- 1CAS Key Laboratory of Behavioral Science, Institute of Psychology, Beijing, China
- 2Department of Psychology, University of Chinese Academy of Sciences, Beijing, China
Wellbeing refers to cognitive and emotional appraisal of an individual’s life and social functioning, which is of great significance to the quality of life of an individual and society. Previous studies have revealed the neural basis of wellbeing, which mostly focused on human brain morphology or network-level connectivity. However, local-to-remote cortical connectivity, which plays a crucial role in defining the human brain architecture, has not been investigated in wellbeing. To examine whether wellbeing was associated with local-to-remote cortical connectivity, we acquired resting-state images from 60 healthy participants and employed the Mental Health Continuum Short Form to measure wellbeing, including three dimensions, namely, emotional wellbeing, psychological wellbeing, and social wellbeing. Functional homogeneity (ReHo) and seed-based functional connectivity were used to evaluate local-to-remote cortical connectivity in these participants. For local connectivity, our results showed that ReHo in the right orbitofrontal sulcus was significantly positively correlated with psychological wellbeing but negatively correlated with social wellbeing. For remote connectivity, connectivity within the right orbitofrontal cortex and interhemispheric connectivity of the orbitofrontal sulcus were both positively associated with psychological wellbeing; functional connectivity between the right orbitofrontal sulcus and the left postcentral sulcus was positively associated with social wellbeing. Our results showed that wellbeing was indeed associated with local-to-remote cortical connectivity, and our findings supplied a new perspective of distance-related neural mechanisms of wellbeing.
Introduction
Over decades, the increasing attention of neuroscientists has been devoted to wellbeing, which is a complex construct that pursuits optimal experience and functioning (Ryan and Deci, 2001). Wellbeing could be divided into two distinct aspects: one is hedonic wellbeing, as the hedonic aspect of subjective wellbeing, which means pleasure or happiness attainment (Diener, 1994), and the second is eudaimonic wellbeing, which means the actualization of human potentials or true value (Waterman, 1993; Ryan and Deci, 2001). Recently, Keyes (2009) integrated previous definitions of happiness and diagnosis methods of mental health and proposed that positive mental health conditions can be evaluated on the following three aspects, namely, emotional wellbeing, psychological wellbeing, and social wellbeing. Such a concept of the mental health continuum could also be used in neuropsychiatric patients (Keyes, 2007). Wellbeing is so important for the quality of an individual’s life and society, and studies on wellbeing are also important to behavioral sciences (Diener et al., 2003). In a summary, exploring the neural mechanisms of wellbeing could enrich our comprehension of how to live a happy life.
Recently, with the development of brain imaging techniques, an increasing number of neuroscientists began to investigate the neural mechanisms of wellbeing. Most of them used structural MRI (sMRI) or resting-state functional MRI (r-fMRI) to measure the human brain and used social wellbeing and subjective wellbeing to measure wellbeing. For the brain structure, Kong et al. (2015a,2019) employed the voxel-based morphometry method to reveal that both gray matter density in the orbitofrontal cortex (OFC) and gray matter volume in the left mid-dorsolateral prefrontal cortex (mid-DLPFC) were negatively correlated with social wellbeing. Meanwhile, eudaimonic wellbeing was associated with insular cortex volume (Lewis et al., 2014). Subjective wellbeing was reported to be related to subcortical brain volumes (Van’t Ent et al., 2017) as well as brain morphology in the superior temporal gyrus and the insula (Song et al., 2019). For brain function, using the regional fractional amplitude of low-frequency fluctuation (fALFF) method, Kong et al. (2016a) demonstrated that social wellbeing was positively associated with the fALFF method in the bilateral posterior superior temporal gyrus, right anterior cingulate, right thalamus, and right insula. Subjective wellbeing was negatively correlated with the fALFF in the bilateral superior frontal gyrus (SFG) and the right OFC (Kong et al., 2015b), together with that, eudaimonic wellbeing was positively correlated with fALFF in the right posterior superior temporal gyrus and the thalamus and negatively correlated with the strength of thalamic-insular connectivity (Kong et al., 2015c). There were also studies reporting that wellbeing was associated with within-network connectivity rather than between-network connectivity (Heller et al., 2013; Luo et al., 2017; Shi et al., 2018; Ren et al., 2019; Li et al., 2020), and the main networks included the fronto-parietal network, the default-mode network, and the insular network. Kong et al. (2016b) also demonstrated that local functional connectivity ReHo in the inferior frontal gyrus and the OFC was associated with wellbeing. One note was that many of the above studies detected significant mediation effects such as personality, emotional intelligence, mindfulness, pursuit, and thought patterns associated with brain-wellbeing (Vatansever et al., 2020). Since wellbeing was such a general construct involving a lot of psychological processes such as cognitive, emotional, and self, the first step should be clarifying its neural basis but not involve another behavioral measurement.
Most previous studies on wellbeing focused on correlations between wellbeing and gray matter volume, gray matter density, or r-fMRI metrics, such as fALFF and ReHo, which mainly concentrated on local characteristics of the human brain. There were few studies combining local short-range functional connectivity and remote long-range functional connectivity. Anatomical distance is of great significance to human brain architecture regarding the cost and efficiency of information processing (Jiang et al., 2015). A previous study has revealed that the development of large-scale brain networks was characterized by the weakening of short-range functional connectivity and the strengthening of long-range functional connectivity (Supekar et al., 2009). Also, individual connectivity variability related to the cortical hierarchy positively correlated with the degree of long-range connectivity but negatively correlated with the local connectivity (Mueller et al., 2013). In addition, the anatomical distance of functional connectivity played important roles on patient brains of various diseases. For example, one study suggested that the long-range connections linking dorsal anterior cingulate to posterior cingulate and precuneus should be considered as a candidate locus of dysfunction in attention-deficit hyperactivity disorder (ADHD; Castellanos et al., 2008). Guo et al. (2015) demonstrated a pattern of increased long-range connectivity in the posterior default-mode network (DMN) in schizophrenia. From the perspective of information transferring and dynamics, long-range functional connectivity was based on short-range functional connectivity as well as neurodevelopment through short-range functional connectivity (Jiang et al., 2015). Therefore, it was important to investigate local-to-remote cortical connectivity in wellbeing for a complete picture of brain mechanisms of wellbeing.
In this study, we employed a novel seed-based method, which mainly included the computation of 2dReHo (Zuo et al., 2013) and seed-based functional connectivity (SFC), to examine local-to-remote cortical connectivity (Jiang et al., 2015) in individual wellbeing. We aimed to investigate whether wellbeing correlated with the brain spontaneous activity as well as the relations between wellbeing and local-to-remote functional connectivity. Studies on neural mechanisms of wellbeing not only enriched our comprehension of wellbeing and how to live a happy life but studies on the local-to-remote cortical connectivity might also shed new insights on wellbeing studies from perspectives of both neuroimaging and behavior.
Materials and Methods
Participants
A total of 67 healthy subjects (32 men, age 18.6–64.3 years) were recruited from the local community or universities by advertisements. All the participants underwent a detailed mental health interview by two trained psychologists using the Mini-International Neuro-Psychiatric Interview. People with a history of major neuropsychiatric illness, head injury, alcohol, and drug abuse were excluded. They were also assessed with the Wechsler Adult Intelligence Scale-4th Edition (in Chinese, WAIS-IV), the Schutte Self-Report Emotional Intelligence scale in Chinese Version (SSEIS), State-Trait Anxiety Inventory, Mental Health Continuum-Short Form (MHC-SF), Emotion Regulation Questionnaire, Chinese Perceived Stress Scale, the Achievement Motivation Scale, and the Self-Control Scale. The Institutional Review Board of the Institute of Psychology, Chinese Academy of Sciences approved this study, and written informed consent was obtained from individual participants prior to data acquisition.
