based and case-control studies. Invest. Ophthalmol. Vis. Sci. 53, 4152–4157. doi: 10.1167/iovs.11-9268
Cariello, A. J., Passos, R. M., Yu, M. C., and Hofling-Lima, A. L. (2011). Microbial keratitis at a referral center in Brazil. Int. Ophthalmol. 31, 197–204. doi: 10.1007/s10792-011-9441-0
Chang, T. C., Parrish, R. K., Fujino, D., Kelly, S. P., and Vanner, E. A. (2021). Factors associated with favorable laser trabeculoplasty response: IRIS registry analysis. Am. J. Ophthalmol. 223, 149–158. doi: 10.1016/j.ajo.2020.10.004
Chen, J. Y., Jones, M. N., Srinivasan, S., Neal, T. J., Armitage, W. J., Kaye, S. B., et al. (2015). Endophthalmitis after penetrating keratoplasty. Ophthalmology 122, 25–30. doi: 10.1016/j.ophtha.2014.07.038
Chen, R., Zhang, Z., Deng, K., Wang, D., Ke, H., Cai, L., et al. (2021). Blink-sensing glasses: a flexible iontronic sensing wearable for continuous blink monitoring. iScience 24, 102399. doi: 10.1016/j.isci.2021.102399
Cheng, C. Y., Soh, Z. D., Majithia, S., Thakur, S., Rim, T. H., Tham, Y. C., et al. (2020). Big data in ophthalmology. Asia Pac. J. Ophthalmol. 9, 291–298. doi: 10.1097/APO.0000000000000304
Chiang, M. F., Sommer, A., Rich, W. L., Lum, F., and Parke, D. W. (2018). 2nd. The 2016 American Academy of Ophthalmology IRIS(®) Registry (Intelligent Research in Sight) Database: characteristics and methods. Ophthalmology 125, 1143–8. doi: 10.1016/j.ophtha.2017.12.001
Chua, S. Y. L., Thomas, D., Allen, N., Lotery, A., Desai, P., Patel, P., et al. (2019). Cohort profile: design and methods in the eye and vision consortium of UK Biobank. BMJ Open 9, e025077. doi: 10.1136/bmjopen-2018-025077
Coster, D. J., Lowe, M. T., Keane, M. C., and Williams, K. A. A. (2014). comparison of lamellar and penetrating keratoplasty outcomes: a registry study. Ophthalmology 121, 979–987. doi: 10.1016/j.ophtha.2013.12.017
Czugala, M., Karolak, J. A., Nowak, D. M., Polakowski, P., Pitarque, J., Molinari, A., et al. (2012). Novel mutation and three other sequence variants segregating with phenotype at keratoconus 13q32 susceptibility locus. Eur. J. Hum. Genet. 20, 389–397. doi: 10.1038/ejhg.2011.203
Das, A. V., and Basu, S. (2022). Epidemic keratoconjunctivitis in India: electronic medical records-driven big data analytics report IV. Br. J. Ophthalmol. 106, 331–335. doi: 10.1136/bjophthalmol-2020-317330
Dash, S., Shakyawar, S. K., Sharma, M., and Kaushik, S. (2019). Big data in healthcare: management, analysis and future prospects. J. Big Data 6, 54. doi: 10.1186/s40537-019-0217-0
Day, A. C., Burr, J. M., Bennett, K., Bunce, C., Doré, C. J., Rubin, G. S., et al. (2020). Femtosecond laser-assisted cataract surgery versus phacoemulsification cataract surgery (FACT): a randomized noninferiority trial. Ophthalmology 127, 1012–1019. doi: 10.1016/j.ophtha.2020.02.028
Day, A. C., Donachie, P. H., Sparrow, J. M., and Johnston, R. L. (2015). The Royal College of Ophthalmologists' National Ophthalmology Database study of cataract surgery: report 1, visual outcomes and complications. Eye 29, 552–560. doi: 10.1038/eye.2015.3
Deol, M., Taylor, D. A., and Radcliffe, N. M. (2015). Corneal hysteresis and its relevance to glaucoma. Curr. Opin. Ophthalmol. 26, 96–102. doi: 10.1097/ICU.0000000000000130
DesRoches, C. M., Campbell, E. G., Rao, S. R., Donelan, K., Ferris, T. G., Jha, A., et al. (2008). Electronic health records in ambulatory care–a national survey of physicians. N. Engl. J. Med. 359, 50–60. doi: 10.1056/NEJMsa0802005
Donthineni, P. R., Kammari, P., Shanbhag, S. S., Singh, V., Das, A. V., Basu, S., et al. (2019). Incidence, demographics, types and risk factors of dry eye disease in India: electronic medical records driven big data analytics report I. Ocul. Surf. 17, 250–256. doi: 10.1016/j.jtos.2019.02.007
Dow, E. R., Keenan, T. D. L., Lad, E. M., Lee, A. Y., Lee, C. S., and Loewenstein, A. (2022). From data to deployment: the collaborative community on ophthalmic imaging roadmap for artificial intelligence in age-related macular degeneration. Opthalmology 129:e43–e59. doi: 10.1016/j.ophtha.2022.01.002
Dudakova, L., Palos, M., Jirsova, K., Stranecky, V., Krepelova, A., Hysi, P. G., et al. (2015). Validation of rs2956540:G>C and rs3735520:G>A association with keratoconus in a population of European descent. Eur. J. Hum. Genet. 23, 1581–1583. doi: 10.1038/ejhg.2015.28
Dunker, S. L., Armitage, W. J., Armitage, M., Brocato, L., Figueiredo, F. C., Heemskerk, M. B. A., et al. (2021). Practice patterns of corneal transplantation in Europe: first report by the European Cornea and Cell Transplantation Registry. J. Cataract Refract. Surg. 47, 865–869. doi: 10.1097/j.jcrs.0000000000000520
