Neural representations of anticipated reward value are core to models of the mechanisms for learning (
Schultz et al., 1997
;
Sutton and Barto, 1998
;
O’Doherty et al., 2004
;
Seymour et al., 2004
) and decision making (
Montague and Berns, 2002
;
Bayer and Glimcher, 2005
;
Balleine et al., 2007
;
Rangel et al., 2008
). These models associate predictive cues with their subsequent outcomes, in order to describe behavior. Accordingly, the subjective experience of the cue-outcome association prior to the occurrence of the outcome reflects “anticipation”.
The most common functional neuroimaging paradigms for studying reward anticipation use learned cue-response-outcome contingencies (
Delgado et al., 2000
;
Knutson et al., 2001
,
2005
). On each trial an initial cue indicates a potential reward (e.g., a monetary gain). Then, following a short delay, a target appears, and if participants respond sufficiently quickly and/or accurately, they receive a reward. Studies using variants of this approach have demonstrated that the ventral striatum (vSTR), particularly its nucleus accumbens (NAcc), exhibits increases in blood oxygenation level-dependent (BOLD) contrast (hereafter, “activation”) to anticipated rewards (
Knutson et al., 2000
;
Ernst et al., 2005
;
Adcock et al., 2006
;
Knutson and Gibbs, 2007
;
Dillon et al., 2008
). Yet, despite the prevalence of this approach, several important questions about reward anticipation remain incompletely answered: How do these findings generalize to other regions within the dopaminergic system (e.g., the ventral tegmental area, VTA)? Does activation of these regions reflect the motivational salience of cued stimuli (i.e., imperative for action) or the affective properties of the anticipated reward (i.e., valence)? And, is anticipatory activation modulated by decreases in motivational salience if magnitude and valence are held constant? To address these questions we examined brain activation during anticipation of rewards that varied in valence and in personal relevance. Decreased personal relevance should reduce – but not eliminate – motivational salience, while leaving magnitude and valence unchanged.
How Does VTA Contribute to Reward Anticipation and Learning?
The neural mechanisms that underlie motivation depend on activity of neurons in the NAcc (
Wise, 1980
,
2004
;
Kalivas et al., 2005
;
Berridge, 2007
;
Salamone et al., 2007
), which are themselves modulate by dopaminergic producing neurons in the VTA (
Swanson, 1982
;
Ikemoto, 2007
). While much is known about VTA function from single-unit recordings in non-human animals, there have been relatively few neuroimaging studies that report effects in the VTA, largely because of technical constraints. The VTA is a small nucleus within the midbrain, and its boundaries with adjacent nuclei are not readily visible on standard structural magnetic resonance images. Researchers targeting the VTA, therefore, have used a combination of anatomical region-of-interest (ROI) analyses and targeted pulse sequences (e.g., inferior slices, tilted orientation). As one initial example, research by
Adcock et al. (2006)
evaluated the potential modulatory role of VTA in shaping memory, demonstrating specifically improved recall for stimuli associated with greater potential rewards. Using a combination of standard regression analyses and functional connectivity measures, they found that voxels within the anatomical location of VTA both increased in activation to larger potential rewards and exhibited functional connectivity with the hippocampus in effective memory formation.
More recently,
D’Ardenne et al. (2008)
describe VTA responses to the experience of primary and secondary rewards, as a functional neuroimaging analog of the prediction error signals previously reported in single-unit recordings (
Ljungberg et al., 1992
;
Schultz et al., 1997
;
Bayer and Glimcher, 2005
). They found that VTA activation increased to unexpected rewards, both primary (liquid) and secondary (money), consistent with single-unit studies showing that its neurons convey a positive reward prediction error. Of note, D’Ardenne et al. found no significant changes in VTA activation to the omission of an expected liquid reward nor to an unexpected monetary loss, as would be expected if that region also signaled negative reward prediction errors. Where imaging volumes have allowed, some prior studies have reported qualitatively similar results in both NAcc and midbrain (
Knutson et al., 2005
) and NAcc and VTA (
Moll et al., 2006
), although a systematic comparison is needed.
What does Neural Activity during Reward Anticipation Represent?
Understanding how the brain encodes, represents, and manipulates signals that indicate potential and experienced rewards has been an area of considerable basic (
Montague and Berns, 2002
;
Bayer and Glimcher, 2005
;
Phillips et al., 2007
;
Delgado et al., 2008a
,
b
,
Knutson and Greer, 2008
) and clinical research (
Kilts et al., 2001
;
Grusser et al., 2004
;
Kienast and Heinz, 2006
;
Bjork et al., 2008
;
Knutson et al., 2008a
,
Schlagenhauf et al., 2008
;
Scott et al., 2008
;
Strohle et al., 2008
;
Pizzagalli et al., 2009
). The common thread in this extensive literature is that the neural representation of reward does not reflect any simple unitary construct. In particular, there has been an ongoing debate about whether and how the brain represents two different aspects of reward. The first aspect is the absolute value of the outcome (i.e., important vs. unimportant outcomes), referred to as
energization (
Elliot, 2006
),
salience (
Zink et al., 2003
),
incentive salience (
Berridge et al., 2009
), and
magnitude (
Knutson et al., 2001
). A second aspect differentiates positive from negative outcomes; this aspect has been described in terms of
affect (
Knutson and Greer, 2008
),
valence, and
approach/
avoidance (
Elliot, 2006
). In the current paper, we will refer to these two aspects, which we intended to manipulate separately, as
motivation and
affective valence.
In the influential framework advanced by Berridge and colleagues, there are functional and neural dissociations between the valenced and non-valenced aspects of reward (
Berridge, 2004
;
Berridge et al., 2009
). Specifically, these authors contend that the response of dopaminergic neurons in the VTA and NAcc reflect a motivational signal associated with information about future rewards (i.e., “wanting” the reward). In contrast, other neurotransmitters (e.g., opioids) affect the valence component of reward [i.e., “liking” the reward (
Wise, 1980
)]; they make pleasurable stimuli more pleasurable and aversive experiences less aversive (
Pecina and Berridge, 2005
). These potentially dissociable concepts – motivational significance and affective valence – recur in functional neuroimaging studies of reward anticipation and experience, although some reports discuss activation in these brain regions from the perspective of approach/avoidance behavior (
Elliot, 2006
), others invoke changes in affect evoked by rewards (
Knutson and Greer, 2008
), and still others consider responses in these regions as markers of prediction error [both valenced and non-valenced (
O’Doherty et al., 2004
;
Seymour et al., 2007
)].
Despite this ongoing debate, motivation and affective valence can be difficult to tease apart experimentally. Rewards in neuroeconomic research are commonly monetary gains implemented in paradigms where they have both motivational significance and affective valence. The resulting activation in NAcc, VTA, or other reward-related regions may thus be attributed to either motivation or valence. Some reports indicate that stimuli of similar motivational significance but different valence (e.g., monetary gains and losses) evoke similar activation in reward-related regions. For example,
Cooper and Knutson (2008)
show that when an outcome is uncertain, activation in the NAcc increases for both gain and loss anticipation. Other studies have suggested that activation in some components of the reward system does indeed depend on valence, whether because of distinct spatial loci evoked by positive and negative stimuli (
Seymour et al., 2007
) or because of decreases in activation to negative events (
Breiter et al., 2001
).
Tom et al. (2007)
tracked parametric effects of gain and loss magnitudes in a loss-aversion paradigm, and found that activation in regions including the vSTR increased with magnitude for decisions about potential gains and decreased with magnitude for potential losses. Based on these and other conflicts in the literature, how motivation and affective valence information interact within the multiple regions that constitute the reward system remains unknown.
Are the Neural Substrates of Outcome Anticipation Similar When Playing for Self and others?
Finally, there exists considerable evidence that anticipatory activation, at least in the NAcc, generalizes across a wide range of rewards. Most neuroimaging studies of reward have used monetary outcomes, typically repeated opportunities to gain or lose about a dollar (
Knutson et al., 2001
;
Daw, 2007
). Yet, similar patterns of NAcc activation can be evoked using fluid rewards (
Valentin et al., 2007
), food items (
Hare et al., 2008
,
2009
), valuable consumer goods (
Knutson et al., 2007
,
2008b
), social cooperation (
Rilling et al., 2002
), and even the opportunity to punish others (
Singer et al., 2006
). Recent studies have related the increases in NAcc activation preceding a decision to the value of rewards earned for others (
Moll et al., 2006
;
Harbaugh et al., 2007
). Based on these studies, one natural conclusion is that any anticipated reward, even one with reduced personal relevance (and thus motivational salience), would evoke activation in multiple regions within the reward system (e.g., NAcc and VTA). While plausible, this conjecture has not yet been demonstrated.
