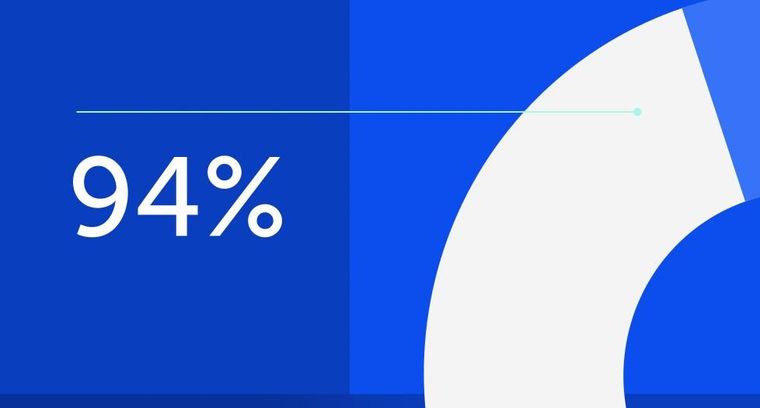
94% of researchers rate our articles as excellent or good
Learn more about the work of our research integrity team to safeguard the quality of each article we publish.
Find out more
REVIEW article
Front. Behav. Neurosci., 09 June 2021
Sec. Pathological Conditions
Volume 15 - 2021 | https://doi.org/10.3389/fnbeh.2021.698753
This article is part of the Research TopicOscillotherapeutics - Toward Real-Time Control of Pathological Oscillations in The BrainView all 11 articles
Emotional behavior and psychological disorders are expressed through coordinated interactions across multiple brain regions. Brain electrophysiological signals are composed of diverse neuronal oscillations, representing cell-level to region-level neuronal activity patterns, and serve as a biomarker of mental disorders. Here, we review recent observations from rodents demonstrating how neuronal oscillations in the hippocampus, amygdala, and prefrontal cortex are engaged in emotional behavior and altered by psychiatric changes such as anxiety and depression. In particular, we focus mainly on theta-range (4–12 Hz) oscillations, including several distinct oscillations in this frequency range. We then discuss therapeutic possibilities related to controlling such mental disease-related neuronal oscillations to ameliorate psychiatric symptoms and disorders in rodents and humans.
The accumulation of mental stress loads is a primary risk factor for psychiatric disorders such as major depressive disorder (MDD), anxiety disorders, and posttraumatic stress disorder (PTSD) (Yehuda and LeDoux, 2007; Arnsten, 2015). A number of studies have revealed that brain regions such as the medial prefrontal cortex (mPFC), cingulate cortex, amygdala (AMY), hippocampus (HPC), and hypothalamus play crucial roles in the regulation of affective and visceral functions and undergo marked changes in their activity caused by stress-induced mental disease (Greicius et al., 2007; Sheline et al., 2010; Nugent et al., 2015; Tovote et al., 2015; Drysdale et al., 2017). In particular, the HPC-PFC-AMY circuit is a core network formed by long-range projections (Caliskan and Stork, 2019) in which the ventral HPC (vHPC) and the mPFC transfer sensory and contextual information to the basolateral amygdala (BLA) (Orsini et al., 2011; Ciocchi et al., 2015) and the BLA, in turn, transfers information of negative valence back to the mPFC and vHPC (Ishikawa and Nakamura, 2003; Senn et al., 2014; Kim et al., 2016; Burgos-Robles et al., 2017).
To date, a key technique to understand the basic neuronal mechanisms and devise therapeutic strategies based on pathophysiology is the recording of electrophysiological signals that represent brain activity patterns and provide great temporal resolution at the millisecond scale. The mammalian forebrain generates extracellular field potentials containing a mixture of diverse neural oscillations at frequency bands ranging from 0.1 to 250 Hz (in health) and up to 500 Hz (in disease) that show dynamic changes associated with arousal levels, emotional valence, and memory performance (Buzsaki and Draguhn, 2004; Buzsaki et al., 2012; Buzsaki and Watson, 2012). In addition to representing the activity patterns of individual brain areas, electrical signals from multiple brain regions are sensitive to changes in their functional connectivity defined as their correlational power changes and coherence. A number of clinical studies have reported altered patterns of electroencephalogram (EEG) oscillatory signals in depressed patients, such as altered power and functional coupling in the alpha (8–13 Hz) and gamma (30–100 Hz) bands in the frontal cortex (Jesulola et al., 2015; Fitzgerald and Watson, 2018; Grunewald et al., 2018). Similarly, in PTSD patients, resting electrical signals in the PFC show decreased alpha power-mediated inhibition and increased gamma power, suggesting hypofunction in the PFC (Huang et al., 2014; Clancy et al., 2017). Accumulated evidence from these studies suggests that brain field potential signals serve as a physiological sign of mood disorders (Iosifescu, 2011; Baskaran et al., 2012; Buzsaki and Watson, 2012; Fitzgerald and Watson, 2018).
On the other hand, at microscopic levels, a number of studies from animal models to human patients have demonstrated stress-related molecular and cellular mechanisms that could lead to psychiatric disorders (Krishnan and Nestler, 2008; Arnsten, 2015; McEwen et al., 2015). However, it remains largely unknown how these mechanisms are integrated in the expression of psychiatric symptoms and behavioral phenotypes. The necessity to bridge the gap between these insights also highlights the importance of electrical field signals as a measure to estimate neuronal network-level dynamics. In particular, animal experiments allow us to directly measure local field potential (LFP) signals from target brain regions with high signal-to-noise ratios and compare how their oscillatory patterns dynamically change with emotional behavior in both health and disease. Such basic non-clinical experiments are crucial for devising novel therapeutic strategies, including drug discovery and timed interventions on brain activity, which have been termed oscillotherapeutics (Takeuchi and Berenyi, 2020).
This paper introduces recent techniques to measure brain LFP signals from freely moving rodents, summarizes recent reports showing anxiety- and fear-related changes in LFP patterns, especially focusing on the HPC-PFC-AMY circuit, observed in non-pathological animals, and then describes how LFP signals from these brain regions are affected by stress accumulation. Finally, we discuss potential therapeutic strategies to ameliorate stress-induced psychiatric disorders based on oscillatory LFP patterns.
A key experimental technique related to oscillotherapeutics in rodent research is chronic electrophysiological recordings of extracellular signals representing collective oscillatory field potentials from neuronal populations and spike patterns from individual neurons in freely moving animals (Figure 1A). A number of studies have utilized various types of recording electrodes, such as tetrode arrays and silicon probes (Figure 1B), that enable multisite (tens or hundreds of sites) recordings from target brain regions. These electrodes are chronically implantable for several months and are flexibly movable to adjust the depth of electrodes with micrometer precision in the brain tissue after implantation using microdrives. Recent advancements in 3D printer technology make it easy to customize plastic parts to accommodate these electrodes into a microdrive (Figure 1C). For example, we recently created a recording device to cover wide ranges of cortical regions from anterior to posterior and from medial and lateral parts in rodents (Figure 1B, left) (Konno et al., 2019; Nakayama et al., 2019). Conveniently, most of the CAD files designed by developers for multichannel recordings are now available from cloud-based repositories, such as Mendeley data, and laboratory websites, which enables researchers to freely create these devices. Furthermore, wireless recording systems are recently available (Zuo et al., 2012; Martinez et al., 2018; Iturra-Mena et al., 2019), which are especially useful for stress research because they reduce the physical stress of animals.