Behavior Measurement
We employed a 14-item version of MHC-SF to measure the wellbeing of the participants, which was composed of emotional wellbeing, psychological wellbeing, and social wellbeing (Keyes, 2007). Specifically, emotional wellbeing reflects the hedonic aspect of wellbeing that encompasses positive affective states and high levels of life satisfaction (Diener et al., 2003). Psychological and social wellbeing are collectively known as eudaimonic wellbeing, which refers to the actualization of individuals’ potential or true value as well as the evaluation of one’s circumstance and functioning in the society (Ryff and Keyes, 1995; Keyes, 1998). There are three items in emotional wellbeing (1–3), which are divided into 2 dimensions, namely, positive affect and avowed quality of life. There are five items in social wellbeing (4–8), which are divided into 5 dimensions, namely, social acceptance, social actualization, social contribution, social coherence, and social integration. There are six items in psychological wellbeing (9–14), which are divided into 6 dimensions, namely, self-acceptance, personal growth, purpose in life, environmental mastery, autonomy, and positive relations with others. Participants were asked to evaluate the number of times they felt the problems mentioned in the past 1 month and to respond with “1” representing “never” and “6” representing “every day.” The scores of each subscale for each participant were calculated by summing the scores of the items. The total scores are positively associated with the level of wellbeing. The Chinese version has high validity and reliability and could effectively measure individual wellbeing (Yin and He, 2012).
Magnetic Resonance Imaging
Magnetic resonance imaging images were collected using the 3.0 T GE scanner Discovery MR750 at the Institute of Psychology, Chinese Academy of Sciences. All the participants completed a T1-weighted structural MRI scan (eyes closed) with a 3D-FSPGR sequence [repetition time (TR) = 6.652 ms; echo time (TE) = 2.928 ms; flip angle (FA) = 12°; matrix = 256 × 256; slice thickness = 1 mm; field of view = 224 mm × 224 mm] and an 8-min resting-state fMRI scan (eyes open with a fixation cross) using a gradient echo EPI sequence [TR = 2,000 ms; TE = 30 ms; FA = 90°; number of slices = 33 (interleaved); slice thickness = 3.5 mm; gap = 0.7 mm; and matrix = 64 × 64].
Data Preprocessing
Magnetic resonance imaging images were preprocessed using the Connectome Computation System (CCS)1 developed by our laboratory (Xu et al., 2015), which integrated three common neuroimaging toolboxes including AFNI (Cox, 2012), FSL (Jenkinson et al., 2012), and FreeSurfer (Fischl, 2012), as well as in-house MATLAB scripts. The CCS pipelines were employed to preprocess all individual images, including both structural and functional images, as well as quality control (Zuo et al., 2013; Xu et al., 2015). The structural processing pipelines mainly included (1) intensity inhomogeneity correction, (2) brain extraction, (3) tissue segmentation, (4) white and pial surface generation, and (5) deformation estimation between the resulting spherical mesh and a common spherical coordinate system. The functional processing pipelines were consistent with our previous publications (Jiang et al., 2015; Zhang et al., 2017) and were mainly included (1) excluding the first 5 volumes from each scan; (2) removing and interpolating time series spikes; (3) correcting slice timing and head motion; (4) extracting functional brain; (5) normalizing 4D global mean of image intensity; and (6) co-registration between functional and anatomical images by employing a boundary-based registration (BBR) algorithm.
Quality Control Procedure
Following the preprocessed individual MRI images, the CCS also provided a quality control procedure (QCP) for both functional and structural images. The QCP includes the following steps (Zuo et al., 2013): (1) brain extraction or skull stripping, (2) brain tissue segmentation, (3) pial and white surface reconstruction, (4) BBR-based functional image registration, and (5) head motion during r-FMRI. The pipeline also computed the mean frame-wise displacement (meanFD; Power et al., 2012) and the minimal cost of the BBR co-registration (mcBBR) for the subsequent statistical tests as covariates. All participants with bad brain extraction, tissue segmentation, and bad surface construction will be excluded from the subsequent analysis. One participant did not complete MRI scanning, and one participant did not pass the mental health interview. Five participants were excluded because their mcBBR was greater than 0.65. Therefore, we had 60 participants (31 males and 29 females, aged from 19.5 to 64.3 years with an average of 37.8 years, educated from 8 to 22 years with an average of 15.5 years) for final group analysis. The detailed participant information is shown in Table 1.
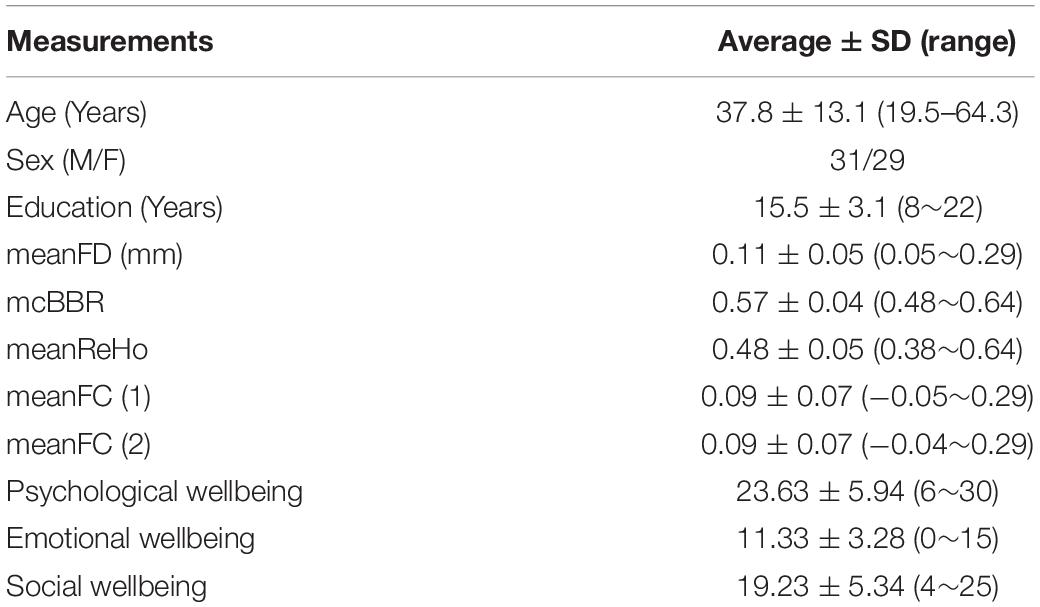
Table 1. Participant information of all the 60 subjects including basic demographics, brain characteristics, and wellbeing scores.
Local Short-Range Functional Connectivity: 2dReHo
To examine the local short-range functional homogeneity, we used surface-based functional homogeneity, namely, 2dReHo, which has been documented in the previous study (Zuo and Xing, 2014). Specifically, for a given vertex in the grid (fsaverage5), we identified its nearest neighbors and computed Kendall’s coefficient of concordance of the rfMRI time series of the nearest neighbors, including the vertex itself, as the local short-range connectivity of this vertex. Every vertex on the cortical surface of both hemispheres was computed to produce 2dReHo maps to characterize the local functional connectivity. In addition, all individual 2dReHo maps were further smoothed with a Gaussian kernel with 6-mm full width at half maximum (FWHM) using the fsaverage5. 2dReHo has been demonstrated to have high robustness against temporal and spatial noise and outliers (Zuo et al., 2013).
We employed a general linear model with the covariates of age, sex, education, meanFD, mcBBR, and global mean 2dReHo to evaluate the associations of 2dReHo with wellbeing. Then, we used the false discovery rate (FDR) method to correct the vertex-wise significance values for each contrast of group analysis. Clusters of smaller than 5 vertices were also excluded. The detailed statistical model is shown in the following equation:
Remote Long-Range Functional Connectivity: Seed-Based Correlation
Because of the limitations of the only characterization of the local functional connectivity of 2dReHo, to compensate for the lack of this metric, we further developed a local-to-remote cortical connectivity method integrating 2dReHo and the SFC method (Jiang et al., 2015). Each cluster showing effects on 2dReHo was used as a seed region for subsequent functional connectivity analysis. On the basis of individual preprocessed rfMRI time series that were spatially smoothed with 6 mm FWHM, the mean time sequence was computed across all vertices in the cluster, and then we calculated its correlations with the time series of all the other vertices using the fsaverage5. All individual correlation maps were further spatially smoothed with 6 mm FWHM. Similar to 2dReHo, we also used a general linear model with the covariates of sex, age, education, meanFD, mcBBR, and global mean SFC to evaluate the associations of wellbeing with remote cortical connectivity. The same FDR method was used to correct the vertex-wise significance values for each contrast of group analysis. Clusters of smaller than 5 vertices were also excluded.