Edelstein, S. L., DeMatteo, J., Stoeger, C. G., Macsai, M. S., and Wang, C-. H. (2016). Report of the Eye Bank Association of America medical review subcommittee on adverse reactions reported from 2007 to 2014. Cornea 35, 917–926. doi: 10.1097/ICO.0000000000000869
Erie, J. C. (2014). Rising cataract surgery rates: demand and supply. Ophthalmology 121, 2–4. doi: 10.1016/j.ophtha.2013.10.002
Evans, R. S. (2016). Electronic health records: then, now, and in the future. Yearb. Med. Inform. (Suppl. 1), S48–61. doi: 10.15265/IYS-2016-s006
Farhoudi, D. B., Behndig, A., Montan, P., Lundström, M., Zetterström, C., Kugelberg, M., et al. (2018). Spectacle use after routine cataract surgery: a study from the Swedish National Cataract Register. Acta Ophthalmol. 96, 283–287. doi: 10.1111/aos.13554
Flaxman, S. R., Bourne, R. R. A., Resnikoff, S., Ackland, P., Braithwaite, T., Cicinelli, M. V., et al. (2017). Global causes of blindness and distance vision impairment 1990-2020: a systematic review and meta-analysis. Lancet Glob. Health.5, e1221–e1234. doi: 10.1016/S2214-109X(17)30393-5
Flores, M., Glusman, G., Brogaard, K., Price, N. D., and Hood, L. (2013). P4 medicine: how systems medicine will transform the healthcare sector and society. Per. Med. 10, 565–576. doi: 10.2217/pme.13.57
Friling, E., Johansson, B., Lundström, M., and Montan, P. (2022). Postoperative endophthalmitis in immediate sequential bilateral cataract surgery: a nationwide registry study. Ophthalmology 129, 26–34. doi: 10.1016/j.ophtha.2021.07.007
Fuest, M., Ang, M., Htoon, H. M., Tan, D., and Mehta, J. S. (2017). Long-term visual outcomes comparing Descemet stripping automated endothelial keratoplasty and penetrating keratoplasty. Am. J. Ophthalmol. 182, 62–71. doi: 10.1016/j.ajo.2017.07.014
Gain, P., Jullienne, R., He, Z., Aldossary, M., Acquart, S., Cognasse, F., et al. (2016). Global survey of corneal transplantation and eye banking. JAMA Ophthalmol. 134, 167–173. doi: 10.1001/jamaophthalmol.2015.4776
Ganguly, S., Salma, K. C., Kansakar, I., Sharma, M., Bastola, P., Pradhan, R., et al. (2011). Pattern of fungal isolates in cases of corneal ulcer in the western periphery of Nepal. Nepal J. Ophthalmol. 3, 118–122. doi: 10.3126/nepjoph.v3i2.5262
Gauthier, A. S., Castelbou, M., Garnier, M. B., Pizzuto, J., Roux, S., Gain, P., et al. (2017). Corneal transplantation: study of the data of a regional eye bank for the year 2013 and analysis of the evolution of the adverse events reported in France since 2010. Cell Tissue Bank 18, 83–89. doi: 10.1007/s10561-016-9593-2
Gogia, V., Gupta, S., Agarwal, T., Pandey, V., and Tandon, R. (2015). Changing pattern of utilization of human donor cornea in India. Indian J. Ophthalmol. 63, 654–658. doi: 10.4103/0301-4738.169790
Green, M., Carnt, N., Apel, A., and Stapleton, F. (2019). Queensland microbial keratitis database: 2005–2015. Br. J. Ophthalmol. 103, 1481–1486. doi: 10.1136/bjophthalmol-2018-312881
Guk, K., Han, G., Lim, J., Jeong, K., Kang, T., Lim, E. K., et al. (2019). Evolution of wearable devices with real-time disease monitoring for personalized healthcare. Nanomaterials. 9, 813. doi: 10.3390/nano9060813
Gupta, N., Vashist, P., Ganger, A., Tandon, R., and Gupta, S. K. (2018). Eye donation and eye banking in India. Natl. Med. J. India 31, 283–286. doi: 10.4103/0970-258X.261189
Gupta, N., Vashist, P., Tandon, R., Gupta, S. K., Kalaivani, M., Dwivedi, S. N., et al. (2017). Use of traditional eye medicine and self-medication in rural India: a population-based study. PLoS ONE 12, e0183461. doi: 10.1371/journal.pone.0183461
Gutierrez, L., Lim, J. S., Foo, L. L., Ng, W. Y. Y., Yip, M., Lim, G. Y. S., et al. (2022). Application of artificial intelligence in cataract management: current and future directions. Eye Vis. 9, 3. doi: 10.1186/s40662-021-00273-z
Haleem, A., Javaid, M., Khan, I. H., and Vaishya, R. (2020). Significant applications of big data in COVID-19 pandemic. Ind. J. Orthop. 54, 1–3. doi: 10.1007/s43465-020-00129-z
Hardcastle, A. J., Liskova, P., Bykhovskaya, Y., McComish, B. J., Davidson, A. E., Inglehearn, C. F., et al. (2021). A multi-ethnic genome-wide association study implicates collagen matrix integrity and cell differentiation pathways in keratoconus. Commun. Biol. 4, 266. doi: 10.1038/s42003-021-01784-0
Hidalgo, I. R., Rodriguez, P., Rozema, J. J., Dhubhghaill, S. N., Zakaria, N., Tassignon, M. J., et al. (2016). Evaluation of a machine-learning classifier for keratoconus detection based on scheimpflug tomography. Cornea 35, 827–832. doi: 10.1097/ICO.0000000000000834
Ho, C. S., Avery, A. J., Livingstone, I. A. T., and Ting, D. S. J. (2021). Virtual consultation for red eye. BMJ 373, n1490. doi: 10.1136/bmj.n1490
Househ, M. S., Aldosari, B., Alanazi, A., Kushniruk, A. W., and Borycki, E. M. (2017). Big data, big problems: a healthcare perspective. Stud. Health Technol. Inform. 238, 36–39.