Figure 1. Recent recording methods to study brain oscillations in rodents. (A) A picture of a mouse implanted with an electrode assembly. (B) Typical electrode assemblies with multiple recording sites. (Left) Tetrode arrays to target several separated brain regions. The dotted region is magnified in the right panel, showing the electrode tips (indicated by arrows). (Middle) A microdrive with multiple tetrode arrays to record spike patterns of neurons in a target region. (Right) A microdrive with 8-shanks silicon probes to record spike patterns of neurons in a target region. (C) Typical CAD illustrations of plastic parts created by a 3D printer. (D) Simultaneous electrophysiological recordings of a brain LFP signal, a vagus nerve (VN) signal, an ECG signal, an EMG signal, and a Respiration (Resp) signal.
In addition to brain electrophysiological recordings, we conceived a recording approach in which a multichannel recording device extends to the collection of bioelectrical signals from peripheral organs, such as electrocardiogram (ECG) signals, electromyogram (EMG) signals (Okada et al., 2016; Okonogi et al., 2018; Shikano et al., 2018), olfactory bulb respiratory (Resp) signals (Kuga et al., 2019), and vagus nerve (VN) signals (Shikano et al., 2019) (Figure 1D), all of which can be captured by a single recording device. This recording method is useful for precisely monitoring signals representing changes in peripheral organ activity related to emotion, stress, and mental disorders, in addition to simple behavioral phenotypes.
Electrophysiological recordings, compared with imaging techniques, provide higher temporal resolution at millisecond timescales, allowing real-time detection of electrical signals and preciselytimed interventions involving neuronal activity immediately upon theemergence of target features in the signals (e.g., signal amplitude, phase, and spikes), a so-called closed-loop neurostimulation system (Figures 2B,C). On-demand stimulation protocols based on this system enable a high-quality physiological experimental design for both basic and pathological studies. As an example of targeting transient brain signals, time-specific stimulation during seizure events can inhibit subsequent seizure-like behavior in epilepsy animal models (Berenyi et al., 2012; Takeuchi et al., 2021). At frequencies lower than 10 Hz, phase-targeting stimulation (e.g., peaks and troughs of a given oscillation) is an effective technique to test their contributions to brain functions. For instance, theta (4–12 Hz) phase-specific manipulations of neuronal activity in the hippocampus and the subthalamic nucleus have been shown to induce memory enhancement and parkinsonian symptoms, respectively (Siegle and Wilson, 2014; Cordon et al., 2018). Additional details of applications to studies of emotion and psychiatric disease are described later.
Figure 2. Theta-range oscillations as a target of oscillotherapeutics. (A) Recent studies suggest that theta-range (4–12 Hz) oscillations in the HPC-PFC-AMY circuit are crucial for emotionalbehavior and susceptibility to stress. (B) Examples of stimulation patterns (orange) upon brain oscillatory signals (magenta) in the open-loop and closed-loop systems. (C) (Top) Closed-loop stimulation is applied to the brain region where a target brain signal is recorded. (Bottom) Closed-loop stimulation is applied to a brain region that is different from the brain region where a target signal is recorded.
Dysregulation of emotions and increased anxiety are crucial hallmarks of stress-induced mental disorders in both animal models and human patients. Regarding rodent studies, behavioral paradigms have been established to subjectively estimate the levels of emotional valence and anxiety. From these behavioral experiments, a number of studies with genetic and pharmacological approaches have suggested that the HPC-PFC-AMY regions is a hub network related to fear and anxiety-like behavior (as reviewed by Tovote et al., 2015). In particular, electrophysiological studies have indicated the importance of theta-range (4–12 Hz, including multiple distinct oscillations) LFP signals in the HPC-PFC-AMY circuit related to emotional behavior as potential substrates for temporal circuit coordination and long-term plasticity in neuronal networks (Figure 2A; as reviewed by Caliskan and Stork, 2019). Experimentally, such low-frequency signals are a good model for a closed-loop system (e.g., phase-targeting stimulation) to test their causal roles in behavior and to associate neuronal activity patterns with single spike levels. Here, we focus on several major findings of theta-range LFP oscillations in the HPC-PFC-AMY regions observed in non-pathological animals, which are subsequently discussed in later chapters from the perspective of pathology. Details regarding the involvement of the other oscillations and the other brain regions are beyond the scope of this paper.
Fear is an adaptive component of transient responses to internal and external aversive events such as potentially threatening stimuli. Fear conditioning tests are often used to assess rodents’ learned fear, in which a conditioned stimulus (e.g., auditory stimulus) is paired with an aversive unconditioned stimulus. During an acquisition phase of fear conditioning, the mPFC-BLA circuit has been shown to increase 4-Hz LFP power (Karalis et al., 2016; Davis et al., 2017). During REM sleep periods after fear conditioning, the vHPC-mPFC-BLA circuit exhibits long-lasting enhancement of theta (4–12 Hz) power and interregional theta synchrony for hours (Popa et al., 2010; Ognjanovski et al., 2014; Totty et al., 2017), possibly serving as a substrate to consolidate fear memories (Boyce et al., 2016). During retrieval phases where the same conditioned stimulus is applied, similar tendencies of the two types of LFP oscillations are detected; increased mPFC-BLA 4-Hz (Dejean et al., 2016; Karalis et al., 2016; Ozawa et al., 2020) and HPC-mPFC-BLA theta (4–12 Hz) oscillations (Seidenbecher et al., 2003; Likhtik et al., 2014; Stujenske et al., 2014), each of which entrain oscillatory spike patterns of cell ensembles in this frequency band. Note that Karalis et al. (2016) suggested that the mPFC-BLA 4-Hz oscillation is distinct from the theta oscillations with higher frequency ranges as they are generated through different mechanisms. First, medial septum inactivation selectively eliminated the theta oscillations, possibly via the HPC, while the mPFC 4-Hz oscillation remained intact, which suggests that medial septum is an upstream brain region providing theta-locked inputs to the hippocampus (Buzsaki, 2002) but not 4 Hz-locked inputs to the mPFC. Second, HPC theta oscillations appear to represent atropine-sensitive type 2 theta oscillations as they are specific to periods of immobility (Seidenbecher et al., 2003) and responses to danger (e.g., predator odor) (Mikulovic et al., 2018). This insight suggests that HPC-mPFC-BLA theta oscillations depend on cholinergic inputs, possibly through the medial septum. Third, dmPFC interneurons exhibit spike patterns phase-locked to 4-Hz oscillations (Karalis et al., 2016) and selective activation of dmPFC parvalbumin (PV)-expressing interneurons replicates dmPFC 4-Hz oscillation (Dejean et al., 2016). These results suggest that dmPFC 4-Hz oscillation is intrinsically induced from the inhibitory neuronal circuit in the dmPFC.