Results
Well-Being Was Associated With Local Cortical Connectivity 2dReHo
Consistent with our previous studies (Jiang et al., 2014), the posteromedial cortex and the parietal cortex exhibited high local functional connectivity ReHo (Figure 1A). In addition, in Figures 1B,C, we observed that local functional connectivity (2dReHo) in the right orbitofrontal sulcus positively correlated with psychological wellbeing but negatively correlated with social wellbeing. No other significant correlations were observed. For a more intuitive illustration of the associations of wellbeing with local functional connectivity, we also depicted scatters of the residues of both wellbeing and 2dReHo in Figure 1. One note was that the vertices with significant correlations of psychological wellbeing were not the same as the vertices with significant correlations of social wellbeing, although they were both within the right orbitofrontal sulcus. Also, we only reported the clusters of more than 5 vertices, and the detailed information of the brain regions with significant correlations between cortical connectivity and wellbeing is illustrated in Table 2.
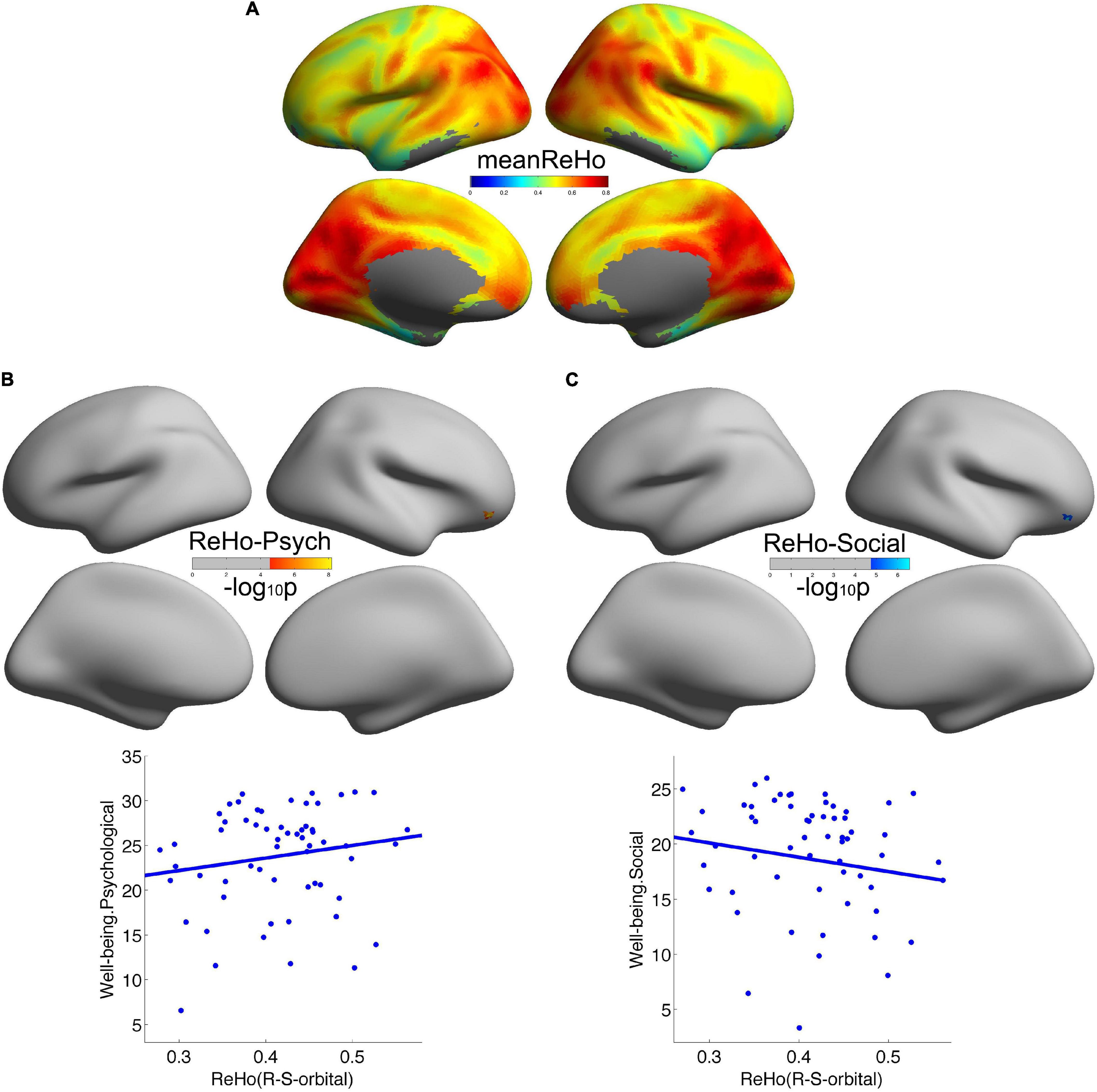
Figure 1. Local cortical connectivity in healthy participants and its associations with wellbeing. (A) Showed the average local cortical connectivity 2dReHo across all the participants. 2dReHo in the right orbitofrontal sulcus positively correlated with psychological wellbeing (B) but negatively correlated with social wellbeing (C).
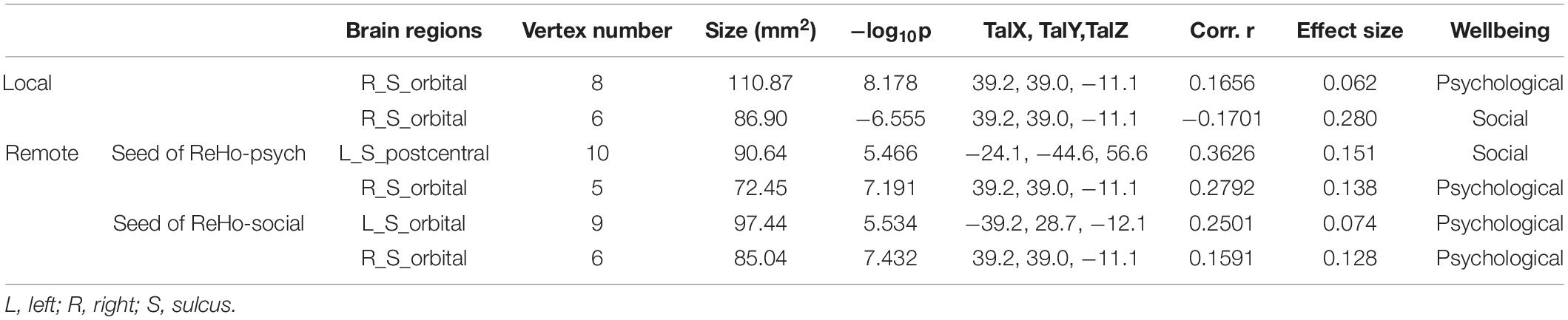
Table 2. Brain regions with significant associations of wellbeing with local-to-remote cortical connectivity.
Well-Being Was Associated With Remote Cortical Connectivity
We employed the right orbitofrontal sulcus showing the effect on psychological wellbeing as seed region, and the seed-based functional connectivity analysis revealed that a significant increment of short-range connectivity with psychological wellbeing was spatially extended to long-range connectivity within the right OFC: within the right OFC, both local and remote cortical connectivity increased with psychological wellbeing. We also observed that the remote cortical connectivity between the right orbitofrontal sulcus and the left postcentral sulcus positively predicted social wellbeing (Figure 2). Additionally, we employed the cluster showing effect on social wellbeing as seed region, and the local-to-remote cortical connectivity results demonstrated that the remote cortical connectivity within the right orbitofrontal sulcus and the remote connectivity of contralateral orbitofrontal sulcus were both positively correlated with psychological wellbeing (Figure 3). We only reported the clusters of more than 5 vertices, and the detailed information of the brain regions with significant correlations between cortical connectivity and wellbeing is illustrated in Table 2.
