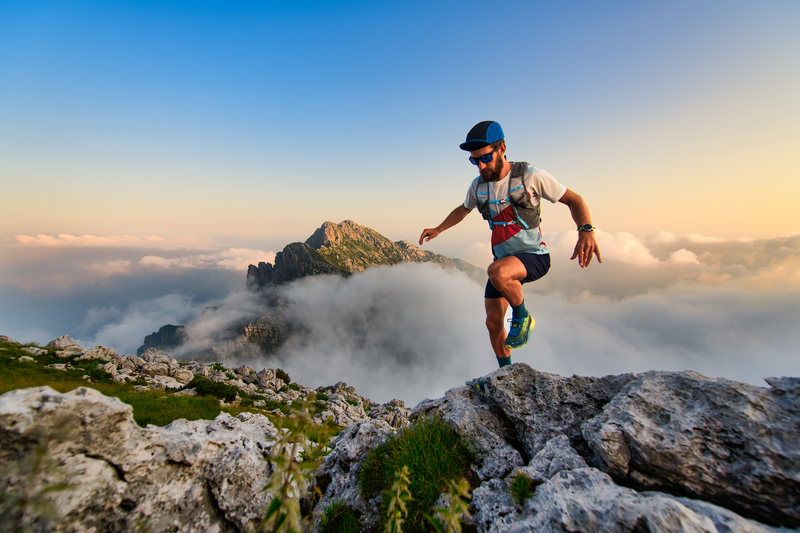
94% of researchers rate our articles as excellent or good
Learn more about the work of our research integrity team to safeguard the quality of each article we publish.
Find out more
ORIGINAL RESEARCH article
Front. Behav. Neurosci. , 21 March 2011
Sec. Motivation and Reward
volume 5 - 2011 | https://doi.org/10.3389/fnbeh.2011.00015
This article is part of the Research Topic Reinforcement Learning View all 5 articles
The basal ganglia are thought to play a crucial role in reinforcement learning. Central to the learning mechanism are dopamine (DA) D1 and D2 receptors located in the cortico-striatal synapses. However, it is still unclear how this DA-mediated synaptic plasticity is deployed and coordinated during reward-contingent behavioral changes. Here we propose a computational model of reinforcement learning that uses different thresholds of D1- and D2-mediated synaptic plasticity which are antagonized by DA-independent synaptic plasticity. A phasic increase in DA release caused by a larger-than-expected reward induces long-term potentiation (LTP) in the direct pathway, whereas a phasic decrease in DA release caused by a smaller-than-expected reward induces a cessation of long-term depression, leading to LTP in the indirect pathway. This learning mechanism can explain the robust behavioral adaptation observed in a location-reward-value-association task where the animal makes shorter latency saccades to reward locations. The changes in saccade latency become quicker as the monkey becomes more experienced. This behavior can be explained by a switching mechanism which activates the cortico-striatal circuit selectively. Our model also shows how D1- or D2-receptor blocking experiments affect selectively either reward or no-reward trials. The proposed mechanisms also explain the behavioral changes in Parkinson’s disease.
Many of our skillful daily actions are a result of constant positive and negative reinforcements. It is postulated that the basal ganglia (BG) contribute to this kind of reinforcement learning (see Hikosaka et al., 2006 for a review). Accordingly, reward-related activities have been observed in most of the BG components including dorsal striatum (Hikosaka et al., 1989; Apicella et al., 1992; Kawagoe et al., 1998; Lauwereyns et al., 2002; Costa et al., 2004; Samejima et al., 2005; Oyama et al., 2010), ventral striatum (Schultz et al., 1992; Kalenscher et al., 2010), subthalamic nucleus (STN; Darbaky et al., 2005), and even along the border region of the globus pallidus (GPb; DeLong, 1971; Hong and Hikosaka, 2008). Consequently, an insult in the BG, such as Parkinson’s disease (PD), severely affects the patient’s learning ability (Frank et al., 2004; Ell et al., 2010; Voon et al., 2010).
Previous studies gave an insight how the BG may contribute to this kind of learning. Particularly, neurons in the caudate nucleus (CD; part of the striatum) flexibly encode visual cues that predict different amounts or probabilities of reward (Apicella et al., 1992; Kawagoe et al., 1998; Lauwereyns et al., 2002; Samejima et al., 2005). For example, when a monkey performs a visually guided saccade task with positionally biased reward outcomes, called the “one direction reward (1DR)” task (Figure 1A), many CD neurons respond to a visual cue and the responses are often enhanced (and occasionally depressed) when the cue indicates a larger-than-average amount of reward during a block of trials. Also, there was a tight block-to-block correlation between the changes in CD neuronal activity preceding target onset and the changes in saccade latency (Lauwereyns et al., 2002). This relatively rapid modulation of CD neuronal activity seems to reflect a mechanism underlying reward-based learning. It has thus been hypothesized that these neuronal changes in the BG facilitate the eye movements to reward (Hikosaka et al., 2006).
Figure 1. Reinforcement learning experiments and involved learning circuit. (A) Sequence of events in the one direction rewarded saccade task (1DR). The monkey first fixated at the central spot (the dotted circle indicates the eye position). As the fixation point disappeared, a target appeared randomly on the right or left and the monkey was required to make a saccade to it immediately. Correct saccades in one direction were followed by a tone and juice reward; saccades in the other direction followed by a tone alone. The rewarded direction was fixed in a block of 24 trials, and was changed in the following block. (B) Distribution of saccade latencies in reward trials (in red) and in no-reward trials (in blue). (C) Illustration of D1 and D2 antagonist experiments. D1 or D2 antagonist was administrated in the caudate to examine the behavioral consequence in the 1DR task. Black, red, and purple connections indicate excitatory, inhibitory, and dopaminergic modulatory connections, respectively. (D) Hypothesized circuit involving D1 and D2 mediated plasticities. D1 and D2 mediated plasticities in direct and indirect pathways are assumed to contribute to eye movements. The purple arrows indicate dopaminergic modulatory connections. The lines with rectangle ends indicate inhibitory connections. Arrow ends indicate excitatory connections. Figures (A) and (B) are from Hong and Hikosaka (2008). Abbreviations: CD, caudate nucleus; D1, D2, D1, and D2 receptors; SC, superior colliculus; SNc/SNr, substantia nigra pars compacta/reticulata; GPe, globus pallidus external segment; FEF, frontal eye field; SEF, supplementary eye field; DLPF, dorsolateral prefrontal cortex; LIP, lateral intraparietal area; STN, subthalamic nucleus.
It has also been shown that dopamine (DA) plays a crucial role in learning in the BG. Phasic DA signals, in particular, have been hypothesized to cause reinforcement learning (Montague et al., 1996; Schultz et al., 1997; Schultz, 2007). This hypothesis has been supported by a recent study where suppression of phasic DA release by pharmacological manipulation impairs the acquisition of reward-related behavior in healthy human subjects (Pizzagalli et al., 2008). Also, it was shown that tonic occupation of DA receptors (Breitenstein et al., 2006; Mehta et al., 2008) and systemic manipulation of DA level (Pessiglione et al., 2006) block normal learning. In the case of PD patients, the level of DA determines how the patients learn. For example, subjects on L-DOPA medication are better in positive learning and worse in negative learning, and PD subjects off medication are better in negative learning and worse in positive learning (Frank et al., 2004).