Overview of the Current Experiment
In the current study, we manipulated the valence (i.e., gain vs. loss) and motivational relevance (i.e., oneself vs. charity as beneficiary) of anticipated rewards, using an incentive-compatible response-time game modeled on common paradigms in the literature (
Knutson et al., 2000
,
2001
). In these paradigms, the trial cue is the earliest possible predictor of the potential gain or loss, and thus initiates anticipation. We focus on reward anticipation, rather than reward outcome, because the motivational and affective explanations for reward-system activation make clear and opposing predictions. If motivational influences alone drive activation during anticipation, and if manipulating the beneficiary of the reward changes the motivational salience (
Mobbs et al., 2009
), then gain- and loss-related activation should be positively correlated across individuals, with greater responses observed to self- compared to other-directed outcomes. Conversely, if affective valence alone determines anticipatory activation, activation should be greatest when playing for gains and least when playing to avoid losses (relative to neutral outcomes), but with no differences between Self and Charity treatments. Moreover, by assessing participants’ reward sensitivity and other-regarding preferences, we obtained independent predictors of individual differences in the neural responses to each reward type.
This paradigm can also test predictions of temporal difference (TD) models of anticipatory association. According to common TD models (
Sutton and Barto, 1990
), a well-learned reward cue should evoke activation that reflects the value of the expected outcome. This prediction error signal can be described in terms of the value of the associated outcome (i.e., valenced) or the association value (i.e., the strength of the prediction), as discussed further below. In the case where prediction error is valenced, a pattern similar to the valence interpretation of anticipation would be expected: positive for gains, negative for losses. In the case where prediction error mirrors the strength of the association a result similar to the motivational salience signal model would be expected: positive for both gains and losses, with neutral cues producing the least activation. Importantly, both prediction error accounts would dictate identical results in charity and self conditions, unless the predictive system also represented the motivational significance of the cues.
Participants
Twenty young adults (mean age 24 years; range 19–29 years; 10 females) participated in this study. Two were excluded because of misalignments in acquisition coverage, and one was excluded due to a Beck Depression Inventory (BDI) score indicating depression, leaving 17 participants in the reported data. All participants provided informed consent under a protocol approved by the Duke Medical Center Internal Review Board.
Experimental Procedure
The experimental session comprised initial selection of a charity, task training outside the scanner, an fMRI session using a reward anticipation task, and completion of questionnaires to assess reward attitudes.
Following informed consent, subjects read descriptions of four non-profit organizations – Easter Seals, Durham Literacy Center, Animal Protection Society, and the American Red Cross – and then selected one as their charitable target. They were then provided full information about the task structure and payment contingencies (see below for task details), and were told that no deception was used in the experiment. All participants reported that they understood the task procedures and that they believed that their earnings for charity would go to the selected target. Before entering the scanner, they completed one practice run of the task using only gain trials. We separated gain trials and loss trials into different runs, to minimize cue conflict. Then, the participants were taken to the scanner for the MRI session. During acquisition of initial structural images, each participant completed a second practice run (using only loss trials). Participants then completed four 7-min task runs during collection of fMRI data. The first run always involved monetary gains, so that subjects built up balances within cumulative banks, and the second run always involved monetary losses. The last two runs consisted of one gain run and one loss run, with their order randomly determined.
Each run consisted of 50 trials (Figure
1
), evenly split between five conditions according to potential outcome: Self $4, Charity $4, Self $0, Charity $0, and Neutral Control $0. Every trial began with a 500-ms cue whose composition indicated the target (picture), monetary amount at stake [background color: red (Self) or blue (Charity) for $4, yellow for $0 control conditions], and valence (gain: square frame, loss: circular frame). Following a variable delay of between 4 and 4.5 s, a target appeared on the screen. The subject’s task was to respond by pressing a button with the index finger of the right hand, before the target disappeared. Within gain runs, responses that were sufficiently fast added $4 to the subject’s or charity’s bank (visually indicated by a coin), and responses that were longer than the current threshold had no financial consequences (visually indicated by a ‘0’). Within loss runs, responses that were sufficiently fast resulted in no financial consequences (visually indicated by a ‘0’), whereas responses that were longer than the current threshold subtracted $4 from the subject’s or charity’s bank (visually indicated by a red circle with a diagonal line). The presentation time of the target was determined by an adaptive algorithm; using information about response times on previous similar trials, the algorithm estimated the response time threshold at which the subject would be successful on approximately 65% of trials. We emphasize that independent thresholds were used for each trial type.
Figure 1. Participants performed a monetary incentive reaction time task. An initial cue marked the start of the trial and indicated whether money was at stake and, if so, who would receive it. Each trial offered either $4 or $0, for the participant (Self), a charity (Charity), or no one. Gain and loss outcomes occurred in separate runs, to minimize cue conflict. After a variable wait (4–4.5 s) a response target appeared indicating that participants were to press a button using their right index finger as quickly as possible. The trial was scored as a hit if the participant responded in time or as a miss if they did not. Changes to the bank as a result of that trial were then displayed for 0.5 s. In gain runs on $4 trials, if the subject responded to the target in time they won $4 for themselves or a charity, if they missed the trial there was no change to that bank. During loss runs on $4 trials, if the subject responded to the target in time there was no change to that bank, if they responded too slowly, they lost $4 for either themselves or their charity. Control trials resulted in no change to the bank but participants were asked to respond as quickly as possible. Reaction time thresholds for hits and misses were set using an adaptive algorithm to allow the subject to win approximately 65% of the time. Thresholds were set independently for each trial type.
At the end of all runs, the participants exited the scanner and completed a series of behavioral questionnaires (see below). Participants were paid a base sum of $15. In addition, cumulative bank totals were calculated for both the participant (M $22.35, SD 11.75) and charity (M $22.59, SD $6.62), and participants were paid the full amount of their bank in cash (participants were guaranteed a minimum of $40 for participation). Following completion of data collection from all subjects, the researchers paid the cumulative earnings to each charity.
Behavioral Questionnaires
After completing the experiment, participants were asked to fill out a series of psychological questionnaires. These included: the BDI (a screening tool for depression) (
Beck et al., 1961
); Behavioral Inhibition System/Behavioral Activation System (BIS/BAS, an index of approach and avoidance tendencies) (
Carver and White, 1994
); Interpersonal Reactivity Index (IRI, an assessment of other-regarding
behavior) (
Davis, 1983
); Personal Altruism Level (PAL, a questionnaire using indices of other-regarding personal
efforts) (
Tankersley et al., 2007
); Self Report Altruism Scale (SRAS, an index of other-regarding
preferences) (
Rushton et al., 1981
); Temporal Experience of Pleasure Scale (TEPS, an index of reward experience and anticipation) (
Gard et al., 2006
). By taking the average of
Z-score-transformed subscales from these measures, we constructed three individual-difference covariates. We defined the covariates based on
a priori relations between the above scales: a personal
reward-sensitivity covariate (BAS and TEPS, combined); an
other-regarding preference covariate (PAL, IRI, and SRAS); and a
behavioral inhibition covariate (BIS and BDI). A factor analysis presented by
Pulos et al. (2004)
suggests the personal-distress subscale of the IRI, included in our other-regarding preference covariate, may differ from the other-regarding trait targeted by the rest of the included subscales. Therefore, as a control test, we also evaluated a more limited empathy covariate that eliminated the personal distress subscale from the IRI.
fMRI Acquisition and Preprocessing
At the beginning of the scanning session, we collected initial localizer images to identify the participant’s head position within the scanner, followed by IR-SPGR high-resolution whole-volume T1-weighted images to aid in normalization and registration (voxel size: 1 mm × 1 mm × 1 mm). We also collected 17 slice IR-SPGR images, coplanar with the BOLD contrast images described below, for use in registration and normalization.
