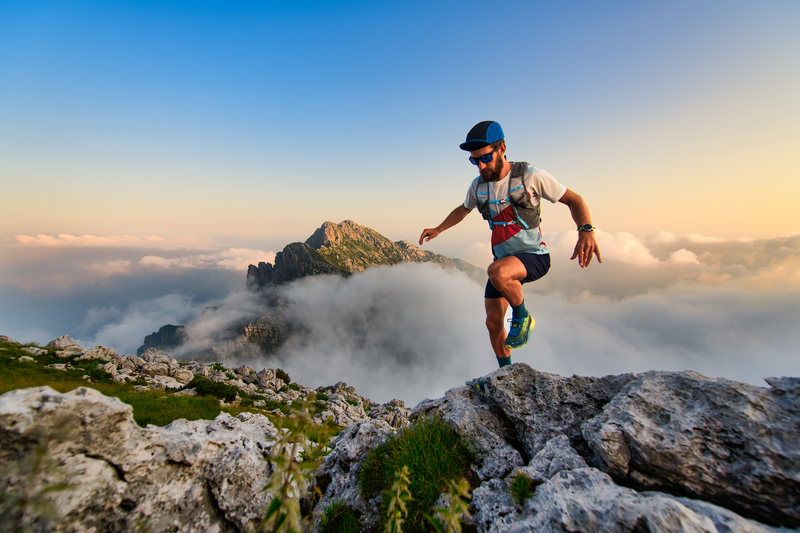
95% of researchers rate our articles as excellent or good
Learn more about the work of our research integrity team to safeguard the quality of each article we publish.
Find out more
SYSTEMATIC REVIEW article
Front. Behav. Neurosci. , 07 January 2025
Sec. Learning and Memory
Volume 18 - 2024 | https://doi.org/10.3389/fnbeh.2024.1457663
Reasoning is a fundamental cognitive process that allows individuals to make inferences, decisions, and solve problems. Understanding the neural mechanisms of reasoning and the gender differences in these mechanisms is crucial for comprehending the neural foundations of reasoning and promoting gender equality in cognitive processing. This study conducted an Activation Likelihood Estimation (ALE) meta-analysis of 275 studies, revealing that reasoning involves multiple brain regions, including the parts of frontal, parietal, occipital, temporal lobes, limbic system, and subcortical areas. These findings indicate that reasoning is a complex cognitive process requiring the coordinated activity of multiple brain regions. Additionally, 25 studies focusing on the Wisconsin Card Sorting Test (WCST) paradigm confirmed the importance of these regions in reasoning processes. The gender-specific activation results indicate that males and females utilize different neural networks during reasoning and WCST tasks. While significant differences exist in specific regions, the overall activation patterns do not show marked gender differences. Notably, females exhibit greater activation in the limbic system compared to males, suggesting that emotional states may play a more prominent role for females when engaging in reasoning tasks.
Reasoning is an advanced cognitive process that enables individuals to make inferences, decisions, and solve problems. It is a crucial aspect of human intelligence, underpinning various daily activities from social interactions to conducting complex scientific analyses. Understanding the neural mechanisms behind reasoning helps to elucidate how the brain supports these higher cognitive functions, aligning with the goals of cognitive neuroscience to connect brain function with neural substrates (Poldrack, 2010). Consequently, research utilizing neuroimaging tools has emphasized the role of reasoning in analyzing social cognitive processes (Lieberman, 2010) and exploring the neural processes of reasoning (Calzavarini and Cevolani, 2022).