However, there is evidence that DA has complex effects on BG neurons during reinforcement learning, including different effects on different BG pathways. In the BG there are two anatomically distinct pathways: the “direct” pathway whose striatal neurons have abundant D1 receptors, and the “indirect” pathway whose striatal neurons have abundant D2 receptors (Deng et al., 2006; Kravitz et al., 2010). These two receptors appear to modulate the glutamatergic synaptic plasticity in medium spiny neurons (MSNs) differently. Namely, D1 receptor-mediated DA signaling promotes long-term potentiation (LTP; Reynolds et al., 2001; Calabresi et al., 2007) whereas D2 receptor-mediated DA signaling induces long-term depression (LTD; Gerdeman et al., 2002; Kreitzer and Malenka, 2007). These findings suggest that the LTP is dominant in the direct pathway MSNs whereas LTD is dominant in the indirect pathway MSNs. However, such unidirectional plasticity might cause saturation of synaptic efficacy. To overcome this problem, some computational models (e.g., Brown et al., 2004) have implemented bidirectional plasticity (both LTP and LTD) in both pathways. Indeed, recent experimental studies indicate that the direct pathway, as well as the indirect pathway, implements both LTP and LTD (Picconi et al., 2003; Fino et al., 2005; Wang et al., 2006; Shen et al., 2008). For example, Shen et al. (2008) found that direct pathway MSNs also show LTD and indirect pathway MSNs also show LTP, both of which are independent of DA signaling.
Numerous studies have examined the influence of the D1 and D2 mediated processes in the BG on animal learning behavior (e.g., Frank et al., 2004; Yin et al., 2009). However, few of them have provided quantitative data that can be used to test a computational model (Frank et al., 2004). The 1DR task (Figure 1A) that has been used extensively in our laboratory is ideal for this purpose because trial-by-trial changes in the reaction time (or latency) of saccadic eye movements reflects reward-contingent learning and can be measured quantitatively. Furthermore, experimental manipulations of DA transmission in the CD and observation of ensuing oculomotor behavior were done by Nakamura and Hikosaka (2006; Figure 1C). They reported that after a D1 antagonist was injected in the CD, the saccadic latencies increased in reward trials, but not in no-reward trials. In contrast, after D2 antagonist injections, the saccadic latencies increased in no-reward trials, but not in reward trials.
In addition to the quantitative behavioral data, we have accumulated a rich set of data on the neuronal activity in many brain areas in the BG that relay visuo-oculomotor information including the CD, substantia nigra pars reticulata (SNr), STN, globus pallidus external segment (GPe), superior colliculus (SC), as well as frontal cortical areas (see Hikosaka et al., 2000, 2006; Figure 1D). We also have an extensive set of data that indicates how DA neurons change their activity during the 1DR task (e.g., Kawagoe et al., 2004; Matsumoto and Hikosaka, 2007; Bromberg-Martin et al., 2010), which is a pre-requisite for making a computational model of reinforcement learning.
In the following we propose a formal version of our theory of BG, where the BG “orients” the eyes to reward (Hikosaka, 2007). The present model accounts for reward-contingent oculomotor behavioral changes in normal monkeys, as well as, experimentally induced oculomotor behavioral changes.
We examined the possibility that the plasticity mediated by the DA actions on direct pathway MSNs and indirect pathway MSNs are responsible for the observed saccadic latency changes in normal and Parkinsonian monkeys. The model circuit was implemented with cell membrane differential equations (see Appendix) in Visual C++ using a PC. Our model implements only half of the hemisphere of the brain. This is because, during the left-ward saccades, for example, the right part of the BG is assumed to be active in learning because of the prevalent frontal eye filed (FEF)-to-striatum activation in the right hemisphere. This permits the striatal learning on the right side of the brain while the left side is not being affected. Because the 1DR alternates the left-side-reward and right-side-reward blocks of trials, in a symmetrical way, implementing one side with alternating blocks could represent the learning processes happening in both sides of the brain. Below, we will describe the basic architecture of the model, including how it generates saccades and how it is modulated by DA-independent and DA-dependent synaptic plasticity. For full details of the model equations, see the Appendix.
Our model simulates the data from 1DR task. In the task, a visual target was presented randomly on the left or right, and the monkey had to make a saccade to it immediately. Correct saccades were signaled by a tone stimulus after the saccade. Saccades to one position were rewarded, whereas saccades to the other position were not rewarded. The rewarded position was the same in a block of 20–30 consecutive trials and was then changed to the other position abruptly for the next block with no external instruction. Thus, the target instructed the saccade direction and also indicated the presence or absence of the upcoming reward.
While the monkey was performing 1DR task, the latency was consistently shorter for the saccade to reward target than for the saccade to no-reward target. Such a bias evolved gradually becoming more apparent as trials progressed (Figures 1B and 3B,E). The slow change in saccadic latency was particularly evident initially (Figure 3B). After experiencing 1DR task extensively the monkey became able to switch the bias rapidly (Figure 3E; Takikawa et al., 2004).
Figure 2A shows neuronal circuits in and around the BG included in our model. In the BG, there are two opposing pathways: (1) direct pathway which facilitates movement initiation and is under the control of D1 DA receptors, and (2) indirect pathway which suppresses movement initiation and is under the control of D2 DA receptors (Kravitz et al., 2010). For the initiation of saccades, several cortical areas including the FEF, upon receiving visual spatial information, send signals to the SC to prime a saccade to the visual cue (Sommer and Wurtz, 2001). They also send signals to the BG to facilitate or suppress saccades. The activation of the direct pathway facilitates saccade initiation by removal of inhibition (i.e., disinhibition): it inhibits SNr neurons which otherwise exert tonic inhibition on SC neurons. The disinhibition of SC neurons increases the probability of a saccade in response to the priming signal from the cortical areas (Hikosaka and Wurtz, 1985). In contrast, the activation of the indirect pathway suppresses the saccade: it inhibits GPe neurons which causes disinhibition of STN neurons and consequently enhancement of the SNr-induced inhibition of SC neurons. The enhanced inhibition of SC neurons reduces the probability of making a saccade in response to the priming signal from the cortical areas (Hikosaka and Wurtz, 1985).
Figure 2. Dopamine-mediated learning mechanisms in the striatum. DA-dependent LTP and LTD are labeled in red; DA-independent LTP and LTD are in blue. (A) Null state, where free saccades happen with no-task. Indirect pathway MSNs, which express D2 receptors, show weak DA-dependent LTD and DA-independent LTP. Direct pathway MSNs, which express D1 receptors, show DA-independent LTD. (B) Hypothesized D1 and D2 thresholds in relation to the levels of DA during big-reward and no (or small) reward trials. (C) In big-reward trials, the increased level of DA causes DA-dependent LTP in the direct pathway and enhances DA-dependent LTD in the indirect pathway. (D) In no-reward trials, the decreased level of DA causes an attenuation of DA-dependent LTD in the indirect pathway. The changes in DA level are assumed to coincide with the activation of the cortical input and the activation of the connected MSN neuron to enable the DA-dependent LTP and LTD (see the eligibility traces in Eqs 6–8). In both cases (C and D) DA-independent LTD in the direct pathway and DA-independent LTP in the indirect pathway remain unchanged, but their effects become relatively weak in big-reward trials (C) and relatively strong in no-reward trials (D). The red arrows indicate the amplitudes (large: 2 arrows, small: 1 arrow) and directions (up, down) of the change of neural activity compared to the null state shown in (A). Note, that even though the DA level in figure (A) and (D) are both under the threshold, LTD in the case of figure (D) happens more vigorously because of enabled eligibility (Eqs 6–8). The thickness of the connections indicates the resulting output in each module. Black and open circles indicate inhibitory and excitatory neurons, respectively. In (C) and (D), the GPb–LHb–SNc circuit is omitted for clarity. LTP/LTD, long-term potentiation/depression; GPb, border region of globus pallidus; LHb, lateral habenula.