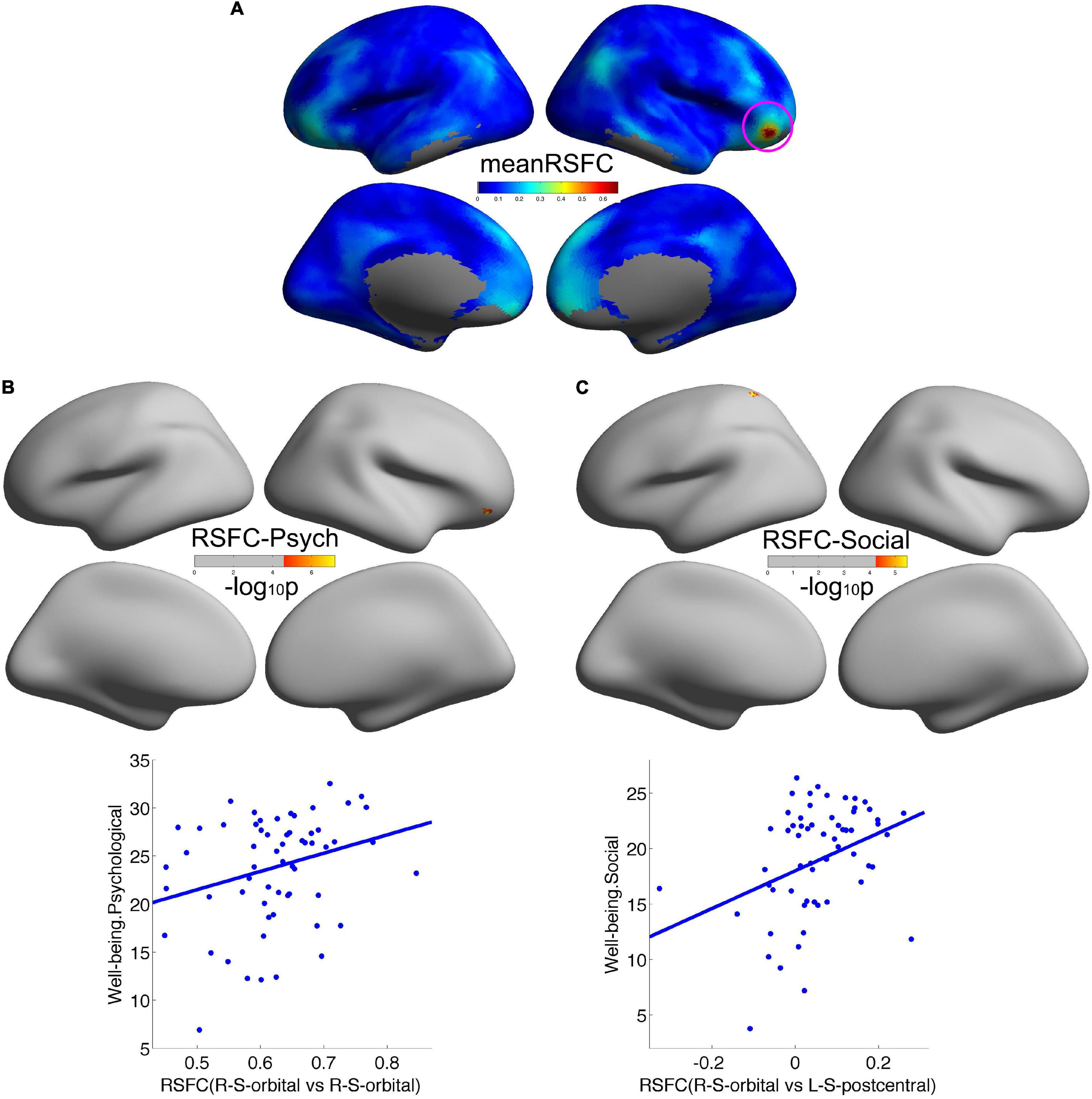
Figure 2. Remote cortical connectivity in healthy participants and its associations with wellbeing. (A) Illustrated the average resting-state functional connectivity (RSFC) across all the participants taking the psychological wellbeing-related right orbitofrontal sulcus as the seed region. Remote cortical connectivity within the right orbitofrontal cortex was positively correlated with psychological wellbeing (B), as well as remote cortical connectivity between the right orbitofrontal sulcus and the left postcentral sulcus was positively correlated with social wellbeing (C).
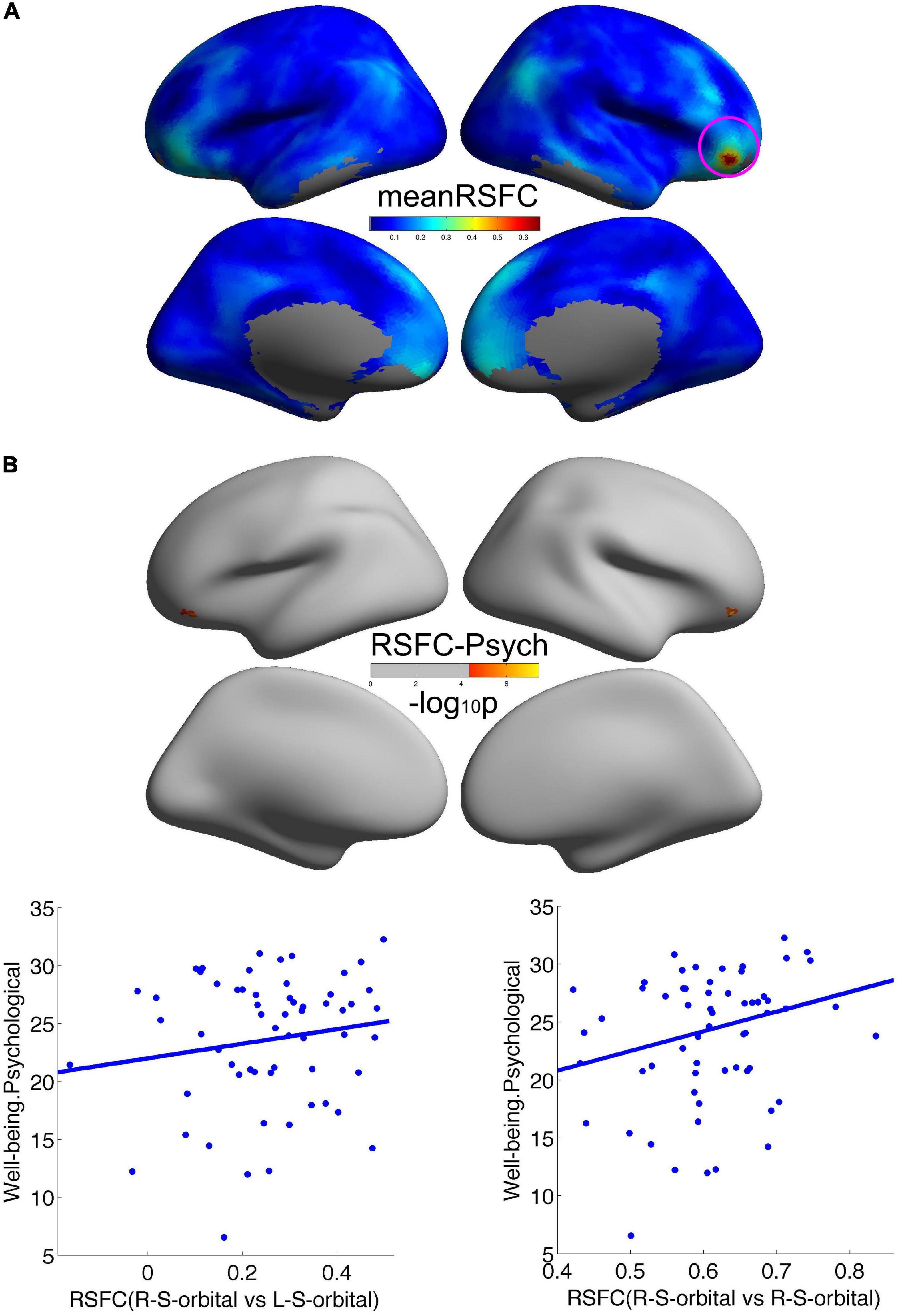
Figure 3. Remote cortical connectivity and its associations with wellbeing. (A) Illustrated the average RSFC across all the participants taking the social wellbeing-related right orbitofrontal sulcus as the seed region. The remote cortical connectivity within the right orbitofrontal sulcus and the remote connectivity of contralateral orbitofrontal sulcus were both positively correlated with psychological wellbeing (B).