Numerous neuroimaging studies have investigated the neural correlates of reasoning (Melrose et al., 2007) found that the prefrontal-striatal circuit is involved in abstract reasoning. Other studies have shown that deductive reasoning activates parietal-occipital regions (Prado et al., 2011), and paradoxical reasoning activates the prefrontal/orbital frontal cortex. The prefrontal cortex (PFC) is crucial for both conditional and syllogistic reasoning, playing a key role in integrating information and managing cognitive tasks (Wertheim and Ragni, 2020). These studies used different concepts and paradigms of reasoning, leading to various identified brain activation regions. Nevertheless, activation of DLPFC and parietal regions during reasoning is widely recognized (Christoff et al., 2001; Kroger et al., 2002; Prabhakaran et al., 1997). The rostrolateral PFC may also significantly contribute to reasoning (Modi et al., 2018). A meta-analysis of fMRI studies highlights the collaboration between the PFC and parietal regions in supporting relational reasoning by processing and integrating complex information (Prado et al., 2011). And, during problem-solving, connectivity between the PFC and regions like the parietal lobe is essential for efficient reasoning and decision-making (Bartley et al., 2018).
Additionally, cognitive neuroscience research increasingly focuses on exploring gender differences in brain structure and function. Studies have demonstrated differences in brain activity and cognitive performance between men and women, highlighting the importance of considering gender factors in cognitive neuroscience research (Grady, 2008). There have been studies on gender differences in different reasoning tasks. For example, Prado et al. (2011) found that while males and females perform similarly on deductive reasoning tasks (e.g., syllogistic reasoning, conditional inferences), they show different brain activation patterns. Males tend to activate the left parietal and occipital regions more, whereas females are more likely to activate prefrontal regions. Gur et al. (2000) discovered that males activate more of the right parietal region during spatial reasoning tasks (e.g., mental rotation, spatial visualization), while females tend to activate the left prefrontal cortex. Furthermore, research has shown that females exhibit more significant activation in the medial prefrontal cortex (mPFC) and superior temporal sulcus (STS) during tasks involving emotion and social cognition, whereas males show more pronounced activation in the prefrontal cortex during tasks involving rational analysis (Schlaffke et al., 2015). Therefore, understanding the neural mechanisms of reasoning and the neural differences between gender is crucial for comprehending the neural foundations of reasoning and promoting gender equality in reasoning abilities.
This study aims to utilize existing neuroimaging data to conduct a comprehensive meta-analysis of the neural imaging studies on reasoning and its typical experimental paradigm, the Wisconsin Card Sorting Test (WCST), to elucidate gender-specific brain activation patterns in reasoning functions. The WCST is a well-established neuropsychological test that evaluates executive functions such as cognitive flexibility, problem-solving, and the ability to shift strategies in response to changing environmental contingencies (Grant and Berg, 1993). These functions are critical components of reasoning, as they require individuals to adapt and apply logical principles to solve novel problems. Our choice of the WCST was motivated by its robust ability to engage and measure the cognitive processes underlying reasoning, making it a valuable tool for investigating the neural mechanisms involved.
Using the Activation Likelihood Estimation (ALE) method, we systematically identify consistent activation patterns associated with reasoning tasks in males and females. First, ALE allows for the comprehensive aggregation of data from multiple neuroimaging studies, identifying consistent brain activation patterns across different datasets, which enhances the generalizability of findings (Eickhoff et al., 2012), also statistically robust, employing probabilistic modeling and permutation testing to ensure the significance of observed activation patterns, reducing the risk of false positives (Laird et al., 2005a). Turkeltaub et al. (2002) accounts for variability across studies, such as differences in scanner resolution and participant populations, by modeling activation foci as probability distributions. This makes it ideal for comparing sex-specific brain activation. Moreover, Laird et al. (2009) proposed a meta-analytic activation consistency mapping (MACM) method that can identify common activation patterns in functionally related regions, and ALE facilitates subgroup analysis, enabling the detection of distinct neural activation patterns between males and females, which is essential for uncovering sex-specific differences in reasoning (Kohn et al., 2014). Overall, ALE’s ability te data from diverse studies provides a more generalized and reliable understanding of the neural mechanisms underlying reasoning and their potential gender differences(Costafreda, 2012; Fox et al., 2005). This approach provides a robust and generalized understanding of the sex differences in the neural foundations of reasoning.