Manipulation of the mPFC 4-Hz oscillations is useful to test their causal roles in fear expression and retrieval. Karalis et al. (2016) has demonstrated that optogenetic induction of dmPFC 4-Hz oscillations drives conditioned freezing and Dejean et al. (2016) has demonstrated that inhibition of dmPFC principal neurons in the descending phase of the oscillation increased conditioned freezing. These results suggest the sufficiency of dmPFC 4-Hz oscillations in the induction of learned freezing behavior.
Conditioned fear memories are extinguished by repeated presentations of the conditioned stimulus alone without presentation of an unconditioned stimulus, which is termed extinction learning. Consistent with increased theta oscillations during freezing throughout the phases of fear conditioning, theta coupling across the HPC-mPFC-BLA regions declined as animals underwent extinction learning, whereas it recurred during extinction recall (Lesting et al., 2011). Davis et al. (2017) demonstrated that increased power of a 3–6-Hz BLA oscillation, possibly classified as a 4-Hz oscillation, induced postextinction fear memory retrieval. In contrast, outcompeting this BLA oscillation by chemogenetic inhibition of BLA parvalbumin-positive interneurons (Davis et al., 2017) or 8-Hz sinusoidal stimulation (Ozawa et al., 2020) could inhibit the recurrence of fear behavior following extinction learning, which suggests the necessity of a 4-Hz oscillation in fear extinction recall and/or the induction of conditioned freezing.
Anxiety is an emotional state characterized by an unpleasant state with heightened awareness even without actual exposure to danger. Anxiety-like behavior in rodents is generally assessed in an elevated plus maze (EPM) test or an open field (OF) test. Through these behavioral tests, a number of studies have suggested that the expression of both fear and anxiety is mediated by the HPC-PFC-AMY circuit, possibly through overlapping neuronal mechanisms (Tovote et al., 2015). As a typical example, mice lacking serotonin 1A receptors showing higher anxiety-like behavior in an EPM test exhibited larger increases in HPC theta (4–12 Hz) power (Gordon et al., 2005). In contrast, treatment with anxiolytics, such as serotonin 1A receptor agonists and benzodiazepines, has been shown to exhibit decreases in HPC theta oscillations (Zhu and McNaughton, 1995). Adhikari et al. (2010) recorded LFP signals simultaneously from the mPFC and vHPC in mice engaging in an EPM test. They showed that theta-frequency communication between the mPFC and vHPC was specifically augmented under anxiogenic environments in an EPM and an OF and correlated with behavioral performance (Adhikari et al., 2010). At a single-neuron level, mPFC neuronal firing patterns were precisely entrained by vHPC theta oscillations (Adhikari et al., 2011), which were related to anxiogenic behavior in an EPM. Together with the fact that the vHPC neurons preferentially send anxiety-related information to the mPFC (Ciocchi et al., 2015), it is conceivable that vHPC theta oscillations lead to mPFC theta-locked spiking activity, and such theta-locked mPFC neuronal activity is crucial for the expression of anxiety. This idea was further supported by the observation that artificial oscillatory activation of mPFC-projecting vHPC neurons at a theta (8-Hz) frequency increased anxiety-like behavior in an EPM test (Padilla-Coreano et al., 2019). In addition, Likhtik et al. (2014) demonstrated that mPFC theta oscillations precede BLA spiking activity and that coherence and power changes at the theta band in the mPFC-BLA circuit predicted stay time in anxiogenic environments. These results all suggest that interregional coordination of neuronal population activity in theta bands in the vHPC-mPFC-BLA circuit is crucial for the expression of anxiogenic behavior. Together with the observations of fear-related theta-range oscillations, anxiogenic behavior and fear behavior may be expressed partly through a common mechanism: theta-range power increases as a means for enhanced synchronization and entrainment of the HPC-PFC-AMY circuit.
The findings of theta-range oscillations in the HPC-PFC-AMY circuitfrom non-pathological animals related to fear and anxiety-like behavior suggest that stress susceptibility and stress-induced dysregulation of emotion may be due to altered interplay based on these oscillatory communications. In rodents, behavioral and physiological changes reminiscent of depressive symptoms are induced by repeated exposures to chronic social defeat stress in which a mouse is defeated by a larger animal; this procedure has been utilized as an excellent murine model with etiological, predictive, discriminative and face validity (Berton et al., 2006; Golden et al., 2011; Abe et al., 2019). Socially defeated mice with impaired extinction learning, possibly replicating a PTSD symptom, showed increased theta (4–8 Hz) synchronization between the PFC and AMY (Narayanan et al., 2011). In addition, defeated mice with depressive-like behavior showed increased power of a PFC 2–7-Hz oscillation during interactions with an aggressor mouse (Kumar et al., 2014), which entrained beta (14–23 Hz) coherence between the AMY and ventral tegmental area (VTA) (Hultman et al., 2016). These stress-sensitive changes in oscillatory patterns possibly represented an increase in the 4-Hz or theta oscillations based on their frequency bands and common properties. Consistently, increased theta (4–12 Hz) power in the vHPC-mPFC-BLA circuit has also been reported in a chronic unpredictable stress model (Jacinto et al., 2013; Oliveira et al., 2013). Taken together, these findings suggest that stressed animals appear to exacerbate aversive emotion-related oscillations in the HPC-PFC-AMY circuit that originally operated in non-pathological conditions.
Notably, the power of this 2–7-Hz PFC oscillation differs across individual animals even before stress exposure, and these differences can predict the manifestation of subsequent stress-induced depression-like behavior (Kumar et al., 2014). This finding suggested that LFP signals can serve as a predictive factor of vulnerability to mental stress in individual animals. Recently, this idea of predictive stress vulnerability has been expanded to multiple brain regions, including the nucleus accumbens (NAc) and VTA, in addition to the HPC-PFC-AMY circuit. An elegant study with a machine learning algorithm by Hultman et al. (2018) identified several patterns of prestress LFP power and coherence in frequency bands ranging from 1 to 50 Hz across these brain regions and termed these patterns electome factors. These factors differentiate early life-associated stress vulnerability and stress susceptibility that can be reversed by antidepressants. Our group applied a similar strategy to LFP signals recorded from multiple cortical areas and demonstrated that rats with lower theta power and higher delta power correlations across the cortical regions before stress exposure were more likely to exhibit irregular heartbeat signals after stress load (Nakayama et al., 2019). In the future, these new types of studies with multivariate statistics and machine learning algorithms on large-scale physiological datasets are expected to reveal how the core region, i.e., the HPC-PFC-AMY circuit, and neuromodulatory regions, such as the NAc and VTA, functionally interact with each other. These new approaches are expected to provide a more comprehensive entire picture of functional organizations of brain networks that have not been defined by existing statistics with limited dimensions.