Discussion
In this study, we used local-to-remote cortical connectivity to explore whether wellbeing was associated with local-to-remote cortical connectivity. Our analysis revealed that local cortical connectivity (2dReHo) in the right orbitofrontal sulcus not only positively predicted individual differences in psychological wellbeing but also negatively predicted differences in social wellbeing. More importantly, we demonstrated that the local cortical connectivity indeed expanded to remote cortical connectivity: the remote connectivity within the right orbitofrontal sulcus and the remote connectivity of the contralateral orbitofrontal sulcus positively predicted psychological wellbeing, as well as the remote connectivity between the right orbitofrontal sulcus and the left precentral sulcus positively predicted social wellbeing. Taking the significance of anatomical distance in human brain functional architecture into consideration, this study may shed new light on the neural mechanisms underlying wellbeing.
For local cortical connectivity, we discovered that 2dReHo in the right orbitofrontal sulcus was positively correlated with psychological wellbeing but negatively correlated with social wellbeing, suggesting that this region probably contributed to wellbeing-related experience in the brain. On the one hand, the OFC locates the ventral region of the prefrontal cortex, and neuroimaging studies have demonstrated that it played an important role in the regulation and processing of emotion (Bechara et al., 2000; Ochsner et al., 2002, 2004; Kringelbach and Rolls, 2004), encoding rewards of hedonic or painful experience (O’Doherty et al., 2001; Kringelbach, 2005), as well as dispositional mindfulness (Kong et al., 2015b), which were crucial to the integration of appropriate information, including emotions and behaviors to promote individual’s psychological and physical health. Meanwhile, compared with healthy participants, decreased 2dReHo was found in patients with various diseases such as depression (Yao et al., 2009) and migraine (Yu et al., 2012) in the right OFC, which also demonstrated the significance of spontaneous activities of the OFC in individual psychological health. Therefore, healthy participants with higher 2dReHo in the orbitofrontal sulcus have a higher level of psychological wellbeing. On the other hand, the negative relationship between social wellbeing and local cortical connectivity 2dReHo was consistent with prior studies. For example, by using the voxel-based morphometry (VBM) method, researchers found that the regional gray matter volume (rGMV) in the OFC was negatively associated with social wellbeing (Kong et al., 2019). These results consistently verified that the OFC might be a complex site for social wellbeing. Furthermore, the OFC has also been proved to play an important role in social cognition and interaction (Adolphs, 2001; Amodio and Frith, 2006), social cooperation (Rilling et al., 2002), theory of mind and empathy (Sabbagh, 2004; Vollm et al., 2006), and social decision-making such as trust, reciprocity, altruism, and social norm conformity (Sanfey, 2007; Rilling et al., 2008; Rilling and Sanfey, 2011). All of these social-cognitive processes are prerequisites for an individual’s social functioning, and the negative correlation between 2dReHo and social wellbeing in the orbitofrontal sulcus might imply a reorganization mechanism for neuroplasticity and cortical remapping or disruption of inhibitory mechanisms (Xu et al., 2014; Zhou et al., 2014). The negative correlation between the OFC and social wellbeing may also be due to synaptic pruning of redundant neurons during development, which contributed to more efficient information processing in the human brain (Kanai and Rees, 2011).
Based on the above local cortical connectivity associations with wellbeing, our remote cortical connectivity findings confirmed that the local short-range functional connectivity indeed diffused remote long-range functional connectivity in individual wellbeing. Previous studies on the cortical functional connectivity have revealed that anatomical distance played an important role in designing the functional architecture of the human brain (Ercsey-Ravasz et al., 2013; Samu et al., 2014). Also, local short connections and remote long connections were, respectively, related to within-module and intermodular connections in different cortical hierarchies (Alexander-Bloch et al., 2013; Sporns, 2013). Local-to-remote cortical connectivity has also been demonstrated to be correlated with various diseases such as schizophrenia (Sigurdsson et al., 2010; Jiang et al., 2015), chronic insomnia disorder (Zhou et al., 2020), renal disease (Chen et al., 2020), Parkinson’s disease (Harrington et al., 2020), and Alzheimer’s disease (Dai et al., 2015). Our findings demonstrated, for the first time, that wellbeing was associated with the local-to-remote cortical connectivity of the human brain. We found that the remote long-range connectivity between the right OFC and the left postcentral sulcus was positively correlated with social wellbeing. The postcentral sulcus locates the primary somatosensory cortex, which played an important role in touch, temperature, pain, and proprioception (Kreisman and Zimmerman, 1973; Ploner et al., 2000). Therefore, the cultivation of social wellbeing might need the integration and processing of sensory information and social cognition that led to the remote functional connectivity of these two brain regions. The local-to-remote method to investigate the short- and long-range functional connectivity of wellbeing, which has not been employed in previous wellbeing-related studies, could extend and enrich our knowledge of the neural mechanisms of wellbeing and provide novel insights in future neuroimaging studies.
Among the multiple remote long-range functional connectivity associations, the most remarkable one was that interhemispheric functional connectivity between the contralateral orbitofrontal sulcus positively correlated with psychological wellbeing. This kind of connectivity between contralateral brain regions, called functional homotopy, has been noticed and deeply investigated in previous studies: as a fundamental characteristic of the intrinsic human brain architecture (Stark et al., 2008), functional homotopy reflects high degree of synchrony in spontaneous activity between geometrically corresponding (i.e., homotopic) regions in each hemisphere (Zuo et al., 2010). Also, impairments of interhemispheric homotopic connectivity have been reported in various neuropsychiatric disorders such as obsessive-compulsive disorder (OCD; Jia et al., 2020), cervical dystonia (Jiang et al., 2019), depressive disorder (Guo et al., 2018), somatization disorder (Su et al., 2016), and autism spectrum disorder (Li et al., 2019). All these validated that the homotopic connectivity might be one of the basic organizational principles of neural underpinning. Interestingly, in this study, we found remote functional connectivity of the contralateral orbitofrontal sulcus with psychological wellbeing, which was consistent with other studies that also demonstrated significant homotopic connectivity changes in the OFC (Jiang et al., 2020). In summary, the strong connection between homotopic brain regions within the OFC in healthy participants might contribute to the integration of emotional and cognitive information to promote individual wellbeing.
Using the local-to-remote cortical connectivity method, we concluded that wellbeing was associated with local-to-remote cortical connectivity, especially when we identified the OFC as a key region for wellbeing characterization. However, our study still had several limitations: first, we focused only on the cortical connectivity of the human brain, and in future, we might take subcortical structures into account to investigate whole-brain connectivity. Second, our sample size was not large, and we need to recruit more participants to get a more convincing result and a better statistical power. Finally, the current wellbeing measurement depends on participants’ self-report. Subjective wellbeing, which belongs to a category of psychology, is not a good guideline for understanding how the human brain works or how subjective wellbeing works, and it is necessary to employ more objective cognitive or emotional tests to get a deeper understanding of wellbeing itself as well as the associations of wellbeing with the human brain.
