- 1Department of Neurology, Chinese PLA General Hospital, Beijing, China
- 2Department of Neurology, Chinese PLA 305 Hospital, Beijing, China
Object-location memory is particularly fragile and specifically impaired in Alzheimer’s disease (AD) patients. Electroencephalogram (EEG) was utilized to objectively measure memory impairment for memory formation correlates of EEG oscillatory activities. We aimed to construct an object-location memory paradigm and explore EEG signs of it. Two groups of 20 probable mild AD patients and 19 healthy older adults were included in a cross-sectional analysis. All subjects took an object-location memory task. EEG recordings performed during object-location memory tasks were compared between the two groups in the two EEG parameters (spectral parameters and phase synchronization). The memory performance of AD patients was worse than that of healthy elderly adults The power of object-location memory of the AD group was significantly higher than the NC group (healthy elderly adults) in the alpha band in the encoding session, and alpha and theta bands in the retrieval session. The channels-pairs the phase lag index value of object-location memory in the AD group was clearly higher than the NC group in the delta, theta, and alpha bands in encoding sessions and delta and theta bands in retrieval sessions. The results provide support for the hypothesis that the AD patients may use compensation mechanisms to remember the items and episode.
Introduction
Alzheimer’s disease (AD) is a degenerative brain disease, and episodic memory impairment is an early sign of it. Numerous studies have focused on the early detection of episodic memory impairment to resolve the differential diagnosis, estimate the disease progression, and determine eligible treatment.
Spatial memory, remembering the place of objects in our environment, is a prominent aspect of episodic memory. It is fundamental to human survival in everyday life. A few studies have demonstrated that spatial memory of AD patients is impaired, such as route learning tasks and scene memory assessment (Cherrier et al., 2001; deIpolyi et al., 2007; Iaria et al., 2007; Bird et al., 2010; Moodley et al., 2015). It was reported that impairments of spatial memory are typical and early symptoms of AD (Parra et al., 2010; Pertzov et al., 2012, 2013).
Memory for object-location is one type of spatial information memory. A number of investigations revealed that object-location memory is particularly fragile (Parra et al., 2010; Pertzov et al., 2012) and specifically impaired in patients whose damage area focused on medial temporal lobes (MTLs) (Pertzov et al., 2013, 2015). Thus, memory for object-location needs to be better researched in AD.
A few spatial memory paradigms have been used to study object-location memory in AD. The common object-location memory test consists of two procedures, including showing participants objects and performing recognition memory tests with time delay. For instance, patients were asked to pick out the items they have seen before and relocated them to previous positions (Kessels et al., 2000); the patients relocated the same objects to the positions previously presented (positions-only condition), different objects to the position marked by dots (object-to-position-assignment condition), different objects to the position without marks (combined condition) (Postma and De Haan, 1996; Kessels et al., 2002, 2010; van Asselen et al., 2008; Mazurek et al., 2015). Some paradigms also use pictures of real-life objects (e.g., buildings) on a map to recognize objects-location pairings (Kulzow et al., 2014; Edler et al., 2015). The 4 Mountains Test (4MT) is another brief behavioral test of spatial memory, and performance on it has been found to diagnose AD with high sensitivity and specificity (Hartley et al., 2007; Bird et al., 2010; Moodley et al., 2015). The primary design for 4MT paradigms encompassed spatial and non-spatial memory perceptions. The next two conditions involve alterations of light and vegetation color (Moodley et al., 2015). In this study, we used a new object-location memory task with less influence from other cognitive processing, in order to better understanding the spatial memory impairment in AD patients.
Electroencephalogram (EEG) has been used as the method to measure spatial memory. Numerous studies have found that brain oscillatory correlates of memory formation (Hanslmayr and Staudigl, 2014; Lee and Yang, 2014), and almost all frequency bands, from 3 Hz up to 100 Hz, are associated with the formation of memory (Nyhus and Curran, 2010; Hanslmayr et al., 2012; Hanslmayr and Staudigl, 2014). For example, activity in the theta and gamma frequency bands changes during the encoding and retrieval phases of a working-memory task, and the rate of correct responses correlated with the synchronization index. The analysis of electrophysiological data obtained while a spatial memory task is being performed may provide information about the function of neuronal systems involved in the type of spatial memory activity investigated. Theta band oscillatory in post-rhinal cortex and gamma band in CA3 of hippocampus were correlated with objects place memory in rats (Lu et al., 2011; Furtak et al., 2012). Delta, theta, gamma bands oscillations were correlated with spatial navigation in human (Snider et al., 2013; Park et al., 2014). P300, N200, N300 were the factors in an integrated object-location task in event-related potentials (ERPs) study (Simon-Thomas et al., 2003; van Hoogmoed et al., 2012).
Compared to the controls, AD patients showed an increase in slow (theta and delta) activities and a decrease in fast (alpha and beta) activities in resting-state EEG (Bennys et al., 2001), and decreased coherence at the alpha bands and beta bands (Locatelli et al., 1998; Leocani and Comi, 1999; Knott et al., 2000; Stam et al., 2003). The differences in spectral power and functional connectivity during cognitive tasks in relation to memory decline have also been studied (Rypma and D’Esposito, 1999; Hidasi et al., 2007; Bajo et al., 2010). Nonetheless, few oscillatory activity studies for object-location memory have been reported in AD patients.
Therefore, the aim of this study was to construct an object-location memory paradigm and explore objective signs of it, using parameters of electrophysiological EEG signal (spectral parameters and phase synchronization) in AD patients.
Materials and Methods
Subjects
The patient group included 20 mild AD patients, while the normal control (NC) group included 19 healthy older adults. The two groups were gender, age, and education matched (Table 1). All subjects were right-handed. The study was approved by the PLA general hospital Ethical Committee, and was performed in accordance with the Helsinki declaration. Written informed consent was obtained from all participants before study enrollment.
Alzheimer disease patients fulfilled the criteria of probable AD dementia according to the National Institute on Aging-Alzheimer’s Association workgroups on diagnostic guidelines for AD (McKhann et al., 2011). Each patient was interviewed by expert neurologists and received a comprehensive medical assessment, including demographic data, past medical history, physical and neurological assessment, neuropsychological test, blood-screening tests, and structural magnetic resonance imaging (MRI) brain scan, to diagnose and exclude other causes of dementia.
All subjects took part in a battery of neuropsychological assessments, including Mini-Mental State Examination (MMSE) (Folstein et al., 1975), Clinical Dementia Rating (CDR) (Hughes et al., 1982; Morris, 1993), Neuropsychiatric Inventory Questionnaire (NPI) (Cummings et al., 1994), the Bristol Activities of Daily Living Scale (BADLS) (Bucks et al., 1996), Hachinski Ischemic Score (HIS) (Rosen et al., 1980), Rey-Osterrieth complex figure (ROCF) immediate recall and 10 min delay recall (Lezak, 2004; Strauss et al., 2006). The Taylor scoring unit method was used in scoring ROCF recall (Lezak, 2004). Patients diagnosed with mild AD in the patient group met the scores (20 ≤ MMSE ≤ 26, CDR = 1) in the neuropsychological test.
Experimental Paradigm
The pictures used in this study were the same as the Snodgrass, some of which were selected by Chinese researchers to fit for Chinese subjects and were normative measured (Snodgrass and Vanderwart, 1980; Cycowicz et al., 1997). Those pictures were line drawings of common objects. From the total pool, 36 pictures were randomly selected. Pictures presented on the right or left were counterbalanced according to name agreement, familiarity, and visual complexity. E-prime software (version 2.0, Psychological Software Tools, Inc.) was used to present pictures and collect responses. All subjects were given the instructions prior to the test and there was a practice test consisting of two left pictures and two right pictures that the experimenter and subjects performed together in order to be sure that the subject completely understood the procedure. The practice pictures were different from those in the test phase.
All presentations were on a 22-inch computer screen. The process was shown in Figure 1. The test started with the instructions displayed on the screen for 30 s, followed by a 1000 ms blank. Afterward, each session started with the presentation of a black square, similar in size to the test phase questions, for 3000 ms. In the encoding session, six pictures were randomly presented on the left and six on the right. Each picture was displayed for up to 3000 ms with a 1000 ms inter-stimulus-interval (ISI). While the pictures were being presented, subjects were instructed to press the “left” or “right” button on the keyboard to indicate the location of the picture. The duration of this screen was response terminated. The subjects were instructed to memorize the pictures for the subsequent memory test in the retrieval session.
