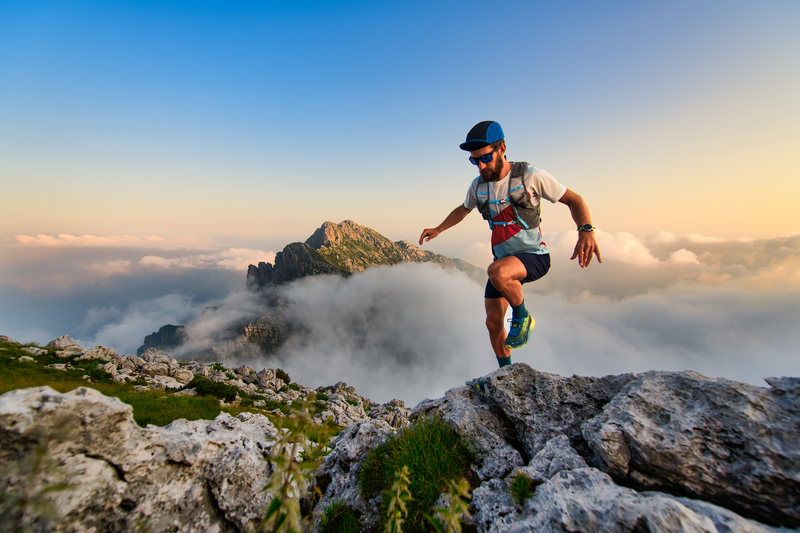
94% of researchers rate our articles as excellent or good
Learn more about the work of our research integrity team to safeguard the quality of each article we publish.
Find out more
ORIGINAL RESEARCH article
Front. Behav. Neurosci.
Sec. Learning and Memory
Volume 19 - 2025 | doi: 10.3389/fnbeh.2025.1567905
The final, formatted version of the article will be published soon.
You have multiple emails registered with Frontiers:
Please enter your email address:
If you already have an account, please login
You don't have a Frontiers account ? You can register here
Procedural Perceptual-Motor Learning (PPML) enables the acquisition of new motor procedures and is fundamental for a wide range of human behaviors. While traditional research has focused on taskrelated characteristics, there is growing interest in individual factors to account for inter-individual differences in PPML. This study aims to investigate the roles of two individual factors related to learners' strategies and mindsets: (a) explicit knowledge of the task's characteristics and regularities and (b) subjective evaluation of the task and performance. Participants were asked to answer post-learning questions about (a) their explicit knowledge of the task's rules and (b) their subjective evaluations, including perceived levels of motivation, attention, progress, stress, and tiredness. We hypothesized that (a) participants reporting explicit knowledge of the task would exhibit higher PPML scores compared to those who did not, and (b) PPML scores would be related to levels of stress, tiredness, motivation, attention, and perceived progress. We tested these hypotheses using two types of PPML tasks: motor sequence learning assessed by a Serial Reaction Time Task (SRTT) (Experiment 1) and visuomotor adaptation assessed by a Target Jumping Task (TJT) (Experiment 2). The findings of Experiment 1 revealed that participants reporting explicit knowledge of the SRTT exhibited higher learning scores, which were related to perceived stress and progress. In Experiment 2, participants reporting explicit knowledge of the TJT exhibited lower learning scores, which were related to perceived stress, tiredness, concentration, and progress. This study offers a novel and comprehensive perspective on interindividual differences in PPML by considering the roles of explicit knowledge and subjective evaluations in two types of PPML tasks. Although further replication and generalization are necessary, the findings provide valuable insights into how learner-task interactions may explain inter-individual differences and highlight the importance of considering participants' subjective reports research for future studies on PPML. They also provide interesting perspectives for clinical interventions dedicated to populations with PPML disorders (e.g., Developmental Coordination Disorder, Cerebral Palsy, Parkinson disease, etc).
Keywords: sequence learning, Serial Reaction Time Task, SRTT, visuomotor adaptation, double step pointing paradigm, target jumping task, subjective reports
Received: 28 Jan 2025; Accepted: 20 Mar 2025.
Copyright: © 2025 Seiwert, Martin, Lagarrigue, Amarantini, Fautrelle, Tisseyre and TALLET. This is an open-access article distributed under the terms of the Creative Commons Attribution License (CC BY). The use, distribution or reproduction in other forums is permitted, provided the original author(s) or licensor are credited and that the original publication in this journal is cited, in accordance with accepted academic practice. No use, distribution or reproduction is permitted which does not comply with these terms.
* Correspondence:
Sarah Seiwert, ToNIC, Toulouse NeuroImaging Center, UMR 1214, University of Toulouse, INSERM, Paul Sabatier University (UT3), Toulouse, France
Disclaimer: All claims expressed in this article are solely those of the authors and do not necessarily represent those of their affiliated organizations, or those of the publisher, the editors and the reviewers. Any product that may be evaluated in this article or claim that may be made by its manufacturer is not guaranteed or endorsed by the publisher.
Research integrity at Frontiers
Learn more about the work of our research integrity team to safeguard the quality of each article we publish.