Hulsen, T., Jamuar, S. S., Moody, A. R., Karnes, J. H., Varga, O., Hedensted, S., et al. (2019). From big data to precision medicine. Front. Med. 6, 34. doi: 10.3389/fmed.2019.00034
Iliff, B. W., Riazuddin, S. A., and Gottsch, J. D. (2012). The genetics of Fuchs' corneal dystrophy. Exp. Rev. Ophthalmol. 7, 363–375. doi: 10.1586/eop.12.39
Inomata, T., Iwagami, M., Nakamura, M., Shiang, T., Fujimoto, K., Okumura, Y., et al. (2020a). Association between dry eye and depressive symptoms: large-scale crowdsourced research using the DryEyeRhythm iPhone application. Ocul. Surf. 18, 312–319. doi: 10.1016/j.jtos.2020.02.007
Inomata, T., Iwagami, M., Nakamura, M., Shiang, T., Yoshimura, Y., Fujimoto, K., et al. (2020b). Characteristics and risk factors associated with diagnosed and undiagnosed symptomatic dry eye using a smartphone application. JAMA Ophthalmol. 138, 58–68. doi: 10.1001/jamaophthalmol.2019.4815
Inomata, T., Nakamura, M., Sung, J., Midorikawa-Inomata, A., Iwagami, M., Fujio, K., et al. (2021). Smartphone-based digital phenotyping for dry eye toward P4 medicine: a crowdsourced cross-sectional study. NPJ Digit Med. 4, 171. doi: 10.1038/s41746-021-00540-2
Inomata, T., Sung, J., Nakamura, M., Iwagami, M., Okumura, Y., Iwata, N., et al. (2020c). Using medical big data to develop personalized medicine for dry eye disease. Cornea 39(Suppl. 1), S39–S46. doi: 10.1097/ICO.0000000000002500
Issarti, I., Consejo, A., Jiménez-García, M., Hershko, S., Koppen, C., Rozema, J. J., et al. (2019). Computer aided diagnosis for suspect keratoconus detection. Comput. Biol. Med. 109, 33–42. doi: 10.1016/j.compbiomed.2019.04.024
Joseph, N., Kolluru, C., Benetz, B. A. M., Menegay, H. J., Lass, J. H., Wilson, D. L., et al. (2020). Quantitative and qualitative evaluation of deep learning automatic segmentations of corneal endothelial cell images of reduced image quality obtained following cornea transplant. J. Med. Imaging 7, 014503. doi: 10.1117/1.JMI.7.1.014503
Kaliamurthy, J., Kalavathy, C. M., Parmar, P., Nelson Jesudasan, C. A., and Thomas, P. A. (2013). Spectrum of bacterial keratitis at a tertiary eye care centre in India. Biomed Res. Int. 2013, 181564. doi: 10.1155/2013/181564
Karmakar, R., Nooshabadi, S., and Eghrari, A. (2022). An automatic approach for cell detection and segmentation of corneal endothelium in specular microscope. Graefes Arch. Clin. Exp. Ophthalmol. 260, 1215–1224. doi: 10.1007/s00417-021-05483-8
Karolak, J. A., and Gajecka, M. (2017). Genomic strategies to understand causes of keratoconus. Mol. Genet. Genom. 292, 251–269. doi: 10.1007/s00438-016-1283-z
Kaye, S., Tuft, S., Neal, T., Tole, D., Leeming, J., Figueiredo, F., et al. (2010). Bacterial susceptibility to topical antimicrobials and clinical outcome in bacterial keratitis. Invest. Ophthalmol. Vis. Sci. 51, 362–368. doi: 10.1167/iovs.09-3933
Keay, L. J., Gower, E. W., Iovieno, A., Oechsler, R. A., Alfonso, E. C., Matoba, A., et al. (2011). Clinical and microbiological characteristics of fungal keratitis in the United States, 2001-2007: a multicenter study. Ophthalmology 118, 920–926. doi: 10.1016/j.ophtha.2010.09.011
Keenan, T. D., Jones, M. N., Rushton, S., and Carley, F. M. (2012). Trends in the indications for corneal graft surgery in the United Kingdom: 1999 through 2009. Arch. Ophthalmol. 130, 621–628. doi: 10.1001/archophthalmol.2011.2585
Kelly, J. T., Campbell, K. L., Gong, E., and Scuffham, P. (2020). The internet of things: impact and implications for health care delivery. J. Med. Int. Res. 22, e20135. doi: 10.2196/20135
Khanani, A. M., Zarbin, M. A., Barakat, M. R., Albini, T. A., Kaiser, P. K., Guruprasad, B., et al. (2022). Safety outcomes of brolucizumab in neovascular age-related macular degeneration: results from the IRIS registry and Komodo healthcare map. JAMA Ophthalmol. 140, 20–28. doi: 10.1001/jamaophthalmol.2021.4585
Khawaja, A. P., Rojas Lopez, K. E., Hardcastle, A. J., Hammond, C. J., Liskova, P., Davidson, A. E., et al. (2019). Genetic variants associated with corneal biomechanical properties and potentially conferring susceptibility to keratoconus in a genome-wide association study. JAMA Ophthalmol. 137, 1005–1012. doi: 10.1001/jamaophthalmol.2019.2058
Khoo, P., Cabrera-Aguas, M. P., Nguyen, V., Lahra, M. M., and Watson, S. L. (2020). Microbial keratitis in Sydney, Australia: risk factors, patient outcomes, and seasonal variation. Graefes Arch. Clin. Exp. Ophthalmol. 258, 1745–1755. doi: 10.1007/s00417-020-04681-0
Khor, W. B., Prajna, V. N., Garg, P., Mehta, J. S., Xie, L., Liu, Z., et al. (2018). The Asia Cornea Society Infectious Keratitis Study: a prospective multicenter study of infectious keratitis in Asia. Am. J. Ophthalmol. 195, 161–170. doi: 10.1016/j.ajo.2018.07.040
Kim, S. E., Logeswaran, A., Kang, S., Stanojcic, N., Wickham, L., Thomas, P., et al. (2021). Digital transformation in ophthalmic clinical care during the COVID-19 pandemic. Asia Pac. J. Ophthalmol. 10, 381–387. doi: 10.1097/APO.0000000000000407
Kinoshita, S., Koizumi, N., Ueno, M., Okumura, N., Imai, K., Tanaka, H., et al. (2018). Injection of cultured cells with a ROCK inhibitor for bullous keratopathy. N. Engl. J. Med. 378, 995–1003. doi: 10.1056/NEJMoa1712770
Kowalski, R. P., Nayyar, S. V., Romanowski, E. G., Shanks, R. M. Q., Mammen, A., Dhaliwal, D. K., et al. (2020). The prevalence of bacteria, fungi, viruses, and acanthamoeba from 3,004 cases of keratitis, endophthalmitis, and conjunctivitis. Eye Contact Lens 46, 265–268. doi: 10.1097/ICL.0000000000000642