Following the findings by Shen et al. (2008), our model implements several mechanisms to change the efficacy of cortico-striatal synapses. In short, there are increasing (LTP) and decreasing (LTD) “forces” of opposing processes in each pathway: In the direct pathway, the co-occurrence of pre- and post-synaptic activity, together with an increase in DA concentration above a threshold, induces LTP (DA-dependent LTP), while the co-occurrence of pre- and post-synaptic activity alone induces LTD (DA-independent LTD). In the indirect pathway, the co-occurrence of pre- and post-synaptic activity, together with DA concentration above a threshold, induces LTD (DA-dependent LTD), while the co-occurrence of pre- and post-synaptic activity alone induces LTP (DA-independent LTP).
We define “DA-dependent synaptic plasticity” as synaptic changes facilitated by over-the-threshold DA level. This situation occurs mostly during positive learning experience when DA neurons burst phasically, notably due to changes in reward expectation (Figure 2C). In contrast, the DA-independent synaptic plasticity happens as an opposing process constantly antagonizing the “DA-dependent synaptic plasticity,” and becoming prominent whenever “DA-dependent synaptic plasticity” loses its strength. For this reason, DA-independent synaptic plasticity acts as a “forgetting” mechanism.
Figure 2A shows the BG circuit of the model in its no-task (null) state where the subject makes saccades without any DA modulation. It has been shown that DA affinity is higher for D2 receptors than for D1 receptors (Richfield et al., 1989; Jaber et al., 1996). Here, we hypothesize that the background level of DA concentration in the CD stays above the threshold of D2 receptor activation and below the threshold of D1 receptor activation (Figure 2B). Accordingly, during the no-task state, indirect pathway MSNs are under the influence of D2-mediated LTD in addition to DA-independent LTP, while direct pathway MSNs only experience DA-independent LTD (Figure 2A). Note in the figures, DA-dependent LTP and LTD are shown in red while DA-independent processes are shown in blue. Also, the model circuit includes the recently identified lateral habenula (LHb; Matsumoto and Hikosaka, 2007)and LHb-projecting neurons in the GPb (Hong and Hikosaka, 2008) that have been shown to participate in reinforcement learning by modulating DA neurons in the substantia nigra pars compacta (SNc) and the ventral tegmental area.
When the animal detects a signal indicating an upcoming reward, DA neurons exhibit a short burst of spikes (Eq. 17), causing a phasic increase in the concentration of DA in the CD which temporarily exceeds the threshold of D1 receptors (Figure 2C). This phasic elevation of DA concentration, together with co-occurrence of pre- and post-synaptic activations, leads to the emergence of LTP in the direct pathway and an enhancement of LTD in the indirect pathway. Following the DA-induced changes in either the direct or indirect pathway, SNr neurons are inhibited and therefore SC neurons are activated (through disinhibition), leading to the facilitation of the saccade toward the target (Figure 2C). The changes in activity through the direct or indirect pathway are illustrated by the directions of arrows (upward: increase, downward: decrease). Note that the direction of arrows remains unchanged after an excitatory connection (shown by open “cell body” with an arrow ending, as in STN–SNr connection), but reverses after an inhibitory connection (filled “cell body” with a rectangular ending, as in CD–GPe connection).
When the animal detects a signal of no-reward, the level of DA in CD will go below the threshold of D2 receptors (Figure 2D). In the indirect pathway this leads to an attenuation of LTD leaving DA-independent LTP intact. In the direct pathway, this leads to only DA-independent LTD. As a result, the activity of SNr neurons increases and the saccadic eye movement toward the target is suppressed (Figure 2D).
After experiencing 1DR task extensively the monkey became able to switch the saccade latency bias more rapidly (Takikawa et al., 2004) after the position-reward contingency is reversed. This raises the possibility that, in addition to the BG-based learning processes described above, a switching-like process emerges in the brain and contributes to the quick saccade latency changes. Indeed, it is reported that the reward-dependent change in saccade latency occurs by inference (Watanabe and Hikosaka, 2005). For example, suppose the task changed from the left-reward block to the right-reward block. On the first trial of a new block, the monkey made a saccade to the left target and did not receive a reward. This allowed the monkey to detect that the block had changed, and to infer that the reward had switched from the left side to the right side. Then the monkey immediately made a rapid (short latency) saccade to the right target, even though the monkey had not yet received a reward from that target. Furthermore, such inference-dependent activities have been observed in all the neurons tested for the circuit diagram shown in Figure 2: CD neurons (Watanabe and Hikosaka, 2005) as well as GPb, LHb, and DA neurons (Bromberg-Martin et al., 2010).
We hypothesize that this rapid switching is enabled by a population of neurons on each side of the hemisphere which becomes active when a reward is available on the contralateral side but not on the ipsilateral side. Such neurons, which we hereafter call “reward-category neurons,” are assumed to have excitatory connections to neurons in the FEF and to the direct pathway MSNs on the same side. This assumption is based on our previous findings: presumed projection neurons in the CD (Lauwereyns et al., 2002; Takikawa et al., 2002; Watanabe et al., 2003) as well as neurons in the FEF (Ding and Hikosaka, 2006) ramp-up their activity when a reward was expected on the contralateral side. Further, many SNr neurons decrease their activity selectively when a reward is expected on the contralateral side (Sato and Hikosaka, 2002), suggesting that the reward-category neurons excite direct pathway MSNs, but not indirect pathway MSNs. However, where the reward-category neurons are located is unknown, and it is possible that the reward-category activity emerges from interactions of neurons in the cerebral cortex and the BG.
The model implements the reward-category neurons, tentatively, as a module in the cerebral cortex, as illustrated in Figure 3D. When a reward is expected on the left side, for example, the reward-category neurons in the right cortex (red circle in Figure 3D; CgRWD in Eq. 4 in Appendix) will ramp-up their activity before the execution of a saccade. This will excite the right FEF neuron and direct pathway MSNs, therefore boosting the activity of these neurons. Note that there will be no boost of activity in the FEF and MSNs in the left (ipsilateral) hemisphere. Due to this construction, the striatum receives strong cortal inputs boosted by the excitatory reward-category neurons only during contralateral reward trials. The reward-category activity also affects the SC directly via the FEF–SC excitatory connection (Figure 2) making the SC react more rapidly during reward trials (Ikeda and Hikosaka, 2003; Isoda and Hikosaka, 2008). Our model hypothesizes that the combination of cortical switching and trial-to-trial updates of learning in the BG explain the change of saccadic latencies during the 1DR task (Bromberg-Martin et al., 2010). Note that we use the word “switching” only to mean the inferential abrupt change in the cortical circuit, and the ensuing abrupt behavioral change in saccadic latency in the second trial of a block. The plasticity in the FEF–MSN synapse is assumed to contribute to the gradual changes in saccadic latency reaching an asymptote. In other words, the change in saccade latency after reversal of position-reward contingency is caused by the activity of “reward-category” and synaptic plasticity in the BG.