We collected BOLD contrast images acquired using a standard echo-planar sequence on a 3T GE Signa MRI scanner. Each of the four runs comprised 416 volumes (TR: 1 s; TE: 27 ms; Flip angle: 77°; voxel size: 3.75 mm × 3.75 mm × 3.8 mm) of 17 axial slices positioned to provide coverage of the midbrain and striatum (Figure
2
). A TR of 1 s, and consequently a smaller acquisition volume, was chosen to increase the sampling rate in our ROIs (NAcc and VTA). We note that the GE Signa EPI sequence automatically passes images through a Fermi filter with a transition width of 10 mm and radius of half the matrix size, which resulted in an effective smoothing kernel of approximately 4.8 mm
3. Thus, we did not include additional smoothing as part of our preprocessing protocol. Following reorientation, raw BOLD images were skull stripped using FSL’s BET, corrected for intervolume head motion using MCFLIRT (
Jenkinson et al., 2002
), intensity normalized by a single multiplicative factor, and subjected to a high-pass temporal filter (Gaussian-weighted least-squares straight line fitting, with sigma = 50.0 s). Registration to high-resolution structural and standard-space images were carried out using FLIRT (
Jenkinson and Smith, 2001
;
Jenkinson et al., 2002
). All coordinates are reported in MNI space.
Figure 2. Medial surface sagittal image showing overlap of fMRI volumes acquired in the 17 included participants.
fMRI Analysis: General Linear Model
All fMRI analyses were carried out using FEAT (FMRI Expert Analysis Tool) Version 5.92, part of FSL (FMRIB’s Software Library,
www.fmrib.ox.ac.uk/fsl
). Time-series statistical analyses used FILM with local autocorrelation correction (
Woolrich et al., 2001
).
Our first-level (i.e., within-run) analysis model included five regressors for the anticipation period with two regressors (gain and loss) for the outcome period of each trial type. The anticipation period was modeled as a unit-amplitude response with 1 s duration following the disappearance of the trial indicator cue. The outcome period was modeled as a unit-amplitude response with 1 s duration following the onset of feedback. Trial timing and numbers are noted in the task description above. Self $4 trials were contrasted against Self $0 trials (and Charity $4 against Charity $0) to examine anticipation of gain and loss. The Neutral Control $0 trials were modeled but not analyzed. Second-level (i.e., across-run, but within-subject) analyses used a fixed-effects model, while third-level (i.e., across-subjects) mixed-effects analyses (FLAME 1) included the main effects of each regressor from the lower level analysis, along with three covariates: reward sensitivity, empathy (other regarding preference), and inhibition. Whole-brain analyses used a voxel significance threshold of
z > 2.3 and a cluster-significance threshold of
p < 0.05, fully corrected for all voxels in our imaging volume (
Worsley, 2001
). Because clustering algorithms do not easily differentiate large areas of activation, Tables
1
–
4
report the top ten peak voxels present using the elevated threshold indicated in each table.
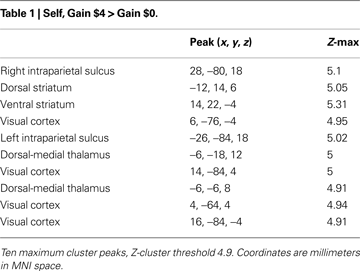
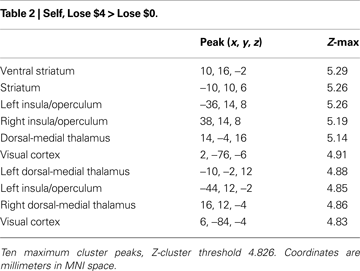
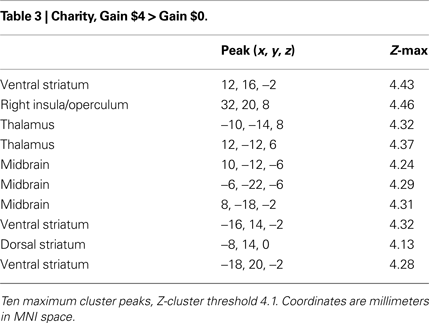
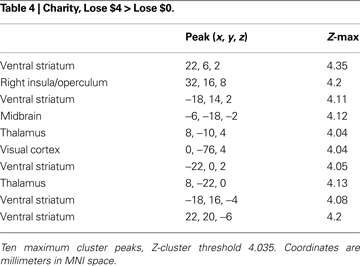
fMRI Analysis: Regions of Interest
Our primary analyses used two anatomically defined ROIs: NAcc and VTA. Hand drawn anatomical ROIs were identified based on the average of all participant’s normalized high-resolution anatomical images. The NAcc ROIs were drawn in each hemisphere according to (
Breiter et al., 1997
). The VTA ROI was drawn by isolating the region medial and anterior to the substantia nigra, following work of
Adcock et al. (2006)
. Only ROI voxels that fell within the group coverage area were included in the analysis.
Behavioral Data
The proportion of successful responses (i.e., those faster than the adaptive response-time threshold) was similar across all four self and charity reward conditions (Self $4: M 64%, SD 7%; Charity $4: M 64%, SD 4%; Self $0: M 63%, SD 6%; Charity $0: M 62%, SD 5%), indicating that our adaptive algorithm successfully matched reward rates. Reaction times to $4 gain trials (M 208 ms, SD 23 ms) were not significantly different from reaction times to $4 loss trials (M 207 ms, SD 24 ms). Reaction times on $4 trials were faster than $0 trials, and $4 trials played for Self were faster than $4 trials played for Charity [2 (beneficiary: Self vs. Charity) × 2 (magnitude: $4 vs. $0) repeated-measures ANOVA; main effect of magnitude: F(1, 16) = 25.7, p < 0.01, $4; beneficiary × magnitude interaction: F(1,16) = 7.5, p < 0.05); paired comparison (M −22 ms, SEM 4 ms, p < 0.001) of $4 (M 207 ms, SD 23 ms) vs. $0 (M 210 ms, SD 24 ms), p < 0.001; paired comparison (M −5ms, SEM 2 ms, p < 0.05) of Self $4 (M 205 ms, SD 24 ms) vs. Charity $4 (M 210 ms, SD 23 ms)]. There were no significant differences in reaction times on $0 trials (Self $0: M 230 ms, SD 28 ms; Charity $0: M 228 ms, SD 23 ms).
fMRI Results: Whole-Volume Analyses
Anticipating gain and loss for self
All analyses reported in this manuscript use regressors associated with reward anticipation (i.e., time-locked to the disappearance of the initial reward cue). We first contrasted parameter estimates between trials that offered the chance to make $4 and trials where no money was at stake (Self-Gain $4 > Self-Gain $0). Activation associated with anticipated monetary gains was widely distributed throughout the imaged volume (Table
1
), with peaks in the dorsal striatum and vSTR, bilateral operculum/insula (Figure
3
A, top), midbrain (Figure
3
A, bottom), mediodorsal thalamus, medial prefrontal, medial orbitofrontal, anterior pole, and visual cortex. These results replicate those found in previous studies of gain anticipation (
Knutson et al., 2001
;
Knutson and Greer, 2008
).
Figure 3. Whole-brain analysis reveals similar patterns of activation during anticipation of gains and losses, whether participants played for self or a charity. Activated regions were larger and more significant in the Self conditions. Activation peaks were present in the NAcc and VTA in all four treatments (i.e., anticipating gain, anticipating loss, playing for self, playing for a charity). ROIs for bilateral NAcc (
A and
B,
top) are shown on a coronal image (
y = −12). The ROI for the VTA (
A and
B,
bottom) is shown on a magnified axial image (
z = −12). ROIs are indicated in white on an anatomical image to the left of the statistical maps. The left side of each image corresponds to the participant’s left. All statistical map colors reflect the
Z-score color scale in the upper right corner. Other significant peaks in each condition are listed in Tables
1
–
4
.
Next, we conducted a similar analysis for anticipated monetary losses, by contrasting trials that offered the chance to avoid losing $4 and trials where no money was at stake (Self-Loss $4 vs. Self-Loss $0). Activations in this loss-anticipation contrast (Table
2
) were distributed similarly to the gain condition. Peaks of activation were also similar to those noted under the gain condition, including in the dorsal striatum and vSTR, bilateral operculum/insula (Figure
3
B, top), midbrain (shown in Figure
3
B, bottom), mediodorsal thalamus, and orbitofrontal and visual cortex.