Koyama, A., Miyazaki, D., Nakagawa, Y., Ayatsuka, Y., Miyake, H., Ehara, F., et al. (2021). Determination of probability of causative pathogen in infectious keratitis using deep learning algorithm of slit-lamp images. Sci. Rep. 11, 22642. doi: 10.1038/s41598-021-02138-w
Lacy, M., Kung, T. H., Owen, J. P., Yanagihara, R. T., Blazes, M., Pershing, S., et al. (2022). endophthalmitis rate in immediately sequential versus delayed sequential bilateral cataract surgery within the intelligent research in sight (IRIS®) registry data. Ophthalmology 129, 129–138. doi: 10.1016/j.ophtha.2021.07.008
Ladas, J., Ladas, D., Lin, S. R., Devgan, U., Siddiqui, A. A., Jun, A. S., et al. (2021). Improvement of multiple generations of intraocular lens calculation formulae with a novel approach using artificial intelligence. Transl. Vis. Sci. Technol. 10, 7. doi: 10.1167/tvst.10.3.7
Lalitha, P., Manoharan, G., Karpagam, R., Prajna, N. V., Srinivasan, M., Mascarenhas, J., et al. (2017). Trends in antibiotic resistance in bacterial keratitis isolates from South India. Br. J. Ophthalmol. 101, 108–113. doi: 10.1136/bjophthalmol-2016-308487
Lavric, A., and Valentin, P. (2019). KeratoDetect: keratoconus detection algorithm using convolutional neural networks. Comput. Intell. Neurosci. 2019, 8162567. doi: 10.1155/2019/8162567
Li, J. O., Liu, H., Ting, D. S. J., Jeon, S., Chan, R. V. P., Kim, J. E., et al. (2021). Digital technology, tele-medicine and artificial intelligence in ophthalmology: a global perspective. Prog. Retin. Eye Res. 82, 100900. doi: 10.1016/j.preteyeres.2020.100900
Li, S., Zhu, Y., Haghniaz, R., Kawakita, S., Guan, S., Chen, J., et al. (2022). A microchambers containing contact lens for the noninvasive detection of tear exosomes. Adv. Funct. Mater. 32, 2206620. doi: 10.1002/adfm.202206620
Li, T., Stein, J., and Nallasamy, N. (2022). AI-powered effective lens position prediction improves the accuracy of existing lens formulas. Br. J. Ophthalmol. 106, 1222–1226. doi: 10.1136/bjophthalmol-2020-318321
Li, W., Yang, Y., Zhang, K., Long, E., He, L., Zhang, L., et al. (2020). Dense anatomical annotation of slit-lamp images improves the performance of deep learning for the diagnosis of ophthalmic disorders. Nat. Biomed. Eng. 4, 767–777. doi: 10.1038/s41551-020-0577-y
Li, X., Bykhovskaya, Y., Canedo, A. L., Haritunians, T., Siscovick, D., Aldave, A. J., et al. (2013). Genetic association of COL5A1 variants in keratoconus patients suggests a complex connection between corneal thinning and keratoconus. Invest. Ophthalmol. Vis. Sci. 54, 2696–2704. doi: 10.1167/iovs.13-11601
Lin, L., Duan, F., Yang, Y., Lou, B., Liang, L., Lin, X., et al. (2019). Nine-year analysis of isolated pathogens and antibiotic susceptibilities of microbial keratitis from a large referral eye center in southern China. Infect. Drug Resist. 12, 1295–1302. doi: 10.2147/IDR.S206831
Lin, L., Lan, W., Lou, B., Ke, H., Yang, Y., Lin, X., et al. (2017). Genus distribution of bacteria and fungi associated with keratitis in a large eye center located in Southern China. Ophthal. Epidemiol. 24, 90–96. doi: 10.1080/09286586.2016.1254250
Liu, Z., Cao, Y., Li, Y., Xiao, X., Qiu, Q., Yang, M., et al. (2020). Automatic diagnosis of fungal keratitis using data augmentation and image fusion with deep convolutional neural network. Comput. Methods Progr. Biomed. 187, 105019. doi: 10.1016/j.cmpb.2019.105019
Londin, E., Yadav, P., Surrey, S., Kricka, L. J., and Fortina, P. (2013). Use of linkage analysis, genome-wide association studies, and next-generation sequencing in the identification of disease-causing mutations. Methods Mol. Biol. 1015, 127–146. doi: 10.1007/978-1-62703-435-7_8
Lu, L., Zhang, J., Xie, Y., Gao, F., Xu, S., Wu, X., et al. (2020). Wearable health devices in health care: narrative systematic review. JMIR Mhealth Uhealth 8, e18907. doi: 10.2196/18907
Lu, Y., Vitart, V., Burdon, K. P., Khor, C. C., Bykhovskaya, Y., Mirshahi, A., et al. (2013). Genome-wide association analyses identify multiple loci associated with central corneal thickness and keratoconus. Nat. Genet. 45, 155–163. doi: 10.1038/ng.2506
Lundström, M., Dickman, M., Henry, Y., Manning, S., Rosen, P., Tassignon, M. J., et al. (2020). Risk factors for dropped nucleus in cataract surgery as reflected by the European Registry of Quality Outcomes for Cataract and Refractive Surgery. J. Cataract Refract. Surg. 46, 287–292. doi: 10.1097/j.jcrs.0000000000000019
Lundström, M., Stenevi, U., and Thorburn, W. (2002). The Swedish National Cataract Register: a 9-year review. Acta Ophthalmol. Scand. 80, 248–257. doi: 10.1034/j.1600-0420.2002.800304.x
Lv, J., Zhang, K., Chen, Q., Chen, Q., Huang, E., Cui, L., et al. (2020). Deep learning-based automated diagnosis of fungal keratitis with in vivo confocal microscopy images. Ann. Transl. Med. 8, 706. doi: 10.21037/atm.2020.03.134
Malhotra, N. A., Greenlee, T. E., Iyer, A. I., Conti, T. F., Chen, A. X., Singh, R. P., et al. (2021). Racial, ethnic, and insurance-based disparities upon initiation of anti-vascular endothelial growth factor therapy for diabetic macular edema in the US. Ophthalmology 128, 1438–1447. doi: 10.1016/j.ophtha.2021.03.010