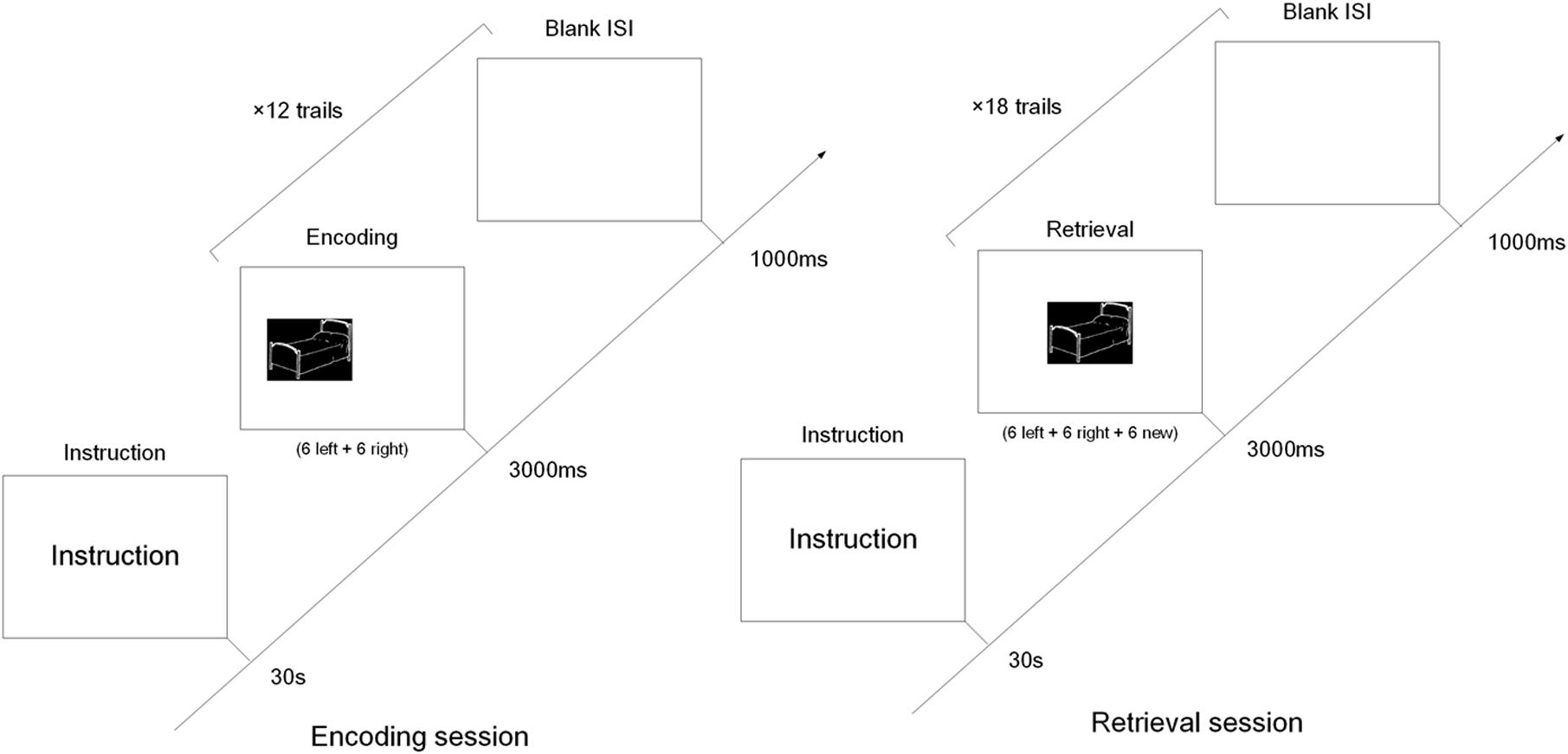
FIGURE 1. The diagram of the encoding and retrieval session of the object-location memory task. In the encoding session, six object pictures were presented left and another six object pictures right. In the retrieval session, the 12 pictures from the encoding session were randomly intermixed with six new pictures, and all pictures were presented in the middle of screen.
The retrieval session was preceded by 30 s of rest following the encoding session. The 12 pictures in the encoding session were randomly intermixed with six new. All pictures were presented in the middle of the screen, and for the same length as in the encoding session. Subjects were instructed to press the “left” or “right” button when they recalled the location of pictures presented in the encoding session. If the picture wasn’t shown in the encoding session, they also pressed the “right” button (Cycowicz et al., 2001, 2008).
EEG Recording
The EEG was recorded using 64 silver chloride electrodes mounted in an elastic cap using an ANT REFA-128 EEG measurement system (ANT Software BV, Enschede, The Netherlands). The electrodes were positioned according to an extended version of the international 10/20 system (Klem et al., 1999), and the electrode impedance was kept below 10 kΩ. Reference electrodes were placed on the mastoids. The vertical EOG (VEOG) was recorded from electrodes above and below the left eye. The EEG and EOG were recorded continuously at 512 Hz, high-pass filtered at 0.1 Hz and low-pass filtered at 100 Hz.
Behavioral Performance
The trials with pictures located on the left in the encoding session were divided into two categories depending on the response given in the retrieval session: later remembered the location (LR-L) and later forgotten the location (LF-L). Trials in the retrieval session were classified as correctly recalled total (CR-T) [CR-T = correctly identified previously seen and recalled the location of pictures (HIT) + correctly rejected new stimuli (CR)] and false recalled total (FR-T) [FR-T = not recognized the location of old pictures (MISS) + new pictures incorrectly identified as old, i.e., false alarms (FAs)].
EEG Data Analysis
Preprocessing
Electroencephalogram data preprocessing and analysis were performed using the Matlab R2012b software (MathWorks, Natick, MA, United States) and the EEGLAB software1. The average of the left and right mastoid was set as the reference electrode, and band-pass filtered from 0.1 and 45 Hz. Next, the EEG was segmented into epochs ranging from the stimulus mark appearing to the response mark appearing. Epochs and channels with visible artifacts were rejected when investigator visually inspected the EEG data. Eye movements artifacts were corrected using an independent component analysis (ICA) procedure. After visible artifacts correction, automated rejection of other EEG artifacts (e.g., muscles) was performed (criteria for rejection: >50.00 μV voltage step per sampling point, absolute voltage value > ±120.00 μV). All epochs with artifacts were excluded from the EEG analysis.
Power Estimation
In this study, EEG power was estimated in five sub-bands, including 1–4 Hz (delta), 4–8 Hz (theta), 8–13 Hz (alpha), 13–30 Hz (beta), and 30–45 Hz (gamma).
For each channel, the power spectrum density (PSD) was estimated by the Welch method with a Hamming window of 1 s and a 50% overlap. The power in sub-band [f1 f2] for some channel “ch” is calculated as follows:
Where the psdch is the PSD of the channel. The power for region R in sub-band [f1 f2] was eventually estimated by averaging the power in sub-band [f1 f2] of channels in the region R.
Where N is the number of channels in region R.
Finally, to evaluate which area showed a differential between AD patient and healthy controls, we statistically compared all channels across subjects using a non-parametric randomization test. A cluster-based randomization approach was used (Maris and Oostenveld, 2007). The method was described previously in Lange’s work (Lange et al., 2015), and the analysis was performed using FieldTrip toolbox (Oostenveld et al., 2011). The threshold of t-values was set at a value of t = 1.96 (i.e., p = 0.05). In cases where the p-value was smaller than an alpha-level of 0.05, we concluded that data in the two groups was significantly different.
PLI Calculation
Functional connectivity between different brain regions was computed using the phase lag index (PLI) (Stam et al., 2007). The segregation of the eight brain areas [left frontal (LF) (FP1, AF7, AF3, F9, F7, F5, F3, F1), left temporal (LT) (FC5, FT7, FT9, C5, T7, T9, CP5, TP7, TP9), left parietal (LP) (FC3, FC1, C1, C3, CP1, CP3), left occipital (LO) (P1, P3, P5, P7, P9, PO3, PO7, O1), right frontal (RF) (FP2, AF8, AF4, F10, F8, F6, F4, F2), right temporal (RT) (FC6, FT8, FT10, C6, T8, T10, CP6, TP8, TP10), right parietal (RP) (FC4, FC2, C2, C4, CP2, CP4), and right occipital (RO) (P2, P4, P6, P8, P10, PO4, PO8, O2)] is the same as that in the analytical system of neuromag (Elekta Oy, Helsinki, Finland).
The PLI is a measure that quantified consistent phase lead or lag between two signals. The method of PLI computed was described previously in Zeng’s work (Zeng et al., 2015). PLI can be computed from a time series of phase differences Δϕ(tk) (k = 1 … N) as follows:
The PLI ranges between 0 and 1. A PLI of zero indicates either no coupling or coupling with a phase difference centered around 0 mod π. And a PLI of 1 indicates perfect phase locking at a value of Δϕ difference from 0 mod π. The stronger the non-zero phase locking is, the larger the PLI will be.
Phase lag index was also computed in five sub-bands, including 1–4 Hz (delta), 4–8 Hz (theta), 8–13 Hz (alpha), 13–30 Hz (beta), and 30–45 Hz (gamma). The result of PLI for all pair-wise combinations of channels is an N × N matrix (N = 61, where each entry PLI, j is the value of PLI for the channels i and j) (Stam et al., 2007).
Statistical Analysis
Student’s independent t-test was used to assess the difference of demographic data and behavioral performance data between two groups. Pearson’s r correlations were assessed between MMSE score and object-location task score in both groups. All statistical analysis were operated using IBM SPSS software (version 19.0).