Figure 3. Experience-dependent emergence of a switching mechanism that allows rapid changes of saccade latency in response to the change in reward location: before (A–C) and after (D–F) sufficient experience of the 1DR task. We hypothesize the presence of “reward-category neurons” (RWD), a key driver of the switching, that have excitatory connections to FEF neurons and direct pathway MSNs in the CD in the same hemisphere. They would become active before target onset selectively when a reward is expected on the contralateral side (see Figure 4), an assumption based on experimental observations of neuronal activity in the FEF, CD, SNr, and SC. Before sufficient experience of the 1DR task (A–C), the saccade latency changes gradually in both the small-to-big-reward transition [red in (B,C)] and the big-to-small-reward transition [blue in (B,C)] similarly by experimental observation (B) and computer simulation (C). The saccade latency data in (B) is from monkeys C, D, and T. After sufficient experience of the 1DR task (D–F), the saccade latency changes quickly as shown in experiments (E) and computer simulation (F). This is mainly due to the additional excitatory input from the reward-category neurons. Note, however, that the decrease in saccade latency in the small-to-big-reward transition [red in (E,F)] is quicker than the increase in saccade latency in the big-to-small-reward transition [blue in (E,F)]. This asymmetry is due to the asymmetric learning algorithm operated by two parallel circuits in the basal ganglia illustrated in Figure 2. Figure (E) from Matsumoto and Hikosaka (2007).
In the following, we first simulate the eye movements in the 1DR showing the baseline performance of the model. Next, we simulate the influence of D1 and D2 antagonist injections in the CD showing how the DA-mediated learning leads to behavioral manifestation. The simulation results for PD are presented to show the potential application of our model to understanding neurological disorders.
In one block of trials in the 1DR task a saccade to a given target is followed by a reward, and in the next block of trials the saccade to the same target is followed by no-reward (Figure 1A). Hence, in each block of trials the monkey learns a new position-reward association, and the learning is evidenced as changes in the saccade reaction time (or latency): decrease in saccade latency for the rewarded target and increase in saccade latency for the unrewarded target (Figures 3B,E). The changes in saccade latency became quicker as the monkey experienced 1DR task extensively (compare Figure 3B and Figure 3E; Takikawa et al., 2004).
Our model simulates these changes in saccade latency reasonably well (Figures 3C,F).
In the early stage of the monkey’s experience with the 1DR task, the saccade latency decreased gradually after a small-to-big-reward transition and increased gradually after a big-to-small-reward transition (Figure 3B). These slow changes in saccade latency are simulated by the model (Figure 3C) by assuming that there is no-reward-category activity (Figure 3A), which would act as a switching mechanism. In other words, these changes in saccade latency, at this stage, are controlled solely by the striatal plasticity mechanisms which are described in the Section “Learning in the Basal Ganglia.”
After sufficient experience with the 1DR task, the changes in saccade latency occur more quickly (Figure 3E). This was simulated by assuming the emergence of reward-category neurons which, before the target comes on, exert an excitation on FEF neurons as well as on the direct pathway MSNs when a reward is expected on the contralateral side (see Switching Mechanism).
The performance of our model in an advanced stage of learning (Figure 3D) is illustrated in Figure 4. Our model combines two kinds of neuronal mechanisms: (1) learning in the BG (i.e., plasticity at cortico-striatal synapses), and (2) switching mechanism (i.e., reward-category activity). Here, the activity of individual neurons (or brain areas) is compared between two reward contexts: a contralateral saccade is followed by a reward (Figure 4A) and no-reward (Figure 4B). Only the contralateral saccade is considered because the neuronal network simulates one hemisphere and is assumed to control only contralateral saccades.
Figure 4. Simulated neural components of the model performing reward and no-reward trials of 1DR task. In reward trials (A) the reward-category unit (REW category) ramps up its activity shortly after the presentation of the fixation point. The activity shuts off in response to the burst activity of DA unit (DA) signaling the reward value of the target. The FEF unit combines the tonic reward-category activity and the phasic target signal. In the BG, both the direct pathway MSN unit (D1) and the indirect pathway MSN unit (D2) receive an input from the FEF. The direct pathway MSN unit (D1), in addition, receives an input directly from the reward-category unit and therefore shows larger ramping activity than the indirect pathway MSN unit (D2). The activity of the direct pathway MSN unit (D1) is further enhanced by DA-dependent LTP, which is triggered by the DA burst, and mediated by D1 receptors. This results in a stronger disinhibition of the SC by the SNr leading to a stronger activity in the SC. In contrast, the activity of indirect pathway MSN unit (D2) is further depressed by DA-dependent LTD, which is triggered by the DA burst, and mediated by D2 receptors. This results in the suppression of the excitatory input from the STN to the SNr, further enhancing the SC activity. The combined effects from the direct and indirect pathways lead to a shorter latency saccade (see the arrow head on top, indicating the time of saccade initiation). In no-reward trials (B) the activity of the reward-category unit is much weaker, thus lowering the activity of the FEF unit and the direct pathway MSN unit (D1). The activity of the direct pathway MSN unit (D1) is further depressed by DA-independent LTD. In contrast, the activity of D2 MSN increases because DA-dependent LTD is attenuated due to the “pause” of DA activity (DA) and thus is dominated by DA-independent LTP. The combined effects from the direct and indirect pathways lead to a weaker activation of the SC unit and hence a longer latency saccade. The scale of all the ordinate axes is from 0 to 1.
According to our model, the learning in the BG controls, mainly, the phasic response component to target onset. The response of direct pathway MSNs (D1) to the post-target input from the FEF increases when the contralateral saccades were rewarded repeatedly (Figure 4A); this is mainly due to the development of DA-dependent LTP at the corticostriatal synapses. In contrast, the response decreases when the contralateral saccades were unrewarded repeatedly (Figure 4B); this is mainly due to the development of DA-independent LTD at the corticostriatal synapses. Such reward-facilitated visual responses in CD neurons have been reported repeatedly using 1DR task (Kawagoe et al., 1998, 2004), although it is unknown if they were direct pathway MSNs. These changes in the post-target response of direct pathway MSNs lead to a stronger disinhibition of SC neurons via SNr neurons on reward trials (Figure 4A) than no-reward trials (Figure 4B).
Roughly opposite effects occur through the indirect pathway. The response of indirect pathway MSNs (D2) to the post-target input from the FEF decreases when the contralateral saccades were rewarded repeatedly, mainly due to the development of DA-dependent LTD at the corticostriatal synapses (Figure 4A). In contrast, the response increases when the contralateral saccades were unrewarded repeatedly, mainly due to the development of DA-independent LTP at the corticostriatal synapses (Figure 4B). Such reward-suppressed visual responses in CD neurons have been reported (Kawagoe et al., 1998; Watanabe et al., 2003), although it is unknown if they were indirect pathway MSNs. These changes in the post-target response of indirect pathway MSNs lead to a stronger inhibition of SC neurons via GPe and STN neurons on no-reward trials (Figure 4B) than reward trials (Figure 4A).