The direct contrast between gain and loss anticipation (Self-Gain $4 > Self-Loss $4) identified only one cluster along the inferior parietal sulcus (Z = 3.2; max: 32, −82, 20), and no differential activation overlapping our ROIs or in other regions implicated in reward anticipation by prior literature. No significant clusters of activation were identified in the reverse contrast (Self-Loss $4 > Self-Gain $4). Moreover, no clusters exhibited significantly decreased activation during either self-directed gain or loss trials compared to control trials (i.e., Self-Gain $0 > Self-Gain $4, or Self-Loss $0 > Self-Loss $4).
Anticipating gain and loss for charity
We repeated all of the analyses from the previous section for trials that offered the chance to gain or lose money for the selected charity. Anticipating potential gains and losses for a charity evoked activation in regions within the dorsal striatum and vSTR, midbrain, thalamus, prefrontal cortex, bilateral insula, and visual cortex. Note that there was very good match between the peak loci of activation for self-directed and charity-directed rewards (Tables
3 and
4
). Direct contrasts of trials involving potential gains and potential losses (Charity-Gain $4 > Charity-Loss $4, or Charity-Loss $4 > Charity-Gain $4) revealed no clusters of activation that survived whole-volume correction.
Playing for self vs. playing for charity
We next identified regions that exhibited significant differences in activation depending on whether participants were anticipating playing for themselves or for their charity. The direct contrast of self-directed gains greater than charity-directed gains (Self-Gain $4 > Charity-Gain $4) identified activations similar to those found for self-gains (i.e., Self-Gain $4 > Self-Gain $0); i.e., within reward-related regions like the NAcc and VTA. Activation in these regions was greatest to self-directed rewards, intermediate to charity-directed rewards, and least on trials where no reward could be obtained. Additional regions whose activation increased to self-directed gains (Table
5
) included the prefrontal cortex, temporal–parietal–occipital junction (TPO), and posterior insula/inferior parietal lobule (IPL). Likewise, the direct contrast of self-directed losses greater than charity-directed losses (Self-Loss $4 > Charity-Loss $4, Table
6
) evoked activation in reward-related regions, along with additional clusters in the TPO and IPL.
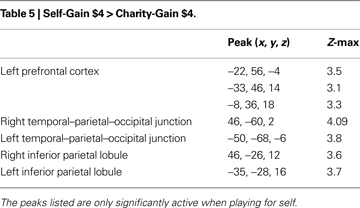
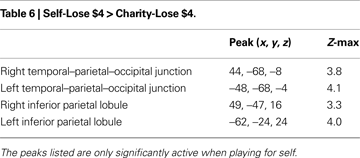
The only region exhibiting greater charity-directed activation than self-directed activation was the posterior cingulate cortex (PCC). This activation survived whole-volume correction for the loss trials (Charity-Loss $4 > Self-Loss $4, Table
7
), but not for the gain trials (Charity-Gain $4 > Loss-Gain $4;
z = 2.8 for coordinates: 2, −56, −18).
fMRI Results: ROI Analysis
Anticipatory activations in the VTA and NAcc are similar for self and charity
We defined ROIs in the VTA and NAcc, collapsed across hemispheres (see Section “Materials and Methods” for details). For each subject, we calculated parameter estimates for each ROI and reward type within a two-factor (beneficiary: Self vs. Charity; valence: gain vs. loss) repeated measures ANOVA. Note that for each trial type, we subtracted the mean activation associated with the matched $0-reward trial (e.g., Self-Gain $4 minus Self-gain $0), to control for non-task-related processing (e.g., cue perception).
We found that both VTA and NAcc showed greater activation to self-directed rewards compared to charity-directed rewards [VTA:
F(1, 13) = 7.41,
p < 0.05; NAcc:
F(1, 13) = 12.31,
p < 0.05] though on average activations were positive in both the VTA [
F(1,13) = 70.14,
p < 0.05] and NAcc [
F(1,13) = 79.97,
p < 0.05]. Neither the VTA nor the NAcc ROIs showed significant main effect of valence though the VTA did exhibit a trend [Gain vs. Loss,
F(1,13) = 3.96
p = 0.07]. However, the VTA did show a significant effect of valence that scaled with our Reward Sensitivity covariate [Gain vs. Loss × Reward Sensitivity,
F(1,13) = 5.74,
p < 0.05]. Although the NAcc did not show any main effects or direct interactions of valence, it did show a three-way interaction incorporating an effect of valence [Self vs. Charity × Gain vs. Loss × Reward Sensitivity,
F(1,13) = 5.81,
p = 0.031; Figure
4
. We also note that we found no significant differences in these regions between mean signal changes in the $0 conditions, indicating that these effects are contingent upon the presence of anticipated reward.
Figure 4. Percent signal change in the NAcc and VTA for $4 vs. $0 trials. Mean activations, relative to $0 conditions, were positive for all trial types. Activations were larger in the Self than Charity treatment condition, reflecting reliable differences on both gain and loss runs. A trend for a main effect of valence was present in the VTA but not the NAcc. Valence effects that are modulated by the reward sensitivity of the participant were present in both regions. We found no significant differences between $0 conditions. Error bars are ±standard error of the mean.
To assess the localization of each ROI and test for potential spatial inhomogeneity, we also restricted our analyses to the single voxel with the highest Z-score (i.e., most significant) to self-directed gains within the NAcc (MNI coordinates: 12, 6, −6) and VTA (MNI coordinates: 4, −16, −10) ROIs. Results of these ANOVAs are consistent with the results of the whole ROI ANOVAs in both the VTA and NAcc with two exceptions. In the NAcc, the three-way interaction present in the complete NAcc ROI (Gain vs. Loss × Reward Sensitivity) was non-significant for the peak voxel alone [F(1,13) = 2.38, p = 0.15]. Second, in the VTA, the peak reward-anticipation-sensitive voxel showed a significant main effect of valence [Gain vs. Loss: F(1,13) = 8.30, p < 0.05], an effect only significant at the trend level in the analysis of the complete VTA ROI. Note that this increase in significance may simply reflect a selection bias, given that this voxel was selected for its robust responses in the self-gain condition. As further confirmation of a motivational salience signal, significant increases in activation to self-directed losses, to charity-directed gains, and to charity-directed losses were also present in the whole-brain analysis from this voxel. In addition, there were no voxels in the VTA or NAcc that showed negative activity on loss trials (with respect to the $0 condition) across participants.
Recent work by
Matsumoto and Hikosaka (2009)
indicates there are two varieties of dopaminergic neurons in the VTA, one population that responds to positive conditions and one population that responds to both positive and negative conditions. With this in mind we also interrogated voxels in the VTA (MNI coordinates: −10, −16, −12) and NAcc (MNI coordinates: −12, 10, −6) that showed the peak activation increase (i.e., greatest
Z-score) during anticipation on loss trials. The NAcc loss peak results were consistent with those of the complete ROI and gain peak in that they showed a positive average response in all conditions, a main effect of beneficiary, a trend toward an effect of participant reward sensitivity, and significant three-way interaction of Self vs. Charity × Gain vs. Loss × Reward Sensitivity. Consistent with Matsumoto and Hikosaka, the peak loss voxel in the VTA differed from the peak gain voxel in that it exhibited no significant main effect of valence [
F(1,13) = 0.713,
p = 0.41]. We caution that these analyses do not directly test spatial inhomogeneity effects and that such results may be attributable to selection bias because although our initial definition of ROIs was independent, definitions of the peak voxels was based on non-independent tests.
Individual gain and loss anticipation traits in the NAcc and VTA
In the current study, a main effect of affective valence would manifest in increased activation for anticipation of gains and decreased activation for anticipation of losses (or vice versa). Conversely, a main effect of motivation would lead to increased activation for anticipation of both gains and losses, compared to trials without the possibility of reward. As described above, our whole-volume analyses provided no suggestions of opposite responses for gains and losses within reward-related regions; to the contrary, we found that gains and losses each evoked significant increases in activation within the NAcc and VTA, among other regions. We repeated these analyses for our anatomically defined ROIs and found a similar result: increased activation for both gain and loss trials, with greater activation for self-directed compared to charity-directed trials. Thus, we found no evidence for group-level main effects of valence in our target regions.