Conclusion
In this study, we used the local-to-remote cortical connectivity method to explore whether wellbeing was associated with local to remote cortical connectivity. Our results showed that the local connectivity in the right orbitofrontal sulcus was significantly positively correlated with psychological wellbeing but negatively correlated with social wellbeing; the remote connectivity within the right OFC and interhemispheric connectivity of the orbitofrontal sulcus were both positively associated with psychological wellbeing; the remote functional connectivity between the right orbitofrontal sulcus and the left postcentral sulcus was positively associated with social wellbeing. Our results showed that wellbeing was indeed associated with local-to-remote cortical connectivity, and our findings supplied a new perspective of distance-related neural mechanisms of wellbeing.
Data Availability Statement
The raw data supporting the conclusions of this article will be made available upon request from the corresponding author.
Ethics Statement
The studies involving human participants were reviewed and approved by the Institutional Review Board of Institute of Psychology, Chinese Academy of Sciences. The patients/participants provided their written informed consent to participate in this study.
Author Contributions
LJ: conceptualization and methodology. LJ, YL, and CL: formal analysis, investigation, and writing–review and editing. All authors contributed to the article and approved the submitted version.
Funding
This work was supported by the National Natural Science Foundation of China (11674388).
Conflict of Interest
The authors declare that the research was conducted in the absence of any commercial or financial relationships that could be construed as a potential conflict of interest.
Publisher’s Note
All claims expressed in this article are solely those of the authors and do not necessarily represent those of their affiliated organizations, or those of the publisher, the editors and the reviewers. Any product that may be evaluated in this article, or claim that may be made by its manufacturer, is not guaranteed or endorsed by the publisher.
Supplementary Material
The Supplementary Material for this article can be found online at: https://www.frontiersin.org/articles/10.3389/fnbeh.2022.737121/full#supplementary-material
Footnotes
References
Adolphs, R. (2001). The neurobiology of social cognition. Curr. Opin. Neurobiol. 11, 231–239. doi: 10.1016/s0959-4388(00)00202-6
Alexander-Bloch, A. F., Vertes, P. E., Stidd, R., Lalonde, F., Clasen, L., Rapoport, J., et al. (2013). The anatomical distance of functional connections predicts brain network topology in health and schizophrenia. Cereb. Cortex 23, 127–138. doi: 10.1093/cercor/bhr388
Amodio, D. M., and Frith, C. D. (2006). Meeting of minds: the medial frontal cortex and social cognition [Review]. Nat. Rev. Neurosci. 7, 268–277. doi: 10.1038/nrn1884
Bechara, A., Damasio, H., and Damasio, A. R. (2000). Emotion, decision making and the orbitofrontal cortex [Article]. Cereb. Cortex 10, 295–307. doi: 10.1093/cercor/10.3.295
Castellanos, F. X., Margulies, D. S., Kelly, C., Uddin, L. Q., Ghaffari, M., Kirsch, A., et al. (2008). Cingulate-precuneus interactions: A new locus of dysfunction in adult attention-deficit/hyperactivity disorder [Article]. Biol. Psychiat. 63, 332–337. doi: 10.1016/j.biopsych.2007.06.025
Chen, P. N., Hu, R. Y., Gao, L., Wu, B. L., Peng, M., Jiang, Q. Q., et al. (2020). Abnormal degree centrality in end-stage renal disease (ESRD) patients with cognitive impairment: a resting-state functional MRI study [Article; Early Access]. Brain Imaging Behav. 11:3. doi: 10.1007/s11682-020-00317-3
Cox, R. W. (2012). AFNI: What a long strange trip it’s been. Neuroimage 62, 743–747. doi: 10.1016/j.neuroimage.2011.08.056
Dai, Z. J., Yan, C. G., Li, K. C., Wang, Z. Q., Wang, J. H., Cao, M., et al. (2015). Identifying and Mapping Connectivity Patterns of Brain Network Hubs in Alzheimer’s Disease [Article]. Cereb. Cortex 25, 3723–3742. doi: 10.1093/cercor/bhu246
Diener, E. (1994). ASSESSING SUBJECTIVE WELL-BEING - PROGRESS AND OPPORTUNITIES [Review]. Soc. Indicat. Res. 31, 103–157. doi: 10.1007/bf01207052
Diener, E., Oishi, S., and Lucas, R. E. (2003). Personality, culture, and subjective well-being: Emotional and cognitive evaluations of life [Review]. Annu. Rev. Psychol. 54, 403–425. doi: 10.1146/annurev.psych.54.101601.145056
Ercsey-Ravasz, M., Markov, N. T., Lamy, C., Van Essen, D. C., Knoblauch, K., Toroczkai, Z., et al. (2013). A Predictive Network Model of Cerebral Cortical Connectivity Based on a Distance Rule. Neuron 80, 184–197. doi: 10.1016/j.neuron.2013.07.036
Fischl, B. (2012). FreeSurfer [Review]. Neuroimage 62, 774–781. doi: 10.1016/j.neuroimage.2012.01.021
Guo, W. B., Cui, X. L., Liu, F., Chen, J. D., Xie, G. R., Wu, R. R., et al. (2018). Decreased interhemispheric coordination in the posterior default-mode network and visual regions as trait alterations in first-episode, drug-naive major depressive disorder [Article]. Brain Imaging Behav. 12, 1251–1258. doi: 10.1007/s11682-017-9794-8
Guo, W. B., Liu, F., Xiao, C. Q., Liu, J. R., Yu, M. Y., Zhang, Z. K., et al. (2015). Increased short-range and long-range functional connectivity in first-episode, medication-naive schizophrenia at rest [Article]. Schizophrenia Res. 166, 144–150. doi: 10.1016/j.schres.2015.04.034
Harrington, D. L., Shen, Q., Filoteo, J. V., Litvan, I., Huang, M. X., Castillo, G. N., et al. (2020). Abnormal distraction and load-specific connectivity during working memory in cognitively normal Parkinson’s disease [Article]. Hum. Brain Mapp. 41, 1195–1211. doi: 10.1002/hbm.24868
Heller, A. S., van Reekum, C. M., Schaefer, S. M., Lapate, R. C., Radler, B. T., Ryff, C. D., et al. (2013). Sustained striatal activity predicts eudaimonic well-being and cortisol output. Psychol. Sci. 24, 2191–2200. doi: 10.1177/0956797613490744
Jenkinson, M., Beckmann, C. F., Behrens, T. E., Woolrich, M. W., and Smith, S. M. (2012). FSL [Review]. Neuroimage 62, 782–790. doi: 10.1016/j.neuroimage.2011.09.015
