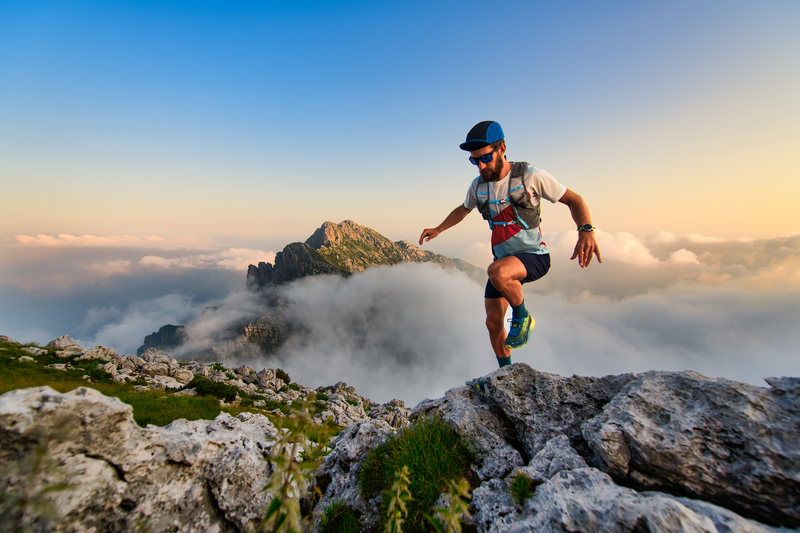
94% of researchers rate our articles as excellent or good
Learn more about the work of our research integrity team to safeguard the quality of each article we publish.
Find out more
EDITORIAL article
Front. Big Data , 05 May 2022
Sec. Data-driven Climate Sciences
Volume 5 - 2022 | https://doi.org/10.3389/fdata.2022.898643
This article is part of the Research Topic Statistical Learning for Predicting Air Quality View all 5 articles
Editorial on Research Topic
Statistical Learning for Predicting Air Quality
The concentration of air pollutants is traditionally explained by complex physical and chemical processes of dispersion and advection. This is the reason why the prediction of air quality is usually addressed through deterministic models, such as Chemical Transport Models (CTMs).
However, the CTMs show several limitations and constraints. Their performance depends on an updated emission inventory of the urban area, which is often compromised in developing countries. They also struggle to make an accurate air pollution forecast in complex terrain regions. Moreover, they require high computational power, in order to run time-consuming simulations.
More recently, statistical models based on Machine Learning (ML) algorithms have appeared as a valuable alternative to tackle many disadvantages of the CTMs. They seem particularly relevant to provide a fine resolution at an urban scale, where the estimation of air contamination is of the most importance for health concerns. In that sense, ML could become the new paradigm for pollution forecasting.
The main goal of this Research Topic is to understand if ML can become the new standard for air quality prediction. Among the several ML methods, we intend to identify the most suitable algorithms for atmospheric pollution forecasting. Such an investigation considers all the dimensions of the prediction performance, which includes both the accuracy and the interpretability of the models. For example, the non-linear models (e.g., ensemble learning or artificial neural networks) tend to be more accurate but less interpretable than a linear regression.
The first paper highlights the fact that a data-driven method such as ML can consider an infinite number of factors affecting air quality, which can improve drastically the prediction. Saheer et al. explain that ML can take into account several heterogenous factors, such as urban traffic, aerial imagery of terrains and vegetation, and weather conditions, for a more reliable prediction of air quality. The authors propose a cost-effective framework composed of different machine learning methods, from statistical to deep learning algorithms.
The benefit of ML over the CTM approach is demonstrated in the second article. Fan et al. compare the performance of a chemical transport model (AIRPACT) and two machine leaning (ML) models to forecast O3 in Kennewick (WA, USA). The first ML model (ML1) uses the random forest (RF) classifier and multiple linear regression (MLR) models, and the second model (ML2) uses a two-phase RF regression model. ML1 and ML2 are the best models to predict high and low O3 pollution events, respectively. On top of that, the ML models require much less computational resources than AIRPACT, which suggests that ML is a better solution than CTMs to forecast O3.
The third study shows that ML is not only a suitable method to predict O3 but can be applied to predict any kind of pollutants. Mendes et al. use Classification and Regression Tree (CART) and multiple regression (MR) to forecast PM10, PM2.5, NO2, and O3 concentrations in Portugal (Lisbon and Madeira) and Macao. The proposed models are able to predict the concentration of the pollutants for the next day, with a good accuracy.
Finally, the last manuscript addresses the question of the effect of the COVID-19 Lockdown on air quality change. Chau et al. propose a new approach based on Weather Normalized Modeling to get a more reliable estimation of the concentration of pollutants under a business-as-usual assumption. Several Deep Learning (DL) algorithms and Gradient Boosted Machine (GBM) are tested to quantify the impact of the human mobility reduction on the concentration of the criteria pollutants (CO, NO2, PM2.5, SO2, and O3) in Quito, Ecuador. The results show that Long-Short Term Memory (LSTM) and Bidirectional Recurrent Neural Network (BiRNN) outperform the other algorithms. All the pollutants have significantly reduced, except O3 that increased by titration effect. Besides revealing the better accuracy of DL over the other methods, this work identifies the most important factors to predict air pollution.
Overall, the studies of this Research Topic tend to demonstrate that statistical or machine learning is a powerful alternative method to the traditional CTM approach, whatever the aspect of pollution forecasting considered. ML is a fast and affordable technique which requires less computational power for an accuracy that can be higher than CTM. Also, the recent progress in the ML algorithms allow a disclosure of the models, which were until now considered as a black box. Resolving the model interpretation issue can definitely rank the ML approach as the best method for predicting air quality.
YR has written the article. RZ has revised and edited the text. All authors contributed to the article and approved the submitted version.
The authors declare that the research was conducted in the absence of any commercial or financial relationships that could be construed as a potential conflict of interest.
All claims expressed in this article are solely those of the authors and do not necessarily represent those of their affiliated organizations, or those of the publisher, the editors and the reviewers. Any product that may be evaluated in this article, or claim that may be made by its manufacturer, is not guaranteed or endorsed by the publisher.
Keywords: machine learning, urban pollution, deep learning, chemical transport model (CTM), forecast
Citation: Rybarczyk YP and Zalakeviciute R (2022) Editorial: Statistical Learning for Predicting Air Quality. Front. Big Data 5:898643. doi: 10.3389/fdata.2022.898643
Received: 17 March 2022; Accepted: 31 March 2022;
Published: 05 May 2022.
Edited and Reviewed by: John S. Kimball, University of Montana, United States
Copyright © 2022 Rybarczyk and Zalakeviciute. This is an open-access article distributed under the terms of the Creative Commons Attribution License (CC BY). The use, distribution or reproduction in other forums is permitted, provided the original author(s) and the copyright owner(s) are credited and that the original publication in this journal is cited, in accordance with accepted academic practice. No use, distribution or reproduction is permitted which does not comply with these terms.
*Correspondence: Yves Philippe Rybarczyk, cnliYXI2M0BnbWFpbC5jb20=
Disclaimer: All claims expressed in this article are solely those of the authors and do not necessarily represent those of their affiliated organizations, or those of the publisher, the editors and the reviewers. Any product that may be evaluated in this article or claim that may be made by its manufacturer is not guaranteed or endorsed by the publisher.
Research integrity at Frontiers
Learn more about the work of our research integrity team to safeguard the quality of each article we publish.