To verify the neural basis of reasoning, specifically the neural basis of reasoning across genders, the BrainMap database was queried using Sleuth version 3.0.4 (Fox et al., 2005; Laird et al., 2009; Laird et al., 2005b). Sleuth1 is a free, publicly available search tool that allows users to search the BrainMap database according to two different categories (Hill et al., 2014). As an important note in terminology in the literature and BrainMap database search, we chose to use “gender” rather than “sex” throughout our study. We formulated the following search criteria:
(1) Find researches on reasoning or using the WCST paradigm (e.g., Experiments → Behavioral Domain → Cognition → Reasoning).
(2) Studies reporting only activation results (e.g., Experiments → Activation → Activations (or Deactivations) Only).
(3) Studies using normal, healthy subjects (e.g., Experiments → Context → Normal Mapping).
(4) Studies using three searches for gender: only males or only females or no restrict for gender (e.g., Subjects → Gender → Females Only).
The global activation and deactivation coordinates of the search results were obtained. The comprehensive results for reasoning were shown as Table 1.
In addition to these general reasoning findings, our search also identified a subset of studies that specifically utilized the WCST paradigm. These WCST-related studies reported activation patterns associated with this reasoning-based cognitive task. For the WCST paradigm, the results of activation were:
(1) All studies: 25 papers, 908 locations, 113 experiments, 83 conditions, 765 subjects.
(2) Female-specific studies: 4 papers, 91 locations, 23 experiments, 8 conditions, 157 subjects.
(3) Male-specific studies: 5 papers, 298 locations, 17 experiments, 16 conditions, 57 subjects.
For the WCST studies, in addition to the activation findings, the search identified 4 papers that reported deactivations (decreases in neural activity) associated with this reasoning task. These 4 WCST studies reported a total of 47 deactivation locations, 11 experiments, and 20 conditions, involving 44 participants. However, the BrainMap database did not contain any gender-specific data for these deactivation findings - the results were not stratified by male or female participants. The obtained results were then downloaded locally. GingerALE2 is a commonly used tool for coordinate-based meta-analysis. The ALE method, first proposed by Laird et al. (2005a) and further verified and improved by Turkeltaub et al. (2002), has been enhanced by Eickhoff et al. (2009) and implemented in the statistical toolbox GingerALE. Using the extracted coordinate values, we performed meta-analyses on the peak coordinate points for the different results using GingerALE. In addition, in the exploration of the neural mechanisms related to reasoning, the results of the image meta-analysis of reasoning were used as a reference, and the largest 5 ROIs were set for MACM.
In ALE meta-analysis, the False Discovery Rate (FDR) correction is a commonly used method for multiple comparison corrections. The specific calculation of FDR depends on the number of permutation tests performed (Laird et al., 2005a). In this study, we conservatively corrected ALE statistical images using FDR (FDR corrected p-value < 0.05) and set a minimum clustering threshold of 200 mm3. This correction method helps control the risk of Type I errors, allowing for more reliable identification of consistent and stable activation patterns in our integrated analysis. Additionally, it ensures that the discovered activation regions have high credibility.
For visualization, we utilized the Workbench3 software to present the neural activation images. Workbench is a powerful tool for viewing and analyzing neuroimaging data, allowing us to effectively illustrate the gender-specific neural activation patterns associated with reasoning.
The ALE meta-analysis of 275 studies identified 10 clusters, with the largest cluster having a volume of 119,576 mm3 and containing 26 peak points, and the largest ALE value is 0.218. This comprehensive list of brain regions highlights the widespread involvement of frontal, parietal, occipital, temporal, limbic, and subcortical areas in supporting reasoning processes (Figure 1). The main regions include the left and right inferior frontal gyrus (BA9), middle frontal gyrus (BA9), and superior frontal gyrus (BA6); the inferior parietal lobule (BA40) and superior parietal lobule (BA7); the precuneus (BA7); the inferior occipital gyrus (BA18) and middle occipital gyrus (BA19); the fusiform gyrus (BA37); the anterior cingulate (BA24); and the parahippocampal gyrus. Detailed brain regions can be found in Supplementary Table 1. These areas collectively illustrate the extensive neural network engaged in reasoning.