Mallappallil, M., Sabu, J., Gruessner, A., and Salifu, M. A. (2020). review of big data and medical research. SAGE Open Med. 8, 2050312120934839. doi: 10.1177/2050312120934839
McComish, B. J., Sahebjada, S., Bykhovskaya, Y., Willoughby, C. E., Richardson, A. J., Tenen, A., et al. (2019). Association of genetic variation with keratoconus. JAMA Ophthalmol. 138, 174–181. doi: 10.1001/jamaophthalmol.2019.5293
McCormick, I., Butcher, R., Evans, J. R., Mactaggart, I. Z., Limburg, H., Jolley, E., et al. (2022). Effective cataract surgical coverage in adults aged 50 years and older: estimates from population-based surveys in 55 countries. Lancet Glob. Health 10, e1744–e1753. doi: 10.1016/S2214-109X(22)00419-3
Mehra, D., and Galor, A. (2020). Digital screen use and dry eye: a review. Asia Pac. J. Ophthalmol. 9, 491–497. doi: 10.1097/APO.0000000000000328
Mehta, P., Petersen, C. A., Wen, J. C., Banitt, M. R., Chen, P. P., Bojikian, K. D., et al. (2021). Automated detection of glaucoma with interpretable machine learning using clinical data and multimodal retinal images. Am. J. Ophthalmol. 231, 154–169. doi: 10.1016/j.ajo.2021.04.021
Menachemi, N., and Collum, T. H. (2011). Benefits and drawbacks of electronic health record systems. Risk Manag. Healthc. Policy 4, 47–55. doi: 10.2147/RMHP.S12985
Milea, D., Najjar, R. P., Zhubo, J., Ting, D., Vasseneix, C., Xu, X., et al. (2020). Artificial intelligence to detect papilledema from ocular fundus photographs. N. Engl. J. Med. 382, 1687–1695. doi: 10.1056/NEJMoa1917130
Millenson, M. L., Baldwin, J. L., Zipperer, L., and Singh, H. (2018). Beyond Dr. Google: the evidence on consumer-facing digital tools for diagnosis. Diagnosis 5, 95–105. doi: 10.1515/dx-2018-0009
Mooney, S. J., Westreich, D. J., and El-Sayed, A. M. (2015). Commentary: epidemiology in the era of big data. Epidemiology 26, 390–394. doi: 10.1097/EDE.0000000000000274
Narendran, N., Jaycock, P., Johnston, R. L., Taylor, H., Adams, M., Tole, D. M., et al. (2009). The Cataract National Dataset electronic multicentre audit of 55,567 operations: risk stratification for posterior capsule rupture and vitreous loss. Eye 23, 31–37. doi: 10.1038/sj.eye.6703049
Olivier, M. M. G., Smith, O. U., Croteau-Chonka, C. C., VanderBeek, B. L., Maguire, M. G., Lum, F., et al. (2021). Demographic and clinical characteristics associated with minimally invasive glaucoma surgery use: an intelligent research in sight (IRIS®) registry retrospective cohort analysis. Ophthalmology 128, 1292–1299. doi: 10.1016/j.ophtha.2021.02.012
Owen, J. P., Blazes, M., Lacy, M., Yanagihara, R. T., Van Gelder, R. N., Lee, A. Y., et al. (2021). Refractive outcomes after immediate sequential vs delayed sequential bilateral cataract surgery. JAMA Ophthalmol. 139, 876–885. doi: 10.1001/jamaophthalmol.2021.2032
Park, C. Y., Lee, J. K., Gore, P. K., Lim, C. Y., and Chuck, R. S. (2015). Keratoplasty in the United States: a 10-year review from 2005 through 2014. Ophthalmology 122, 2432–2442. doi: 10.1016/j.ophtha.2015.08.017
Park, J., Kim, J., Kim, S. Y., Cheong, W. H., Jang, J., and Park, Y. G. (2018) Soft, smart contact lenses with integrations of wireless circuits, glucose sensors, displays. Sci. Adv. 4:eaap9841. doi: 10.1126/sciadv.aap9841.
Parke Ii, D. W., Lum, F., and Rich, W. L. (2017). The IRIS® registry: purpose and perspectives. Ophthalmologe 114, 1–6. doi: 10.1007/s00347-016-0265-1
Peng, M. Y., Cevallos, V., McLeod, S. D., Lietman, T. M., and Rose-Nussbaumer, J. (2018). Bacterial keratitis: isolated organisms and antibiotic resistance patterns in San Francisco. Cornea 37, 84–87. doi: 10.1097/ICO.0000000000001417
Pershing, S., Lum, F., Hsu, S., Kelly, S., Chiang, M. F., Rich, W. L., et al. (2020). Endophthalmitis after cataract surgery in the United States: a report from the intelligent research in sight registry, 2013-2017. Ophthalmology 127, 151–8. doi: 10.1016/j.ophtha.2019.08.026
Pineda, R. (2015). Corneal transplantation in the developing world: lessons learned and meeting the challenge. Cornea 34(Suppl. 10), S35–S40. doi: 10.1097/ICO.0000000000000567
Porth, J. M., Deiotte, E., Dunn, M., and Bashshur, R. A. (2019). Review of the literature on the global epidemiology of corneal blindness. Cornea 38, 1602–1609. doi: 10.1097/ICO.0000000000002122
Rampat, R., Deshmukh, R., Chen, X., Ting, D. S. W., Said, D. G., Dua, H. S., et al. (2021). Artificial intelligence in cornea, refractive surgery, and cataract: basic principles, clinical applications, and future directions. Asia Pac. J. Ophthalmol. 10, 268–281. doi: 10.1097/APO.0000000000000394
Riazuddin, S. A., Eghrari, A. O., Al-Saif, A., Davey, L., Meadows, D. N., Katsanis, N., et al. (2009). Linkage of a mild late-onset phenotype of Fuchs corneal dystrophy to a novel locus at 5q33.1-q35.2. Invest. Ophthalmol. Vis. Sci. 50, 5667–5671. doi: 10.1167/iovs.09-3764
Riazuddin, S. A., Vasanth, S., Katsanis, N., and Gottsch, J. D. (2013). Mutations in AGBL1 cause dominant late-onset Fuchs corneal dystrophy and alter protein-protein interaction with TCF4. Am. J. Hum. Genet. 93, 758–764. doi: 10.1016/j.ajhg.2013.08.010
Riazuddin, S. A., Zaghloul, N. A., Al-Saif, A., Davey, L., Diplas, B. H., Meadows, D. N., et al. (2010). Missense mutations in TCF8 cause late-onset Fuchs corneal dystrophy and interact with FCD4 on chromosome 9p. Am. J. Hum. Genet. 86, 45–53. doi: 10.1016/j.ajhg.2009.12.001