Jia, C. C., Ou, Y. P., Chen, Y. H., Li, P., Lv, D., Yang, R., et al. (2020). Decreased Resting-State Interhemispheric Functional Connectivity in Medication-Free Obsessive-Compulsive Disorder [Article]. Front. Psychiat. 11:559729. doi: 10.3389/fpsyt.2020.559729
Jiang, L., Ma, X. J., Li, S. G., Luo, H. J., Zhang, G. M., Wang, Y. N., et al. (2020). Frequency-Dependent Changes in Interhemispheric Functional Connectivity Measured by Resting-State fMRI in Children With Idiopathic Generalized Epilepsy [Article]. Front. Neurol. 11:645. doi: 10.3389/fneur.2020.00645
Jiang, L., Xu, T., He, Y., Hou, X.-H., Wang, J., Cao, X.-Y., et al. (2014). Toward neurobiological characterization of functional homogeneity in the human cortex: regional variation, morphological association and functional covariance network organization. Brain Struct. Funct. 220, 2485–2507. doi: 10.1007/s00429-014-0795-8
Jiang, L., Xu, Y., Zhu, X. T., Yang, Z., Li, H. J., and Zuo, X. N. (2015). Local-to-remote cortical connectivity in early- and adulthood-onset schizophrenia. Transl. Psychiatry 5:e566. doi: 10.1038/tp.2015.59
Jiang, W. Y., Lei, Y. W., Wei, J., Yang, L., Wei, S. B., Yin, Q., et al. (2019). Alterations of Interhemispheric Functional Connectivity and Degree Centrality in Cervical Dystonia: A Resting-State fMRI Study [Article]. Neural Plastic. 11:7349894. doi: 10.1155/2019/7349894
Kanai, R., and Rees, G. (2011). The structural basis of inter-individual differences in human behaviour and cognition. Nat. Rev. Neurosci. 12, 231–242. doi: 10.1038/nrn3000
Keyes, C. (2009). Brief description of the mental health continuum short form (MHC-SF). Available online at: https://www.aacu.org/sites/default/files/MHC-SFEnglish.pdf
Keyes, C. L. M. (2007). Promoting and protecting mental health as flourishing - A complementary. strategy for improving national mental health. Am. Psychol. 62, 95–108. doi: 10.1037/0003-066x.62.2.95
Kong, F., Hu, S., Xue, S., Song, Y., and Liu, J. (2015a). Extraversion mediates the relationship between structural variations in the dorsolateral prefrontal cortex and social well-being. Neuroimage 105, 269–275. doi: 10.1016/j.neuroimage.2014.10.062
Kong, F., Hu, S., Wang, X., Song, Y., and Liu, J. (2015b). Neural correlates of the happy life: the amplitude of spontaneous low frequency fluctuations predicts subjective well-being. Neuroimage 107, 136–145. doi: 10.1016/j.neuroimage.2014.11.033
Kong, F., Liu, L., Wang, X., Hu, S., Song, Y., and Liu, J. (2015c). Different neural pathways linking personality traits and eudaimonic well-being: a resting-state functional magnetic resonance imaging study. Cognit. Affect. Behav. Neurosci. 15, 299–309. doi: 10.3758/s13415-014-0328-1
Kong, F., Xue, S., and Wang, X. (2016a). Amplitude of low frequency fluctuations during resting state predicts social well-being. Biol. Psychol. 118, 161–168. doi: 10.1016/j.biopsycho.2016.05.012
Kong, F., Wang, X., Song, Y. Y., and Liu, J. (2016b). Brain regions involved in dispositional mindfulness during resting state and their relation with well-being [Article]. Soc. Neurosci. 11, 331–343. doi: 10.1080/17470919.2015.1092469
Kong, F., Yang, K., Sajjad, S., Yan, W., Li, X., and Zhao, J. (2019). Neural correlates of social well-being: gray matter density in the orbitofrontal cortex predicts social well-being in emerging adulthood. Soc. Cogn. Affect. Neurosci. 14, 319–327. doi: 10.1093/scan/nsz008
Kreisman, N. R., and Zimmerman, I. D. (1973). REPRESENTATION OF INFORMATION ABOUT SKIN TEMPERATURE IN DISCHARGE OF SINGLE CORTICAL NEURONS. Brain Res. 55, 343–353. doi: 10.1016/0006-8993(73)90300-4
Kringelbach, M. L. (2005). The human orbitofrontal cortex: Linking reward to hedonic experience [Review]. Nat. Rev. Neurosci. 6, 691–702. doi: 10.1038/nrn1747
Kringelbach, M. L., and Rolls, E. T. (2004). The functional neuroanatomy of the human orbitofrontal cortex: evidence from neuroimaging and neuropsychology [Review]. Prog. Neurobiol. 72, 341–372. doi: 10.1016/j.pneurobio.2004.03.006
Lewis, G. J., Kanai, R., Rees, G., and Bates, T. C. (2014). Neural correlates of the ‘good life’: eudaimonic well-being is associated with insular cortex volume. Soc. Cogn. Affect. Neurosci. 9, 615–618. doi: 10.1093/scan/nst032
Li, Q., Becker, B., Jiang, X., Zhao, Z. Y., Zhang, Q., Yao, S. X., et al. (2019). Decreased interhemispheric functional connectivity rather than corpus callosum volume as a potential biomarker for autism spectrum disorder [Article]. Cortex 119, 258–266. doi: 10.1016/j.cortex.2019.05.003
Li, R., Zhu, X. Y., Zheng, Z. W., Wang, P. Y., and Li, J. (2020). Subjective well-being is associated with the functional connectivity network of the dorsal anterior insula [Article]. Neuropsychologia 141:107393. doi: 10.1016/j.neuropsychologia.2020.107393
Luo, Y., Qi, S., Chen, X., You, X., Huang, X., and Yang, Z. (2017). Pleasure attainment or self-realization: the balance between two forms of well-beings are encoded in default mode network. Soc. Cogn. Affect. Neurosci. 12, 1678–1686. doi: 10.1093/scan/nsx078
Mueller, S., Wang, D. H., Fox, M. D., Yeo, B. T. T., Sepulcre, J., Sabuncu, M. R., et al. (2013). Individual Variability in Functional Connectivity Architecture of the Human Brain [Article]. Neuron 77, 586–595. doi: 10.1016/j.neuron.2012.12.028
Ochsner, K. N., Bunge, S. A., Gross, J. J., and Gabrieli, J. D. E. (2002). Rethinking feelings: An fMRI study of the cognitive regulation of emotion [Article]. J. Cognit. Neurosci. 14, 1215–1229. doi: 10.1162/089892902760807212
Ochsner, K. N., Ray, R. D., Cooper, J. C., Robertson, E. R., Chopra, S., Gabrieli, J. D. E., et al. (2004). For better or for worse: neural systems supporting the cognitive down- and up-regulation of negative emotion [Article]. Neuroimage 23, 483–499. doi: 10.1016/j.neuroimage.2004.06.030
O’Doherty, J., Kringelbach, M. L., Rolls, E. T., Hornak, J., and Andrews, C. (2001). Abstract reward and punishment representations in the human orbitofrontal cortex [Article]. Nat. Neurosci. 4, 95–102.