Figure 1. Shows the activation maps of different brain regions in both the left and right hemispheres, as well as medial and lateral views, without distinguishing by gender, threshold at p < 0.05, FDR-corrected. (A) Represents the activation map dominated by behavioral tasks, while (B) represents the activation map dominated by the WCST paradigm.
The results of the 25 studies on the Wisconsin Card Sorting Test (WCST) are largely encompassed by the brain activation results related to reasoning, with the locations of peak points closely aligning with the peak locations of reasoning activation. There are 18 clusters in total, the largest of which has a volume of 11,944 mm3 and an ALE value of 0.051. Detailed brain regions can be found in Supplementary Table 2.
By ALE meta-analysis of the experiments in BrainMap, the central MNI coordinates of the five largest clusters were used to create 5 mm ROI spheres and were entered into the BrainMap database to search for all activations reported within the boundaries of each ROI. Based on the results obtained, the MACM map of the five selected seed ROIs was calculated, and the activation focus closest to each seed ROI showed a significantly higher probability of co-activation (Figure 2).
Figure 2. Presents the brain regions showing consistent activations associated with reasoning tasks.
The meta-analysis of 29 studies identified 74 clusters, with the largest cluster having a volume of 20,464 mm3 and containing 14 peak activations with a maximum ALE value of 0.021(Figure 3A). These values are much lower than typical positive activations and differ from the patterns of positive activation, primarily involving BA 10, 32, 40, 39, and 31. The results from 4 studies using WCST, largely showed brain deactivations (Figure 3B). The peak coordinates of these deactivations were in close proximity to the peak locations of the reasoning-related deactivations. In total, there were 16 clusters, with the largest cluster having a volume of 19,712 mm3 and an ALE value of 0.011.
Figure 3. Shows the same content as the previous graph, except that this graph is the result of deactivation. (A) Shows the deactivation graph related mainly to reasoning-related behavioral tasks, and (B) shows the deactivation graph mainly based on studies using the WCST paradigm.
Further analysis based on gender-specific activation patterns during reasoning and WCST tasks revealed distinct neural networks for males and females as shown in Figure 4A. The study highlights specific brain regions where different genders show significant neural activity related to reasoning. For females, notable activations include the right sub-lobar insula (BA 13), the left inferior parietal lobule (BA 40), and the right cingulate gyrus (BA 32). The largest cluster for females measures 1,664 mm3. For males, significant activations are observed in the left superior parietal lobule (BA 7), the left precuneus (BA 19), and the right inferior frontal gyrus (BA 46). The largest cluster for males measures 12,464 mm3.
Figure 4. Shows the activation maps of different brain regions for males and females from left, right, medial, and lateral views, threshold at p < 0.05, FDR-corrected. Different colors are used to represent these differences, with varying shades indicating the degree of activation: green represents female brain activation maps, and blue represents male brain activation maps. (A) Shows behavior-driven activation maps, while (B) shows WSCT paradigm-driven activation maps.
The gender differences in WCST activation, showing in Figure 4B. In females, additional areas of activation include the left cingulate gyrus (BA 32), the right medial frontal gyrus (BA 47), and the bilateral parahippocampal gyrus (BA 19 and 30). In contrast, males show prominent activity in the left superior parietal lobule (BA 7) and the right precuneus (BAs 19 and 31). Detailed gender-specific reasoning and WCST activation maps can be found in Supplementary Tables 3–6. The findings emphasize the different neural bases involved in reasoning and WCST tasks for males and females, reflecting gender-specific neural networks engaged in these cognitive processes. Although less articles were found and analyzed, the small amount of data was not sufficient to support the authenticity of the results, so the results of deactivation were not reflected in gender differences.
We also performed a quantitative comparison of the resulting ALE maps using the GingerALE program within the BrainMap environment to objectively determine the differences between male and female networks in a statistically sound manner. GingerALE achieves this by subtracting two ALE result images. Similar to traditional ALE analyses, GingerALE pools the coordinates from the original datasets and randomly assigns them into two new groups of the same size as the original datasets. These new pairings are then subtracted (i.e., using permutations to create and compare the null distribution of the real data). The resulting images are converted into z-score maps as shown in Figure 5.