While LFP oscillatory signals in the HPC-PFC-AMY circuit related to stress-induced symptoms are beginning to be revealed (Figure 2A), they remain correlative and leave open questions as to whether they are causal factors for the pathogenesis of mental disorders. Addressing these issues will help identify true therapeutic targets of endogenous oscillatory signals in the development of oscillotherapeutics. Ideal research strategies are to selectively manipulate target ongoing oscillatory signals using open-loop or closed-loop systems and test their phenotypic effects. In open-loop interventions, external stimulation with sinusoidal waveforms, mimicking oscillatory signals, or pulse trains is applied without feedback from biological oscillatory signals (Figure 2B, left). In closed-loop interventions, stimulation is applied with the appearance of target brain signals, enabling on-demand stimulation with reference to brain states while avoiding out-of-target overstimulation (Figure 2B, right). Target signal variables to be detected are transient neuronal events with certain amplitudes, such as ictal seizure events, or the phase and amplitude of specific continuous oscillations, such as theta oscillations. Signals to be manipulated are the signals detected at the recording sites or signals generated at distant areas, such as upstream or downstream brain regions, depending on the locations of stimulation (Figure 2C). This chapter summarizes recent studies employing these experimental challenges in rodents and discusses the possibility of further clinical applications. Other therapeutic strategies using pharmacological, behavioral, and psychological methods to alter brain oscillations (e.g., Leuchter et al., 2015) are beyond the scope of this paper.
Transient neuronal events within a short time window (hundreds ofmilliseconds) are widely utilized target signals for closed-loopsystems. For instance, transcranial and intracranial closed-loopstimulation at the time of detection of large amplitude andhigh-frequency cortical seizure events have been shown to suppresssubsequent seizures in epilepsy animal models (Berenyi et al., 2012; Takeuchi et al., 2021). This technique has also been applied to LFP oscillations under non-pathological conditions to test their causal roles in memory processing. For example, closed-loop amplification and disruption of hippocampal ripples (150–250 Hz), which represent transient neuronal synchronization within a short time window (∼100 ms), can improve (Fernandez-Ruiz et al., 2019) and inhibit (Jadhav et al., 2012; Oliva et al., 2020; Igata et al., 2021), respectively, subsequent memory processing. While targeting this type of transient signal is an excellent means to manipulate large short neuronal events, it appears inappropriate for modulating emotional states, as brain signals underlying emotion and stress-induced disease are much longer and oscillate in lower frequency bands, as described above.
Given the importance of theta-range (4–12 Hz) oscillations in the HPC-PFC-AMY circuit in emotional behavior and stress-induced psychiatric disorders, manipulation of neuronal activity that impact these theta-range oscillations might be effective in ameliorating psychiatric symptoms. Using open-loop systems, theta-range oscillations can be exogenously induced by injecting sinusoidal stimulation at the corresponding frequencies. In particular, optogenetic photostimulation is appropriate for creating sinusoidal stimulus patterns with particular rise and decay amplitudes to manipulate specific types of neurons. Padilla-Coreano et al. (2019) demonstrated that selective activation of mPFC-projecting neurons in the vHPC by optogenetic photostimulation with a theta (8-Hz) sinusoidal pattern evoked open arm avoidance in an EPM, suggesting increased anxiety. Interestingly, this effect was not observed when an 8-Hz pulsatile stimulation pattern was applied, suggesting the importance of oscillatory neuronal activity. Karalis et al. (2016) demonstrated that modulation of mPFC parvalbumin-expressing (PV) interneurons by 4-Hz, but not 8-Hz, sinusoidal photostimulation induced synchronized mPFC and BLA spikes and contextual fear behavior, suggesting a causal role of 4-Hz oscillations in the expression of aversive memories. Furthermore, using a similar strategy, Ozawa et al. (2020) showed that rhythmic stimulation of BLA PV interneurons with a sinusoidal waveform of light at 4 Hz or 8 Hz augmented or suppressed freezing behavior, respectively, specifically after a postextinction learning trial, demonstrating the bidirectional modulation of extinction memories by BLA PV interneurons depending on different oscillatory frequencies. In addition, they suggested that extinction learning with suppression of conditioned fear cell ensembles in the BLA-mPFC circuit was mediated by BLA PV interneurons via enhancement of a BLA 8-Hz (6–12 Hz) oscillation that interfered with a BLA-mPFC 4-Hz (3–6 Hz) oscillation for fear expression (Davis et al., 2017). Further causal relationships can be tested by a closed-loop system with higher temporal resolution in which stimulation is applied at a specific phase of each cycle of oscillations. Dejean et al. (2016) demonstrated that phase-targeting optogenetic activation of mPFC PV interneurons in the ascending or descending phases of mPFC 4-Hz oscillations decreases and increases conditioned freezing behavior, respectively, suggesting the importance of phase-specific mPFC cell ensemble activity for bidirectional control of fear behavior. Using similar experimental strategies, increased anxiety and impaired fear extinction by chronic stress might be restored by adjusting the intensity of theta-range oscillations and phase-specific modulation of neuronal activity on theta-range oscillations in the HPC-PFC-AMY circuit. Similar ideas that target theta-range oscillations have been recently applied to other animal models of Parkinson’s disease and Alzheimer’s disease (Cordon et al., 2018; Senova et al., 2018).
For more complex challenges regarding the understanding of functional connectivity and interregional information transfer underlying emotional behavior, manipulations of multiple brain regions at the same or different phases in oscillations may be more useful (Figure 2C), as demonstrated by Ozawa et al. (2020). Finally, animals’ behavioral states (e.g., sleep states and attentional levels) are also crucial factors in interventions of neuronal activity. For example, Boyce et al. (2016) demonstrated that a reduction in HPC theta oscillations specifically during REM sleep eliminated conditioned fear memories, suggesting that applying interventions in the proper behavioral states may be important to maximize their outputs and avoid side effects.
While it is not possible to simply extrapolate clinical applications based on insights from basic animal studies, interventions targeting brain oscillations may also be an effective therapeutic strategy in clinical studies if properly applied. Deep brain stimulation (DBS) is an invasive treatment approach for treatment-resistant depressions applied by electrodes implanted in brain tissue (Mayberg et al., 2005; Scangos et al., 2021). On the other hand, transcranial electric stimulation is a non-invasive approach that is roughly divided into two types depending on stimulus patterns: transcranial direct current stimulation (tDCS) with non-oscillating static currents and transcranial alternating current stimulation (tACS) with oscillating currents. For more focal and intense stimulation, repetitive transcranial magnetic stimulation (rTMS) applies magnetic pulses to induce current flow in the brain. Accumulating clinical evidence suggests that restorations of brain oscillations by these interventions ameliorate pathological symptoms in depressive patients. For instance, rTMS on the frontal cortex increased gamma oscillations in patients showing improved depressive symptoms (Noda et al., 2017), consistent with a pharmacological effect of ketamine on gamma oscillations (Hong et al., 2010). Vagus nerve stimulation (VNS) is another intervention approach for treatment-resistant depression (Moeller et al., 2019). This method directly activates subcortical arousal-promoting nuclei through stimulation of the vagus nerve. Recently, a non-invasive transcutaneous auricular vagus nerve stimulation (taVNS) method has been developed (Hein et al., 2013; Rong et al., 2016; Trevizol et al., 2016), which can alter alpha EEG oscillations (Sharon et al., 2021).