We next investigated whether there were any across-subjects relationships between the magnitude of the responses to gain and loss trials. If there were a
negative correlation across individuals between activations to gain and loss trials, even though the mean activation for both types of trials was positive, then that would be strong evidence that both motivation and affective valence modulate activation in reward-related regions. Alternatively, a
positive correlation between activations to gain and loss trials would provide evidence in favor of a motivational explanation, alone. Our results support the motivation explanation. In the NAcc, activations during gain anticipation scaled positively with loss anticipation (Figure
5
), with a significant correlation in self-directed-trials (
r = 0.64) and a non-significant but numerically positive correlation on charity-directed trials. In the VTA (Figure
6
), activations during gain and loss anticipation were positively correlated for both self-directed (
r = 0.58) and charity-directed (
r = 0.63) trials.
Figure 5. BOLD responses in the NAcc during gain and loss anticipation are positively correlated when participants play for themselves. Top: Average percent signal change differences (paid-control) for anticipation of gain and loss trials for the Self (left) and Charity (right) treatments. Each point is colored according to the participant’s relative reward sensitivity index (z transformed). Bottom: Individual Gain vs. Loss signal change differences are plotted against the participant’s reward sensitivity index (z transformed). Each plot includes the orthogonal distance regression best fit line, as well as the correlation coefficient (r) and the p-value of that correlation (p). Only the regression in the Self condition was significant.
Figure 6. BOLD responses in the VTA during the anticipation of gain and loss are positively correlated whether participants play for themselves or for a charity. Top: Average percent signal change differences (paid-control) for anticipation of gain and loss trials for the Self (left) and Charity (right) treatments. Each point is colored according to the participant’s relative reward sensitivity index (z transformed). Gain and loss responses differences are positively correlated in both the self and charity conditions. Bottom: Individual Gain vs. Loss signal change differences are plotted against the participant’s reward sensitivity index (z transformed). Each plot includes the orthogonal distance regression best fit line, as well as the correlation coefficient (r) and the p-value of that correlation (p). Only the regression in the Self condition was significant.
We next used a hierarchical regression analysis to evaluate whether the neural bias toward gains, compared to losses, was predicted by our reward sensitivity covariate. We found that there were strong correlations between reward sensitivity and the differential activation between gains and losses (e.g., Self-Gain $4 minus Self-Loss $4) in both the NAcc and VTA (Figures
5
A and
6
A). Individuals who had the greatest reward sensitivity exhibited the greatest relative increment in activation gains compared to losses. (We note that this is a fully independent correlation, in that we are using an independent behavioral test, an anatomical ROI, and the residual activation following a contrast of conditions.) This effect was significant for self-directed trials in both NAcc and VTA, but not for charity-directed trials in either ROI. We conducted similar analyses using covariates for other-regarding preferences and behavioral inhibition, and found no significant effects. Based on these results, we conducted a
post hoc test looking at the relationship between our reward sensitivity covariate and activation to each trial type (as opposed to the difference between trial types described above). We found that, within our sample, the NAcc and VTA responses to self-directed gains were largely similar regardless of reward sensitivity, but that high reward-sensitivity scores correlated with a relative decrease in activation on the other trial types (Figure
7
; see also colored circles on the upper right panels of Figures
5 and
6
).
Figure 7. BOLD responses in the Self-Gain condition are unrelated to reward sensitivity. BOLD responses ($4 > $0) in both the NAcc (white circle) and VTA (black triangles) were anticorrelated with reward sensitivity across subjects except in the Self-Gain condition (NAcc self-gain: r = 0.06, p = 0.81; VTA self-gain: r = 0.11, p = 0.68; NAcc charity-gain: r = −0.72, p = 0.001; VTA charity-gain: r = −0.58, p = 0.01; NAcc self-loss: r = −0.57, p = 0.02; VTA self-loss: r = −0.50, p = 0.04; NAcc charity-loss: r = −0.51, p = 0.04; VTA charity-loss: r = −0.58, p = 0.02). Solid lines indicate significant regressions. Dashed lines indicate regressions that were not significant.
We examined brain activation during the anticipation of monetary rewards that varied in their valence (i.e., gain vs. loss) and beneficiary (i.e., self-directed vs. charity-directed). We found that activation in putatively reward-related regions, specifically the NAcc and VTA, increased during both gain- and loss-anticipation, with greater responses to self-directed than charity-directed trials. Moreover, there was a strong positive correlation between these responses across individuals, such that those individuals with the greatest anticipatory response to potential gains also had the greatest response to potential losses. Together, these results indicate that anticipatory activation reflects the motivational properties of the potential reward, not its valence. However, we found evidence, using an independent behavioral covariate, that individual differences in reward sensitivity modulated the relative response to gains and losses, with more reward-sensitive individuals exhibiting relatively more activation to gains compared to losses. Below, we consider the implications of each of these results.
Reward Anticipation: Motivation vs. Valence
In group analyses, we found no evidence that anticipatory activations in either VTA or NAcc reflect a univariate value signal that scales according to both the valence and magnitude of the potential reward (i.e., gain > neutral > loss). Both potential gains and potential losses evoked increased activation compared to control stimuli in the NAcc and VTA, as shown within a whole-volume analysis, an anatomical ROI analysis, and in an analysis restricted to the most-active voxel in each region. And, as even stronger evidence that anticipatory activations reflect motivational salience, we found that activations associated with gains and with losses were positively correlated across participants. These results lie in contrast to some previous studies that have shown increased NAcc activation to anticipated gains, compared to anticipated losses(
Knutson et al., 2001
), or have failed to find increased activation to anticipated losses compared to a neutral control condition (
Knutson et al., 2003
,
2008a
). For example, a study of loss aversion by
Tom et al. (2007)
showed that activation in the vSTR to decisions about mixed gambles (i.e., that involved a potential gain and a potential loss) increased with increased size of gain, but decreased with increasing size of loss. This result was interpreted as reflecting the response of a single reward mechanism that codes for both gains and losses along a single axis of reward value. We note that gains and losses were always paired in the design of
Tom et al. (2007)
, such that the magnitude of the loss attenuated the overall value (i.e., magnitude) of the gamble. Within our study, in contrast, the potential losses were presented in isolation and thus reflected an independent and negative potential outcome, allowing a differentiation between magnitude and valence.
Prior research has suggested that under certain conditions NAcc activation reflects task factors other than value of a potential reward. Activation in the NAcc has been reported to correlate with both the salience of the stimulus presented (
Zink et al., 2003
) as well as the unpredictability of the potential outcome (
Berns et al., 2001
). It could be argued that salience or risk are inherently rewarding. However, there is also evidence that NAcc responses positively correlate with aversive stimuli (
Delgado et al., 2004
;
Jensen et al., 2007
;
Salamone et al., 2007
;
Levita et al., 2009
), as well.. In the current study, although activations to gain and loss anticipation both exceeded those present during all control conditions ($0), we found evidence that reward valence modulates the amplitude of this activation in the NAcc and VTA. In the VTA we found valence modulations related to reward sensitivity. In the NAcc modulation of valence was dependent on both the beneficiary of the reward and the reward sensitivity of the participant. One reasonable possibility is that the VTA and NAcc are primarily sensitive to aspects of motivational salience but that those responses are modulated by affective valence (
Cooper and Knutson, 2008
), especially in those participants who are most reward sensitive. The relative strength of affective valence modulation would then likely be dependent upon task context. This mixed signal may also reflect spatial inhomogeneity within the VTA and NAcc, as discussed below.
A striking result came from the imperfect matching between the neurometric responses to potential gains and to potential losses. While the gain:loss ratio across the entire subject sample was approximately 1:1, some subjects showed a relatively increased response to gains, while others showed a relatively increased response to losses. This residual variation turned out to be systematically related to participants’ reward-sensitivity scores. This behavior–brain correlation could reflect a contribution of some subcomponent of these reward-related regions, or the influence of another region that itself was sensitive to affective valence. An important direction for future research will be identifying the pattern of functional connectivity across regions that predict both trial-to-trial effects of cue value and across-subjects factors that bias those value signals.