Figure 5. The contrast analysis of the resultant ALE maps from females to males, threshold at p < 0.05, FDR-corrected.
The analysis of the “females minus males” results revealed that there are still 18 clusters with volumes exceeding 200 mm3 in the reasoning task (showed Table 2), primarily distributed in the prefrontal cortex and limbic system, as detailed in the figure below. However, the males minus females results and the gender differences driven by the WCST paradigm did not survive correction.
Table 2. Significant clusters (FDR p < 0.05) of contrast from females to males about reasoning revealed by the ALE analysis.
An ALE meta-analysis of 275 studies provided a comprehensive overview of the brain regions involved in reasoning, revealing the extensive neural network engaged in this cognitive process. The reasoning process involves multiple brain regions, including the frontal, parietal, occipital, and temporal lobes, the limbic system, and subcortical areas, indicating that reasoning is a complex cognitive process requiring the coordination of several brain areas. The frontal regions, particularly the inferior, middle, and superior frontal gyri, are well known for their roles in higher cognitive functions, including planning, decision-making, and problem-solving (Fuster, 2001; Miller and Cohen, 2001). The parietal lobes, especially the inferior and superior parietal lobules, are crucial for the integration of sensory information and spatial reasoning (Culham et al., 2001). The occipital lobe and the fusiform gyrus are primarily involved in visual processing, which is essential for reasoning tasks involving visual stimuli or spatial manipulation (Grill-Spector and Malach, 2004; Kanwisher, 2010). The anterior cingulate cortex and the parahippocampal gyrus are involved in emotion regulation and memory, respectively (Bush et al., 2000; Eichenbaum, 2004), highlighting the importance of these functions in integrated reasoning (Dockès et al., 2020). Considering the brain regions and their corresponding functions, reasoning emerges as a complex higher-order function involving multiple cognitive processes.
The findings from 25 studies on the WCST further corroborate the broader findings of reasoning studies. The WCST is a widely used neuropsychological test that assesses executive functions, including cognitive flexibility, problem-solving, and abstract thinking (Grant and Berg, 1993). Although the WCST is primarily used to study executive functions, the peak activations in WCST studies align closely with those identified in the reasoning meta-analysis, suggesting that the cognitive processes involved in the WCST are closely related to reasoning abilities.
Gender-specific activation results indicate different neural networks for males and females in reasoning and WCST tasks, highlighting significant differences in brain activity patterns. In studies on female reasoning, significant activation areas are mainly concentrated in the deep brain regions of the limbic system, emphasizing regions associated with emotion processing, sensory information integration, and cognitive control (Bush et al., 2000; Eichenbaum, 2004). In contrast, studies on male reasoning show significant activation in the occipital, parietal, and parts of the frontal cortical areas, emphasizing regions involved in visuospatial processing, attention, and executive functions (Bush et al., 2000; Grill-Spector and Malach, 2004; Kanwisher, 2010). These differences suggest that males and females may employ different strategies or neural pathways to accomplish reasoning tasks. The WCST studies also reflect this gender difference to some extent.
Beyond describing the above gender results, we quantitatively compared gender differences in reasoning and tasks using GingerALE. The results showed that compared to males, females activate parts of the prefrontal and limbic systems, indicating that emotion processing is also involved in females’ handling of cognitive and complex decision-making tasks. This is consistent with reviews on gender in neuroscience (Cahill, 2006) and findings on gender in learning and memory (Andreano and Cahill, 2009). However, the “male minus female” results and gender differences driven by the WCST paradigm are corrected. Women may use more contextualized strategies in cognitive tasks, which affects brain region activation in executive function tasks (Miller and Halpern, 2014). In addition, the activity of the limbic system is related to differences in emotional processing and may affect women’s task performance (Laird et al., 2005a). Research has also found that women show stronger prefrontal cortex connectivity during certain tasks, which is associated with greater neural integration abilities (Prabhakaran et al., 1997). Finally, hormonal fluctuations have significant effects on women’s performance on emotional and executive function tasks (Bartley et al., 2018). This suggests that while there are significant differences in specific regions, the overall activation patterns between genders may not be as prominent when considering the entire neural network involved in these tasks. This partially supports studies indicating no significant gender differences in reasoning (Miller and Halpern, 2014).