Ridderskär, L., Montan, P., Kugelberg, M., Nilsson, I., Lundström, M., Behndig, A., et al. (2022). Outcome of cataract surgery in eyes with diabetic retinopathy: a Swedish national cataract register report. Acta Ophthalmol. 100, e571–e577. doi: 10.1111/aos.14914
Rim, T. H., Lee, C. J., Tham, Y. C., Cheung, N., Yu, M., Lee, G., et al. (2021). Deep-learning-based cardiovascular risk stratification using coronary artery calcium scores predicted from retinal photographs. Lancet Digit. Health 3, e306–e316. doi: 10.1016/S2589-7500(21)00043-1
Rithalia, A., McDaid, C., Suekarran, S., Myers, L., and Sowden, A. (2009). Impact of presumed consent for organ donation on donation rates: a systematic review. BMJ 338, a3162. doi: 10.1136/bmj.a3162
Rono, H., Bastawrous, A., Macleod, D., Mamboleo, R., Bunywera, C., Wanjala, E., et al. (2021). Effectiveness of an mHealth system on access to eye health services in Kenya: a cluster-randomised controlled trial. Lancet Digit. Health 3, e414–e424. doi: 10.1016/S2589-7500(21)00083-2
Roski, J., Bo-Linn, G. W., and Andrews, T. A. (2014). Creating value in health care through big data: opportunities and policy implications. Health Aff. 33, 1115–1122. doi: 10.1377/hlthaff.2014.0147
Rowland, S. P., Fitzgerald, J. E., Holme, T., Powell, J., and McGregor, A. (2020). What is the clinical value of mHealth for patients? NPJ Digit. Med. 3, 4. doi: 10.1038/s41746-019-0206-x
Sahebjada, S., Schache, M., Richardson, A. J., Snibson, G., MacGregor, S., Daniell, M., et al. (2013). Evaluating the association between keratoconus and the corneal thickness genes in an independent Australian population. Invest. Ophthalmol. Vis. Sci. 54, 8224–8228. doi: 10.1167/iovs.13-12982
Sahu, K. S., Majowicz, S. E., Dubin, J. A., and Morita, P. P. (2021). NextGen public health surveillance and the internet of things (IoT). Front Public Health 9, 756675. doi: 10.3389/fpubh.2021.756675
Sauer, A., Greth, M., Letsch, J., Becmeur, P. H., Borderie, V., Daien, V., et al. (2020). Contact lenses and infectious keratitis: from a case-control study to a computation of the risk for wearers. Cornea.39, 769–774. doi: 10.1097/ICO.0000000000002248
Segers, M. H. M., Behndig, A., van den Biggelaar, F., Brocato, L., Henry, Y. P., Nuijts, R., et al. (2022). Risk factors for posterior capsule rupture in cataract surgery as reflected in the European Registry of Quality Outcomes for Cataract and Refractive Surgery. J. Cataract Refract. Surg. 48, 51–55. doi: 10.1097/j.jcrs.0000000000000708
Shah, R. L., and Guggenheim, J. A. (2018). Genome-wide association studies for corneal and refractive astigmatism in UK Biobank demonstrate a shared role for myopia susceptibility loci. Hum. Genet. 137, 881–896. doi: 10.1007/s00439-018-1942-8
Sharma, N., Arora, T., Singhal, D., Maharana, P. K., Garg, P., Nagpal, R., et al. (2019). Procurement, storage and utilization trends of eye banks in India. Indian J. Ophthalmol. 67, 1056–1059. doi: 10.4103/ijo.IJO_1551_18
Shilpashree, P. S., Suresh, K. V., Sudhir, R. R., and Srinivas, S. P. (2021). Automated image segmentation of the corneal endothelium in patients with fuchs dystrophy. Transl. Vis. Sci. Technol. 10, 27. doi: 10.1167/tvst.10.13.27
Sibley, D., Hopkinson, C. L., Tuft, S. J., Kaye, S. B., and Larkin, D. F. P. (2020). Differential effects of primary disease and corneal vascularisation on corneal transplant rejection and survival. Br. J. Ophthalmol. 104, 729–734. doi: 10.1136/bjophthalmol-2019-314200
Sim, D. A., Mitry, D., Alexander, P., Mapani, A., Goverdhan, S., Aslam, T., et al. (2016). The evolution of teleophthalmology programs in the United Kingdom: beyond diabetic retinopathy screening. J. Diabetes Sci. Technol. 10, 308–317. doi: 10.1177/1932296816629983
Smadja, D., Touboul, D., Cohen, A., Doveh, E., Santhiago, M. R., Mello, G. R., et al. (2013). Detection of subclinical keratoconus using an automated decision tree classification. Am. J. Ophthalmol. 156, 237–246.e1. doi: 10.1016/j.ajo.2013.03.034
Soh, Y. Q., Peh, G. S. L., Naso, S. L., Kocaba, V., and Mehta, J. S. (2021). Automated clinical assessment of corneal guttae in fuchs endothelial corneal dystrophy. Am. J. Ophthalmol. 221, 260–272. doi: 10.1016/j.ajo.2020.07.029
Somerville, T. F., Herbert, R., Neal, T., Horsburgh, M., and Kaye, S. B. (2021). An evaluation of a simplified impression membrane sampling method for the diagnosis of microbial keratitis. J. Clin. Med. 10, 5671. doi: 10.3390/jcm10235671
Song, A., Deshmukh, R., Lin, H., Ang, M., Mehta, J. S., Chodosh, J., et al. (2021). Post-keratoplasty infectious keratitis: epidemiology, risk factors, management, and outcomes. Front Med. 8, 707242. doi: 10.3389/fmed.2021.707242
Sudhir, R. R., Dey, A., Bhattacharrya, S., and Bahulayan, A. (2019). AcrySof IQ PanOptix intraocular lens versus extended depth of focus intraocular lens and trifocal intraocular lens: a clinical overview. Asia Pac. J. Ophthalmol. 8, 335–349. doi: 10.1097/APO.0000000000000253
Tam, V., Patel, N., Turcotte, M., Bossé, Y., Paré, G., Meyre, D., et al. (2019). Benefits and limitations of genome-wide association studies. Nat. Rev. Genet. 20, 467–484. doi: 10.1038/s41576-019-0127-1
Tan, D., Ang, M., Arundhati, A., and Khor, W. B. (2015). Development of selective lamellar keratoplasty within an asian corneal transplant program: the singapore corneal transplant study (An American Ophthalmological Society Thesis). Trans. Am. Ophthalmol. Soc. 113, T10.