The Role of the VTA in Reward Anticipation
Most prior neuroimaging studies of reward processing have focused on the vSTR, specifically the NAcc, which has been reliably reported to exhibit increased activation during anticipation. Much less evidence exists for the modulatory effects of anticipation in the VTA, the primary dopaminergic input to the NAcc (
Swanson, 1982
;
Ikemoto, 2007
). Prior research on VTA function, mostly using single-unit recording in non-human primates, has implicated that region in the processing of rewards, generally, and in transient responses to changing reward expectations (
Ljungberg et al., 1992
). Based on data showing that VTA neurons respond to both unexpected primary rewards and cues that predict future rewards, it has been theorized that these neurons code a reward prediction error, critical for TD learning (
Schultz et al., 1997
). It would be difficult to account for our results using prediction error signals that treat gains and losses as a single continuum. Because we separated our gain and loss cues into separate blocks, and used two types of rewards, a single continuum prediction error model would predict that we should observe the greatest anticipatory responses to Self-Gain cues, smallest (or most negative) responses to Self-Loss cues, responses in the same directions, but possibly attenuated, to both types of Charity cues, and minimal responses to the non-rewarded control cues. In contrast, we found very similar activation, both in spatial pattern and amplitude, for anticipated gains and anticipated losses, with both gains and losses greater than control cues or charitable cues. Alternatively, the opportunity to avoid losses may be might be seen as rewarding. In fact, there is evidence that relief from pain (
Seymour et al., 2005
) and even avoiding potential negative outcomes (
Kim et al., 2006
) can be viewed as rewards. However, this kind of “pure valence” explanation is inconsistent with the observation that activations on loss trials were still greater than neutral ($0) trials.
We emphasize that these results are not necessarily incompatible with the numerous prior demonstrations that prediction errors modulate the responses of VTA neurons, for three reasons. First, as is proposed by
Seymour et al. (2007)
, there may be multiple and potentially valence-dependent prediction error signals. That is, separate neuronal prediction-error signals may increase in anticipation of gains and of losses, each contributing to observed BOLD activation. Second, monetary losses may not have similar psychological and neural effects as omitted primary rewards or aversive stimuli. In particular, the loss of money reflects an opportunity cost that affects the total value of a future reward, rather than an immediate negative consequence (e.g., a painful shock). Accordingly, humans frequently reframe decision problems to minimize decision difficulty or to maximize perceived value (
Tversky and Kahneman, 1974
); in our paradigm, like many others, the loss cue may have been reframed as an opportunity to avoid negative consequences. Third, activation measured using fMRI does not necessarily map onto the firing rate of individual neurons. Substantial methodological work suggests that the amplitude of BOLD activation matches best to local field potential and multi-unit activity within a region (
Goense and Logothetis, 2008
), and less well to single-unit activity. The relatively coarse timescale of fMRI data collection, combined with the filtering effects of the BOLD hemodynamic response, precludes determination of the relative timing of the contributing neuronal activity. In addition, evidence from
Ungless et al. (2004)
indicates the VTA may not be homogenous in its responsiveness to gain and loss. They find evidence of two distinct populations of neurons in the VTA, one responsive to positive stimuli and the other to aversive stimuli. Inhomogeneity in the VTA within dopaminergic neurons is supported in recent work by
Matsumoto and Hikosaka (2009)
who show not only distinct populations of neurons responsive to positively valenced stimuli but also provide evidence of a dorsal/ventral spatial distinction. Preliminary findings in the current study suggest that an fMRI study designed to look for spatial separation of gain-specific neuronal populations in the VTA may be able to isolate them from those responsive to both gains and losses. Given these caveats, our results should be interpreted as showing that some aspect of information processing in VTA is driven by motivational properties of anticipated rewards or by a prediction error that increases with the magnitude of anticipated punishment. We also note that individuals who are more reward sensitive display effects of valence not present in those relatively less sensitive to reward.
Modulation of Anticipatory Reward Signals by Self- vs. other-Directed Context
The NAcc not only responds to meaningful self-directed outcomes, it also responds to a variety of other-directed outcomes: social cooperation (
Rilling et al., 2002
), altruistic punishment (
Singer et al., 2006
), and rewards for a favored charity (
Moll et al., 2006
;
Harbaugh et al., 2007
), among others. In these latter cases, the reward may be emulated as if it were being personally received and is therefore represented within the same system, albeit with reduced magnitude. We note that prior research showing activation in the reward system to charitable rewards used tasks involving active decisions or passive receipt of those rewards. Here, we show that mere anticipation of potential reward is sufficient to evoke activation within the NAcc; moreover, like the work of Moll et al., we extend our conclusions to include VTA, as well.
Notably, all of our main-effect analyses indicated that self-directed and charity-directed rewards evoked very similar patterns of activation: for both types of rewards, activation in the NAcc and VTA increased for both anticipated gains and anticipated losses. What differentiated these two reward types was our participants’ relative reward sensitivity, such that individuals with higher reward-sensitive individuals showed lower responses for all charitable rewards. Somewhat surprisingly, we found no similar across-participant effect of our other-regarding-preference covariate. We note that prior studies have shown that the relative subjective value of different charitable rewards, defined by the participant’s willingness to engage in a transaction as opposed to individual differences in overall other-regarding-preferences, modulates activation of the vSTR (
Moll et al., 2006
;
Harbaugh et al., 2007
). In contrast, self-reported trait measures of other-regarding preferences have been reported to relate to structural (
Yamasue et al., 2008
) and functional (
Tankersley et al., 2007
) differences in other brain regions associated with social cognition. The independence of other-regarding preferences and likelihood of engaging in a charitable transaction is worthy of further investigation.
We have presented evidence that motivational salience modulates activation in the VTA and NAcc. Activations during the anticipation phase of all trial types were positive with respect to a $0 trial. However, the magnitude of this positive activation was modulated by three factors. First, the beneficiary: activations were smaller in magnitude when the outcome of the trial was not directed toward the participant, suggesting that a single system processes social and personal rewards according to their motivational salience. Second, the valence: in the VTA, the anticipation of gains evokes greater activation than the anticipation of losses, even though both conditions are greater than trials where no reward or punishment could be obtained. Third, the reward sensitivity of the individual: for participants who are more reward sensitive, the magnitude of activations to anticipation in the VTA and NAcc is largest on gain trials played for themselves. We conclude that both the VTA and NAcc provide anticipatory signals that largely reflect the motivational significance of potential rewards.
Conflict of Interest Statement
The authors declare that the research was conducted in the absence of any commercial or financial relationships that could be construed as a potential conflict of interest.
Julia Parker Goyer and Elizabeth B. Johnson provided assistance in task programming and data collection. We thank John Clithero, O’Dhaniel Mullette-Gillman, David Smith, and Vinod Venkatraman for manuscript comments. This project was supported by NIMH 70685 (Scott A. Huettel), NINDS 41328 (Scott A. Huettel), and NARSAD (R. Alison Adcock). R. McKell Carter is supported by NIH Fellowship (NIH 51156). Scott A. Huettel is supported by an Incubator Award from the Duke Institute for Brain Sciences. R. Alison Adcock is supported by the Alfred P. Sloan Foundation.
Adcock, R. A., Thangavel, A., Whitfield-Gabrieli, S., Knutson, B., and Gabrieli, J. D. E. (2006). Reward-motivated learning: mesolimbic activation precedes memory formation.
Neuron 50, 507–517.
Balleine, B. W., Delgado, M. R., and Hikosaka, O. (2007). The role of the dorsal striatum in reward and decision-making.
J. Neurosci. 27, 8161–8165.
Bayer, H. M., and Glimcher, P. W. (2005). Midbrain dopamine neurons encode a quantitative reward prediction error signal.
Neuron 47, 129–141.
Beck, A. T., Erbaugh, J., Ward, C. H., Mock, J., and Mendelsohn, M. (1961). An inventory for measuring depression.
Arch. Gen. Psychiatry 4, 561–571.
Berns, G. S., McClure, S. M., Pagnoni, G., and Montague, P. R. (2001). Predictability modulates human brain response to reward.
J. Neurosci. 21, 2793–2798.
Berridge, K. C. (2004). Motivation concepts in behavioral neuroscience.