The effects of the switching mechanism mainly lead to tonic changes in neuronal activity before target onset. When a reward is expected on the contralateral side, the reward-category neurons (RWD category in Figure 4) ramp-up their activity shortly after the presentation of a fixation point (Figure 4A). FEF neurons (FEF in Figure 4) receive excitatory input from the reward-category neurons in addition to a phasic excitatory input encoding the onset of the target (Ding and Hikosaka, 2006). In the BG, both the D1-mediated direct pathway (D1) and the D2-mediated indirect pathway (D2) receive the reward-category signal from the FEF. However, direct pathway MSNs (D1) also receive an excitatory input directly from the reward-category neurons and therefore show larger ramping activity than indirect pathway MSNs (D2; Lauwereyns et al., 2002). This results in a ramp-down of the activity of SNr neurons (SNr; Sato and Hikosaka, 2002) before target onset. In consequence, SC neurons receive the reward-category signal via two routes: (1) pre-target tonic decrease in SNr-induced inhibition (disinhibition), and (2) pre-target tonic increase in FEF-induced excitation. When a reward is not expected on the contralateral side, the reward-category neurons are less active (Figure 4B) and therefore the pre-target facilitation is weak in SC neurons. Indeed, SC neurons exhibit such pre-target ramp-up activity which is stronger when the contralateral saccade was rewarded than unrewarded (Ikeda and Hikosaka, 2003; Isoda and Hikosaka, 2008).
In summary, the learning mechanism and the switching mechanism, when working together, enable quick adaptation of oculomotor behavior depending on expected reward. It is important to note that the two mechanisms interact in a mutually facilitatory manner. First, the reward-category activity facilitates the development of DA-dependent LTP in direct pathway MSNs (Figure 4A) because it increases the likelihood of the co-occurrence of the pre-synaptic activity (i.e., FEF activity) and the post-synaptic activity (i.e., MSN activity) which is thought (and here assumed) to be a pre-requisite of this type of LTP (Wickens, 2009). Second, the changes in activity of DA neurons could modulate the reward-category activity. For example, when a reward is expected after a contralateral saccade, DA neurons exhibit a burst of spikes which then would cause LTP in the cortico-striatal synapses in direct pathway MSNs carrying the reward-category activity, leading to an enhancement of the reward-category activity in the MSNs. In contrast, the reward-category activity in indirect pathway MSNs would be suppressed because the same DA activity would cause LTD. On the other hand, the reward-category activity in indirect pathway MSNs would be facilitated when no-reward is expected because the DA neurons pause and therefore the DA-dependent LTD becomes weaker and instead DA-independent LTP becomes dominant. Such changes in the reward-category activity are evident in Figure 4 by comparing the pre-target activity in direct pathway MSNs (D1) and indirect pathway MSNs (D2) between the two reward contexts (Figures 4A,B). In short, the learning mechanism and the switching mechanism cooperate to enhance and accelerate the reward-dependent bias in saccade latency.
Our computational model has simulated reward-dependent oculomotor behavior successfully. Central to our model is the DA-dependent plasticity at the cortico-striatal synapses. Therefore, experimental manipulations of DA transmission in the striatum could provide critical tests of our model. Such experiments were done by Nakamura and Hikosaka (2006). They showed that the saccadic latency in the 1DR task changed differently and selectively after injections of D1 antagonist and D2 antagonist in the CD. Below, we will simulate the behavioral effects of these experimental manipulations based on the model.
After the D1 antagonist injection, latency increased for the saccades made toward the reward position without affecting the saccades toward the no-reward position (Figure 5C left). Simulation results correctly follow this trend (Figure 5C right). As explained above (Figure 2B) the model assumes that, in a normal condition, the threshold for D1 receptor activation (hereafter called “D1 threshold”) is above the default concentration of DA level in the CD, and the threshold for D2 receptor activation (hereafter called “D2 threshold”) is below it. After the injection of the D1 antagonist, the D1 threshold increases significantly while the D2 threshold remains unchanged (compare Figure 5D with Figure 2C). This leads to a selective suppression of DA-dependent LTP in the direct pathway which would be triggered by a phasic increase of DA concentration in reward trials (Figure 5D). In consequence, the activation of direct pathway MSNs by the reward-predicting visual input becomes weaker. In turn, this causes SNr neurons to be less inhibited, SC saccadic neurons to be less disinhibited, and saccades to occur at longer latencies.
Figure 5. Influence of D1 antagonist on saccadic latency. (A) Trial-by-trial changes in the latency of contralateral saccades, before (black) and after (red) injection of a D1 antagonist into the CD. Data are from Nakamura and Hikosaka (2006, p. 60). (B) Simulated trial-by-trial changes in saccade latency. (C) After D1 antagonist injection, average saccade latency increased in big-reward trials, but not in small-reward trials. The experimental data was replicated by computer simulation. (D) Hypothesized mechanism of the effect of D1 antagonist in big-reward trials. The D1 antagonist effectively elevates the D1 threshold and therefore induces a smaller-than-usual LTP in the direct pathway MSNs, whereas the indirect pathway MSNs are unaffected. The attenuated LTP leads to a weaker activation of the SC and therefore a longer latency saccade. (E) Hypothesized mechanism of the effect of D1 antagonist in big-reward trials. The DA level remains below the D1 threshold similarly to the control condition (Figure 2D) and therefore the saccade latency is not changed.
In the case of no-reward trials, the situation remains unchanged after D1 antagonist injection because the D1 threshold, while elevated by the D1 antagonist, remains higher than the DA concentration (compare Figure 5E with Figure 2D) and the D1 antagonist does not affect the D2 threshold. Consequently, the saccade latency remains unchanged in no-reward trials (Figure 5C right), similar to the experimental data (Figure 5C left).
In the preceding section we showed that our model can simulate the time course of saccade latency changes during the 1DR task (Figure 3). As seen in Figure 5A the D1 antagonist injection in the CD alters the saccade latency over time and our model simulates this change (Figure 5B).
In contrast, after the D2 antagonist injection in the CD, the saccadic latency increased selectively in no-reward trials (Nakamura and Hikosaka, 2006). Our model explains this change as a consequence of the increased threshold for the D2 receptor activation (Figure 6E). Note that in the normal condition, the threshold, of the D2 receptors, is assumed to be below the level of DA concentration in the striatum (Figure 2D). After the injection of the D2 antagonist, the D2 threshold increases significantly while the D1 threshold remains unchanged, which leads to selective changes in the indirect pathway. This change will not grossly affect saccades in reward trials because DA concentration is assumed to exceed both D1 and D2 thresholds (Figure 6D). In no-reward trials, however, the change in the D2 threshold affects processes in the indirect pathway (compare Figure 6E with Figure 2D). This is because the removal of DA-dependent LTD enhances the activity of indirect pathway MSNs. The increased output in the indirect pathway leads to an increase in the SNr-induced inhibition on SC saccadic neurons, leading to longer saccade latencies, as shown in the simulated results in Figure 6C right. These results are similar to the experimental data (Figure 6C, left). The simulation also replicates the trial-by-trial changes in saccade latencies and their alteration by D2 antagonist (compare Figure 6A with Figure 6B).