Tan, D. T., Dart, J. K., Holland, E. J., and Kinoshita, S. (2012). Corneal transplantation. Lancet 379, 1749–1761. doi: 10.1016/S0140-6736(12)60437-1
Tan, J. C. K., Ferdi, A. C., Gillies, M. C., and Watson, S. L. (2019). Clinical registries in ophthalmology. Ophthalmology 126, 655–662. doi: 10.1016/j.ophtha.2018.12.030
Tan, S. Z., Walkden, A., Au, L., Fullwood, C., Hamilton, A., Qamruddin, A., et al. (2017). Twelve-year analysis of microbial keratitis trends at a UK tertiary hospital. Eye 31, 1229–1236. doi: 10.1038/eye.2017.55
Tavassoli, S., Nayar, G., Darcy, K., Grzeda, M., Luck, J., Williams, O. M., et al. (2019). An 11-year analysis of microbial keratitis in the South West of England using brain-heart infusion broth. Eye 33, 1619–1625. doi: 10.1038/s41433-019-0463-6
Ting, D. S., Potts, J., Jones, M., Lawther, T., Armitage, W. J., Figueiredo, F. C., et al. (2016a). Impact of telephone consent and potential for eye donation in the UK: the Newcastle Eye Centre study. Eye 30, 342–348. doi: 10.1038/eye.2015.216
Ting, D. S., Potts, J., Jones, M., Lawther, T., Armitage, W. J., Figueiredo, F. C., et al. (2016b). Changing trend in the utilisation rate of donated corneas for keratoplasty in the UK: The North East England Study. Eye 30, 1475–1480. doi: 10.1038/eye.2016.145
Ting, D. S., Sau, C. Y., Srinivasan, S., Ramaesh, K., Mantry, S., Roberts, F., et al. (2012). Changing trends in keratoplasty in the West of Scotland: a 10-year review. Br. J. Ophthalmol. 96, 405–408. doi: 10.1136/bjophthalmol-2011-300244
Ting, D. S. J., Ang, M., Mehta, J. S., and Ting, D. S. W. (2019a). Artificial intelligence-assisted telemedicine platform for cataract screening and management: a potential model of care for global eye health. Br. J. Ophthalmol. 103, 1537–1538. doi: 10.1136/bjophthalmol-2019-315025
Ting, D. S. J., Bignardi, G., Koerner, R., Irion, L. D., Johnson, E., Morgan, S. J., et al. (2019b). Polymicrobial keratitis with Cryptococcus curvatus, Candida parapsilosis, and Stenotrophomonas maltophilia after penetrating keratoplasty: a rare case report with literature review. Eye Contact Lens 45, e5–e10. doi: 10.1097/ICL.0000000000000517
Ting, D. S. J., Cairns, J., Gopal, B. P., Ho, C. S., Krstic, L., Elsahn, A., et al. (2021a). Risk factors, clinical outcomes, and prognostic factors of bacterial keratitis: the Nottingham Infectious Keratitis Study. Front. Med. 8, 715118. doi: 10.3389/fmed.2021.715118
Ting, D. S. J., Chua, D., May, K. O., Aung, M., Kumar, A., Farook, M., et al. (2020a). Modified manual small incision cataract surgery technique for phacoemulsification-trained surgeons. Ther. Adv. Ophthalmol. 12, 2515841420977372. doi: 10.1177/2515841420977372
Ting, D. S. J., Deshmukh, R., Said, D. G., and Dua, H. S. (2020b). The impact of COVID-19 pandemic on ophthalmology services: are we ready for the aftermath? Ther. Adv. Ophthalmol. 12, 2515841420964099. doi: 10.1177/2515841420964099
Ting, D. S. J., Foo, V. H., Yang, L. W. Y., Sia, J. T., Ang, M., Lin, H., et al. (2021b). Artificial intelligence for anterior segment diseases: Emerging applications in ophthalmology. Br. J. Ophthalmol. 105, 158–168. doi: 10.1136/bjophthalmol-2019-315651
Ting, D. S. J., Galal, M., Kulkarni, B., Elalfy, M. S., Lake, D., Hamada, S., et al. (2021c). Clinical characteristics and outcomes of fungal keratitis in the United Kingdom 2011-2020: a 10-year study. J. Fungi 7, 966. doi: 10.3390/jof7110966
Ting, D. S. J., Ho, C. S., Cairns, J., Elsahn, A., Al-Aqaba, M., Boswell, T., et al. (2021d). 12-year analysis of incidence, microbiological profiles and in vitro antimicrobial susceptibility of infectious keratitis: the Nottingham Infectious Keratitis Study. Br. J. Ophthalmol. 105, 328–333. doi: 10.1136/bjophthalmol-2020-316128
Ting, D. S. J., Ho, C. S., Deshmukh, R., Said, D. G., and Dua, H. S. (2021e). Infectious keratitis: an update on epidemiology, causative microorganisms, risk factors, and antimicrobial resistance. Eye 35, 1084–1101. doi: 10.1038/s41433-020-01339-3
Ting, D. S. J., Krause, S., Said, D. G., and Dua, H. S. (2021f). Psychosocial impact of COVID-19 pandemic lockdown on people living with eye diseases in the UK. Eye 35, 2064–2066. doi: 10.1038/s41433-020-01130-4
Ting, D. S. J., Peh, G. S. L., Adnan, K., and Mehta, J. S. (2022). Translational and regulatory challenges of corneal endothelial cell therapy: a global perspective. Tissue Eng Part B Rev. 28, 52–62. doi: 10.1089/ten.teb.2020.0319
Ting, D. S. J., Rees, J., Ng, J. Y., Allen, D., and Steel, D. H. W. (2017). Effect of high-vacuum setting on phacoemulsification efficiency. J. Cataract. Refract. Surg. 43, 1135–1139. doi: 10.1016/j.jcrs.2017.09.001
Ting, D. S. J., Settle, C., Morgan, S. J., Baylis, O., and Ghosh, S. A. (2018). 10-year analysis of microbiological profiles of microbial keratitis: the North East England Study. Eye 32, 1416–1417. doi: 10.1038/s41433-018-0085-4