Physiol. Behav. 81, 179–209.
Berridge, K. C. (2007). The debate over dopamine’s role in reward: the case for incentive salience.
Psychopharmacology 191, 391–431.
Berridge, K. C., Robinson, T. E., and Aldridge, J. W. (2009). Dissecting components of reward: ‘liking’, ‘wanting’, and learning.
Curr. Opin. Pharmacol. 9, 65–73.
Bjork, J. M., Knutson, B., and Hommer, D. W. (2008). Incentive-elicited striatal activation in adolescent children of alcoholics.
Addiction 103, 1308–1319.
Breiter, H. C., Aharon, I., Kahneman, D., Dale, A., and Shizgal, P. (2001). Functional imaging of neural responses to expectancy and experience of monetary gains and losses.
Neuron 30, 619–639.
Breiter, H. C., Gollub, R. L., Weisskoff, R. M., Kennedy, D. N., Makris, N., Berke, J. D., Goodman, J. M., Kantor, H. L., Gastfriend, D. R., Riorden, J. P., Mathew, R. T., Rosen, B. R., and Hyman, S. E. (1997). Acute effects of cocaine on human brain activity and emotion.
Neuron 19, 591–611.
Carver, C. S., and White, T. L. (1994). Behavioral inhibition, behavioral activation, and affective responses to impending reward and punishment: the BIS/BAS Scales.
J. Pers. Soc. Psychol. 67, 319–333.
Cooper, J. C., and Knutson, B. (2008). Valence and salience contribute to nucleus accumbens activation.
Neuroimage 39, 538–547.
D’Ardenne, K., McClure, S. M., Nystrom, L. E., and Cohen, J. D. (2008). BOLD responses reflecting dopaminergic signals in the human ventral tegmental area.
Science 319, 1264–1267.
Davis, M. H. (1983). Measuring individual-differences in empathy – evidence for a multidimensional approach.
J. Pers. Soc. Psychol. 44, 113–126.
Daw, N. D. (2007). Dopamine: at the intersection of reward and action.
Nat. Neurosci. 10, 1505–1507.
Delgado, M. R., Gillis, M. M., and Phelps, E. A. (2008a). Regulating the expectation of reward via cognitive strategies.
Nat. Neurosci. 11, 880–881.
Delgado, M. R., Li, J., Schiller, D., and Phelps, E. A. (2008b). The role of the striatum in aversive learning and aversive prediction errors.
Philos. Trans. R. Soc. Lond., B, Biol. Sci. 363, 3787–3800.
Delgado, M. R., Nystrom, L. E., Fissell, C., Noll, D. C., and Fiez, J. A. (2000). Tracking the hemodynamic responses to reward and punishment in the striatum.
J. Neurophysiol. 84, 3072–3077.
Delgado, M. R., Stenger, V. A., and Fiez, J. A. (2004). Motivation-dependent responses in the human caudate nucleus.
Cereb. Cortex 14, 1022–1030.
Dillon, D. G., Holmes, A. J., Jahn, A. L., Bogdan, R., Wald, L. L., and Pizzagalli, D. A. (2008). Dissociation of neural regions associated with anticipatory versus consummatory phases of incentive processing.
Psychophysiology 45, 36–49.
Elliot, A. J. (2006). The hierarchical model of approach-avoidance motivation.
Motiv. Emot. 30, 111–116.
Ernst, M., Nelson, E. E., Jazbec, S., McClure, E. B., Monk, C. S., Leibenluft, E., Blair, J., and Pine, D. S. (2005). Amygdala and nucleus accumbens in responses to receipt and omission of gains in adults and adolescents.
Neuroimage 25, 1279–1291.
Gard, D. E., Gard, M. G., Kring, A. M., and John, O. P. (2006). Anticipatory and consummatory components of the experience of pleasure: a scale development study.
J. Res. Pers. 40, 1086–1102.
Goense, J. B. M., and Logothetis, N. K. (2008). Neurophysiology of the BOLD fMRI signal in awake monkeys.
Curr. Biol. 18, 631–640.
Grusser, S. M., Wrase, J., Klein, S., Hermann, D., Smolka, M. N., Ruf, M., Weber-Fahr, W., Flor, H., Mann, K., Braus, D. F., and Heinz, A. (2004). Cue-induced activation of the striatum and medial prefrontal cortex is associated with subsequent relapse in abstinent alcoholics.
Psychopharmacology 175, 296–302.
Harbaugh, W. T., Mayr, U., and Burghart, D. R. (2007). Neural responses to taxation and voluntary giving reveal motives for charitable donations.
Science 316, 1622–1625.
Hare, T. A., Camerer, C. F., and Rangel, A. (2009). Self-control in decision-making involves modulation of the vmPFC valuation system.
Science 324, 646–648.
Hare, T. A., O’Doherty, J., Camerer, C. F., Schultz, W., and Rangel, A. (2008). Dissociating the role of the orbitofrontal cortex and the striatum in the computation of goal values and prediction errors.
J. Neurosci. 28, 5623–5630.
Ikemoto, S. (2007). Dopamine reward circuitry: two projection systems from the ventral midbrain to the nucleus accumbens-olfactory tubercle complex.
Brain Res. Rev. 56, 27–78.
Jenkinson, M., Bannister, P., Brady, M., and Smith, S. (2002). Improved optimization for the robust and accurate linear registration and motion correction of brain images.
Neuroimage 17, 825–841.
Jenkinson, M., and Smith, S. (2001). A global optimisation method for robust affine registration of brain images.
Med. Image Anal. 5, 143–156.
Jensen, J., Smith, A. J., Willeit, M., Crawley, A. P., Mikulis, D. J., Vitcu, I., and Kapur, S. (2007). Separate brain regions code for salience vs. valence during reward prediction in humans.
Hum. Brain Mapp. 28, 294–302.
Kalivas, P. W., Volkow, N., and Seamans, J. (2005). Unmanageable motivation in addiction: a pathology in prefrontal-accumbens glutamate transmission.
Neuron 45, 647–650.
Kienast, T., and Heinz, A. (2006). Dopamine and the diseased brain.
CNS Neurol. Disord. Drug Targets 5, 109–131.
Kilts, C. D., Schweitzer, J. B., Quinn, C. K., Gross, R. E., Faber, T. L., Muhammad, F., Ely, T. D., Hoffman, J. M., and Drexler, K. P. G. (2001). Neural activity related to drug craving in cocaine addiction.
Arch. Gen. Psychiatry 58, 334–341.
Kim, H., Shimojo, S., and O’Doherty, J. P. (2006). Is avoiding an aversive outcome rewarding? Neural substrates of avoidance learning in the human brain.
Plos Biol 4, e233. doi: 10.1371/journal.pbio.0040233
Knutson, B., Adams, C. M., Fong, G. W., and Hommer, D. (2001). Anticipation of increasing monetary reward selectively recruits nucleus accumbens.
J. Neurosci. 21, RC159.
Knutson, B., Bhanji, J. P., Cooney, R. E., Atlas, L. Y., and Gotlib, I. H. (2008a). Neural responses to monetary incentives in major depression.
Biol. Psychiatry 63, 686–692.
Knutson, B., Wimmer, G. E., Rick, S., Hollon, N. G., Prelec, D., and Loewenstein, G. (2008b). Neural antecedents of the endowment effect.
Neuron 58, 814–822.
Knutson, B., Fong, G. W., Bennett, S. M., Adams, C. M., and Hommer, D. (2003). A region of mesial prefrontal cortex tracks monetarily rewarding outcomes: characterization with rapid event-related fMRI.
Neuroimage 18, 263–272.
Knutson, B., and Gibbs, S. E. B. (2007). Linking nucleus accumbens dopamine and blood oxygenation.
Psychopharmacology 191, 813–822.
Knutson, B., and Greer, S. M. (2008). Anticipatory affect: neural correlates and consequences for choice.
Philos. Trans. R. Soc. Lond., B, Biol. Sci. 363, 3771–3786.
Knutson, B., Rick, S., Wirnmer, G. E., Prelec, D., and Loewenstein, G. (2007). Neural predictors of purchases.
Neuron 53, 147–156.
Knutson, B., Taylor, J., Kaufman, M., Peterson, R., and Glover, G. (2005). Distributed neural representation of expected value.