The original contributions presented in this study are included in this article/Supplementary material, further inquiries can be directed to the corresponding author.
LC: Data curation, Formal analysis, Methodology, Writing – original draft, Writing – review and editing. ZZ: Data curation, Formal analysis, Project administration, Software, Writing – review and editing. JL: Conceptualization, Investigation, Writing – review and editing. YL: Software, Visualization, Writing – review and editing. QM: Conceptualization, Funding acquisition, Project administration, Resources, Supervision, Writing – review and editing.
The author(s) declare that financial support was received for the research, authorship, and/or publication of this article. This study was supported by the National Social Science Fund General Project (No. 19BYY084), the Northwest Normal University Project (No. NWNU-SKQN2023-15), and the Beijing Normal University Doctoral Interdisciplinary Fund Project (No. BNUXKJC2112).
The authors declare that the research was conducted in the absence of any commercial or financial relationships that could be construed as a potential conflict of interest.
All claims expressed in this article are solely those of the authors and do not necessarily represent those of their affiliated organizations, or those of the publisher, the editors and the reviewers. Any product that may be evaluated in this article, or claim that may be made by its manufacturer, is not guaranteed or endorsed by the publisher.
The Supplementary Material for this article can be found online at: https://www.frontiersin.org/articles/10.3389/fnbeh.2024.1457663/full#supplementary-material
Andreano, J. M., and Cahill, L. (2009). Sex influences on the neurobiology of learning and memory. Learn. Mem. 16, 248–266. doi: 10.1101/lm.918309
Bartley, J. E., Boeving, E. R., Riedel, M. C., Bottenhorn, K. L., Salo, T., Eickhoff, S. B., et al. (2018). Meta-analytic evidence for a core problem solving network across multiple representational domains. Neurosci. Biobehav. Rev. 92, 318–337. doi: 10.1016/j.neubiorev.2018.06.009
Bush, G., Luu, P., and Posner, M. I. (2000). Cognitive and emotional influences in anterior cingulate cortex. Trends Cogn. Sci. 4, 215–222. doi: 10.1016/S1364-6613(00)01483-2
Cahill, L. (2006). Why sex matters for neuroscience. Nat. Rev. Neurosci. 7, 477–484. doi: 10.1038/nrn1909
Calzavarini, F., and Cevolani, G. (2022). Abductive reasoning in cognitive neuroscience: weak and strong reverse inference. Synthese 200:70. doi: 10.1007/s11229-022-03585-2
Christoff, K., Prabhakaran, V., Dorfman, J., Zhao, Z., Kroger, J. K., Holyoak, K. J., et al. (2001). Rostrolateral prefrontal cortex involvement in relational integration during reasoning. Neuroimage 14, 1136–1149. doi: 10.1006/nimg.2001.0922
Costafreda, S. G. (2012). Parametric coordinate-based meta-analysis: valid effect size meta-analysis of studies with differing statistical thresholds. J. Neurosci. Methods 210, 291–300. doi: 10.1016/j.jneumeth.2012.07.016
Culham, J. C., Cavanagh, P., and Kanwisher, N. G. (2001). Attention response functions: characterizing brain areas using fMRI activation during parametric variations of attentional load. Neuron 32, 737–745. doi: 10.1016/S0896-6273(01)00499-8
Dockès, J., Poldrack, R. A., Primet, R., Gözükan, H., Yarkoni, T., Suchanek, F., et al. (2020). NeuroQuery, comprehensive meta-analysis of human brain mapping. Elife 9:e53385. doi: 10.7554/eLife.53385