Results
Behavioral Performance
The correct number and response time (RT) were calculated to compare the performance of the healthy older adults and the patients with mild AD. Details are shown in Table 2. MMSE scores (t = 10.219, p < 0.001), ROCF scores (immediate recall) (t = 5.391, p < 0.001) and ROCF scores (delayed recall) (t = 9.793, p < 0.001) of AD patients were lower than that of healthy older adults. More than half of the AD patients stated that they could not remember anything in ROCF delay recall test. The number of correctly recalled total (CR-T) was higher for the healthy older adults compared to the patients with mild AD (t = 4.192, p < 0.001). Furthermore, the number of later remembered the location was also more for the healthy older adults compared to the patients with mild AD (t = 8.722, p < 0.001). In the retrieval session, RTs for the CR-T of the NC group was significantly shorter than that of the AD group (t = -4.761, p < 0.001). MMSE correlated significantly with the number of LR-L items (r = 0.575, p < 0.01) and the number of CR-T items (r = 0.748, p < 0.01) in two groups. These results indicate that the function of object-location memory in AD patients is obviously impaired compared to healthy elders.
Power Spectrum
Power during the successful encoding and retrieval stages was compared between the AD and NC groups. In our study, only object-location on the left and subsequent remembered (LR-L) items were analyzed because they reflect successful encoding object-location binding. The comparison was run across encoding and retrieval sessions in five frequency bands. The unpaired t-test was used to compare the average power of LR-L between two groups in every band frequency. In the encoding session, as shown in Figure 2A, the power of object-location memory encoding in the alpha band of the AD group was significantly higher than the NC group (p < 0.05). There were no significant differences in the delta, beta or gamma bands in the AD and NC groups. Further cluster analysis revealed that there were topographic differences in alpha power between two groups (Figure 2B). The difference of activation areas were almost distributed in the whole brain cortical areas. The AD group seems to have a trend toward frontal area activation increase (marked in red). Channels in the right hemisphere seem to show more channels with higher alpha power than the left hemisphere. In the retrieval session, as shown in Figure 2C, the power of object-location memory encoding in the alpha and theta bands of the AD group was significantly higher than the NC group (p < 0.05), while no significant differences in the delta, beta, or gamma bands between the AD and NC groups were observed. Finally, only the average power of whole channels was significantly different, while there were no topographic differences found between the two groups.
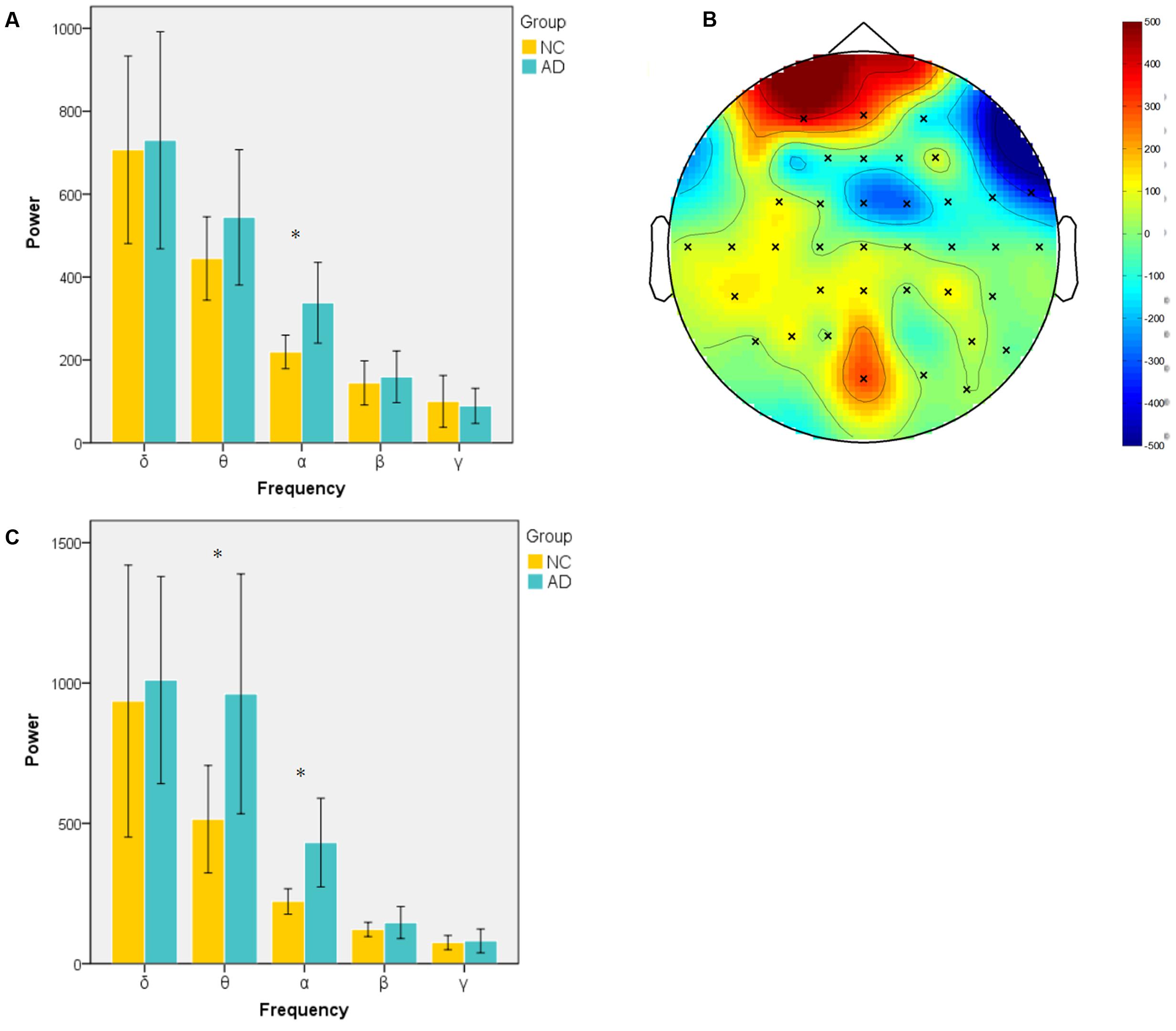
FIGURE 2. Spectral power in encoding and retrieval session. (A) Averaged power across all channels in encoding session for two groups at five frequency bands (delta, 1–4 Hz; theta, 4–8 Hz; alpha, 8–13 Hz; beta, 13–30 Hz; gamma, 30–45 Hz). Alpha band power of AD group was significantly higher than NC group; (B) topography of different areas between two groups at alpha band in encoding session (×: significantly different channels between two groups); (C) averaged power of two group in five frequency band in retrieval session. (∗p < 0.05).
Functional Connectivity
In the encoding session, differences of channel pairs PLI value between the AD and NC groups among 8 brain areas were plotted in Figure 3A. The PLI value was higher in the AD group compared to that in the NC group. The PLI value of channel pairs that showed the significant difference between two groups is shown in Figure 3B. The number of different channels-pairs were 75 (delta), 267 (theta), 243 (alpha), 482 (beta), and 183 (gamma). Combining the results of Figures 3A,B, the most obvious differences in functional connectivity between different brain regions were in both hemispheres of the frontal and parietal regions in the alpha frequency band; frontal and temporal of both hemispheres in the delta band; and almost the majority of brain regions in the theta band.
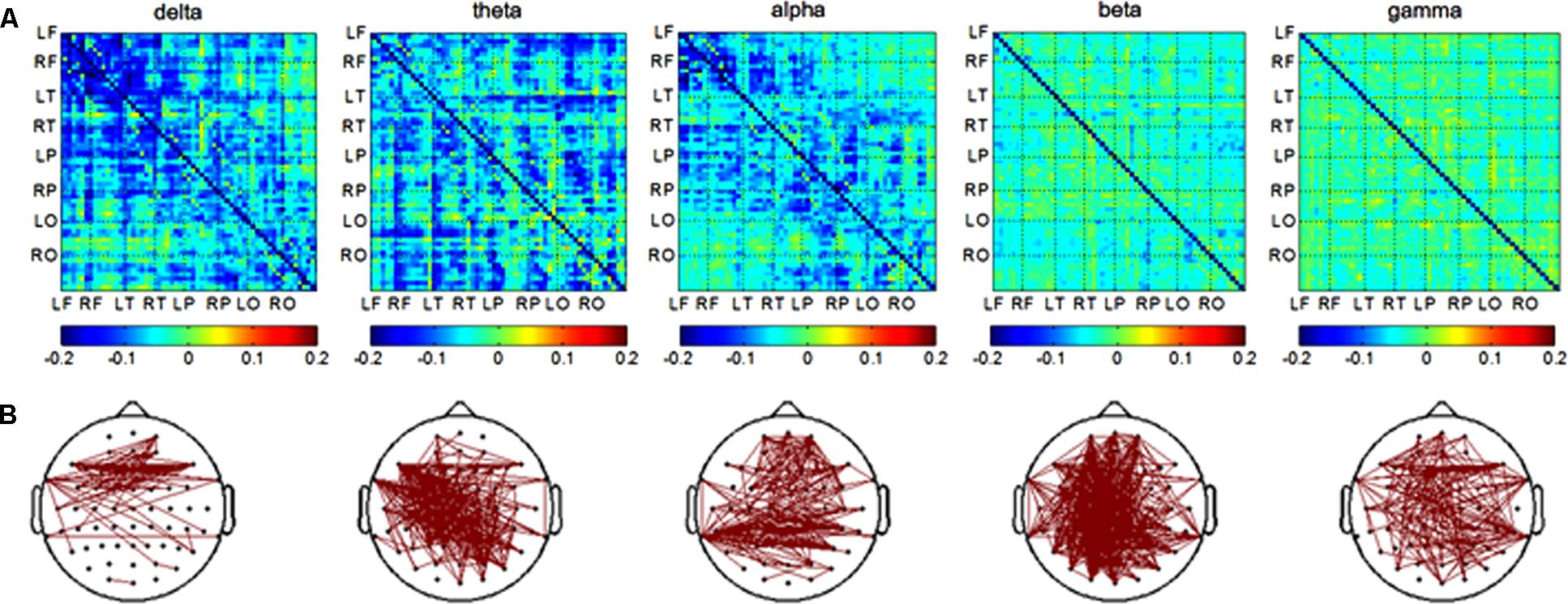
FIGURE 3. Functional connectivity in encoding session. (A) Difference of PLI value between two groups among eight brain areas (LF, RF, LT, RT, LP, RP, LO, RO) in five frequency band. NC < AD; (B) the PLI value of channels-pairs that had significantly difference between two groups in five frequency band.