J. Neurosci. 25, 4806–4812.
Knutson, B., Westdorp, A., Kaiser, E., and Hommer, D. (2000). FMRI visualization of brain activity during a monetary incentive delay task.
Neuroimage 12, 20–27.
Levita, L., Hare, T. A., Voss, H. U., Glover, G., Ballon, D. J., and Casey, B. J. (2009). The bivalent side of the nucleus accumbens.
Neuroimage 44, 1178–1187.
Ljungberg, T., Apicella, P., and Schultz, W. (1992). Responses of monkey dopamine neurons during learning of behavioral reactions.
J. Neurophysiol. 67, 145–163.
Matsumoto, M., and Hikosaka, O. (2009). Two types of dopamine neuron distinctly convey positive and negative motivational signals.
Nature 459, 837–841.
Mobbs, D., Yu, R., Meyer, M., Passamonti, L., Seymour, B., Calder, A. J., Schweizer, S., Frith, C. D., and Dalgleish, T. (2009). A key role for similarity in vicarious reward.
Science 324, 900.
Moll, J., Krueger, F., Zahn, R., Pardini, M., de Oliveira-Souzat, R., and Grafman, J. (2006). Human fronto-mesolimbic networks guide decisions about charitable donation.
Proc. Natl. Acad. Sci. USA 103, 15623–15628.
Montague, P. R., and Berns, G. S. (2002). Neural economics and the biological substrates of valuation.
Neuron 36, 265–284.
O’Doherty, J., Dayan, P., Schultz, J., Deichmann, R., Friston, K., and Dolan, R. J. (2004). Dissociable roles of ventral and dorsal striatum in instrumental conditioning.
Science 304, 452–454.
Pecina, S., and Berridge, K. C. (2005). Hedonic hot spot in nucleus accumbens shell: where do mu-opioids cause increased hedonic impact of sweetness?
J. Neurosci. 25, 11777–11786.
Phillips, P. E., Walton, M. E., and Jhou, T. C. (2007). Calculating utility: preclinical evidence for cost-benefit analysis by mesolimbic dopamine.
Psychopharmacology (Berl.) 191, 483–495.
Pizzagalli, D. A., Holmes, A. J., Dillon, D. G., Goetz, E. L., Birk, J. L., Bogdan, R., Dougherty, D. D., Iosifescu, D. V., Rauch, S. L., and Fava, M. (2009). Reduced caudate and nucleus accumbens response to rewards in unmedicated individuals with major depressive disorder.
Am. J. Psychiatry 166, 702–710.
Pulos, S., Elison, J., and Lennon, R. (2004). The hierarchical structure of the Interpersonal Reactivity Index.
Soc. Behav. Pers. 32, 355–359.
Rangel, A., Camerer, C., and Montague, P. R. (2008). A framework for studying the neurobiology of value-based decision making.
Nat. Rev. Neurosci. 9, 545–556.
Rilling, J. K., Gutman, D. A., Zeh, T. R., Pagnoni, G., Berns, G. S., and Kilts, C. D. (2002). A neural basis for social cooperation.
Neuron 35, 395–405.
Rushton, J. P., Chrisjohn, R. D., and Fekken, G. C. (1981). The altruistic personality and the Self-Report Altruism Scale.
Pers. Individ. Dif. 2, 293–302.
Salamone, J. D., Correa, M., Farrar, A., and Mingote, S. M. (2007). Effort-related functions of nucleus accumbens dopamine and associated forebrain circuits.
Psychopharmacology 191, 461–482.
Schlagenhauf, F., Juckel, G., Koslowski, M., Kahnt, T., Knutson, B., Dembler, T., Kienast, T., Gallinat, J., Wrase, J., and Heinz, A. (2008). Reward system activation in schizophrenic patients switched from typical neuroleptics to olanzapine.
Psychopharmacology 196, 673–684.
Schultz, W., Dayan, P., and Montague, P. R. (1997). A neural substrate of prediction and reward.
Science 275, 1593–1599.
Scott, D. J., Stohler, C. S., Egnatuk, C. M., Wang, H., Koeppe, R. A., and Zubieta, J. K. (2008). Placebo and nocebo effects are defined by opposite opioid and dopaminergic responses.
Arch. Gen. Psychiatry 65, 220–231.
Seymour, B., Daw, N., Dayan, P., Singer, T., and Dolan, R. (2007). Differential encoding of losses and gains in the human striatum.
J. Neurosci. 27, 4826–4831.
Seymour, B., O’Doherty, J. P., Dayan, P., Koltzenburg, M., Jones, A. K., Dolan, R. J., Friston, K. J., and Frackowiak, R. S. (2004). Temporal difference models describe higher-order learning in humans.
Nature 429, 664–667.
Seymour, B., O’Doherty, J. P., Koltzenburg, M., Wiech, K., Frackowiak, R., Friston, K., and Dolan, R. (2005). Opponent appetitive-aversive neural processes underlie predictive learning of pain relief.
Nat. Neurosci. 8, 1234–1240.
Singer, T., Seymour, B., O’Doherty, J. P., Stephan, K. E., Dolan, R. J., and Frith, C. D. (2006). Empathic neural responses are modulated by the perceived fairness of others.
Nature 439, 466–469.
Strohle, A., Stoy, M., Wrase, J., Schwarzer, S., Schlagenhauf, F., Huss, M., Hein, J., Nedderhut, A., Neumann, B., Gregor, A., Juckel, G., Knutson, B., Lehmkuhl, U., Bauer, M., and Heinz, A. (2008). Reward anticipation and outcomes in adult males with attention-deficit/hyperactivity disorder.
Neuroimage 39, 966–972.
Sutton, R. S., and Barto, A. G. (1990). Foundations of adaptive networks. In Learning and Computational Neuroscience, M. Gabriel and J. Moore, eds (Cambridge, MA, MIT Press), pp. 497–537.
Sutton, R. S., and Barto, A. G. (1998). Reinforcement Learning. Cambridge, MA, MIT Press.
Swanson, L. W. (1982). The projections of the ventral tegmental area and adjacent regions – a combined fluorescent retrograde tracer and immunofluorescence study in the rat.
Brain Res. Bull. 9, 321–353.
Tankersley, D., Stowe, C. J., and Huettel, S. A. (2007). Altruism is associated with an increased neural response to agency.
Nat. Neurosci. 10, 150–151.
Tom, S. M., Fox, C. R., Trepel, C., and Poldrack, R. A. (2007). The neural basis of loss aversion in decision-making under risk.
Science 315, 515–518.
Tversky, A., and Kahneman, D. (1974). Judgment under uncertainty – heuristics and biases.
Science 185, 1124–1131.
Ungless, M. A., Magill, P. J., and Bolam, J. P. (2004). Uniform inhibition of dopamine neurons in the ventral tegmental area by aversive stimuli.
Science 303, 2040–2042.
Valentin, V. V., Dickinson, A., and O’Doherty, J. P. (2007). Determining the neural substrates of goal-directed learning in the human brain.
J. Neurosci. 27, 4019–4026.
Wise, R. (1980). The dopamine synapse and the notion of pleasure centers in the brain.
Trends Neurosci. 3, 91–95.
Wise, R. A. (2004). Dopamine, learning and motivation.
Nat. Rev. Neurosci. 5, 483–494.
Woolrich, M. W., Ripley, B. D., Brady, M., and Smith, S. M. (2001). Temporal autocorrelation in univariate linear modeling of FMRI data.
Neuroimage 14, 1370–1386.
Worsley, K. J. (2001). Statistical analysis of activation images. In Functional MRI: An Introduction to Methods, P. Jezzard, P. M. Matthews, S. M. Smith, eds (Oxford, Oxford University Press), pp. 251–270.
Yamasue, H., Abe, O., Suga, M., Yamada, H., Rogers, M. A., Aoki, S., Kato, N., and Kasai, K. (2008). Sex-linked neuroanatomical basis of human altruistic cooperativeness.
Cereb. Cortex 18, 2331–2340.
Zink, C. F., Pagnoni, G., Martin, M. E., Dhamala, M., and Berns, G. S. (2003). Human striatal response to salient nonrewarding stimuli.
J. Neurosci. 23, 8092–8097.