Ploner, M., Schmitz, F., Freund, H. J., and Schnitzler, A. (2000). Differential organization of touch and pain in human primary somatosensory cortex. J. Neurophysiol. 83, 1770–1776. doi: 10.1152/jn.2000.83.3.1770
Power, J. D., Barnes, K. A., Snyder, A. Z., Schlaggar, B. L., and Petersen, S. E. (2012). Spurious but systematic correlations in functional connectivity MRI networks arise from subject motion [Article]. Neuroimage 59, 2142–2154. doi: 10.1016/j.neuroimage.2011.10.018
Ren, Z., Shi, L., Wei, D., and Qiu, J. (2019). Brain Functional Basis of Subjective Well-being During Negative Facial Emotion Processing Task-Based fMRI. Neuroscience 423, 177–191. doi: 10.1016/j.neuroscience.2019.10.017
Rilling, J. K., and Sanfey, A. G. (2011). “The Neuroscience of Social Decision-Making,” in Annual Review of Psychology, Vol. 62, eds S. T. Fiske, D. L. Schacter, and S. E. Taylor (Palo Alto, CA: Annual Reviews), 23–48. doi: 10.1146/annurev.psych.121208.13164
Rilling, J. K., Gutman, D. A., Zeh, T. R., Pagnoni, G., Berns, G. S., and Kilts, C. D. (2002). A neural basis for social cooperation [Article]. Neuron 35, 395–405. doi: 10.1016/s0896-6273(02)00755-9
Rilling, J. K., King-Casas, B., and Sanfey, A. G. (2008). The neurobiology of social decision-making. Curr. Opin. Neurobiol. 18, 159–165. doi: 10.1016/j.conb.2008.06.003
Ryan, R. M., and Deci, E. L. (2001). On happiness and human potentials: A review of research on hedonic and eudaimonic well-being [Review]. Annu. Rev. Psychol. 52, 141–166. doi: 10.1146/annurev.psych.52.1.141
Ryff, C. D., and Keyes, C. L. M. (1995). THE STRUCTURE OF PSYCHOLOGICAL WELL-BEING REVISITED. J. Pers. Soc. Psychol. 69, 719–727. doi: 10.1037/0022-3514.57.6.1069
Sabbagh, M. A. (2004). Understanding orbitofrontal contributions to theory-of-mind reasoning: Implications for autism. Brain Cognit. 55, 209–219. doi: 10.1016/j.bandc.2003.04.002
Samu, D., Seth, A. K., and Nowotny, T. (2014). Influence of Wiring Cost on the Large-Scale Architecture of Human Cortical Connectivity. PLoS Computat. Biol. 10:1003557. doi: 10.1371/journal.pcbi.1003557
Sanfey, A. G. (2007). Social decision-making: Insights from game theory and neuroscience [Review]. Science 318, 598–602. doi: 10.1126/science.1142996
Shi, L., Sun, J., Wu, X., Wei, D., Chen, Q., Yang, W., et al. (2018). Brain networks of happiness: dynamic functional connectivity among the default, cognitive and salience networks relates to subjective well-being. Soc. Cogn. Affect. Neurosci. 13, 851–862. doi: 10.1093/scan/nsy059
Sigurdsson, T., Stark, K. L., Karayiorgou, M., Gogos, J. A., and Gordon, J. A. (2010). Impaired hippocampal-prefrontal synchrony in a genetic mouse model of schizophrenia [Article]. Nature 464, 763–U139. doi: 10.1038/nature08855
Song, L., Meng, J., Liu, Q., Huo, T. B., Zhu, X. X., Li, Y. M., et al. (2019). Polygenic Score of Subjective Well-Being Is Associated with the Brain Morphology in Superior Temporal Gyrus and Insula [Article]. Neuroscience 414, 210–218. doi: 10.1016/j.neuroscience.2019.05.055
Sporns, O. (2013). Network attributes for segregation and integration in the human brain. Curr. Opin. Neurobiol. 23, 162–171. doi: 10.1016/j.conb.2012.11.015
Stark, D. E., Margulies, D. S., Shehzad, Z. E., Reiss, P., Kelly, A. M. C., Uddin, L. Q., et al. (2008). Regional Variation in Interhemispheric Coordination of Intrinsic Hemodynamic Fluctuations. J. Neurosci. 28, 13754–13764. doi: 10.1523/jneurosci.4544-08.2008
Su, Q. J., Yao, D. P., Jiang, M. L., Liu, F., Long, L. L., Dai, Y., et al. (2016). Decreased interhemispheric functional connectivity in insula and angular gyrusisupramarginal gyrus: Significant findings in first-episode, drug-naive somatization disorder [Article]. Psychiat. Res. Neuroimaging 248, 48–54. doi: 10.1016/j.pscychresns.2016.01.008
Supekar, K., Musen, M., and Menon, V. (2009). Development of Large-Scale Functional Brain Networks in Children [Article]. PLoS Biol. 7:e1000157. doi: 10.1371/journal.pbio.1000157
Van’t Ent, D., den Braber, A., Baselmans, B. M. L., Brouwer, R. M., Dolan, C. V., Hulshoff Pol, H. E., et al. (2017). Associations between subjective well-being and subcortical brain volumes. Sci. Rep. 7:6957. doi: 10.1038/s41598-017-07120-z
Vatansever, D., Karapanagiotidis, T., Margulies, D. S., Jefferies, E., and Smallwood, J. (2020). Distinct patterns of thought mediate the link between brain functional connectomes and well-being. Netw. Neurosci. 4, 637–657. doi: 10.1162/netn_a_00137
Vollm, B. A., Taylor, A. N. W., Richardson, P., Corcoran, R., Stirling, J., McKie, S., et al. (2006). Neuronal correlates of theory of mind and empathy: A functional magnetic resonance imaging study in a nonverbal task [Article]. Neuroimage 29, 90–98. doi: 10.1016/j.neuroimage.2005.07.022
Waterman, A. S. (1993). Two conceptions of happiness : contrasts of personal expressiveness (eudaimonia) and hedonic enjoyment. J. Pers. Soc. Psychol. 64, 678–691. doi: 10.1037//0022-3514.64.4.678
Xu, K., Liu, H., Li, H. H., Tang, Y. Q., Womer, F., Jiang, X. W., et al. (2014). Amplitude of low-frequency fluctuations in bipolar disorder: A resting state fMRI study [Article]. J. Affect. Disord. 152, 237–242. doi: 10.1016/j.jad.2013.09.017
Xu, T., Yang, Z., Jiang, L., Xing, X.-X., and Zuo, X.-N. (2015). A Connectome Computation System for discovery science of brain. Sci. Bull. 60, 86–95. doi: 10.1007/s11434-014-0698-3
Yao, Z., Wang, L., Lu, Q., Liu, H., and Teng, G. (2009). Regional homogeneity in depression and its relationship with separate depressive symptom clusters: A resting-state fMRI study. J. Affect. Disord. 115, 430–438. doi: 10.1016/j.jad.2008.10.013
Yin, K., and He, J. (2012). Reliability and validity of the Mental Health Continuum Short Form in adults []. Chin. Mental Health J. 26, 388–392.
Yu, D. H., Yuan, K., Zhao, L., Zhao, L. M., Dong, M. H., Liu, P., et al. (2012). Regional homogeneity abnormalities in patients with interictal migraine without aura: a resting-state study [Article]. NMR Biomed. 25, 806–812. doi: 10.1002/nbm.1796
Zhang, Y. W., Zhao, Z. L., Qi, Z., Hu, Y., Wang, Y. S., Sheng, C., et al. (2017). Local-to-remote cortical connectivity in amnestic mild cognitive impairment. Neurobiol. Aging 56, 138–149. doi: 10.1016/j.neurobiolaging.2017.04.016
Zhou, F. Q., Zhu, Y. Y., Zhu, Y. J., Huang, M. H., Jiang, J., He, L. C., et al. (2020). Altered long- and short-range functional connectivity density associated with poor sleep quality in patients with chronic insomnia disorder: A resting-state fMRI study [Article]. Brain Behav. 10:e01844. doi: 10.1002/brb3.1844
Zhou, Y. X., Lui, Y. W., Zuo, X. N., Milham, M. P., Reaume, J., Grossman, R. I., et al. (2014). Characterization of Thalamo-cortical Association Using Amplitude and Connectivity of Functional MRI in Mild Traumatic Brain Injury [Article]. J. Magnetic Reson. Imaging 39, 1558–1568. doi: 10.1002/jmri.24310
Zuo, X. N., and Xing, X. X. (2014). Test-retest reliabilities of resting-state FMRI measurements in human brain functional connectomics: a systems neuroscience perspective. Neurosci. Biobehav. Rev. 45, 100–118. doi: 10.1016/j.neubiorev.2014.05.009
Zuo, X. N., Kelly, C., Di Martino, A., Mennes, M., Margulies, D. S., Bangaru, S., et al. (2010). Growing Together and Growing Apart: Regional and Sex Differences in the Lifespan Developmental Trajectories of Functional Homotopy [Article]. J. Neurosci. 30, 15034–15043. doi: 10.1523/jneurosci.2612-10.2010
Keywords: MRI, well-being, ReHo, functional connectivity, local-to-remote cortical connectivity
Citation: Li Y, Li C and Jiang L (2022) Well-Being Is Associated With Local to Remote Cortical Connectivity. Front. Behav. Neurosci. 16:737121. doi: 10.3389/fnbeh.2022.737121
Received: 06 July 2021; Accepted: 07 February 2022;
Published: 11 March 2022.
Edited by:
Walter Adriani, Italian National Institute of Health (ISS), ItalyReviewed by:
Ivan V. Brak, State Scientific-Research Institute of Physiology & Basic Medicine, RussiaAkitoshi Ogawa, Juntendo University, Japan
Copyright © 2022 Li, Li and Jiang. This is an open-access article distributed under the terms of the Creative Commons Attribution License (CC BY). The use, distribution or reproduction in other forums is permitted, provided the original author(s) and the copyright owner(s) are credited and that the original publication in this journal is cited, in accordance with accepted academic practice. No use, distribution or reproduction is permitted which does not comply with these terms.
*Correspondence: Lili Jiang, amlhbmdsbEBwc3ljaC5hYy5jbg==