Compared with simple pulsatile stimulation such as tDCS, resonance approaches using oscillatory stimulus patterns such as tACS may be more effective in entraining brain oscillations at the corresponding or other specific frequency bands [for more detail, see Hanslmayr et al. (2019)]. For instance, in healthy human subjects, intense tACS with a 1-Hz sinusoidal wave has been shown to induce alpha-band parietal cortical activity (Voroslakos et al., 2018). In older adults, tACS over the frontotemporal regions improves memory performance and cognitive functions (Reinhart and Nguyen, 2019). More specifically, a closed-loop system using DBS may be a promising strategy, in which brief stimulation is delivered in response to ongoing changes in the brain states of patients (Scangos and Ross, 2018). In addition, biofeedback methods in which individuals are provided with real-time information on brain activity have been proposed as another means to modify EEG signals and improve psychiatric states. For instance, restoring frontal alpha symmetry by biofeedback signals has been shown to reduce negative affect in human subjects that learned conscious control of their own alpha asymmetry signals (Mennella et al., 2017).
Recent advancements in electrophysiological recording techniques, optogenetic tools, and analytical methods such as machine learning algorithms unveiled emotion-related and stress-induced oscillations for complex coordination of multiple brain regions. This review specifically focused on theta-range oscillations, as a key oscillation, in the HPC-PFC-AMY circuit in rodents. Precisely timed interventions based on these oscillations by open-loop and closed-loop systems enable us to test a causal role of these target oscillatory signals in the expression of psychiatric symptoms. In the future, in addition to observing changes in behavioral phenotypes, it will be interesting to see how emotional memory-encoding and oscillatory phase-locked neuronal ensembles are recruited at single-neuron levels in response to these interventions. While the same experimental strategies may not be directly extrapolated to clinical studies, the ideas of interventions are adopted as effective therapeutic strategies for stress-induced mental illness. In both animal and clinical studies, a crucial issue is that there are a large number of combinations of technical parameters (e.g., stimulation regions, type, intensity, timing, and frequency band) for interventions. In addition, as the level of impairments in these brain signals and pathological symptoms considerably vary across individuals, interventions need to be ideally personalized based on their conditions, as previously demonstrated (Reinhart and Nguyen, 2019; Grover et al., 2021). Further oscillotherapeutic studies from both basic and clinical experiments are expected to identify more appropriate intervention strategies based on a precise understanding of cellular and circuit dynamics.
TO and TS prepared all the figures and wrote the main manuscript text. Both authors contributed to the article and approved the submitted version.
This work was supported by KAKENHI (19H04897 and 20H03545) from the Japan Society for the Promotion of Science (JSPS), a Precursory Research for Embryonic Science and Technology grant (JPMJPR1785) from the Japan Science and Technology Agency (JST), and a grant from the Advanced Research and Development Programs for Medical Innovation (1041630) of the Japan Agency for Medical Research and Development (AMED) to TS; and a JSPS Research Fellowship for Young Scientists to TO.
The authors declare that the research was conducted in the absence of any commercial or financial relationships that could be construed as a potential conflict of interest.
AMY, amygdala; BLA, basolateral amygdala; LFP, local field potential; mPFC, medial prefrontal cortex; PTSD, posttraumatic stress disorder; vHPC, ventral hippocampus.
Abe, R., Okada, S., Nakayama, R., Ikegaya, Y., and Sasaki, T. (2019). Social defeat stress causes selective attenuation of neuronal activity in the ventromedial prefrontal cortex. Sci. Rep. 9:9447. doi: 10.1038/s41598-019-45833-5
Adhikari, A., Topiwala, M. A., and Gordon, J. A. (2010). Synchronized activity between the ventral hippocampus and the medial prefrontal cortex during anxiety. Neuron 65, 257–269. doi: 10.1016/j.neuron.2009.12.002
Adhikari, A., Topiwala, M. A., and Gordon, J. A. (2011). Single units in the medial prefrontal cortex with anxiety-related firing patterns are preferentially influenced by ventral hippocampal activity. Neuron 71, 898–910.
Arnsten, A. F. (2015). Stress weakens prefrontal networks: molecular insults to higher cognition. Nat. Neurosci. 18, 1376–1385. doi: 10.1038/nn.4087
Baskaran, A., Milev, R., and McIntyre, R. S. (2012). The neurobiology of the EEG biomarker as a predictor of treatment response in depression. Neuropharmacology 63, 507–513. doi: 10.1016/j.neuropharm.2012.04.021
Berenyi, A., Belluscio, M., Mao, D., and Buzsaki, G. (2012). Closed-loop control of epilepsy by transcranial electrical stimulation. Science 337, 735–737. doi: 10.1126/science.1223154
Berton, O., McClung, C. A., Dileone, R. J., Krishnan, V., Renthal, W., Russo, S. J., et al. (2006). Essential role of BDNF in the mesolimbic dopamine pathway in social defeat stress. Science 311, 864–868. doi: 10.1126/science.1120972
Boyce, R., Glasgow, S. D., Williams, S., and Adamantidis, A. (2016). Causal evidence for the role of REM sleep theta rhythm in contextual memory consolidation. Science 352, 812–816. doi: 10.1126/science.aad5252
Burgos-Robles, A., Kimchi, E. Y., Izadmehr, E. M., Porzenheim, M. J., Ramos-Guasp, W. A., Nieh, E. H., et al. (2017). Amygdala inputs to prefrontal cortex guide behavior amid conflicting cues of reward and punishment. Nat. Neurosci. 20, 824–835. doi: 10.1038/nn.4553
Buzsaki, G., Anastassiou, C. A., and Koch, C. (2012). The origin of extracellular fields and currents–EEG, ECoG, LFP and spikes. Nat. Rev. Neurosci. 13, 407–420. doi: 10.1038/nrn3241
Buzsaki, G., and Draguhn, A. (2004). Neuronal oscillations in cortical networks. Science 304, 1926–1929. doi: 10.1126/science.1099745
Buzsaki, G., and Watson, B. O. (2012). Brain rhythms and neural syntax: implications for efficient coding of cognitive content and neuropsychiatric disease. Dialog, Clin. Neurosci. 14, 345–367.