In the retrieval session, differences in channel pairs PLI value between the AD and NC groups among 8 brain areas were plotted in Figure 4A. The PLI value was higher in the AD group compared to that in the NC group. The PLI value of channel pairs had significant differences between groups (Figure 4B). The number of different channel pairs were 80 (delta), 353 (theta), 109 (alpha), 112 (beta), and 46 (gamma). Combined, the results of Figures 4A,B show that the most obvious differences in functional connectivity between different brain regions were in the parietal, temporal and occipital regions of both hemispheres in the theta frequency band, and in the frontal and temporal of both hemispheres in the delta band.
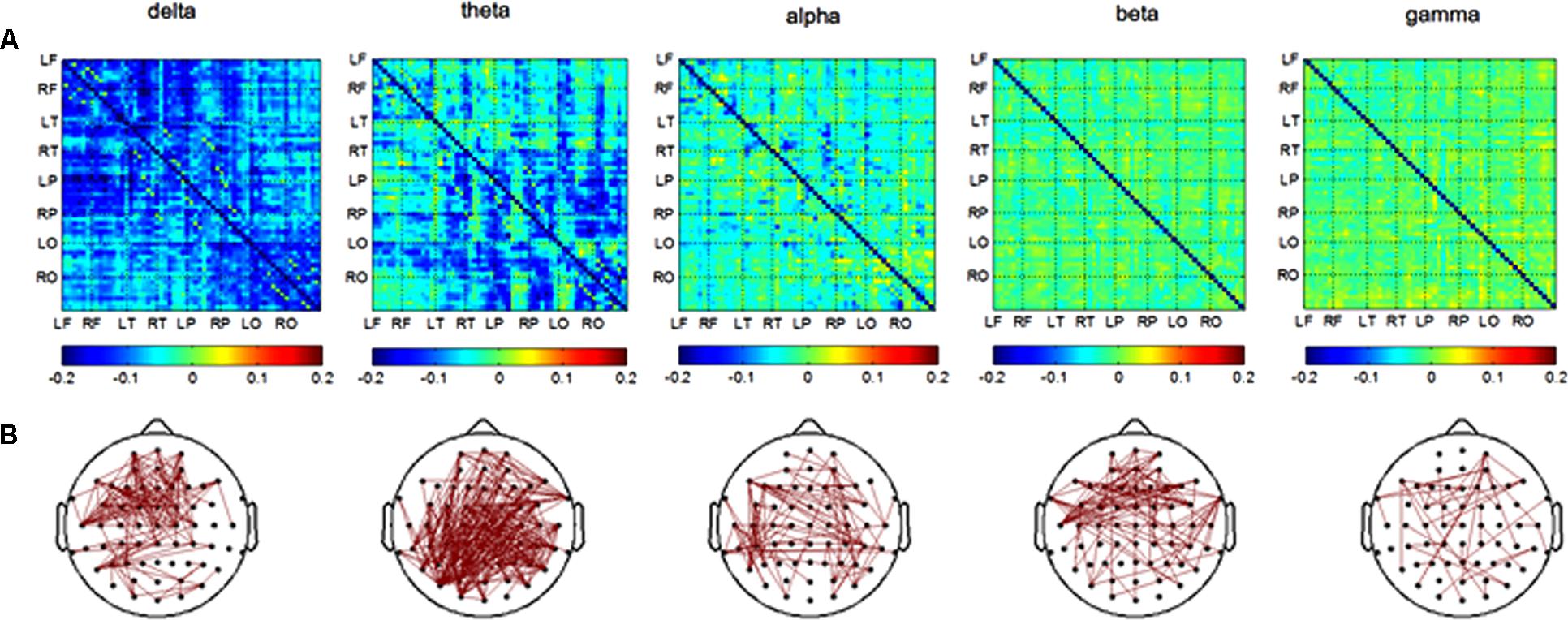
FIGURE 4. Functional connectivity in retrieval session. (A) Difference of PLI value between two groups among eight brain areas (LF, RF, LT, RT, LP, RP, LO, RO) in five frequency band. NC < AD; (B) the PLI value of channels-pairs that had significantly difference between two groups in five frequency band.
Discussion
In our study, higher spectral power was found in alpha band frequencies in the AD group than in the healthy control group during object-location memory encoding. Furthermore, higher spectral power in the theta and alpha band frequencies was also found during memory retrieval. We also found that strengths of connectivity were obviously increased in the AD group when compared to the healthy control group prominent in delta, theta, and alpha band frequencies in memory encoding and delta and theta band frequencies in memory retrieval. The most obvious difference in functional connectivity during memory encoding was between the frontal and parietal in the alpha band and frontal and temporal in the delta band. During memory retrieval, the most obvious difference in functional connectivity was between the parietal, temporal, and occipital regions in the theta frequency band and between the frontal and temporal in the delta band. The significant differences between AD patients and healthy elders in our object-location memory task were in slow EEG oscillations. One possibility would be that slow oscillations modulate the fast at a higher level, which determine the general mode of processing (Knyazev, 2012).
Encoding Stage of Object-Location Memory
At the encoding stage, the strength of functional connectivity in the theta band of AD patients was higher than that of healthy elders, which may indicate that cognitive function correlated with theta oscillations was impaired in AD patients. Theta rhythms are considered to the signs of taking and encoding new information (Colgin, 2013). Theta oscillations demonstrated the process in hippocampo-cortical feedback loops (Klimesch, 1999, 2012). The crucial role of theta rhythms in memory and spatial and temporal organization is well-investigated (Buzsaki, 2002). Studies in rats have demonstrated increase of mPFC-MTL theta phase in spatial memory tasks (Benchenane et al., 2010; Euston et al., 2012). In a study involving the hippocampus and the mPFC, the proportion of mPFC neurons that were phase-locked to hippocampal theta oscillations increased after successful learning of an object-place association (Kim et al., 2011). Since our study indicated a higher strength of functional connectivity in the theta band for AD patients compared to healthy elders, we hypothesize that the AD patients may use neural compensation to encode object spatial information.
The neural compensation demonstrated that brain actively attempts to recruit alternative structures which are not normally used to compensate for brain damage (Stern, 2009, 2012, 2013; Risacher and Saykin, 2013). Thus, brain size is not linearly correlated with brain function. Several studies have found that the neurodegenerative process of abnormal cortical oscillations in AD is accompanied by synaptic compensation mechanisms that are regarded to play a role in preventing the catastrophic amnesia associated with synaptic loss and maintaining excitement of neural circuits (Small, 2004; Turrigiano, 2011, 2012; Abuhassan et al., 2014).
A series of studies explored the concept of neural compensation either in elders, mild cognitive impairment (MCI), or AD patients (Rypma and D’Esposito, 1999; Cabeza et al., 2002; Zarahn et al., 2007; Steffener et al., 2009; Bajo et al., 2010). Deactivating local compensation will lead to rapid decline (cognitive deficit) of network dynamics in the theta and alpha bands (Moretti, 2015). Previous functional neuroimaging and physiological studies have reported several compensatory features such as the increase of activity in the MTL, the plasticity of the cholinergic system and regional cerebral blood flow (DeKosky et al., 2002; Dickerson et al., 2005; Dai et al., 2009). The method of event related desynchronization/synchronization (ERD/ERS), using auditory verbal memory task, was also indicated as a compensatory mechanism in MCI and AD (Karrasch et al., 2006).