Figure 6. Influence of D2 antagonist on saccadic latency. (A) Trial-by-trial changes in the latency of contralateral saccades, before (black) and after (blue) injection of a D2 antagonist into the CD. Data are from Nakamura and Hikosaka (2006). (B) Simulated trial-by-trial changes in saccade latency. (C) After D1 antagonist injection, average saccade latency increased in small-reward trials, but not in big-reward trials. The experimental data was replicated by computer simulation. (D) Hypothesized mechanism of the effect of D2 antagonist in big-reward trials. The phasic increase in the DA level exceeds both the D1 and D2 thresholds, although the D2 antagonist elevates the D2 threshold, and therefore the saccade latency remains largely unchanged. (E) Hypothesized mechanism of the effect of D2 antagonist in small-reward trials. The elevated D2 threshold eliminates the DA-dependent LTD in the indirect pathway MSNs and therefore potentiates the SNr-induced inhibition of the SC, leading to a longer latency saccade.
Our model predicts altered reward-related learning in PD subjects. We first modeled the changes in synaptic plasticity that occur during PD. In animal models of PD, the synaptic plasticity of the BG is disrupted (Figure 7A) such that LTP is induced in indirect pathway MSNs (green dots) and LTD is induced in direct pathway MSNs (purple dots) after stimulation protocols that normally induce LTD and LTP, respectively (Shen et al., 2008). Our model simulates the reversal of synaptic plasticity (Figure 7C) using several assumptions illustrated in Figure 7B. We assume: (1) DA concentration in the striatum of the PD patient is reduced (indicated by the low levels of the purple curves in Figure 7B) by about 84%, as shown by Fearnley and Lees (1991), (2) D1 and D2 receptors become hypersensitive as indicated by the low levels of the red and blue dashed lines in Figure 7B (e.g., Gerfen, 2003), and (3) a small number of DA neurons remain functional so that DA concentration increases and decreases slightly in response to big- and small-reward cues (indicated by up/down deviations of the purple curves from the flat background level in Figure 7B).
Figure 7. Simulation of disrupted plasticity in Parkinson’s disease (PD). (A) Disrupted plasticity in MSNs (green and purple dots) in a rat PD model. When input stimulation was followed by excitation of a MSN repeatedly, the response of the MSN to the input changed gradually, in the directions opposite to control subjects. Data are from Shen et al. (2008), adapted with permission. (B) Hypothesized changes in the DA level and D1/D2 thresholds in PD and PD with l-DOPA. See text for details. (C) Simulated plasticity in the direct pathway MSNs (D1) and the indirect pathway MSNs (D2) in PD subjects performing the 1DR task. The simulation shows disrupted plasticity in PD, which is similar to that shown in the rat PD model (A). Note that the magnitude of the plasticity is larger for no-reward trials than for reward trials. (D) Simulated saccade latency in PD subjects with no treatment (PD) and PD subjects with l-DOPA. See text for further explanations.
Given these assumptions, our model predicts that direct pathway MSNs undergo LTD during either reward or no-reward trials (orange curves in Figure 7C). This is because DA-dependent LTP, which is rendered minimal due to the low DA level, is dominated by DA-independent LTD. In reward trials, however, the slight increase in the DA level can trigger weak LTP because the D1 threshold is lowered due to hypersensitivity (Figure 7B). As a consequence, the net LTD is bigger after no-reward trials than reward trials (orange curves in Figure 7C).
An opposite reaction occurs in indirect pathway MSNs. They undergo LTP in either reward or no-reward trials (black curves in Figure 7C) because DA-dependent LTD, which is rendered minimal due to the low DA level, is dominated by DA-independent LTP. In reward trials, however, the slight increase in the DA level can trigger weak LTD because the D2 threshold is lowered due to hypersensitivity (Figure 7B). In consequence, the net LTP is is bigger after no-reward trials than reward trials (black curves in Figure 7C).
Our model predicts that these changes in synaptic plasticity would cause several changes in the pattern of behavior during the 1DR task (Figure 7D). The results indicate that in reward trials the saccadic latency in the PD subject (red curve in Figure 7D) is longer than in the normal subject (red curve in Figure 3E). The saccade latencies during no-reward trials are even more sluggish as shown by the blue curve in Figure 7D. Interestingly, while both latencies are longer than those of normal subjects, the latencies during reward trials are still shorter than those in no-reward trials in PD patients. This means that even with the reversed directions of plasticity, the subjects show correct direction of learning.
Our model also predicts the impact of L-DOPA in the PD subject. Figure 7B illustrates the hypothesized learning situation in PD with L-DOPA, showing the elevated DA level (green trace) that enables positive reinforcement learning with the assistance of increased sensitivity (e.g., Gerfen, 2003). Our model then predicts that the saccade latency decreases in rewarded trials (Figure 7D, pink line), but not in no-reward trials (Figure 7D, green line), nearly reaching the level of normal experienced subjects (Figures 3B,C). One noticeable difference is that the latency in reward trials reaches its plateau more slowly than in normal subject. This is because of the still inefficient learning in the BG compared to that in normal subjects.
This study explored the possible neuronal mechanisms underlying adaptive changes in oculomotor behavior in response to the change of reward locations. We did so by constructing a computational model and simulating animal’s normal and experimentally manipulated behaviors. Our model, which combines a learning mechanism and a switching mechanism in the cortico-striatal circuit, simulates experimental results obtained using a saccade task with positional reward bias (1DR task) reasonably well. In the following we discuss possible physiological mechanisms presumed to be the bases of these phenomena, as well as, the limitations of our model.
Basal ganglia are well known for their involvement in motor and cognitive functions. It is also known that many neurons in the BG are sensitive to expectation of reward (see Hikosaka et al., 2006; Schultz, 2006, for review). The 1DR task provides quantitative data that is suitable for testing a computational model of reward-based learning. After sufficient experience in the 1DR task, monkeys are able to reverse the positional bias in saccade latency fairly quickly. It may be suggested that such a quick reversal in behavior is achieved by a switching mechanism. Interestingly, the increase in saccade latency after the big-to-small-reward transition is slower than the decrease in saccade latency after the small-to-big-reward transition (Lauwereyns et al., 2002; Watanabe and Hikosaka, 2005; Nakamura and Hikosaka, 2006; Matsumoto and Hikosaka, 2007; Nakamura et al., 2008; Figure 3E). Such an asymmetry in the saccade latency change is best explained by a learning mechanism that distinguishes the two directions of saccade latency changes; it is unlikely to be explained solely by a switching mechanism. Indeed, our study using computational modeling and simulation indicates that a combination of a learning mechanism and a switching mechanism can explain the adaptive oculomotor behavior in experienced animals, while the learning mechanism alone can explain the adaptive oculomotor behavior in less experienced animals. More specifically, the asymmetric change in saccade latency can be explained by an asymmetric learning algorithm operated by two parallel circuits in the BG: D1-modulated direct pathway and D2-modulated indirect pathway. The direct pathway seems to express D1-mediated LTP (Reynolds et al., 2001; Calabresi et al., 2007), whereas the indirect pathway seems to express D2-mediated LTD (Gerdeman et al., 2002; Kreitzer and Malenka, 2007). Explained more mechanistically, D1-mediated LTP and D2-mediated LTD happen actively when the DA level is high (i.e., in response to the reward-predicting target), while the DA-independent LTD in the direct pathway and the DA-independent LTP in the indirect pathway happen rather passively when the DA level is low (i.e., in response to no-reward-predicting target). This bias of speeds in plasticity is suggested to result in faster acquisition and slower forgetting of the motivated behavior expressed as saccade latency change.