Caliskan, G., and Stork, O. (2019). Hippocampal network oscillations at the interplay between innate anxiety and learned fear. Psychopharmacology (Berl) 236, 321–338. doi: 10.1007/s00213-018-5109-z
Ciocchi, S., Passecker, J., Malagon-Vina, H., Mikus, N., and Klausberger, T. (2015). Brain computation. Selective information routing by ventral hippocampal CA1 projection neurons. Science 348, 560–563. doi: 10.1126/science.aaa3245
Clancy, K., Ding, M., Bernat, E., Schmidt, N. B., and Li, W. (2017). Restless ‘rest’: intrinsic sensory hyperactivity and disinhibition in post-traumatic stress disorder. Brain 140, 2041–2050. doi: 10.1093/brain/awx116
Cordon, I., Nicolas, M. J., Arrieta, S., Alegre, M., Artieda, J., and Valencia, M. (2018). Theta-phase closed-loop stimulation induces motor paradoxical responses in the rat model of Parkinson disease. Brain Stimul. 11, 231–238. doi: 10.1016/j.brs.2017.10.004
Davis, P., Zaki, Y., Maguire, J., and Reijmers, L. G. (2017). Cellular and oscillatory substrates of fear extinction learning. Nat. Neurosci. 20, 1624–1633. doi: 10.1038/nn.4651
Dejean, C., Courtin, J., Karalis, N., Chaudun, F., Wurtz, H., Bienvenu, T. C., et al. (2016). Prefrontal neuronal assemblies temporally control fear behaviour. Nature 535, 420–424. doi: 10.1038/nature18630
Drysdale, A. T., Grosenick, L., Downar, J., Dunlop, K., Mansouri, F., Meng, Y., et al. (2017). Resting-state connectivity biomarkers define neurophysiological subtypes of depression. Nat. Med. 23, 28–38. doi: 10.1038/nm.4246
Fernandez-Ruiz, A., Oliva, A., Fermino de Oliveira, E., Rocha-Almeida, F., Tingley, D., and Buzsaki, G. (2019). Long-duration hippocampal sharp wave ripples improve memory. Science 364, 1082–1086. doi: 10.1126/science.aax0758
Fitzgerald, P. J., and Watson, B. O. (2018). Gamma oscillations as a biomarker for major depression: an emerging topic. Transl. Psychiatry 8:177. doi: 10.1038/s41398-018-0239-y
Golden, S. A., Covington, H. E. III, Berton, O., and Russo, S. J. (2011). A standardized protocol for repeated social defeat stress in mice. Nat. Protoc. 6, 1183–1191. doi: 10.1038/nprot.2011.361
Gordon, J. A., Lacefield, C. O., Kentros, C. G., and Hen, R. (2005). State-dependent alterations in hippocampal oscillations in serotonin 1A receptor-deficient mice. J. Neurosci. 25, 6509–6519. doi: 10.1523/JNEUROSCI.1211-05.2005
Greicius, M. D., Flores, B. H., Menon, V., Glover, G. H., Solvason, H. B., Kenna, H., et al. (2007). Resting-state functional connectivity in major depression: abnormally increased contributions from subgenual cingulate cortex and thalamus. Biol. Psychiatry 62, 429–437. doi: 10.1016/j.biopsych.2006.09.020
Grover, S., Nguyen, J. A., Viswanathan, V., and Reinhart, R. M. G. (2021). High-frequency neuromodulation improves obsessive-compulsive behavior. Nat. Med. 27, 232–238. doi: 10.1038/s41591-020-01173-w
Grunewald, B. D., Greimel, E., Trinkl, M., Bartling, J., Grossheinrich, N., and Schulte-Korne, G. (2018). Resting frontal EEG asymmetry patterns in adolescents with and without major depression. Biol. Psychol. 132, 212–216. doi: 10.1016/j.biopsycho.2018.01.003
Hanslmayr, S., Axmacher, N., and Inman, C. S. (2019). Modulating human memory via entrainment of brain oscillations. Trends Neurosci. 42, 485–499. doi: 10.1016/j.tins.2019.04.004
Hein, E., Nowak, M., Kiess, O., Biermann, T., Bayerlein, K., Kornhuber, J., et al. (2013). Auricular transcutaneous electrical nerve stimulation in depressed patients: a randomized controlled pilot study. J. Neural. Transm. (Vienna.) 120, 821–827. doi: 10.1007/s00702-012-0908-6
Hong, L. E., Summerfelt, A., Buchanan, R. W., O’Donnell, P., Thaker, G. K., Weiler, M. A., et al. (2010). Gamma and delta neural oscillations and association with clinical symptoms under subanesthetic ketamine. Neuropsychopharmacology 35, 632–640. doi: 10.1038/npp.2009.168
Huang, M. X., Yurgil, K. A., Robb, A., Angeles, A., Diwakar, M., Risbrough, V. B., et al. (2014). Voxel-wise resting-state MEG source magnitude imaging study reveals neurocircuitry abnormality in active-duty service members and veterans with PTSD. Neuroimage Clin. 5, 408–419. doi: 10.1016/j.nicl.2014.08.004
Hultman, R., Mague, S. D., Li, Q., Katz, B. M., Michel, N., Lin, L., et al. (2016). Dysregulation of prefrontal cortex-mediated slow-evolving limbic dynamics drives stress-induced emotional pathology. Neuron 91, 439–452. doi: 10.1016/j.neuron.2016.05.038
Hultman, R., Ulrich, K., Sachs, B. D., Blount, C., Carlson, D. E., Ndubuizu, N., et al. (2018). Brain-wide electrical spatiotemporal dynamics encode depression vulnerability. Cell 173, 166–180.e114. doi: 10.1016/j.cell.2018.02.012
Igata, H., Ikegaya, Y., and Sasaki, T. (2021). Prioritized experience replays on a hippocampal predictive map for learning. Proc. Natl. Acad. Sci. U.S.A. 118:e2011266118. doi: 10.1073/pnas.2011266118
Iosifescu, D. V. (2011). Electroencephalography-derived biomarkers of antidepressant response. Harv. Rev. Psychiatry 19, 144–154. doi: 10.3109/10673229.2011.586549
Ishikawa, A., and Nakamura, S. (2003). Convergence and interaction of hippocampal and amygdalar projections within the prefrontal cortex in the rat. J. Neurosci. 23, 9987–9995.