In our study, the alpha band frequency power and strengths of functional connectivity of AD patients is higher during the encoding stage of object-location memory task compared to healthy elders. Alpha oscillations relate to a lot of cognitive domains such as perception, encoding, and recognition, which are guided by attention (Friston, 2009; Summerfield and Egner, 2009). Those processes are closely related to access of information in the knowledge system, which comprise of traditional long-term memory, procedural and implicit-perceptual knowledge (Klimesch, 1999, 2012). In addition, alpha activity plays an important role in attention by supporting processes within the attentional focus and inhibiting task-irrelevant memory entries (Sauseng et al., 2010; Benedek et al., 2014), and also correlation with maintenance of sensory representations (VanRullen and Macdonald, 2012). Brain activities during encoding and retrieval memory tasks involve a number of processes, including increased attention, use of elaborative strategies, and the formation of item-to-context associations. In order to complete the specific task in our study, all subjects need to combine their spatial attention and object-based attention, bind object to location, and maintain the information in their minds. Compared with the healthy elderly subjects, AD patients had to use other compensatory mechanisms for those process, thus having a higher alpha band spectral power.
In encoding sessions, the strength of functional connectivity in the delta band of AD patients was higher than that of healthy elders, indicating that the function correlated with delta oscillations is impaired in AD patients as well. While the role of delta frequency oscillations is still being debated, mounting evidence indicates that delta-band oscillations are mostly associated with old evolutionary basic motivational processes (Knyazev, 2012). Several studies considered delta as a ‘cognitive’ rhythm, such as Knyazev suggested that the motivational relevance of the task and the salience of the target stimulus were involved in enhanced delta activity (Knyazev, 2007). The cortical delta oscillations has been found as a mechanism in selective attention to rhythmic auditory or visual stimulus streams (Lakatos et al., 2008; Knyazev, 2012; Harmony, 2013). In our paradigm, in order to encode the item and bind location context successfully, subjects might pay attention to screening of picture representation and be involved in stimuli in search of motivationally salient cues that benefit for memory, which processing may reflect by delta oscillations. Therefore, our results may suggest AD patients recruit more neural network for motivation and attention.
Retrieving Stage of Object-Location Memory
In our study, the higher spectral power and strengths of functional connectivity in theta band were found in AD patients while retrieving the object-location information. The most prominent differences in functional connectivity between different brain regions were between the parietal, temporal, and occipital of the two hemispheres. As previously mentioned, in the encoding stage, theta rhythm is associated with mnemonic function. Previous studies have also proved that recollection of contextual information is associated with increased theta power and phase synchronization (Guderian and Duzel, 2005; Fuentemilla et al., 2014). The functional connectivity between segregated brain regions in the theta frequency is also crucial to memory recall (Klimesch et al., 2010; Colgin, 2013). This indicated that AD patients might recruit compensatory resources for the hippcampo-cortical network.
For the hippocampus is crucial for recollection episode of location, cortical theta oscillations links hippocampal functioning for recollection. During recollection episode of location, theta oscillations might be the dynamic link between hippocampal and neocortical areas (Guderian and Duzel, 2005). Consistent with the hippocampus’s key role in spatial memory processing, several studies have demonstrated links between interregional theta coupling and performance on a variety of spatial memory tasks (Colgin, 2013).
Functional Connectivity Destruction in AD
In our study the most prominent functional connectivity differences between AD and controls were found in the delta, theta, and alpha bands in the encoding session and delta and theta bands in the retrieval session. The findings in spectral power and synchronization values seem not to be closely related to each other. Differences in synchronization between the controls and AD patients were found to be more conspicuous than those seen for the spectral measures. This is not surprising, since spectral power and synchronization attributes reflect different aspects of the EEG, and they are mathematically independent. As the underlying neurophysiological mechanisms of the findings related to the differences between the controls and AD patients are not clear, the two types of analyses may reflect different, perhaps complementary pathophysiological aspects (Hidasi et al., 2007). Distributed networks of brain regions, which are directly connected by anatomical tracts or by functional associations and with a time-varying dynamic and hierarchy, were engaged in the human brain functions (Vecchio et al., 2014). As multimodal information processing at the level of cortico-cortical projections are affected in AD, a hypothetical model of “disconnection syndrome” for AD symptomatology was suggested (Delbeuck et al., 2003). Abnormalities in functional connection between brain cortical regions were found in AD in studies using EEG, functional magnetic resonance image (fMRI), Positron emission tomography (PET), magnetoencephalographic (MEG) (Stam et al., 2006; Liu et al., 2014; Vecchio et al., 2014; Dai et al., 2015; Engels et al., 2015; Romero-Garcia et al., 2016).
The most prominent impairment in brain networks of AD were found in long distance connections, and the degrees of impairment were related to cognitive decline (Dai et al., 2015). However, these studies focused on the resting state of the brain network, which is different from the brain network associated with cognitive tasks. Our study investigated the brain networks associated with object-location memory and obviously, our results showed that the more differences of functional connections between two groups were also long distance connections which were presented in Figures 3, 4. This result maybe indicated that AD patients used compensational long distance network to complete object-location memory when their long-distance connections were destructed. The increased inter-hemispheric functional connectivity related to memory networks were also found in MCI patients, and the researchers believed that the results could reflect a compensatory mechanism (Bajo et al., 2010). The alterations of inter-hemispheric connections might result from axonal degeneration in anterior and posterior regions of the corpus callosum in AD patients (Di Paola et al., 2010; Wang et al., 2014; Romero-Garcia et al., 2016). Moreover, the increased functional connectivity association with visual sensory and cognitive stimulation, which reflected by the increased coherence values in gamma band, were found in AD patients compared to healthy elders (Basar et al., 2017). On the other hand, some previous studies showed decrease of coherence values in AD patients compared to healthy controls during cognition task (Guntekin et al., 2008; Basar et al., 2010; Yener and Basar, 2013). These previous literatures are highly controversial. The discrepancies could be due to differences of recoding state, recoding techniques, and analysis methods (Basar et al., 2017).
Since methods of functional connectivity were more conspicuous to changes in electrophysiological characteristics of interneuronal connectivity than spectral power (Stevens et al., 2001; Adler et al., 2003; Pogarell et al., 2005), functional connectivity may become a sensitivity biomarker for early detection of AD and contribute to finding the mechanism of nerve injury.
Limitations
Because the number of participants was relatively low, one of our limitations is that only preliminary conclusions can be drawn. The difficulty of performing a given task may limit the number of individuals involved in such research. These difficulties may be the reason why there is no simple and commonly recognized approach to assess the spatial memory of AD patients. As a result, we only analysis the item location in left and successful recall subsequently, the right/left location was primitive one of spatial context. And the participant subjects were merely mild AD patients. In order to facilitate operator response, we only use two buttons for each subject. However, this paradigm may lead to subjects using a different memory strategy than subjects using three buttons.
Conclusion
Our results indicate that the function of object-location memory in AD patients is significantly impaired when compared to healthy elders. In the AD group, there was higher spectral power in the alpha band frequency during memory encoding, and the theta and alpha bands during memory retrieval. Strengths of connectivity were clearly increased in the AD group when compared to the healthy control group prominent in theta, alpha, and beta band frequencies in memory encoding and theta band frequency in memory retrieval. Our findings provide support for the hypothesis that AD patients may use compensation mechanism to memory items successfully.
Author Contributions
YH, JJ, and WW conceived the study and coordinated the experiments. YH and KW performed the experiments and analyzed the data. YH wrote the manuscript. JJ and WW revised the manuscript. All authors read and approved the final manuscript.
Funding
This work was supported by Chinese Military Key Medical Research Project (Project No. AWS12J004).
Conflict of Interest Statement
The authors declare that the research was conducted in the absence of any commercial or financial relationships that could be construed as a potential conflict of interest.
Acknowledgments
We thank Yufang Yang (Institute of Psychology, CAS) for providing the object pictures which were normative measured in Chinese by Yufang Yang, thank Jing Wang (Department of Neurobiology, Capital Medical University) for suggestion to experimental design and EEG technical support.
Footnotes
References
Abuhassan, K., Coyle, D., Belatreche, A., and Maguire, L. (2014). Compensating for synaptic loss in Alzheimer’s disease. J. Comput. Neurosci. 36, 19–37. doi: 10.1007/s10827-013-0462-8
Adler, G., Brassen, S., and Jajcevic, A. (2003). EEG coherence in Alzheimer’s dementia. J. Neural Transm. 110, 1051–1058. doi: 10.1007/s00702-003-0024-8
Bajo, R., Maestu, F., Nevado, A., Sancho, M., Gutierrez, R., Campo, P., et al. (2010). Functional connectivity in mild cognitive impairment during a memory task: implications for the disconnection hypothesis. J. Alzheimers Dis. 22, 183–193. doi: 10.3233/JAD-2010-100177
Basar, E., Femir, B., Emek-Savas, D. D., Guntekin, B., and Yener, G. G. (2017). Increased long distance event-related gamma band connectivity in Alzheimer’s disease. Neuroimage Clin. 14, 580–590. doi: 10.1016/j.nicl.2017.02.021
Basar, E., Guntekin, B., Tulay, E., and Yener, G. G. (2010). Evoked and event related coherence of Alzheimer patients manifest differentiation of sensory-cognitive networks. Brain Res. 1357, 79–90. doi: 10.1016/j.brainres.2010.08.054
Benchenane, K., Peyrache, A., Khamassi, M., Tierney, P. L., Gioanni, Y., Battaglia, F. P., et al. (2010). Coherent theta oscillations and reorganization of spike timing in the hippocampal- prefrontal network upon learning. Neuron 66, 921–936. doi: 10.1016/j.neuron.2010.05.013
Benedek, M., Schickel, R. J., Jauk, E., Fink, A., and Neubauer, A. C. (2014). Alpha power increases in right parietal cortex reflects focused internal attention. Neuropsychologia 56, 393–400. doi: 10.1016/j.neuropsychologia.2014.02.010
Bennys, K., Rondouin, G., Vergnes, C., and Touchon, J. (2001). Diagnostic value of quantitative EEG in Alzheimer’s disease. Neurophysiol. Clin. 31, 153–160. doi: 10.1016/S0987-7053(01)00254-4
Bird, C. M., Chan, D., Hartley, T., Pijnenburg, Y. A., Rossor, M. N., and Burgess, N. (2010). Topographical short-term memory differentiates Alzheimer’s disease from frontotemporal lobar degeneration. Hippocampus 20, 1154–1169. doi: 10.1002/hipo.20715
Bucks, R. S., Ashworth, D. L., Wilcock, G. K., and Siegfried, K. (1996). Assessment of activities of daily living in dementia: development of the Bristol Activities of Daily Living Scale. Age. Ageing 25, 113–120. doi: 10.1093/ageing/25.2.113
Buzsaki, G. (2002). Theta oscillations in the hippocampus. Neuron 33, 325–340. doi: 10.1016/S0896-6273(02)00586-X
Cabeza, R., Dolcos, F., Graham, R., and Nyberg, L. (2002). Similarities and differences in the neural correlates of episodic memory retrieval and working memory. Neuroimage 16, 317–330. doi: 10.1006/nimg.2002.1063
Cherrier, M. M., Mendez, M., and Perryman, K. (2001). Route learning performance in Alzheimer disease patients. Neuropsychiatry Neuropsychol. Behav. Neurol. 14, 159–168.
Colgin, L. L. (2013). Mechanisms and functions of theta rhythms. Annu. Rev. Neurosci. 36, 295–312. doi: 10.1146/annurev-neuro-062012-170330
Cummings, J. L., Mega, M., Gray, K., Rosenberg-Thompson, S., Carusi, D. A., and Gornbein, J. (1994). The neuropsychiatric inventory: comprehensive assessment of psychopathology in dementia. Neurology 44, 2308–2314. doi: 10.1212/WNL.44.12.2308
Cycowicz, Y. M., Friedman, D., Rothstein, M., and Snodgrass, J. G. (1997). Picture naming by young children: norms for name agreement, familiarity, and visual complexity. J. Exp. Child Psychol. 65, 171–237. doi: 10.1006/jecp.1996.2356
Cycowicz, Y. M., Friedman, D., and Snodgrass, J. G. (2001). Remembering the color of objects: an ERP investigation of source memory. Cereb. Cortex 11, 322–334. doi: 10.1093/cercor/11.4.322
Cycowicz, Y. M., Nessler, D., Horton, C., and Friedman, D. (2008). Retrieving object color: the influence of color congruity and test format. Neuroreport 19, 1387–1390. doi: 10.1097/WNR.0b013e32830c8df1
Dai, W., Lopez, O. L., Carmichael, O. T., Becker, J. T., Kuller, L. H., and Gach, H. M. (2009). Mild cognitive impairment and alzheimer disease: patterns of altered cerebral blood flow at MR imaging. Radiology 250, 856–866. doi: 10.1148/radiol.2503080751
Dai, Z., Yan, C., Li, K., Wang, Z., Wang, J., Cao, M., et al. (2015). Identifying and mapping connectivity patterns of brain network hubs in Alzheimer’s disease. Cereb. Cortex 25, 3723–3742. doi: 10.1093/cercor/bhu246
deIpolyi, A. R., Rankin, K. P., Mucke, L., Miller, B. L., and Gorno-Tempini, M. L. (2007). Spatial cognition and the human navigation network in AD and MCI. Neurology 69, 986–997. doi: 10.1212/01.wnl.0000271376.19515.c6
DeKosky, S. T., Ikonomovic, M. D., Styren, S. D., Beckett, L., Wisniewski, S., Bennett, D. A., et al. (2002). Upregulation of choline acetyltransferase activity in hippocampus and frontal cortex of elderly subjects with mild cognitive impairment. Ann. Neurol. 51, 145–155. doi: 10.1002/ana.10069
Delbeuck, X., Van der Linden, M., and Collette, F. (2003). Alzheimer’s disease as a disconnection syndrome? Neuropsychol. Rev. 13, 79–92.
Di Paola, M., Spalletta, G., and Caltagirone, C. (2010). In vivo structural neuroanatomy of corpus callosum in Alzheimer’s disease and mild cognitive impairment using different MRI techniques: a review. J. Alzheimers Dis. 20, 67–95. doi: 10.3233/JAD-2010-1370
Dickerson, B. C., Salat, D. H., Greve, D. N., Chua, E. F., Rand-Giovannetti, E., Rentz, D. M., et al. (2005). Increased hippocampal activation in mild cognitive impairment compared to normal aging and AD. Neurology 65, 404–411. doi: 10.1212/01.wnl.0000171450.97464.49
Edler, D., Bestgen, A. K., Kuchinke, L., and Dickmann, F. (2015). True-3D accentuating of grids and streets in urban topographic maps enhances human object location memory. PLoS ONE 10:e0116959. doi: 10.1371/journal.pone.0116959
Engels, M. M., Stam, C. J., van der Flier, W. M., Scheltens, P., de Waal, H., and van Straaten, E. C. (2015). Declining functional connectivity and changing hub locations in Alzheimer’s disease: an EEG study. BMC Neurol. 15:145. doi: 10.1186/s12883-015-0400-07
Euston, D. R., Gruber, A. J., and McNaughton, B. L. (2012). The role of medial prefrontal cortex in memory and decision making. Neuron 76, 1057–1070. doi: 10.1016/j.neuron.2012.12.002
Folstein, M. F., Folstein, S. E., and McHugh, P. R. (1975). Mini-mental state. A practical method for grading the cognitive state of patients for the clinician. J. Psychiatr. Res. 12, 189–198. doi: 10.1016/0022-3956(75)90026-6
Friston, K. (2009). The free-energy principle: a rough guide to the brain? Trends Cogn. Sci. 13, 293–301. doi: 10.1016/j.tics.2009.04.005
Fuentemilla, L., Barnes, G. R., Duzel, E., and Levine, B. (2014). Theta oscillations orchestrate medial temporal lobe and neocortex in remembering autobiographical memories. Neuroimage 85(Pt 2), 730–737. doi: 10.1016/j.neuroimage.2013.08.029
Furtak, S. C., Ahmed, O. J., and Burwell, R. D. (2012). Single neuron activity and theta modulation in postrhinal cortex during visual object discrimination. Neuron 76, 976–988. doi: 10.1016/j.neuron.2012.10.039
Guderian, S., and Duzel, E. (2005). Induced theta oscillations mediate large-scale synchrony with mediotemporal areas during recollection in humans. Hippocampus 15, 901–912. doi: 10.1002/hipo.20125
Guntekin, B., Saatci, E., and Yener, G. (2008). Decrease of evoked delta, theta and alpha coherences in Alzheimer patients during a visual oddball paradigm. Brain Res. 1235, 109–116. doi: 10.1016/j.brainres.2008.06.028
Hanslmayr, S., and Staudigl, T. (2014). How brain oscillations form memories–a processing based perspective on oscillatory subsequent memory effects. Neuroimage 85(Pt 2), 648–655. doi: 10.1016/j.neuroimage.2013.05.121
Hanslmayr, S., Staudigl, T., and Fellner, M. C. (2012). Oscillatory power decreases and long-term memory: the information via desynchronization hypothesis. Front. Hum. Neurosci. 6:74. doi: 10.3389/fnhum.2012.00074
Harmony, T. (2013). The functional significance of delta oscillations in cognitive processing. Front. Integr. Neurosci. 7:83. doi: 10.3389/fnint.2013.00083
Hartley, T., Bird, C. M., Chan, D., Cipolotti, L., Husain, M., Vargha-Khadem, F., et al. (2007). The hippocampus is required for short-term topographical memory in humans. Hippocampus 17, 34–48. doi: 10.1002/hipo.20240
Hidasi, Z., Czigler, B., Salacz, P., Csibri, E., and Molnar, M. (2007). Changes of EEG spectra and coherence following performance in a cognitive task in Alzheimer’s disease. Int. J. Psychophysiol. 65, 252–260. doi: 10.1016/j.ijpsycho.2007.05.002
Hughes, C. P., Berg, L., Danziger, W. L., Coben, L. A., and Martin, R. L. (1982). A new clinical scale for the staging of dementia. Br. J. Psychiatry 140, 566–572. doi: 10.1192/bjp.140.6.566
Iaria, G., Chen, J. K., Guariglia, C., Ptito, A., and Petrides, M. (2007). Retrosplenial and hippocampal brain regions in human navigation: complementary functional contributions to the formation and use of cognitive maps. Eur. J. Neurosci. 25, 890–899. doi: 10.1111/j.1460-9568.2007.05371.x
Karrasch, M., Laine, M., Rinne, J. O., Rapinoja, P., Sinerva, E., and Krause, C. M. (2006). Brain oscillatory responses to an auditory-verbal working memory task in mild cognitive impairment and Alzheimer’s disease. Int. J. Psychophysiol. 59, 168–178. doi: 10.1016/j.ijpsycho.2005.04.006
Kessels, R. P., Jaap Kappelle, L., de Haan, E. H., and Postma, A. (2002). Lateralization of spatial-memory processes: evidence on spatial span, maze learning, and memory for object locations. Neuropsychologia 40, 1465–1473. doi: 10.1016/S0028-3932(01)00199-3
Kessels, R. P., Postma, A., Wester, A. J., and de Haan, E. H. (2000). Memory for object locations in Korsakoff’s amnesia. Cortex 36, 47–57. doi: 10.1016/S0010-9452(08)70835-9
Kessels, R. P., Rijken, S., Joosten-Weyn Banningh, L. W., Van Schuylenborgh, V. A. N. E. N., and Olde Rikkert, M. G. (2010). Categorical spatial memory in patients with mild cognitive impairment and Alzheimer dementia: positional versus object-location recall. J. Int. Neuropsychol. Soc. 16, 200–204. doi: 10.1017/S1355617709990944
Kim, J., Delcasso, S., and Lee, I. (2011). Neural correlates of object-in-place learning in hippocampus and prefrontal cortex. J. Neurosci. 31, 16991–17006. doi: 10.1523/JNEUROSCI.2859-11.2011
Klem, G. H., Luders, H. O., Jasper, H. H., and Elger, C. (1999). The ten-twenty electrode system of the international federation. The international federation of clinical neurophysiology. Electroencephalogr. Clin. Neurophysiol. Suppl. 52, 3–6.
Klimesch, W. (1999). EEG alpha and theta oscillations reflect cognitive and memory performance: a review and analysis. Brain Res. Brain Res. Rev. 29, 169–195. doi: 10.1016/S0165-0173(98)00056-3
Klimesch, W. (2012). Alpha-band oscillations, attention, and controlled access to stored information. Trends Cogn. Sci. 16, 606–617. doi: 10.1016/j.tics.2012.10.007
Klimesch, W., Freunberger, R., and Sauseng, P. (2010). Oscillatory mechanisms of process binding in memory. Neurosci. Biobehav. Rev. 34, 1002–1014. doi: 10.1016/j.neubiorev.2009.10.004
Knott, V., Mohr, E., Mahoney, C., and Ilivitsky, V. (2000). Electroencephalographic coherence in Alzheimer’s disease: comparisons with a control group and population norms. J. Geriatr. Psychiatry Neurol. 13, 1–8. doi: 10.1177/089198870001300101
Knyazev, G. G. (2007). Motivation, emotion, and their inhibitory control mirrored in brain oscillations. Neurosci. Biobehav. Rev. 31, 377–395. doi: 10.1016/j.neubiorev.2006.10.004
Knyazev, G. G. (2012). EEG delta oscillations as a correlate of basic homeostatic and motivational processes. Neurosci. Biobehav. Rev. 36, 677–695. doi: 10.1016/j.neubiorev.2011.10.002
Kulzow, N., Kerti, L., Witte, V. A., Kopp, U., Breitenstein, C., and Floel, A. (2014). An object location memory paradigm for older adults with and without mild cognitive impairment. J. Neurosci. Methods 237, 16–25. doi: 10.1016/j.jneumeth.2014.08.020
Lakatos, P., Karmos, G., Mehta, A. D., Ulbert, I., and Schroeder, C. E. (2008). Entrainment of neuronal oscillations as a mechanism of attentional selection. Science 320, 110–113. doi: 10.1126/science.1154735
Lange, J., Pavlidou, A., and Schnitzler, A. (2015). Lateralized modulation of beta-band power in sensorimotor areas during action observation. Front. Integr. Neurosci. 9:43. doi: 10.3389/fnint.2015.00043
Lee, Y. Y., and Yang, C. Y. (2014). Utilizing the extent of theta-gamma synchronization to estimate visuospatial memory ability. Australas. Phys. Eng. Sci. Med. 37, 665–672. doi: 10.1007/s13246-014-0299-0
Leocani, L., and Comi, G. (1999). EEG coherence in pathological conditions. J. Clin. Neurophysiol. 16, 548–555. doi: 10.1097/00004691-199911000-00006
Liu, Y., Yu, C., Zhang, X., Liu, J., Duan, Y., Alexander-Bloch, A. F., et al. (2014). Impaired long distance functional connectivity and weighted network architecture in Alzheimer’s disease. Cereb. Cortex 24, 1422–1435. doi: 10.1093/cercor/bhs410
Locatelli, T., Cursi, M., Liberati, D., Franceschi, M., and Comi, G. (1998). EEG coherence in Alzheimer’s disease. Electroencephalogr. Clin. Neurophysiol. 106, 229–237. doi: 10.1016/S0013-4694(97)00129-6
Lu, C. B., Jefferys, J. G., Toescu, E. C., and Vreugdenhil, M. (2011). In vitro hippocampal gamma oscillation power as an index of in vivo CA3 gamma oscillation strength and spatial reference memory. Neurobiol. Learn. Mem. 95, 221–230. doi: 10.1016/j.nlm.2010.11.008
Maris, E., and Oostenveld, R. (2007). Nonparametric statistical testing of EEG- and MEG-data. J. Neurosci. Methods 164, 177–190. doi: 10.1016/j.jneumeth.2007.03.024
Mazurek, A., Bhoopathy, R. M., Read, J. C., Gallagher, P., and Smulders, T. V. (2015). Effects of age on a real-world What-Where-When memory task. Front. Aging Neurosci. 7:74. doi: 10.3389/fnagi.2015.00074
McKhann, G. M., Knopman, D. S., Chertkow, H., Hyman, B. T., Jack, C. R. Jr., Kawas, C. H., et al. (2011). The diagnosis of dementia due to Alzheimer’s disease: recommendations from the National Institute on Aging-Alzheimer’s Association workgroups on diagnostic guidelines for Alzheimer’s disease. Alzheimers Dement. 7, 263–269. doi: 10.1016/j.jalz.2011.03.005
Moodley, K., Minati, L., Contarino, V., Prioni, S., Wood, R., Cooper, R., et al. (2015). Diagnostic differentiation of mild cognitive impairment due to Alzheimer’s disease using a hippocampus-dependent test of spatial memory. Hippocampus 25, 939–951. doi: 10.1002/hipo.22417
Moretti, D. V. (2015). Theta and alpha EEG frequency interplay in subjects with mild cognitive impairment: evidence from EEG, MRI, and SPECT brain modifications. Front. Aging Neurosci. 7:31. doi: 10.3389/fnagi.2015.00031
Morris, J. C. (1993). The clinical dementia rating (CDR): current version and scoring rules. Neurology 43, 2412–2414. doi: 10.1212/WNL.43.11.2412-a
Nyhus, E., and Curran, T. (2010). Functional role of gamma and theta oscillations in episodic memory. Neurosci. Biobehav. Rev. 34, 1023–1035. doi: 10.1016/j.neubiorev.2009.12.014
Oostenveld, R., Fries, P., Maris, E., and Schoffelen, J. M. (2011). FieldTrip: Open source software for advanced analysis of MEG, EEG, and invasive electrophysiological data. Comput. Intell. Neurosci. 2011:156869. doi: 10.1155/2011/156869
Park, J., Lee, H., Kim, T., Park, G. Y., Lee, E. M., Baek, S., et al. (2014). Role of low- and high-frequency oscillations in the human hippocampus for encoding environmental novelty during a spatial navigation task. Hippocampus 24, 1341–1352. doi: 10.1002/hipo.22315
Parra, M. A., Abrahams, S., Logie, R. H., Mendez, L. G., Lopera, F., and Della Sala, S. (2010). Visual short-term memory binding deficits in familial Alzheimer’s disease. Brain 133, 2702–2713. doi: 10.1093/brain/awq148
Pertzov, Y., Dong, M. Y., Peich, M. C., and Husain, M. (2012). Forgetting what was where: the fragility of object-location binding. PLoS ONE 7:e48214. doi: 10.1371/journal.pone.0048214
Pertzov, Y., Heider, M., Liang, Y., and Husain, M. (2015). Effects of healthy ageing on precision and binding of object location in visual short term memory. Psychol. Aging 30, 26–35. doi: 10.1037/a0038396
Pertzov, Y., Miller, T. D., Gorgoraptis, N., Caine, D., Schott, J. M., Butler, C., et al. (2013). Binding deficits in memory following medial temporal lobe damage in patients with voltage-gated potassium channel complex antibody-associated limbic encephalitis. Brain 136(Pt 8), 2474–2485. doi: 10.1093/brain/awt129
Pogarell, O., Teipel, S. J., Juckel, G., Gootjes, L., Moller, T., Burger, K., et al. (2005). EEG coherence reflects regional corpus callosum area in Alzheimer’s disease. J. Neurol. Neurosurg. Psychiatry 76, 109–111. doi: 10.1136/jnnp.2004.036566
Postma, A., and De Haan, E. H. (1996). What was where? Memory for object locations. Q. J. Exp. Psychol. A 49, 178–199. doi: 10.1080/713755605
Risacher, S. L., and Saykin, A. J. (2013). Neuroimaging and other biomarkers for Alzheimer’s disease: the changing landscape of early detection. Annu. Rev. Clin. Psychol. 9, 621–648. doi: 10.1146/annurev-clinpsy-050212-185535
Romero-Garcia, R., Atienza, M., and Cantero, J. L. (2016). Different scales of cortical organization are selectively targeted in the progression to Alzheimer’s disease. Int. J. Neural Syst. 26:1650003. doi: 10.1142/S0129065716500039
Rosen, W. G., Terry, R. D., Fuld, P. A., Katzman, R., and Peck, A. (1980). Pathological verification of ischemic score in differentiation of dementias. Ann. Neurol. 7, 486–488. doi: 10.1002/ana.410070516
Rypma, B., and D’Esposito, M. (1999). The roles of prefrontal brain regions in components of working memory: effects of memory load and individual differences. Proc. Natl. Acad. Sci. U.S.A. 96, 6558–6563. doi: 10.1073/pnas.96.11.6558
Sauseng, P., Griesmayr, B., Freunberger, R., and Klimesch, W. (2010). Control mechanisms in working memory: a possible function of EEG theta oscillations. Neurosci. Biobehav. Rev. 34, 1015–1022. doi: 10.1016/j.neubiorev.2009.12.006
Simon-Thomas, E. R., Brodsky, K., Willing, C., Sinha, R., and Knight, R. T. (2003). Distributed neural activity during object, spatial and integrated processing in humans. Brain Res. Cogn. Brain Res. 16, 457–467. doi: 10.1016/S0926-6410(03)00060-0
Small, D. H. (2004). Mechanisms of synaptic homeostasis in Alzheimer’s disease. Curr. Alzheimer Res. 1, 27–32. doi: 10.2174/1567205043480573
Snider, J., Plank, M., Lynch, G., Halgren, E., and Poizner, H. (2013). Human cortical theta during free exploration encodes space and predicts subsequent memory. J. Neurosci. 33, 15056–15068. doi: 10.1523/JNEUROSCI.0268-13.2013
Snodgrass, J. G., and Vanderwart, M. (1980). A standardized set of 260 pictures: norms for name agreement, image agreement, familiarity, and visual complexity. J. Exp. Psychol. Hum. Learn. 6, 174–215. doi: 10.1037/0278-7393.6.2.174
Stam, C. J., Jones, B. F., Manshanden, I., van Cappellen van Walsum, A. M., Montez, T., Verbunt, J. P., et al. (2006). Magnetoencephalographic evaluation of resting-state functional connectivity in Alzheimer’s disease. Neuroimage 32, 1335–1344. doi: 10.1016/j.neuroimage.2006.05.033
Stam, C. J., Nolte, G., and Daffertshofer, A. (2007). Phase lag index: assessment of functional connectivity from multi channel EEG and MEG with diminished bias from common sources. Hum. Brain Mapp. 28, 1178–1193. doi: 10.1002/hbm.20346
Stam, C. J., van der Made, Y., Pijnenburg, Y. A., and Scheltens, P. (2003). EEG synchronization in mild cognitive impairment and Alzheimer’s disease. Acta Neurol. Scand. 108, 90–96. doi: 10.1034/j.1600-0404.2003.02067.x
Steffener, J., Brickman, A. M., Rakitin, B. C., Gazes, Y., and Stern, Y. (2009). The impact of age-related changes on working memory functional activity. Brain Imaging Behav. 3, 142–153. doi: 10.1007/s11682-008-9056-x
Stern, Y. (2009). Cognitive reserve. Neuropsychologia 47, 2015–2028. doi: 10.1016/j.neuropsychologia.2009.03.004
Stern, Y. (2012). Cognitive reserve in ageing and Alzheimer’s disease. Lancet Neurol. 11, 1006–1012. doi: 10.1016/S1474-4422(12)70191-6
Stern, Y. (2013). Cognitive reserve: implications for assessment and intervention. Folia Phoniatr. Logop. 65, 49–54. doi: 10.1159/000353443
Stevens, A., Kircher, T., Nickola, M., Bartels, M., Rosellen, N., and Wormstall, H. (2001). Dynamic regulation of EEG power and coherence is lost early and globally in probable DAT. Eur. Arch. Psychiatry Clin. Neurosci. 251, 199–204. doi: 10.1007/s004060170027
Strauss, E., Sherman, E. M. S., and Spreen, O. (2006). A Compendium of Neuropsychological Tests: Administration, Norms, and Commentary. Oxford: Oxford University Press.
Summerfield, C., and Egner, T. (2009). Expectation (and attention) in visual cognition. Trends Cogn. Sci. 13, 403–409. doi: 10.1016/j.tics.2009.06.003
Turrigiano, G. (2011). Too many cooks? Intrinsic and synaptic homeostatic mechanisms in cortical circuit refinement. Annu. Rev. Neurosci. 34, 89–103. doi: 10.1146/annurev-neuro-060909-153238
Turrigiano, G. (2012). Homeostatic synaptic plasticity: local and global mechanisms for stabilizing neuronal function. Cold Spring Harb. Perspect. Biol. 4:a005736. doi: 10.1101/cshperspect.a005736
van Asselen, M., Kessels, R. P., Kappelle, L. J., and Postma, A. (2008). Categorical and coordinate spatial representations within object-location memory. Cortex 44, 249–256. doi: 10.1016/j.cortex.2006.05.005
van Hoogmoed, A. H., van den Brink, D., and Janzen, G. (2012). Electrophysiological correlates of object location and object identity processing in spatial scenes. PLoS ONE 7:e41180. doi: 10.1371/journal.pone.0041180
VanRullen, R., and Macdonald, J. S. (2012). Perceptual echoes at 10 Hz in the human brain. Curr. Biol. 22, 995–999. doi: 10.1016/j.cub.2012.03.050
Vecchio, F., Miraglia, F., Marra, C., Quaranta, D., Vita, M. G., Bramanti, P., et al. (2014). Human brain networks in cognitive decline: a graph theoretical analysis of cortical connectivity from EEG data. J. Alzheimers Dis. 41, 113–127. doi: 10.3233/JAD-132087
Wang, P. N., Chou, K. H., Chang, N. J., Lin, K. N., Chen, W. T., Lan, G. Y., et al. (2014). Callosal degeneration topographically correlated with cognitive function in amnestic mild cognitive impairment and Alzheimer’s disease dementia. Hum. Brain Mapp. 35, 1529–1543. doi: 10.1002/hbm.22271
Yener, G. G., and Basar, E. (2013). Biomarkers in Alzheimer’s disease with a special emphasis on event-related oscillatory responses. Suppl. Clin. Neurophysiol. 62, 237–273. doi: 10.1016/B978-0-7020-5307-8.00020-X
Zarahn, E., Rakitin, B., Abela, D., Flynn, J., and Stern, Y. (2007). Age-related changes in brain activation during a delayed item recognition task. Neurobiol. Aging 28, 784–798. doi: 10.1016/j.neurobiolaging.2006.03.002
Keywords: Alzheimer’s disease, object-location memory, electroencephalogram, power spectrum, functional connectivity, compensation mechanisms
Citation: Han Y, Wang K, Jia J and Wu W (2017) Changes of EEG Spectra and Functional Connectivity during an Object-Location Memory Task in Alzheimer’s Disease. Front. Behav. Neurosci. 11:107. doi: 10.3389/fnbeh.2017.00107
Received: 16 March 2017; Accepted: 18 May 2017;
Published: 31 May 2017.
Edited by:
Bahar Güntekin, Istanbul Medipol University, TurkeyReviewed by:
Hadj Boumediene Meziane, University of Lausanne, SwitzerlandRoberta Lizio, Sapienza Università di Roma, Italy
Copyright © 2017 Han, Wang, Jia and Wu. This is an open-access article distributed under the terms of the Creative Commons Attribution License (CC BY). The use, distribution or reproduction in other forums is permitted, provided the original author(s) or licensor are credited and that the original publication in this journal is cited, in accordance with accepted academic practice. No use, distribution or reproduction is permitted which does not comply with these terms.
*Correspondence: Weiping Wu, cGxhX25ldXJvQDEyNi5jb20= Jianjun Jia, amlhamlhbmp1bl8zMDFAMTI2LmNvbQ==