We have constructed a model that implements a lumped LTP/LTD, which simplifies underlying complicated intracellular processes. Here we discuss some probable mechanisms, underlying these synaptic changes. The mechanisms of the synaptic plasticity in the BG have been studied extensively, yet there are conflicting experimental results (for reference, see Calabresi et al., 2007; Surmeier et al., 2007). It is shown that DA-mediated D1 receptor signaling promotes LTP (Reynolds et al., 2001; Calabresi et al., 2007) whereas D2 signaling induces LTD (Gerdeman et al., 2002; Kreitzer and Malenka, 2007). As adaptive learning theories require, however, the plasticity in these direct and indirect pathways seem to be bidirectional. For example, Shen et al. (2008) have shown that D1 and D2 receptor-bearing striatal MSNs had both LTP and LTD.
In indirect pathway MSNs, D2 receptor activation is known to promote dephosphorylation processes in a variety of channels including AMPA and NMDA and Na+ channels by suppressing adenylyl cyclase. It has also been reported that DA-independent LTP (or repotentiation) happens in indirect pathway MSNs when the afferents are stimulated with a following post-synaptic depolarization (Shen et al., 2008). This LTP process seems to be dependent on adenosine A2a receptors, which couple to the same second messenger cascades as D1 receptors, and are robustly and selectively expressed by indirect pathway MSNs (Schwarzschild et al., 2006). It was demonstrated that antagonizing these receptors (not D1 receptors) disrupted the induction of LTP in indirect pathway MSNs. This type of LTP seems to be NMDA receptor dependent and post-synaptic (Shen et al., 2008).
In direct pathway MSNs, D1 receptor activation by DA induces LTP by stimulating adenylyl cyclase therefore promoting phosphorylation processes of a variety of channels, such as AMPA and NMDA and Na+ channels. Note that D1 and D2 receptors target the same chemical agent, adenylyl cyclase, in opposite ways. (Picconi et al., 2003) showed that LTD (or synaptic depotentiation) seen in control animals was absent in the L-DOPA treated animals that had too much phospho[Thr34]-DARPP-32, an inhibitor of protein phosphatase. They reported that this DA-mediated phosphorylation pathway was responsible for the persistent LTP in the cortico-striatal synapses in their L-DOPA subjects, leading to dyskinesia. DA-independent LTD in direct pathway MSNs has also been demonstrated (Wang et al., 2006; Shen et al., 2008). Notably, this type of LTD in direct pathway MSNs seems to be dependent upon post-synaptic signaling of endocannabinoid CB1 receptors (Wang et al., 2006; Shen et al., 2008).
These LTP and LTD processes in direct and indirect pathway MSNs seem to depend, directly or indirectly, on the level of DA in the BG. For example, when DA was depleted, the direction of plasticity changed dramatically: direct pathway MSNs showed only LTD and indirect pathway MSNs showed only LTP regardless of the protocol used (Shen et al., 2008). Picconi et al.’s (2003) report of the chronic high level L-DOPA-induced loss of LTD capability at the cortical-MSN synapses and ensuing behavioral symptoms is another piece of evidence pointing to the importance of DA in the synaptic learning of this region. In summary, experimental results suggest that direct and indirect pathway MSNs express both LTP and LTD, and their direction of plasticity is dependent on the level of DA.
The simulation results of our model predict that while having significant learning deficit, PD patients still show some learning, consistent with the literature (e.g., Behrman et al., 2000; Muslimovic et al., 2007). To be more rigorous, the increments of saccadic latencies in Figure 7D (e.g., ∼125% of normal reward trials shown in Figure 7D red curve) are similar to the known increased saccadic latencies (between 120 and 160% of normal subjects, depending on severity) in human PD patients (White et al., 1983) and MPTP monkeys (Tereshchenko et al., 2002). The simulated saccadic latencies in no-reward trials are also slower than the counterparts of the normal subjects (∼110% of normal subjects’ latency during no-reward trials, the blue curve in Figure 7D).
Our model also predicts the impact of L-DOPA in the PD subject. As Figure 7D shows, the simulated PD subject with L-DOPA shortens the saccadic latency compared to the non-medicated counterpart, consistent with previous reports (Highstein et al., 1969; Gibson et al., 1987; Vermersch et al., 1994). In contrast, a recent study reports that in well medicated subjects L-DOPA actually slowed down prosaccadic latency (Hood et al., 2007). More importantly, this longer latency in prosaccades was accompanied by an increased correct rate in anti-saccades (reduced fast reflexive prosaccades), where the impulsive tendency to make a saccade to the visual stimulus needs to be suppressed. It is likely that this L-DOPA-induced elongation of saccadic latency was due to an enhanced compensatory cortical mechanism in PD (e.g., Cunnington et al., 2001; Mallol et al., 2007) to suppress impulsive reflexive saccades as the task demanded. Our current model is focused on modulation of saccades by reward-oriented biases rather than by such task-dependent speed–accuracy tradeoffs, which might be implemented by different BG circuit mechanisms (Lo and Wang, 2006).
It was reported that, compared to normal subjects, PD subjects on L-DOPA medication are better in positive learning and worse in negative learning, and that PD subjects off medication are better in negative learning and worse in positive learning (Frank et al., 2004). Assuming that DA agonist raises the DA level slightly over the optimal range (i.e., over the D1 and D2 thresholds), our model predicts that a slight elevation over the optimal range could drive the system to over learn, resulting in faster than normal saccadic latencies both in the reward and no-reward trials (result not shown). This conclusion directly parallels the conclusion by Frank et al. (2004).
One interesting question arises in our model: Why are there two pathways (the direct and indirect pathways) in the BG even though their jobs could apparently be done by just one pathway? It is possible that the two pathways exist to flexibly control the output of the BG. In other words, while many situations require cooperative operations of the direct and indirect pathways, some other situations may call for separate operations of these two pathways. For example, if an animal meets a conflicting situation, such as, food is in sight while a predator is also nearby, an indirect-pathway-specific “no go” command may save the animal from the recklessly daring situation. Another possible benefit of having two separate pathways comes from the connectional anatomy of the BG. In the rat, the indirect pathway of the BG receives a majority of its inputs from neurons in deep layers of the cerebral cortex which also project to the motoneurons in the spinal cord, whereas the direct pathway receives a majority of its inputs from neurons in the intermediate layers of the cerebral cortex, some of whose axons also contact contralateral BG (Lei et al., 2004). Assuming that this scheme holds true for primates, it is conceivable that an output from a cortical area is used for a motor command by its direct connection to the spinal cord. At the same time, its corollary connection to the indirect pathway may terminate the command once executed. This kind of mechanism may be beneficial especially when the animal needs to execute several sequential actions in a row. In conclusion, while many daily activities may make use of the synergistic learning involving both the direct and indirect pathways, some other occasions may call for learning in just one of the pathways. This dual pathway design of the BG may make motor behavior more flexible.
The authors declare that the research was conducted in the absence of any commercial or financial relationships that could be construed as a potential conflict of interest.
We are grateful to M. Isoda, L. Ding for providing data (monkey T and D, respectively), C. R. Hansen, E. S. Bromberg-Martin for helpful comments. This work was supported by the intramural research program of the National Eye Institute.