Iturra-Mena, A. M., Aguilar-Rivera, M., Arriagada-Solimano, M., Perez-Valenzuela, C., Fuentealba, P., and Dagnino-Subiabre, A. (2019). Impact of stress on Gamma oscillations in the rat nucleus accumbens during spontaneous social interaction. Front. Behav. Neurosci. 13:151. doi: 10.3389/fnbeh.2019.00151
Jacinto, L. R., Reis, J. S., Dias, N. S., Cerqueira, J. J., Correia, J. H., and Sousa, N. (2013). Stress affects theta activity in limbic networks and impairs novelty-induced exploration and familiarization. Front. Behav. Neurosci. 7:127. doi: 10.3389/fnbeh.2013.00127
Jadhav, S. P., Kemere, C., German, P. W., and Frank, L. M. (2012). Awake hippocampal sharp-wave ripples support spatial memory. Science 336, 1454–1458. doi: 10.1126/science.1217230
Jesulola, E., Sharpley, C. F., Bitsika, V., Agnew, L. L., and Wilson, P. (2015). Frontal alpha asymmetry as a pathway to behavioural withdrawal in depression: research findings and issues. Behav. Brain Res. 292, 56–67. doi: 10.1016/j.bbr.2015.05.058
Karalis, N., Dejean, C., Chaudun, F., Khoder, S., Rozeske, R. R., Wurtz, H., et al. (2016). 4-Hz oscillations synchronize prefrontal-amygdala circuits during fear behavior. Nat. Neurosci. 19, 605–612. doi: 10.1038/nn.4251
Kim, J., Pignatelli, M., Xu, S., Itohara, S., and Tonegawa, S. (2016). Antagonistic negative and positive neurons of the basolateral amygdala. Nat. Neurosci. 19, 1636–1646. doi: 10.1038/nn.4414
Konno, D., Nakayama, R., Tsunoda, M., Funatsu, T., Ikegaya, Y., and Sasaki, T. (2019). Collection of biochemical samples with brain-wide electrophysiological recordings from a freely moving rodent. J. Pharmacol. Sci. 139, 346–351. doi: 10.1016/j.jphs.2019.02.006
Krishnan, V., and Nestler, E. J. (2008). The molecular neurobiology of depression. Nature 455, 894–902. doi: 10.1038/nature07455
Kuga, N., Nakayama, R., Shikano, Y., Nishimura, Y., Okonogi, T., Ikegaya, Y., et al. (2019). Sniffing behaviour-related changes in cardiac and cortical activity in rats. J. Physiol. 597, 5295–5306. doi: 10.1113/JP278500
Kumar, S., Hultman, R., Hughes, D., Michel, N., Katz, B. M., and Dzirasa, K. (2014). Prefrontal cortex reactivity underlies trait vulnerability to chronic social defeat stress. Nat. Commun. 5:4537. doi: 10.1038/ncomms5537
Lesting, J., Narayanan, R. T., Kluge, C., Sangha, S., Seidenbecher, T., and Pape, H. C. (2011). Patterns of coupled theta activity in amygdala-hippocampal-prefrontal cortical circuits during fear extinction. PLoS One 6:e21714. doi: 10.1371/journal.pone.0021714
Leuchter, A. F., Hunter, A. M., Krantz, D. E., and Cook, I. A. (2015). Rhythms and blues: modulation of oscillatory synchrony and the mechanism of action of antidepressant treatments. Ann. N.Y. Acad. Sci. 1344, 78–91. doi: 10.1111/nyas.12742
Likhtik, E., Stujenske, J. M., Topiwala, M. A., Harris, A. Z., and Gordon, J. A. (2014). Prefrontal entrainment of amygdala activity signals safety in learned fear and innate anxiety. Nat. Neurosci. 17, 106–113. doi: 10.1038/nn.3582
Martinez, D., Clement, M., Messaoudi, B., Gervasoni, D., Litaudon, P., and Buonviso, N. (2018). Adaptive quantization of local field potentials for wireless implants in freely moving animals: an open-source neural recording device. J. Neural. Eng. 15:025001. doi: 10.1088/1741-2552/aaa041
Mayberg, H. S., Lozano, A. M., Voon, V., McNeely, H. E., Seminowicz, D., Hamani, C., et al. (2005). Deep brain stimulation for treatment-resistant depression. Neuron 45, 651–660. doi: 10.1016/j.neuron.2005.02.014
McEwen, B. S., Bowles, N. P., Gray, J. D., Hill, M. N., Hunter, R. G., Karatsoreos, I. N., et al. (2015). Mechanisms of stress in the brain. Nat. Neurosci. 18, 1353–1363. doi: 10.1038/nn.4086
Mennella, R., Patron, E., and Palomba, D. (2017). Frontal alpha asymmetry neurofeedback for the reduction of negative affect and anxiety. Behav. Res. Ther. 92, 32–40. doi: 10.1016/j.brat.2017.02.002
Mikulovic, S., Restrepo, C. E., Siwani, S., Bauer, P., Pupe, S., Tort, A. B. L., et al. (2018). Ventral hippocampal OLM cells control type 2 theta oscillations and response to predator odor. Nat. Commun. 9, 3638. doi: 10.1038/s41467-018-05907-w
Moeller, S., Lucke, C., Heinen, C., Bewernick, B. H., Aydin, M., Lam, A. P., et al. (2019). Vagus nerve stimulation as an adjunctive neurostimulation tool in treatment-resistant depression. J. Vis. Exp. 143:e58264. doi: 10.3791/58264
Nakayama, R., Ikegaya, Y., and Sasaki, T. (2019). Cortical-wide functional correlations are associated with stress-induced cardiac dysfunctions in individual rats. Sci. Rep. 9:10581. doi: 10.1038/s41598-019-47171-y
Narayanan, V., Heiming, R. S., Jansen, F., Lesting, J., Sachser, N., Pape, H. C., et al. (2011). Social defeat: impact on fear extinction and amygdala-prefrontal cortical theta synchrony in 5-HTT deficient mice. PLoS One 6:e22600. doi: 10.1371/journal.pone.0022600
Noda, Y., Zomorrodi, R., Saeki, T., Rajji, T. K., Blumberger, D. M., Daskalakis, Z. J., et al. (2017). Resting-state EEG gamma power and theta-gamma coupling enhancement following high-frequency left dorsolateral prefrontal rTMS in patients with depression. Clin. Neurophysiol. 128, 424–432. doi: 10.1016/j.clinph.2016.12.023
Nugent, A. C., Robinson, S. E., Coppola, R., Furey, M. L., and Zarate, C. A. Jr. (2015). Group differences in MEG-ICA derived resting state networks: application to major depressive disorder. Neuroimage 118, 1–12. doi: 10.1016/j.neuroimage.2015.05.051
Ognjanovski, N., Maruyama, D., Lashner, N., Zochowski, M., and Aton, S. J. (2014). CA1 hippocampal network activity changes during sleep-dependent memory consolidation. Front. Syst. Neurosci. 8:61. doi: 10.3389/fnsys.2014.00061
Okada, S., Igata, H., Sakaguchi, T., Sasaki, T., and Ikegaya, Y. (2016). A new device for the simultaneous recording of cerebral, cardiac, and muscular electrical activity in freely moving rodents. J. Pharmacol. Sci. 132, 105–108. doi: 10.1016/j.jphs.2016.06.001
Okonogi, T., Nakayama, R., Sasaki, T., and Ikegaya, Y. (2018). Characterization of peripheral activity states and cortical local field potentials of mice in an elevated plus maze test. Front. Behav. Neurosci. 12:62. doi: 10.3389/fnbeh.2018.00062
Oliva, A., Fernandez-Ruiz, A., Leroy, F., and Siegelbaum, S. A. (2020). Hippocampal CA2 sharp-wave ripples reactivate and promote social memory. Nature 587, 264–269. doi: 10.1038/s41586-020-2758-y
Oliveira, J. F., Dias, N. S., Correia, M., Gama-Pereira, F., Sardinha, V. M., Lima, A., et al. (2013). Chronic stress disrupts neural coherence between cortico-limbic structures. Front. Neural Circ. 7:10. doi: 10.3389/fncir.2013.00010
Orsini, C. A., Kim, J. H., Knapska, E., and Maren, S. (2011). Hippocampal and prefrontal projections to the basal amygdala mediate contextual regulation of fear after extinction. J. Neurosci. 31, 17269–17277. doi: 10.1523/JNEUROSCI.4095-11.2011
Ozawa, M., Davis, P., Ni, J., Maguire, J., Papouin, T., and Reijmers, L. (2020). Experience-dependent resonance in amygdalo-cortical circuits supports fear memory retrieval following extinction. Nat. Commun. 11:4358. doi: 10.1038/s41467-020-18199-w
Padilla-Coreano, N., Canetta, S., Mikofsky, R. M., Alway, E., Passecker, J., Myroshnychenko, M. V., et al. (2019). Hippocampal-prefrontal theta transmission regulates avoidance behavior. Neuron 104, 601–610.e604. doi: 10.1016/j.neuron.2019.08.006
Popa, D., Duvarci, S., Popescu, A. T., Lena, C., and Pare, D. (2010). Coherent amygdalocortical theta promotes fear memory consolidation during paradoxical sleep. Proc. Natl. Acad. Sci. U.S.A. 107, 6516–6519. doi: 10.1073/pnas.0913016107
Reinhart, R. M. G., and Nguyen, J. A. (2019). Working memory revived in older adults by synchronizing rhythmic brain circuits. Nat. Neurosci. 22, 820–827. doi: 10.1038/s41593-019-0371-x
Rong, P., Liu, J., Wang, L., Liu, R., Fang, J., Zhao, J., et al. (2016). Effect of transcutaneous auricular vagus nerve stimulation on major depressive disorder: a nonrandomized controlled pilot study. J. Affect. Disord. 195, 172–179. doi: 10.1016/j.jad.2016.02.031
Scangos, K. W., Makhoul, G. S., Sugrue, L. P., Chang, E. F., and Krystal, A. D. (2021). State-dependent responses to intracranial brain stimulation in a patient with depression. Nat. Med. 27, 229–231. doi: 10.1038/s41591-020-01175-8
Scangos, K. W., and Ross, D. A. (2018). What We’ve got here is failure to communicate: improving interventional psychiatry with closed-loop stimulation. Biol. Psychiatry 84, e55–e57. doi: 10.1016/j.biopsych.2018.08.005
Seidenbecher, T., Laxmi, T. R., Stork, O., and Pape, H. C. (2003). Amygdalar and hippocampal theta rhythm synchronization during fear memory retrieval. Science 301, 846–850. doi: 10.1126/science.1085818
Senn, V., Wolff, S. B., Herry, C., Grenier, F., Ehrlich, I., Grundemann, J., et al. (2014). Long-range connectivity defines behavioral specificity of amygdala neurons. Neuron 81, 428–437. doi: 10.1016/j.neuron.2013.11.006
Senova, S., Chaillet, A., and Lozano, A. M. (2018). Fornical closed-loop stimulation for alzheimer’s disease. Trends Neurosci. 41, 418–428. doi: 10.1016/j.tins.2018.03.015
Sharon, O., Fahoum, F., and Nir, Y. (2021). Transcutaneous vagus nerve stimulation in humans induces pupil dilation and attenuates alpha oscillations. J. Neurosci. 41, 320–330. doi: 10.1523/JNEUROSCI.1361-20.2020
Sheline, Y. I., Price, J. L., Yan, Z., and Mintun, M. A. (2010). Resting-state functional MRI in depression unmasks increased connectivity between networks via the dorsal nexus. Proc. Natl. Acad. Sci. U.S.A. 107, 11020–11025. doi: 10.1073/pnas.1000446107
Shikano, Y., Ikegaya, Y., and Sasaki, T. (2018). Monitoring brain neuronal activity with manipulation of cardiac events in a freely moving rat. Neurosci. Res. 136, 56–62. doi: 10.1016/j.neures.2018.02.004
Shikano, Y., Nishimura, Y., Okonogi, T., Ikegaya, Y., and Sasaki, T. (2019). Vagus nerve spiking activity associated with locomotion and cortical arousal states in a freely moving rat. Eur. J. Neurosci. 49, 1298–1312. doi: 10.1111/ejn.14275
Siegle, J. H., and Wilson, M. A. (2014). Enhancement of encoding and retrieval functions through theta phase-specific manipulation of hippocampus. eLife 3:e03061. doi: 10.7554/eLife.03061
Stujenske, J. M., Likhtik, E., Topiwala, M. A., and Gordon, J. A. (2014). Fear and safety engage competing patterns of theta-gamma coupling in the basolateral amygdala. Neuron 83, 919–933. doi: 10.1016/j.neuron.2014.07.026
Takeuchi, Y., and Berenyi, A. (2020). Oscillotherapeutics – Time-targeted interventions in epilepsy and beyond. Neurosci. Res. 152, 87–107. doi: 10.1016/j.neures.2020.01.002
Takeuchi, Y., Harangozo, M., Pedraza, L., Foldi, T., Kozak, G., Li, Q., et al. (2021). Closed-loop stimulation of the medial septum terminates epileptic seizures. Brain 144, 885–908. doi: 10.1093/brain/awaa450
Totty, M. S., Chesney, L. A., Geist, P. A., and Datta, S. (2017). Sleep-dependent oscillatory synchronization: a role in fear memory consolidation. Front. Neural. Circ. 11:49. doi: 10.3389/fncir.2017.00049
Tovote, P., Fadok, J. P., and Luthi, A. (2015). Neuronal circuits for fear and anxiety. Nat. Rev. Neurosci. 16, 317–331. doi: 10.1038/nrn3945
Trevizol, A. P., Shiozawa, P., Taiar, I., Soares, A., Gomes, J. S., Barros, M. D., et al. (2016). Transcutaneous vagus nerve stimulation (taVNS) for major depressive disorder: an open label proof-of-concept trial. Brain Stimul. 9, 453–454. doi: 10.1016/j.brs.2016.02.001
Voroslakos, M., Takeuchi, Y., Brinyiczki, K., Zombori, T., Oliva, A., Fernandez-Ruiz, A., et al. (2018). Direct effects of transcranial electric stimulation on brain circuits in rats and humans. Nat. Commun. 9, 483. doi: 10.1038/s41467-018-02928-3
Yehuda, R., and LeDoux, J. (2007). Response variation following trauma: a translational neuroscience approach to understanding PTSD. Neuron 56, 19–32. doi: 10.1016/j.neuron.2007.09.006
Zhu, X. O., and McNaughton, N. (1995). Minimal changes with long-term administration of anxiolytics on septal driving of hippocampal rhythmical slow activity. Psychopharmacology (Berl) 118, 93–100. doi: 10.1007/BF02245254
Keywords: oscillations, emotion, depression, anxiety, hippocampus, amygdala, prefrontal cortex
Citation: Okonogi T and Sasaki T (2021) Theta-Range Oscillations in Stress-Induced Mental Disorders as an Oscillotherapeutic Target. Front. Behav. Neurosci. 15:698753. doi: 10.3389/fnbeh.2021.698753
Received: 22 April 2021; Accepted: 14 May 2021;
Published: 09 June 2021.
Edited by:
Yuichi Takeuchi, Osaka City University, JapanReviewed by:
Brendon O. Watson, University of Michigan, United StatesCopyright © 2021 Okonogi and Sasaki. This is an open-access article distributed under the terms of the Creative Commons Attribution License (CC BY). The use, distribution or reproduction in other forums is permitted, provided the original author(s) and the copyright owner(s) are credited and that the original publication in this journal is cited, in accordance with accepted academic practice. No use, distribution or reproduction is permitted which does not comply with these terms.
*Correspondence: Takuya Sasaki, dHNhc2FraUBtb2wuZi51LXRva3lvLmFjLmpw
Disclaimer: All claims expressed in this article are solely those of the authors and do not necessarily represent those of their affiliated organizations, or those of the publisher, the editors and the reviewers. Any product that may be evaluated in this article or claim that may be made by its manufacturer is not guaranteed or endorsed by the publisher.
Research integrity at Frontiers
Learn more about the work of our research integrity team to safeguard the quality of each article we publish.