Ting, D. S. W., Carin, L., Dzau, V., and Wong, T. Y. (2020c). Digital technology and COVID-19. Nat. Med. 26, 459–461. doi: 10.1038/s41591-020-0824-5
Ting, D. S. W., Cheung, C. Y., Lim, G., Tan, G. S. W., Quang, N. D., Gan, A., et al. (2017). Development and validation of a deep learning system for diabetic retinopathy and related eye diseases using retinal images from multiethnic populations with diabetes. JAMA 318, 2211–2223. doi: 10.1001/jama.2017.18152
Tiwari, M., Piech, C., Baitemirova, M., Prajna, N. V., Srinivasan, M., Lalitha, P., et al. (2022). Differentiation of active corneal infections from healed scars using deep learning. Ophthalmology 129, 139–146. doi: 10.1016/j.ophtha.2021.07.033
Ung, L., Acharya, N. R., Agarwal, T., Alfonso, E. C., Bagga, B., Bispo, P. J., et al. (2019). Infectious corneal ulceration: a proposal for neglected tropical disease status. Bull. World Health Organ. 97, 854–856. doi: 10.2471/BLT.19.232660
Uslu, A., and Stausberg, J. (2021). Value of the electronic medical record for hospital care: update from the literature. J. Med. Int. Res. 23, e26323. doi: 10.2196/26323
Vigueras-Guillén, J. P., van Rooij, J., Engel, A., Lemij, H. G., van Vliet, L. J., Vermeer, K. A., et al. (2020). Deep learning for assessing the corneal endothelium from specular microscopy images up to 1 year after ultrathin-DSAEK surgery. Transl. Vis. Sci. Technol. 9, 49. doi: 10.1167/tvst.9.2.49
Villanustre, F., Chala, A., Dev, R., Xu, L., LexisNexis, J. S., Furht, B., et al. (2021). Modeling and tracking Covid-19 cases using Big Data analytics on HPCC system platformm. J Big Data. 8, 33. doi: 10.1186/s40537-021-00423-z
Wang, S. Y., Pershing, S., and Lee, A. Y. (2020). Big data requirements for artificial intelligence. Curr. Opin. Ophthalmol. 31, 318–323. doi: 10.1097/ICU.0000000000000676
Wang, W., Yan, W., Fotis, K., Prasad, N. M., Lansingh, V. C., Taylor, H. R., et al. (2016). Cataract surgical rate and socioeconomics: a global study. Invest. Ophthalmol. Vis. Sci. 57, 5872–5881. doi: 10.1167/iovs.16-19894
Whitelaw, S., Mamas, M. A., Topol, E., and Van Spall, H. G. C. (2020). Applications of digital technology in COVID-19 pandemic planning and response. Lancet Digit. Health 2, e435–e440. doi: 10.1016/S2589-7500(20)30142-4
Wilkinson, M. D., Dumontier, M., Aalbersberg, I. J., Appleton, G., Axton, M., Baak, A., et al. (2016). The FAIR guiding principles for scientific data management and stewardship. Sci Data 3, 160018. doi: 10.1038/sdata.2016.18
Williams, K. A., Lowe, M., Bartlett, C., Kelly, T. L., and Coster, D. J. (2008). Risk factors for human corneal graft failure within the Australian corneal graft registry. Transplantation 86, 1720–1724. doi: 10.1097/TP.0b013e3181903b0a
World Health Organization (2016). Report of the Third Global Survey on eHealth. Geneva: World Health Organization; Global Observatory for eHealth.
Wu, X., Huang, Y., Liu, Z., Lai, W., Long, E., Zhang, K., et al. (2019). Universal artificial intelligence platform for collaborative management of cataracts. Br. J. Ophthalmol. 103, 1553–1560. doi: 10.1136/bjophthalmol-2019-314729
Xu, X., Zhang, L., Li, J., Guan, Y., and Zhang, L. A. (2020). Hybrid global-local representation CNN model for automatic cataract grading. IEEE J. Biomed. Health Inform. 24, 556–567. doi: 10.1109/JBHI.2019.2914690
Zetterberg, M., Kugelberg, M., Nilsson, I., Lundström, M., Behndig, A., Montan, P. A., et al. (2021). Composite risk score for capsule complications based on data from the Swedish National Cataract Register: relation to surgery volumes. Ophthalmology 128, 364–371. doi: 10.1016/j.ophtha.2020.07.033
Keywords: big data, cornea, cataract, clinical registry, artificial intelligence, electronic health record (EHR), mHealth, ophthalmology (MeSH)
Citation: Ting DSJ, Deshmukh R, Ting DSW and Ang M (2023) Big data in corneal diseases and cataract: Current applications and future directions. Front. Big Data 6:1017420. doi: 10.3389/fdata.2023.1017420
Received: 12 August 2022; Accepted: 16 January 2023;
Published: 01 February 2023.
Edited by:
João Valente Cordeiro, New University of Lisbon, PortugalReviewed by:
Marisol Martinez Alanis, Universidad Anahuac Mexico, MexicoSachi Nandan Mohanty, College of Engineering Pune, India
Copyright © 2023 Ting, Deshmukh, Ting and Ang. This is an open-access article distributed under the terms of the Creative Commons Attribution License (CC BY). The use, distribution or reproduction in other forums is permitted, provided the original author(s) and the copyright owner(s) are credited and that the original publication in this journal is cited, in accordance with accepted academic practice. No use, distribution or reproduction is permitted which does not comply with these terms.
*Correspondence: Darren S. J. Ting, ting.darren@gmail.com