Eichenbaum, H. (2004). Hippocampus: cognitive processes and neural representations that underlie declarative memory. Neuron 44, 109–120. doi: 10.1016/j.neuron.2004.08.028
Eickhoff, S. B., Bzdok, D., Laird, A. R., Kurth, F., and Fox, P. T. (2012). Activation likelihood estimation meta-analysis revisited. Neuroimage 59, 2349–2361. doi: 10.1016/j.neuroimage.2011.09.017
Eickhoff, S. B., Laird, A. R., Grefkes, C., Wang, L. E., Zilles, K., and Fox, P. T. (2009). Coordinate-based activation likelihood estimation meta-analysis of neuroimaging data: a random-effects approach based on empirical estimates of spatial uncertainty. Hum. Brain Mapp. 30, 2907–2926. doi: 10.1002/hbm.20718
Fox, P. T., Laird, A. R., Fox, S. P., Fox, P. M., Uecker, A. M., Crank, M., et al. (2005). BrainMap taxonomy of experimental design: description and evaluation. Hum. Brain Mapp. 25, 185–198. doi: 10.1002/hbm.20141
Fuster, J. M. (2001). The prefrontal cortex—an update: time is of the essence. Neuron 30, 319–333. doi: 10.1016/S0896-6273(01)00285-9
Grady, C. L. (2008). Cognitive neuroscience of aging. Ann. N. Y. Acad. Sci. 1124, 127–144. doi: 10.1196/annals.1440.009
Grant, D. A., and Berg, E. A. (1993). Wisconsin card sorting test. J. Exp. Psychol. 38:404. doi: 10.1037/h0059831
Grill-Spector, K., and Malach, R. (2004). The human visual cortex. Annu. Rev. Neurosci. 27, 649–677. doi: 10.1146/annurev.neuro.27.070203.144220
Gur, R. C., Alsop, D., Glahn, D., Petty, R., Swanson, C. L., Maldjian, J. A., et al. (2000). An fMRI study of sex differences in regional activation to a verbal and a spatial task. Brain Lang. 74, 157–170. doi: 10.1006/brln.2000.2325
Hill, A. C., Laird, A. R., and Robinson, J. L. (2014). Gender differences in working memory networks: a BrainMap meta-analysis. Biol. Psychol. 102, 18–29. doi: 10.1016/j.biopsycho.2014.06.008
Kanwisher, N. (2010). Functional specificity in the human brain: a window into the functional architecture of the mind. Proc. Natl Acad. Sci. U.S.A. 107, 11163–11170. doi: 10.1073/pnas.1005062107
Kohn, N., Eickhoff, S. B., Scheller, M., Laird, A. R., Fox, P. T., and Habel, U. (2014). Neural network of cognitive emotion regulation—an ALE meta-analysis and MACM analysis. Neuroimage 87, 345–355. doi: 10.1016/j.neuroimage.2013.11.001
Kroger, J. K., Sabb, F. W., Fales, C. L., Bookheimer, S. Y., Cohen, M. S., and Holyoak, K. J. (2002). Recruitment of anterior dorsolateral prefrontal cortex in human reasoning: a parametric study of relational complexity. Cereb. Cortex 12, 477–485. doi: 10.1093/cercor/12.5.477
Laird, A. R., Eickhoff, S. B., Kurth, F., Fox, P. M., Uecker, A. M., Turner, J. A., et al. (2009). ALE meta-analysis workflows via the brainmap database: progress towards a probabilistic functional brain atlas. Front. Neuroinform. 3:23. doi: 10.3389/neuro.11.023.2009
Laird, A. R., Fox, P. M., Price, C. J., Glahn, D. C., Uecker, A. M., Lancaster, J. L., et al. (2005a). ALE meta-analysis: controlling the false discovery rate and performing statistical contrasts. Hum. Brain Mapp. 25, 155–164. doi: 10.1002/hbm.20136
Laird, A. R., Lancaster, J. J., and Fox, P. T. (2005b). BrainMap: the social evolution of a human brain mapping database. Neuroinformatics 3, 65–77. doi: 10.1385/NI:3:1:065
Lieberman, M. D. (2010). Social cognitive neuroscience. Handb. Soc. Psychol. 5, 143–193. doi: 10.1002/9780470561119.socpsy001005