Apicella, P., Scarnati, E., Ljungberg, T., and Schultz, W. (1992). Neuronal activity in monkey striatum related to the expectation of predictable environmental events. J. Neurophysiol. 68, 945–960.
Behrman, A. L., Cauraugh, J. H., and Light, K. E. (2000). Practice as an intervention to improve speeded motor performance and motor learning in Parkinson’s disease. J. Neurol. Sci. 174, 127–136.
Breitenstein, C., Korsukewitz, C., Floel, A., Kretzschmar, T., Diederich, K., and Knecht, S. (2006). Tonic dopaminergic stimulation impairs associative learning in healthy subjects. Neuropsychopharmacology 31, 2552–2564.
Bromberg-Martin, E. S., Matsumoto, M., Hong, S., and Hikosaka, O. (2010). A pallidus-habenula-dopamine pathway signals inferred stimulus values. J. Neurophysiol. 104, 1068–1076.
Brown, J. W., Bullock, D., and Grossberg, S. (2004). How laminar frontal cortex and basal ganglia circuits interact to control planned and reactive saccades. Neural Netw. 17, 471–510.
Calabresi, P., Picconi, B., Tozzi, A., and Di Filippo, M. (2007). Dopamine-mediated regulation of corticostriatal synaptic plasticity. Trends Neurosci. 30, 211–219.
Costa, R. M., Cohen, D., and Nicolelis, M. A. (2004). Differential corticostriatal plasticity during fast and slow motor skill learning in mice. Curr. Biol. 14, 1124–1134.
Cunnington, R., Lalouschek, W., Dirnberger, G., Walla, P., Lindinger, G., Asenbaum, S., Brucke, T., and Lang, W. (2001). A medial to lateral shift in pre-movement cortical activity in hemi-Parkinson’s disease. Clin. Neurophysiol. 112, 608–618.
Darbaky, Y., Baunez, C., Arecchi, P., Legallet, E., and Apicella, P. (2005). Reward-related neuronal activity in the subthalamic nucleus of the monkey. Neuroreport 16, 1241–1244.
Deng, Y. P., Lei, W. L., and Reiner, A. (2006). Differential perikaryal localization in rats of D1 and D2 dopamine receptors on striatal projection neuron types identified by retrograde labeling. J. Chem. Neuroanat. 32, 101–116.
Ding, L., and Hikosaka, O. (2006). Comparison of reward modulation in the frontal eye field and caudate of the macaque. J. Neurosci. 26, 6695–6703.
Ell, S. W., Weinstein, A., and Ivry, R. B. (2010). Rule-based categorization deficits in focal basal ganglia lesion and Parkinson’s disease patients. Neuropsychologia 48, 2974–2986.
Fearnley, J. M., and Lees, A. J. (1991). Ageing and Parkinson’s disease: substantia nigra regional selectivity. Brain 114(Pt 5), 2283–2301.
Fino, E., Glowinski, J., and Venance, L. (2005). Bidirectional activity-dependent plasticity at corticostriatal synapses. J. Neurosci. 25, 11279–11287.
Frank, M. J., Samanta, J., Moustafa, A. A., and Sherman, S. J. (2007). Hold your horses: impulsivity, deep brain stimulation, and medication in Parkinsonism. Science 318, 1309–1312.
Frank, M. J., Seeberger, L. C., and O’Reilly, R. C. (2004). By carrot or by stick: cognitive reinforcement learning in Parkinsonism. Science 306, 1940–1943.
Gerdeman, G. L., Ronesi, J., and Lovinger, D. M. (2002). Postsynaptic endocannabinoid release is critical to long-term depression in the striatum. Nat. Neurosci. 5, 446–451.
Gerfen, C. R. (2003). D1 dopamine receptor supersensitivity in the dopamine-depleted striatum animal model of Parkinson’s disease. Neuroscientist 9, 455–462.
Gibson, J. M., Pimlott, R., and Kennard, C. (1987). Ocular motor and manual tracking in Parkinson’s disease and the effect of treatment. J. Neurol. Neurosurg. Psychiatr. 50, 853–860.
Highstein, S., Cohen, B., and Mones, R. (1969). Changes in saccadic eye movements of patients with Parkinson’s disease before and after L-dopa. Trans. Am. Neurol. Assoc. 94, 277–279.
Hikosaka, O. (2007). Basal ganglia mechanisms of reward-oriented eye movement. Ann. N. Y. Acad. Sci. 1104, 229–249.
Hikosaka, O., Nakamura, K., and Nakahara, H. (2006). Basal ganglia orient eyes to reward. J. Neurophysiol. 95, 567–584.
Hikosaka, O., Sakamoto, M., and Miyashita, N. (1993). Effects of caudate nucleus stimulation on substantia nigra cell activity in monkey. Exp. Brain Res. 95, 457–472.
Hikosaka, O., Sakamoto, M., and Usui, S. (1989). Functional properties of monkey caudate neurons. III. Activities related to expectation of target and reward. J. Neurophysiol. 61, 814–832.
Hikosaka, O., Takikawa, Y., and Kawagoe, R. (2000). Role of the basal ganglia in the control of purposive saccadic eye movements. Physiol. Rev. 80, 953–978.
Hikosaka, O., and Wurtz, R. H. (1983). Visual and oculomotor functions of monkey substantia nigra pars reticulata. IV. Relation of substantia nigra to superior colliculus. J. Neurophysiol. 49, 1285–1301.
Hikosaka, O., and Wurtz, R. H. (1985). Modification of saccadic eye movements by GABA-related substances. I. Effect of muscimol and bicuculline in monkey superior colliculus. J. Neurophysiol. 53, 266–291.
Hong, S., and Hikosaka, O. (2008). The globus pallidus sends reward-related signals to the lateral habenula. Neuron 60, 720–729.
Hood, A. J., Amador, S. C., Cain, A. E., Briand, K. A., Al-Refai, A. H., Schiess, M. C., and Sereno, A. B. (2007). Levodopa slows prosaccades and improves antisaccades: an eye movement study in Parkinson’s disease. J. Neurol. Neurosurg. Psychiatr. 78, 565–570.
Ikeda, T., and Hikosaka, O. (2003). Reward-dependent gain and bias of visual responses in primate superior colliculus. Neuron 39, 693–700.
Isoda, M., and Hikosaka, O. (2008). A neural correlate of motivational conflict in the superior colliculus of the macaque. J. Neurophysiol. 100, 1332–1342.
Jaber, M., Robinson, S. W., Missale, C., and Caron, M. G. (1996). Dopamine receptors and brain function. Neuropharmacology 35, 1503–1519.
Kalenscher, T., Lansink, C. S., Lankelma, J. V., and Pennartz, C. M. (2010). Reward-associated gamma oscillations in ventral striatum are regionally differentiated and modulate local firing activity. J. Neurophysiol. 103, 1658–1672.
Kawagoe, R., Takikawa, Y., and Hikosaka, O. (1998). Expectation of reward modulates cognitive signals in the basal ganglia. Nat. Neurosci. 1, 411–416.
Kawagoe, R., Takikawa, Y., and Hikosaka, O. (2004). Reward-predicting activity of dopamine and caudate neurons-a possible mechanism of motivational control of saccadic eye movement. J. Neurophysiol. 91, 1013–1024.
Kravitz, A. V., Freeze, B. S., Parker, P. R., Kay, K., