- 1ZENEREI Institute and The International Zebrafish Neuroscience Research Consortium, Slidell, LA, USA
- 2Department of Psychology, University of Toronto Mississauga, ON, Canada
- 3Research Institute for Marine Drugs and Nutrients, College of Food Science and Technology, Guangdong Ocean University, Zhanjiang, Guangdong, China
The high prevalence of brain disorders and the lack of their efficient treatments necessitate improved in-vivo pre-clinical models and tests. The zebrafish (Danio rerio), a vertebrate species with high genetic and physiological homology to humans, is an excellent organism for innovative central nervous system (CNS) drug discovery and small molecule screening. Here, we outline new strategies for developing higher-throughput zebrafish screens to test neuroactive drugs and predict their pharmacological mechanisms. With the growing application of automated 3D phenotyping, machine learning algorithms, movement pattern- and behavior recognition, and multi-animal video-tracking, zebrafish screens are expected to markedly improve CNS drug discovery.
Come on, feel the noise
So jump a little higher,
Jump, jump a little higher…
Scooter, “We Take You Higher” (1996)
Zebrafish Screens for CNS Drug Discovery
Brain disorders are complex multifaceted illnesses with poorly understood causes and frequently ineffective therapies (Duman et al., 1994; Nestler, 2013). Despite the growing public health impact of these disorders (Mitchell, 2011), the central nervous system (CNS) drugs have not improved in decades (WHO, 2008; Griebel and Holmes, 2013), necessitating novel pre-clinical in-vivo models for drug discovery (Markou et al., 2009; Stewart and Kalueff, 2013, 2014).
Research in this field is determined by the complex nature of CNS syndromes, the importance of targeting their biological mechanisms, and the need in high-throughput screens (HTS) for drug targets and potential therapies (Kokel and Peterson, 2008; Rihel et al., 2010; Laggner et al., 2012; Stewart and Kalueff, 2014). With the recent progress of medicinal chemistry, mathematical modeling and bioinformatics, drug discovery has started to embrace systematic, large-scale screening approaches (Bruni et al., 2014). As a vertebrate species with high genetic and physiological homology to humans, the zebrafish (Danio rerio) is rapidly emerging as an excellent model to address these needs (Kalueff et al., 2014a,b; Stewart et al., 2014a).
Importantly, using zebrafish as a first-choice vertebrate species for screening has several advantages. First, it helps narrow down the list of potential “hits” for their subsequent validation in more complex and expensive rodent tests. Second, it helps assess drug responses following various genetic manipulations, the toolbox for which is becoming increasingly diverse and efficient in zebrafish (Bernier et al., 2014; Kalueff et al., 2014b; Stewart et al., 2014a). Finally, larval and adult zebrafish screens are also useful for dissecting the drugs' psychopharmacological profiles (e.g., using multiple receptor agonists and antagonists prior to a more targeted rodent testing)—an approach that focuses on “core,” evolutionarily conserved (and, thus, translationally more relevant) molecular pathways shared by zebrafish and humans (Kalueff et al., 2014a,b; Stewart et al., 2014a).
Complementing larval zebrafish HTS, extensively used for modeling brain disorders and testing new compounds, adult zebrafish in-vivo testing is often performed as low-to-moderate neurophenotypic screens (Gerlai et al., 2000; Baraban et al., 2005; Kily et al., 2008; Maximino et al., 2010, 2011, 2013, 2014b; Mathur and Guo, 2011; Mathur et al., 2011a,b; Norton et al., 2011; Pan et al., 2011; Rosemberg et al., 2011; Griffiths et al., 2012; Lange et al., 2012a,b; Parker et al., 2013; Ziv et al., 2013; Stewart et al., 2014b; Li et al., 2015).
Current in-vivo zebrafish HTS typically utilize extensive or intensive approaches to generate “big data” for translational neuroscience research (Figure 1). For instance, applying a barcoding strategy (Glossary), extensive analyses of several basic locomotor and sleep/wake parameters in larval zebrafish have successfully identified neuroactive drugs from a large library of screened compounds (Kokel and Peterson, 2008, 2011; Kokel et al., 2010; Rihel et al., 2010; Laggner et al., 2012; Jin et al., 2013). Recent intensive studies analyzing 20–30 three-dimensional (3D) behavioral endpoints in adult zebrafish (Glossary, Supplementary Table 1S online), have detected potential commonalities and differences in profiles of several tested neuroactive drugs (Egan et al., 2009; Grossman et al., 2010; Wong et al., 2010; Cachat et al., 2011, 2013).
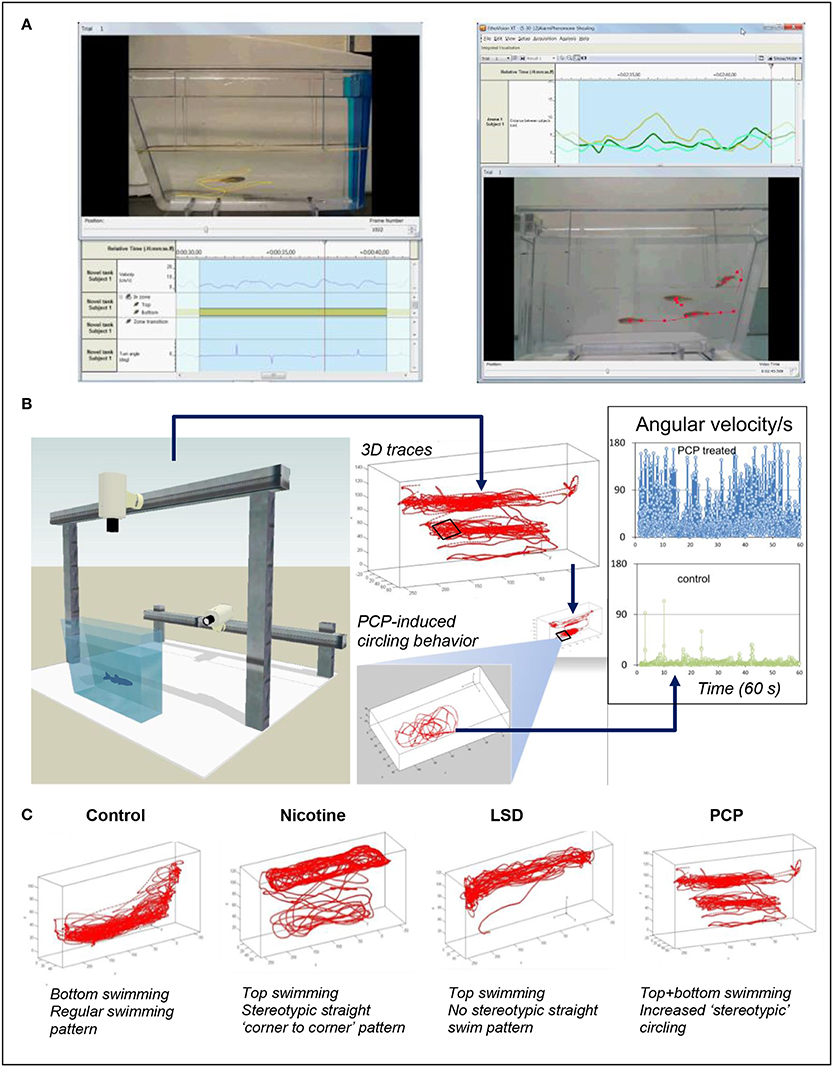
Figure 1. The use of video-tracking tools to assess neural phenotypes in zebrafish. (A) Shows video-tracking of an individual zebrafish (left) or a zebrafish group (shoal, right); side view vide-recording in the novel tank test. Tracking individual fish with one camera in 2D, or with two cameras in 3D, can generate up to 50–60 individual endpoints (see Supplementary Table 1S online for examples) which can be sensitive to neuroactive properties of the drugs. Tracing selected endpoints in zebrafish shoals, such as assessing the average inter-fish distance and velocity, is also possible in zebrafish models (Green et al., 2012) (although more sophisticated computer tools and optimized animal tagging methods are needed to monitor each individual fish within the group). (B) Illustrates the potential of 3D behavioral video-tracking in zebrafish to predict drug pharmacology (also see Soleymani et al., 2014). In this example, top swimming combined with elevated angular velocity in zebrafish treated with a hallucinogenic drug phencyclidine (PCP, inset) shows a striking difference from control fish, supporting the value of various computer-based neural phenotypes for predicting the pharmacological profile of different CNS-active compounds. (C) Shows examples of representative 3D phenotypes for control fish and animals acutely exposed to several CNS drugs. LSD, Lysergic acid diethylamide (images: courtesy of Noldus IT, Netherlands, in collaboration with the Kalueff Laboratory, Stewart et al., 2014a). Note distinct patterns of locomotion evoked by drugs from different pharmacological classes (also see Cachat et al., 2011, 2013; Soleymani et al., 2014 for discussion).
Figures 1, 2 further illustrate how a comprehensive evaluation of individual compounds can foster objective, computer-based prediction of the drugs' pharmacology. For example, phencyclidine (PCP) is a hallucinogenic glutamatergic antagonist that acutely evokes characteristic “top circling” behavior in zebrafish (Figures 1, 2). Assessed by elevated angular velocity and rotation index, this response is similar for anti-glutamatergic hallucinogens, but not other classes of hallucinogenic agents (Kyzar et al., 2012; Neelkantan et al., 2013; Stewart et al., 2013). Analyzing such 3D profiles, it is therefore possible to generate decision trees for predicting the pharmacological profile of different groups of neuroactive compounds, based on zebrafish swimming patterns and their geometry (Figure 2) (Cachat et al., 2010, 2011; Soleymani et al., 2014). Likewise, acute nicotine evokes characteristic top swimming along perimeter of the tank, strikingly differing from top circling (typical for many anti-glutamatergic drugs) or top surfacing without peripheral swimming (typical for serotonergic agents; Figure 1C) (Kyzar et al., 2012; Neelkantan et al., 2013; Stewart et al., 2013). Importantly, such accumulation of large libraries of drugs' behavioral signatures in zebrafish can parallel the application of machine learning algorithms, leading to further refinement and optimization of the prediction of drugs' pharmacological profiles (Soleymani et al., 2014) (Figure 2C).
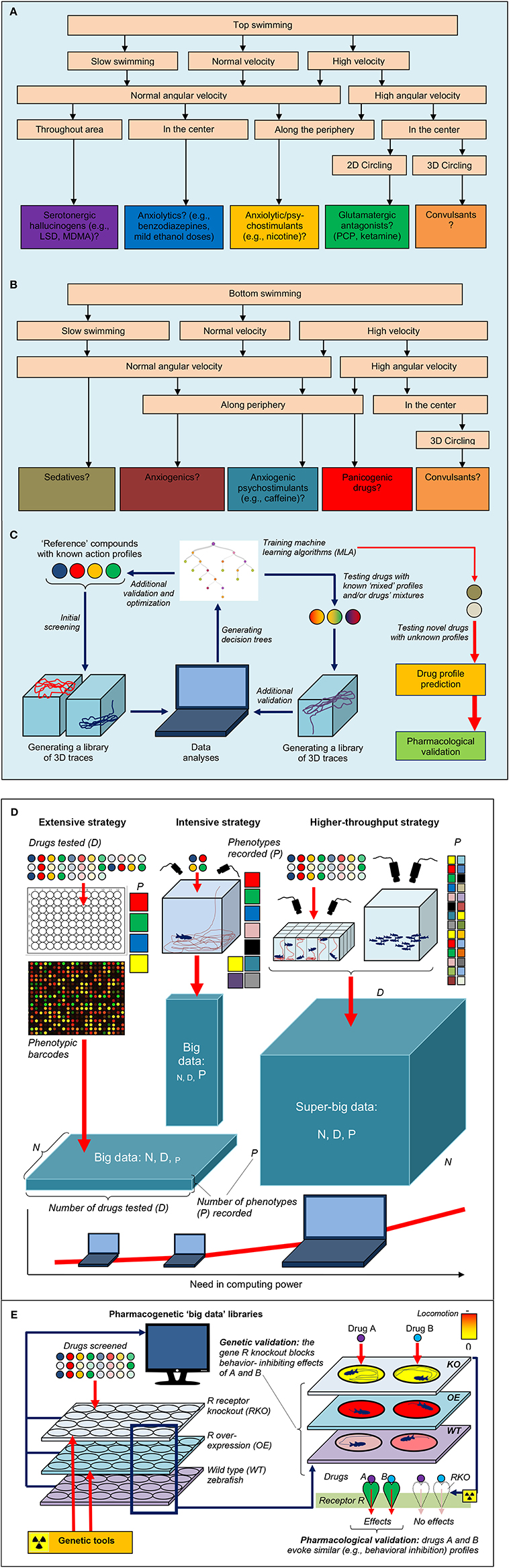
Figure 2. Example of potential decision trees (A,B) that can be used by automated in-vivo drug-screening platforms in adult zebrafish to predict the drugs' pharmacological profiles (see Figures 1B,C for examples of 3D traces). (C) Illustrates the general strategy of drug screening based on machine learning algorithms and 3D trace analyses. Summary of different strategies that can be used to generate high-density biological “big data” from zebrafish in-vivo screens. (D) Illustrated the extensive approach, testing a large number of drugs (D) in multiple animals (N) but recording few endpoints/phenotypes (P). This approach is markedly facilitated by using phenotypic barcoding approaches (Glossary). In contrast, the intensive approach screens few drugs, uses few animals but records many endpoints. The higher-throughput strategy, based on screening many compounds with multiple endpoints in a large number of animals, is empowered by locomotor pattern and behavioral recognition (Glossary) as well as automated slimuli delivery and experimental manipulations. (E) Shows the value of increased drug data “dimensionality” by including pharmacogenetic results (from wild type vs. mutant zebrafish) for providing important mechanistic insights into the drugs action. For example, a hypothetical antagonism of a drug A at a receptor R can be confirmed by screening the reference compound B (with known anti-R activity) and by mutating zebrafish gene R to abolish A/B-like activity in the mutants. Applying bioinformatics-based approaches and combining both lines of such evidence will facilitate the discovery of anti-R compounds (based on A-like pharmacology in zebrafish), followed by subsequent target validation in rodents and clinical studies.
Developing Higher-Throughput Zebrafish Screens
The choice between extensive and intensive analyses in zebrafish models no longer poses a critical dilemma for researchers, because modern information technology tools and the low cost of zebrafish (vs. rodent) assays markedly facilitate the collection and computer processing of data (Figures 2D,E). Here, we argue that the two strategies can now be co-applied in zebrafish screening studies, resulting in “higher-throughput” screens capable of generating “super-big data” (Figures 2D,E, Glossary). Enhancing the efficiency of zebrafish-based models for innovative CNS drug discovery, this strategy combines the advantages of the extensive approach (utilizing large numbers of drugs and animals tested) with the benefits of using extensive studies (focusing on a large number of phenotypes that help generate mechanistic insights; Figures 2D,E).
In addition to zebrafish's utility for HTS per se, this species also enjoys the advantage of having a sophisticated molecular genetic toolset developed for it. For example, traditional N-ethyl-N-nitrosourea (ENU)-induced (Mullins et al., 1994) or viral vector-mediated (Amsterdam et al., 1999) mutagenesis and gene silencing using morpholinos complement other genetic tools recently developed for zebrafish, such as “gene-breaking transposon” (GBT) (Petzold et al., 2009) screens, “clustered regularly interspaced short palindromic repeats” (CRISPR) (Hwang et al., 2013), zinc finger nucleases(Doyon et al., 2008), “transcription activator-like effector nuclease” (TALEN) (Zu et al., 2013) and “targeting induced local lesions in genomes” (TILLING) (Moens et al., 2008). As these genetic tools may manipulate a wide range of zebrafish genes, the ability to apply informatics-driven methods to systematically collect, store and analyze zebrafish pharmacogenetics data further empowers CNS drug discovery. For example, Figure 2E shows how increasing the “dimensionality” of traditional phenotypic screens by including an additional (genetic) dimension becomes a useful strategy of research in this field. Thus, systematic accumulation of pharmacogenetic evidence (with comparative analyses of drug response in wildtype vs. mutant fish) into large online data libraries becomes key for biomolecular data validation and generating novel mechanistic insights into drugs' action (Figure 2E) as part of higher-throughput screening using zebrafish.
Increasingly Sophisticated Behavioral Screening Tools
The higher-throughput screening approach in today's drug discovery also becomes possible due to a combination of rapidly increasing computer processing power with sensitive video-recording tools that generate automated phenotypic data with high spatial and temporal resolution (Figure 2E) (Cachat et al., 2011; Branson, 2014). In addition to testing individual fish with multiple individual endpoints to generate super-big data, simultaneous video-tracking of multiple fish swimming in groups (shoals) (Green et al., 2012; Branson, 2014; Kalueff et al., 2014b) is another strategy to achieve this goal. Moreover, recent advances in automated behavioral recognition (Glossary) further facilitate efficient in-vivo drug screens using zebrafish. For example, monitoring several zebrafish body points (e.g., nose, mid-body and tail) enables both automated quantification of fish locomotion and interpretation of complex zebrafish behaviors (e.g., chasing, biting, social approach or reduced sociability Kalueff et al., 2013) that parallel human phenotypes (see Stewart et al., 2014a for details).
Finally, as behavioral analyses become more efficient, stimuli that induce fish responses also become better standardized in zebrafish models. For example, currently available automated drug and/or food delivery tools improve zebrafish studies of drugs that affect reward mechanisms. Likewise, zebrafish operant behavior can be examined by using changes in zebrafish body position (e.g., swimming to a specific location) to deliver behavioral stimuli, such as punishment or reward. Exposing fish to predator or conspecific images on a computer screen (Luca and Gerlai, 2012), as well as using a computer-animated robotic “fish” (Butail et al., 2013; Cianca et al., 2013), represent other excellent examples of improved controllability of stimulus presentation in zebrafish models. Because stimulus delivery, behavioral quantification and phenotype recognition/interpretation are now increasingly computerized, zebrafish-based in-vivo screening in general is becoming more automated and higher-throughput.
Concluding Remarks
Fully automated robot-based screening platforms for larval and, eventually, adult zebrafish will soon become routine in zebrafish neurophenotyping studies. The HTS systems developed specifically for CNS drugs that affect complex behaviors will increase both the “width” and the “depth” of such screens (Figures 2D,E). Coupled with HTS, the ever increasing sophistication of genetic and behavior-recognition tools transform zebrafish models into higher-throughput in-vivo assays, allowing comprehensive coverage of the biological mechanisms of complex brain disorders, and leading to innovative CNS drug discovery.
Conflict of Interest Statement
The Review Editor Dr. Caroline Helen Brennan declares that, despite having collaborated with the author Dr. Allan V. Kalueff, the review process was handled objectively. The authors declare that the research was conducted in the absence of any commercial or financial relationships that could be construed as a potential conflict of interest.
Acknowledgments
Research is supported by ZENEREI Institute, NIH/NIDA, LA Board of Regents, Guangdong Ocean University and GDOU platform grant (Allan V. Kalueff), as well as by NIH/NIAAA and NSERC (Robert Gerlai). The authors thank Dr. Lucas Noldus, Ruud Tegelenbosch and colleagues (Noldus IT, Netherlands) for providing images for figures used in this paper. The authors acknowledge Michael Nguyen for his assistance with this manuscript.
Supplementary Material
The Supplementary Material for this article can be found online at: http://www.frontiersin.org/journal/10.3389/fnbeh.2015.00014/abstract
References
Amsterdam, A., Burgess, S., Golling, G., Chen, W., Sun, Z., Townsend, K., et al. (1999). A large-scale insertional mutagenesis screen in zebrafish. Genes Dev. 13, 2713–2724. doi: 10.1101/gad.13.20.2713
Pubmed Abstract | Pubmed Full Text | CrossRef Full Text | Google Scholar
Baraban, S. C., Taylor, M. R., Castro, P. A., and Baier, H. (2005). Pentylenetetrazole induced changes in zebrafish behavior, neural activity and c-fos expression. Neuroscience 131, 759–768. doi: 10.1016/j.neuroscience.2004.11.031
Pubmed Abstract | Pubmed Full Text | CrossRef Full Text | Google Scholar
Bernier, R., Golzio, C., Xiong, B., Stessman, H. A., Coe, B. P., Penn, O., et al. (2014). Disruptive CHD8 mutations define a subtype of autism early in development. Cell 158, 263–276. doi: 10.1016/j.cell.2014.06.017
Pubmed Abstract | Pubmed Full Text | CrossRef Full Text | Google Scholar
Branson, K. (2014). Distinguishing seemingly indistinguishable animals with computer vision. Nat. Methods 11, 721–722. doi: 10.1038/nmeth.3004
Pubmed Abstract | Pubmed Full Text | CrossRef Full Text | Google Scholar
Bruni, G., Lakhani, P., and Kokel, D. (2014). Discovering novel neuroactive drugs through high-throughput behavior-based chemical screening in the zebrafish. Front. Pharmacol. 5:153. doi: 10.3389/fphar.2014.00153
Pubmed Abstract | Pubmed Full Text | CrossRef Full Text | Google Scholar
Butail, S., Bartolini, T., and Porfiri, M. (2013). Collective response of zebrafish shoals to a free-swimming robotic fish. PLoS ONE 8:e76123. doi: 10.1371/journal.pone.0076123
Pubmed Abstract | Pubmed Full Text | CrossRef Full Text | Google Scholar
Cachat, J., Kyzar, E. J., Collins, C., Gaikwad, S., Green, J., Roth, A., et al. (2013). Unique and potent effects of acute ibogaine on zebrafish: the developing utility of novel aquatic models for hallucinogenic drug research. Behav. Brain Res. 236, 258–269. doi: 10.1016/j.bbr.2012.08.041
Pubmed Abstract | Pubmed Full Text | CrossRef Full Text | Google Scholar
Cachat, J., Stewart, A., Grossman, L., Gaikwad, S., Kadri, F., Chung, K. M., et al. (2010). Measuring behavioral and endocrine responses to novelty stress in adult zebrafish. Nat. Protoc. 5, 1786–1799. doi: 10.1038/nprot.2010.140
Pubmed Abstract | Pubmed Full Text | CrossRef Full Text | Google Scholar
Cachat, J., Stewart, A., Utterback, E., Hart, P., Gaikwad, S., Wong, K., et al. (2011). Three-dimensional neurophenotyping of adult zebrafish behavior. PLoS ONE 6:e17597. doi: 10.1371/journal.pone.0017597
Pubmed Abstract | Pubmed Full Text | CrossRef Full Text | Google Scholar
Cianca, V., Bartolini, T., Porfiri, M., and Macri, S. (2013). A robotics-based behavioral paradigm to measure anxiety-related responses in zebrafish. PLoS ONE 8:e69661. doi: 10.1371/journal.pone.0069661
Pubmed Abstract | Pubmed Full Text | CrossRef Full Text | Google Scholar
Doyon, Y., McCammon, J. M., Miller, J. C., Faraji, F., Ngo, C., Katibah, G. E., et al. (2008). Heritable targeted gene disruption in zebrafish using designed zinc-finger nucleases. Nat. Biotechnol. 26, 702–708. doi: 10.1038/nbt1409
Pubmed Abstract | Pubmed Full Text | CrossRef Full Text | Google Scholar
Duman, R. S., Heninger, G. R., and Nestler, E. J. (1994). Molecular psychiatry. Adaptations of receptor-coupled signal transduction pathways underlying stress- and drug-induced neural plasticity. J. Nerv. Ment. Dis. 182, 692–700. doi: 10.1097/00005053-199412000-00003
Pubmed Abstract | Pubmed Full Text | CrossRef Full Text | Google Scholar
Egan, R. J., Bergner, C. L., Hart, P. C., Cachat, J. M., Canavello, P. R., Elegante, M. F., et al. (2009). Understanding behavioral and physiological phenotypes of stress and anxiety in zebrafish. Behav. Brain Res. 205, 38–44. doi: 10.1016/j.bbr.2009.06.022
Pubmed Abstract | Pubmed Full Text | CrossRef Full Text | Google Scholar
Gerlai, R., Lahav, M., Guo, S., and Rosenthal, A. (2000). Drinks like a fish: zebra fish (Danio rerio) as a behavior genetic model to study alcohol effects. Pharmacol. Biochem. Behav. 67, 773–782. doi: 10.1016/S0091-3057(00)00422-6
Pubmed Abstract | Pubmed Full Text | CrossRef Full Text | Google Scholar
Green, J., Collins, C., Kyzar, E. J., Pham, M., Roth, A., Gaikwad, S., et al. (2012). Automated high-throughput neurophenotyping of zebrafish social behavior. J. Neurosci. Methods 210, 266–271. doi: 10.1016/j.jneumeth.2012.07.017
Pubmed Abstract | Pubmed Full Text | CrossRef Full Text | Google Scholar
Griebel, G., and Holmes, A. (2013). 50 years of hurdles and hope in anxiolytic drug discovery. Nat. Rev. Drug Discov. 12, 667–687. doi: 10.1038/nrd4075
Pubmed Abstract | Pubmed Full Text | CrossRef Full Text | Google Scholar
Griffiths, B. B., Schoonheim, P. J., Ziv, L., Voelker, L., Baier, H., and Gahtan, E. (2012). A zebrafish model of glucocorticoid resistance shows serotonergic modulation of the stress response. Front. Behav. Neurosci. 6:68. doi: 10.3389/fnbeh.2012.00068
Pubmed Abstract | Pubmed Full Text | CrossRef Full Text | Google Scholar
Grossman, L., Utterback, E., Stewart, A., Gaikwad, S., Chung, K. M., Suciu, C., et al. (2010). Characterization of behavioral and endocrine effects of LSD on zebrafish. Behav. Brain Res. 214, 277–284. doi: 10.1016/j.bbr.2010.05.039
Pubmed Abstract | Pubmed Full Text | CrossRef Full Text | Google Scholar
Hwang, W. Y., Fu, Y., Reyon, D., Maeder, M. L., Tsai, S. Q., Sander, J. D., et al. (2013). Efficient genome editing in zebrafish using a CRISPR-Cas system. Nat. Biotechnol. 31, 227–229. doi: 10.1038/nbt.2501
Pubmed Abstract | Pubmed Full Text | CrossRef Full Text | Google Scholar
Jin, S., Sarkar, K. S., Jin, Y. N., Liu, Y., Kokel, D., Van Ham, T. J., et al. (2013). An in vivo zebrafish screen identifies organophosphate antidotes with diverse mechanisms of action. J. Biomol. Screen. 18, 108–115. doi: 10.1177/1087057112458153
Pubmed Abstract | Pubmed Full Text | CrossRef Full Text | Google Scholar
Kalueff, A. V., Echevarria, D. J., and Stewart, A. M. (2014a). Gaining translational momentum: more zebrafish models for neuroscience research. Prog. Neuropsychopharmacol. Biol. Psychiatry 55, 1–6. doi: 10.1016/j.pnpbp.2014.01.022
Pubmed Abstract | Pubmed Full Text | CrossRef Full Text | Google Scholar
Kalueff, A. V., Gebhardt, M., Stewart, A. M., Cachat, J. M., Brimmer, M., Chawla, J. S., et al. (2013). Towards a comprehensive catalog of zebrafish behavior 1.0 and beyond. Zebrafish 10, 70–86. doi: 10.1089/zeb.2012.0861
Pubmed Abstract | Pubmed Full Text | CrossRef Full Text | Google Scholar
Kalueff, A. V., Stewart, A. M., and Gerlai, R. (2014b). Zebrafish as an emerging model for studying complex brain disorders. Trends Pharmacol. Sci. 35, 63–75. doi: 10.1016/j.tips.2013.12.002
Pubmed Abstract | Pubmed Full Text | CrossRef Full Text | Google Scholar
Kily, L. J., Cowe, Y. C., Hussain, O., Patel, S., McElwaine, S., Cotter, F. E., et al. (2008). Gene expression changes in a zebrafish model of drug dependency suggest conservation of neuro-adaptation pathways. J. Exp. Biol. 211, 1623–1634. doi: 10.1242/jeb.014399
Pubmed Abstract | Pubmed Full Text | CrossRef Full Text | Google Scholar
Kokel, D., Bryan, J., Laggner, C., White, R., Cheung, C. Y., Mateus, R., et al. (2010). Rapid behavior-based identification of neuroactive small molecules in the zebrafish. Nat. Chem. Biol. 6, 231–237. doi: 10.1038/nchembio.307
Pubmed Abstract | Pubmed Full Text | CrossRef Full Text | Google Scholar
Kokel, D., and Peterson, R. T. (2008). Chemobehavioural phenomics and behaviour-based psychiatric drug discovery in the zebrafish. Brief. Funct. Genomic. Proteomic. 7, 483–490. doi: 10.1093/bfgp/eln040
Pubmed Abstract | Pubmed Full Text | CrossRef Full Text | Google Scholar
Kokel, D., and Peterson, R. T. (2011). Using the zebrafish photomotor response for psychotropic drug screening. Methods Cell Biol. 105, 517–524. doi: 10.1016/B978-0-12-381320-6.00022-9
Pubmed Abstract | Pubmed Full Text | CrossRef Full Text | Google Scholar
Kokel, D., Rennekamp, A. J., Shah, A. H., Liebel, U., and Peterson, R. T. (2012). Behavioral barcoding in the cloud: embracing data-intensive digital phenotyping in neuropharmacology. Trends Biotechnol. 30, 421–425. doi: 10.1016/j.tibtech.2012.05.001
Pubmed Abstract | Pubmed Full Text | CrossRef Full Text | Google Scholar
Kyzar, E. J., Collins, C., Gaikwad, S., Green, J., Roth, A., Monnig, L., et al. (2012). Effects of hallucinogenic agents mescaline and phencyclidine on zebrafish behavior and physiology. Prog. Neuropsychopharmacol. Biol. Psychiatry 37, 194–202. doi: 10.1016/j.pnpbp.2012.01.003
Pubmed Abstract | Pubmed Full Text | CrossRef Full Text | Google Scholar
Laggner, C., Kokel, D., Setola, V., Tolia, A., Lin, H., Irwin, J. J., et al. (2012). Chemical informatics and target identification in a zebrafish phenotypic screen. Nat. Chem. Biol. 8, 144–146. doi: 10.1038/nchembio.732
Pubmed Abstract | Pubmed Full Text | CrossRef Full Text | Google Scholar
Lange, M., Norton, W., Coolen, M., Chaminade, M., Merker, S., Proft, F., et al. (2012a). The ADHD-linked gene Lphn3.1 controls locomotor activity and impulsivity in zebrafish. Mol. Psychiatry 17, 855. doi: 10.1038/mp.2012.119
Pubmed Abstract | Pubmed Full Text | CrossRef Full Text | Google Scholar
Lange, M., Norton, W., Coolen, M., Chaminade, M., Merker, S., Proft, F., et al. (2012b). The ADHD-susceptibility gene lphn3.1 modulates dopaminergic neuron formation and locomotor activity during zebrafish development. Mol. Psychiatry 17, 946–954. doi: 10.1038/mp.2012.29
Pubmed Abstract | Pubmed Full Text | CrossRef Full Text | Google Scholar
Li, Q., Lin, J., Zhang, Y., Liu, X., Chen, X. Q., Xu, M. Q., et al. (2015). Differential behavioral responses of zebrafish larvae to yohimbine treatment. Psychopharmacology (Berl.) 232, 197–208. doi: 10.1007/s00213-014-3656-5
Pubmed Abstract | Pubmed Full Text | CrossRef Full Text | Google Scholar
Luca, R. M., and Gerlai, R. (2012). Animated bird silhouette above the tank: acute alcohol diminishes fear responses in zebrafish. Behav. Brain Res. 229, 194–201. doi: 10.1016/j.bbr.2012.01.021
Pubmed Abstract | Pubmed Full Text | CrossRef Full Text | Google Scholar
Markou, A., Chiamulera, C., Geyer, M. A., Tricklebank, M., and Steckler, T. (2009). Removing obstacles in neuroscience drug discovery: the future path for animal models. Neuropsychopharmacology 34, 74–89. doi: 10.1038/npp.2008.173
Pubmed Abstract | Pubmed Full Text | CrossRef Full Text | Google Scholar
Mathur, P., Berberoglu, M. A., and Guo, S. (2011a). Preference for ethanol in zebrafish following a single exposure. Behav. Brain Res. 217, 128–133. doi: 10.1016/j.bbr.2010.10.015
Pubmed Abstract | Pubmed Full Text | CrossRef Full Text | Google Scholar
Mathur, P., and Guo, S. (2011). Differences of acute versus chronic ethanol exposure on anxiety-like behavioral responses in zebrafish. Behav. Brain Res. 219, 234–239. doi: 10.1016/j.bbr.2011.01.019
Pubmed Abstract | Pubmed Full Text | CrossRef Full Text | Google Scholar
Mathur, P., Lau, B., and Guo, S. (2011b). Conditioned place preference behavior in zebrafish. Nat. Protoc. 6, 338–345. doi: 10.1038/nprot.2010.201
Pubmed Abstract | Pubmed Full Text | CrossRef Full Text | Google Scholar
Maximino, C., Da Silva, A. W., Araujo, J., Lima, M. G., Miranda, V., Puty, B., et al. (2014a). Fingerprinting of psychoactive drugs in zebrafish anxiety-like behaviors. PLoS ONE 9:e103943. doi: 10.1371/journal.pone.0103943
Pubmed Abstract | Pubmed Full Text | CrossRef Full Text | Google Scholar
Maximino, C., Da Silva, A. W., Gouveia, A. Jr., and Herculano, A. M. (2011). Pharmacological analysis of zebrafish (Danio rerio) scototaxis. Prog. Neuropsychopharmacol. Biol. Psychiatry 35, 624–631. doi: 10.1016/j.pnpbp.2011.01.006
Pubmed Abstract | Pubmed Full Text | CrossRef Full Text | Google Scholar
Maximino, C., De Brito, T. M., Colmanetti, R., Pontes, A. A., De Castro, H. M., De Lacerda, R. I., et al. (2010). Parametric analyses of anxiety in zebrafish scototaxis. Behav. Brain Res. 210, 1–7. doi: 10.1016/j.bbr.2010.01.031
Pubmed Abstract | Pubmed Full Text | CrossRef Full Text | Google Scholar
Maximino, C., Lima, M. G., Costa, C. C., Guedes, I. M., and Herculano, A. M. (2014b). Fluoxetine and WAY 100,635 dissociate increases in scototaxis and analgesia induced by conspecific alarm substance in zebrafish (Danio rerio Hamilton 1822). Pharmacol. Biochem. Behav. 124, 425–433. doi: 10.1016/j.pbb.2014.07.003
Pubmed Abstract | Pubmed Full Text | CrossRef Full Text | Google Scholar
Maximino, C., Puty, B., Benzecry, R., Araujo, J., Lima, M. G., De Jesus Oliveira Batista, E., et al. (2013). Role of serotonin in zebrafish (Danio rerio) anxiety: relationship with serotonin levels and effect of buspirone, WAY 100635, SB 224289, fluoxetine and para-chlorophenylalanine (pCPA) in two behavioral models. Neuropharmacology 71, 83–97. doi: 10.1016/j.neuropharm.2013.03.006
Pubmed Abstract | Pubmed Full Text | CrossRef Full Text | Google Scholar
Mitchell, K. (2011). The miswired brain: making connections from neurodevelopment to psychopathology. BMC Biol. 9:23. doi: 10.1186/1741-7007-9-23
Pubmed Abstract | Pubmed Full Text | CrossRef Full Text | Google Scholar
Moens, C. B., Donn, T. M., Wolf-Saxon, E. R., and Ma, T. P. (2008). Reverse genetics in zebrafish by TILLING. Brief. Funct. Genomic. Proteomic. 7, 454–459. doi: 10.1093/bfgp/eln046
Pubmed Abstract | Pubmed Full Text | CrossRef Full Text | Google Scholar
Mullins, M. C., Hammerschmidt, M., Haffter, P., and Nüsslein-Volhard, C. (1994). Large-scale mutagenesis in the zebrafish: in search of genes controlling development in a vertebrate. Curr. Biol. 4, 189–202. doi: 10.1016/S0960-9822(00)00048-8
Pubmed Abstract | Pubmed Full Text | CrossRef Full Text | Google Scholar
Neelkantan, N., Mikhaylova, A., Stewart, A. M., Arnold, R., Gjeloshi, V., Kondaveeti, D., et al. (2013). Perspectives on zebrafish models of hallucinogenic drugs and related psychotropic compounds. ACS Chem. Neurosci. 4, 1137–1150. doi: 10.1021/cn400090q
Pubmed Abstract | Pubmed Full Text | CrossRef Full Text | Google Scholar
Nestler, E. J. (2013). The origins of molecular psychiatry. J. Mol. Psychiatry 1:3. doi: 10.1186/2049-9256-1-3
Pubmed Abstract | Pubmed Full Text | CrossRef Full Text | Google Scholar
Norton, W. H., Stumpenhorst, K., Faus-Kessler, T., Folchert, A., Rohner, N., Harris, M. P., et al. (2011). Modulation of Fgfr1a signaling in zebrafish reveals a genetic basis for the aggression-boldness syndrome. J. Neurosci. 31, 13796–13807. doi: 10.1523/JNEUROSCI.2892-11.2011
Pubmed Abstract | Pubmed Full Text | CrossRef Full Text | Google Scholar
Pan, Y., Kaiguo, M., Razak, Z., Westwood, J. T., and Gerlai, R. (2011). Chronic alcohol exposure induced gene expression changes in the zebrafish brain. Behav. Brain Res. 216, 66–76. doi: 10.1016/j.bbr.2010.07.017
Pubmed Abstract | Pubmed Full Text | CrossRef Full Text | Google Scholar
Parker, M. O., Brock, A. J., Millington, M. E., and Brennan, C. H. (2013). Behavioural phenotyping of casper mutant and 1-pheny-2-thiourea treated adult zebrafish. Zebrafish 10, 466–471. doi: 10.1089/zeb.2013.0878
Pubmed Abstract | Pubmed Full Text | CrossRef Full Text | Google Scholar
Petzold, A. M., Balciunas, D., Sivasubbu, S., Clark, K. J., Bedell, V. M., Westcot, S. E., et al. (2009). Nicotine response genetics in the zebrafish. Proc. Natl. Acad. Sci. 106, 18662–18667. doi: 10.1073/pnas.0908247106
Pubmed Abstract | Pubmed Full Text | CrossRef Full Text | Google Scholar
Rihel, J., Prober, D. A., Arvanites, A., Lam, K., Zimmerman, S., Jang, S., et al. (2010). Zebrafish behavioral profiling links drugs to biological targets and rest/wake regulation. Science 327, 348–351. doi: 10.1126/science.1183090
Pubmed Abstract | Pubmed Full Text | CrossRef Full Text | Google Scholar
Rosemberg, D. B., Rico, E. P., Mussulini, B. H., Piato, A. L., Calcagnotto, M. E., Bonan, C. D., et al. (2011). Differences in spatio-temporal behavior of zebrafish in the open tank paradigm after a short-period confinement into dark and bright environments. PLoS ONE 6:e19397. doi: 10.1371/journal.pone.0019397
Pubmed Abstract | Pubmed Full Text | CrossRef Full Text | Google Scholar
Soleymani, A., Cachat, J. M., Robinson, K., Dodge, S., Kalueff, A., and Wurbel, R. (2014). Integrating cross-scale analysis in the spatial and temporal domains for classification of behavioral movement. J. Spatial Inform. Sci. 8, 1–25. doi: 10.5311/JOSIS.2014.8.162
Stewart, A. M., Braubach, O., Spitsbergen, J., Gerlai, R., and Kalueff, A. V. (2014a). Zebrafish models for translational neuroscience research: from tank to bedside. Trends Neurosci. 37, 264–278. doi: 10.1016/j.tins.2014.02.011
Pubmed Abstract | Pubmed Full Text | CrossRef Full Text | Google Scholar
Stewart, A. M., Cachat, J., Gaikwad, S., Robinson, K. S., Gebhardt, M., and Kalueff, A. V. (2013). Perspectives on experimental models of serotonin syndrome in zebrafish. Neurochem. Int. 62, 893–902. doi: 10.1016/j.neuint.2013.02.018
Pubmed Abstract | Pubmed Full Text | CrossRef Full Text | Google Scholar
Stewart, A. M., Grossman, L., Nguyen, M., Maximino, C., Rosemberg, D. B., Echevarria, D., et al. (2014b). Aquatic toxicology of fluoxetine:understanding the knowns and the unknowns. Aquat. Toxicol. 156, 269–273. doi: 10.1016/j.aquatox.2014.08.014
Pubmed Abstract | Pubmed Full Text | CrossRef Full Text | Google Scholar
Stewart, A. M., and Kalueff, A. V. (2013). Controlled substances and innovation of biomedicine: a preclinical perspective. Nat. Rev. Neurosci. 14, 877. doi: 10.1038/nrn3530-c1
Pubmed Abstract | Pubmed Full Text | CrossRef Full Text | Google Scholar
Stewart, A. M., and Kalueff, A. V. (2014). Anxiolytic drug discovery: what are the novel approaches and how can we improve them? Expert Opin. Drug Discov. 9, 15–26. doi: 10.1517/17460441.2014.857309
Pubmed Abstract | Pubmed Full Text | CrossRef Full Text | Google Scholar
Wong, K., Stewart, A., Gilder, T., Wu, N., Frank, K., Gaikwad, S., et al. (2010). Modeling seizure-related behavioral and endocrine phenotypes in adult zebrafish. Brain Res. 1348, 209–215. doi: 10.1016/j.brainres.2010.06.012
Pubmed Abstract | Pubmed Full Text | CrossRef Full Text | Google Scholar
Ziv, L., Muto, A., Schoonheim, P. J., Meijsing, S. H., Strasser, D., Ingraham, H. A., et al. (2013). An affective disorder in zebrafish with mutation of the glucocorticoid receptor. Mol. Psychiatry 18, 681–691. doi: 10.1038/mp.2012.64
Pubmed Abstract | Pubmed Full Text | CrossRef Full Text | Google Scholar
Zu, Y., Tong, X., Wang, Z., Liu, D., Pan, R., Li, Z., et al. (2013). TALEN-mediated precise genome modification by homologous recombination in zebrafish. Nat. Methods 10, 329–331. doi: 10.1038/nmeth.2374
Pubmed Abstract | Pubmed Full Text | CrossRef Full Text | Google Scholar
Glossary
Barcoding is a phenotyping approach that expresses complex behavioral changes by splitting them into simple behavioral “barcodes” (Kokel et al., 2010, 2012), to classify psychotropic drugs and determine their mechanisms of action. This approach streamlines and facilitates the analysis of zebrafish phenotypes by providing a concise quantitative and visualized summary of the observed behaviors (Figures 2D,E) (Kokel et al., 2010, 2012). Typically applied to larval fish, this approach has recently been applied to adult zebrafish (Maximino et al., 2014a).