Melrose, R. J., Poulin, R. M., and Stern, C. E. (2007). An fMRI investigation of the role of the basal ganglia in reasoning. Brain Res. 1142, 146–158. doi: 10.1016/j.brainres.2007.01.060
Miller, D. I., and Halpern, D. F. (2014). The new science of cognitive sex differences. Trends Cogn. Sci. 18, 37–45. doi: 10.1016/j.tics.2013.10.011
Miller, E. K., and Cohen, J. D. (2001). An integrative theory of prefrontal cortex function. Annu. Rev. Neurosci. 24, 167–202. doi: 10.1146/annurev.neuro.24.1.167
Modi, S., Kumar, M., Nara, S., Kumar, P., and Khushu, S. (2018). Trait anxiety and neural efficiency of abstract reasoning: an fMRI investigation. J. Biosci. 43, 877–886. doi: 10.1007/s12038-018-9800-3
Poldrack, R. A. (2010). Mapping mental function to brain structure: how can cognitive neuroimaging succeed? Perspect. Psychol. Sci. 5, 753–761. doi: 10.1177/1745691610388777
Prabhakaran, V., Smith, J. A. L., Desmond, J. E., Glover, G. H., and Gabrieli, J. D. E. (1997). Neural substrates of fluid reasoning: an fMRI study of neocortical activation during performance of the Raven’s Progressive Matrices Test. Cogn. Psychol. 33, 43–63. doi: 10.1006/cogp.1997.0659
Prado, J., Chadha, A., and Booth, J. R. (2011). The brain network for deductive reasoning: a quantitative meta-analysis of 28 neuroimaging studies. J. Cogn. Neurosci. 23, 3483–3497. doi: 10.1162/jocn_a_00063
Schlaffke, L., Lissek, S., Lenz, M., Juckel, G., Schultz, T., Tegenthoff, M., et al. (2015). Shared and nonshared neural networks of cognitive and affective theory-of-mind: a neuroimaging study using cartoon picture stories. Hum. Brain Mapp. 36, 29–39. doi: 10.1002/hbm.22610
Turkeltaub, P. E., Eden, G. F., Jones, K. M., and Zeffiro, T. A. (2002). Meta-analysis of the functional neuroanatomy of single-word reading: method and validation. Neuroimage 16, 765–780. doi: 10.1006/nimg.2002.1131
Keywords: reasoning, gender differences, meta-analysis, ALE, WCST
Citation: Chen L, Zheng Z, Liang J, Lin Y and Miao Q (2025) Understanding gender differences in reasoning and specific paradigm using meta-analysis of neuroimaging. Front. Behav. Neurosci. 18:1457663. doi: 10.3389/fnbeh.2024.1457663
Received: 01 July 2024; Accepted: 11 December 2024;
Published: 07 January 2025.
Edited by:
Dezhi Liao, University of Minnesota Twin Cities, United StatesReviewed by:
Jennifer T. Sneider, McLean Hospital, United StatesCopyright © 2025 Chen, Zheng, Liang, Lin and Miao. This is an open-access article distributed under the terms of the Creative Commons Attribution License (CC BY). The use, distribution or reproduction in other forums is permitted, provided the original author(s) and the copyright owner(s) are credited and that the original publication in this journal is cited, in accordance with accepted academic practice. No use, distribution or reproduction is permitted which does not comply with these terms.
*Correspondence: Qingqing Miao, bWlhb3FxQG53bnUuZWR1LmNu
Disclaimer: All claims expressed in this article are solely those of the authors and do not necessarily represent those of their affiliated organizations, or those of the publisher, the editors and the reviewers. Any product that may be evaluated in this article or claim that may be made by its manufacturer is not guaranteed or endorsed by the publisher.
Research integrity at Frontiers
Learn more about the work of our research integrity team to safeguard the quality of each article we publish.