Behavioral recognition complements traditional behavioral quantification methods (e.g., Supplementary Table 1S online) and analyzes the location and temporal dynamics of multiple body parts, in order to interpret complex behaviors. For instance, moving fast nose-to-tail with another fish represents chasing, fast moving head toward another fish is attack, whereas slow moving head to head of another fish can be interpreted as social investigation (Kalueff et al., 2013). Behavioral recognition is markedly enhanced by using computer-based automated videotracking combined with analyses of multiple body parts (Stewart et al., 2014a).
“Big data” represents collected data sets which are difficult to process using traditional data processing approaches due to their size/density (e.g., currently, big data involves millions of terabites of information). In zebrafish screens, big data can be generated using sophisticated automated computer-based video-tracking tools, recording spatial coordinates of zebrafish and their alterations over time.
Extensive strategy in zebrafish drug discovery (Figures 2D,E) employs multiple zebrafish (large animal numbers, N) to rapidly screen many CNS drugs (large D's) and identify novel promising compounds, based on alteration in selected well-validated individual phenotypes (small P's).
High-throughput screens (HTS) in zebrafish involve rapid testing of a very large number of compounds (typically >500–1000 per day) vs. medium- or low-throughput screens. The process often entails a high degree of automation. Traditionally, HTS utilize an extensive strategy (Figure 2D), yet are limited in their ability to thoroughly examine large numbers of endpoints and their associated patterns.
Intensive strategy in zebrafish drug discovery (Figure 2D) focuses on in-depth analyses of multiple phenotypes (large P's) evoked by a small number of experimental manipulations (e.g., drugs, small D's) in a fewer animals (small N's), aiming to increase our mechanistic understanding of various CNS processes and their experimental modulation.
Neurophenomics is an area of neuroscience research (part of phenomics) that examines the interactions between various CNS phenotypes (e.g., zebrafish behaviors) and their response to endogenous (e.g., genetic mutation) or exogenous (e,g., drugs, environmental stressors) factors. A primary aim of zebrafish neurophenomics is to increase the ability to measure and dissect various phenotypes (e.g., by using HTS and test batteries).
Higher-throughput screening in zebrafish neurophenomics combines principles of intensive and extensive screening high-throughput approaches to generate a higher-density data (“super-big data,” Figure 2D). Specifically, the extensive nature of conventional zebrafish HTS is utilized while further assessing in-depth multiple novel endpoints and motor patterning. In general, the higher-throughput strategy “digs deeper” as it uncovers rich phenotypical information hidden in datasets, while still maintaining the rapid and expansive output of HTS.
Three-dimensional (3D) phenotyping is an approach that is based on recording zebrafish locomotor responses, such as 3D swim traces in XYZ coordinates, by several cameras (e.g., two angled cameras as in Figure 1B) (Cachat et al., 2011), enabling in-depth characterization of phenotypic patterns after acute or chronic drug treatment.
Video-tracking is a method of behavioral registration in a single or grouped zebrafish (Figure 1A), using computer-generated 2D (e.g., top- or side-view recording) or 3D phenotyping analyses. Importantly, video-tracking technology is highly automated, and offers the ability to measure a wide array of endpoints not quantifiable through manual observation (Cachat et al., 2011; Branson, 2014).
Keywords: high-throughput screens, zebrafish models, big data, CNS drug discovery, phenomics
Citation: Stewart AM, Gerlai R and Kalueff AV (2015) Developing highER-throughput zebrafish screens for in-vivo CNS drug discovery. Front. Behav. Neurosci. 9:14. doi: 10.3389/fnbeh.2015.00014
Received: 12 August 2014; Accepted: 14 January 2015;
Published online: 12 February 2015.
Edited by:
Nuno Sousa, University of Minho, PortugalReviewed by:
Caroline Helen Brennan, Queen Mary University of London, UKCarla Denise Bonan, Pontifícia Universidade Católica do Rio Grande do Sul, Brazil
Copyright © 2015 Stewart, Gerlai and Kalueff. This is an open-access article distributed under the terms of the Creative Commons Attribution License (CC BY). The use, distribution or reproduction in other forums is permitted, provided the original author(s) or licensor are credited and that the original publication in this journal is cited, in accordance with accepted academic practice. No use, distribution or reproduction is permitted which does not comply with these terms.
*Correspondence: Allan V. Kalueff, ZENEREI Institute and The International Zebrafish Neuroscience Research Consortium, 309 Palmer Court, Slidell, LA 70458, USA e-mail:YXZrYWx1ZWZmQGdtYWlsLmNvbQ==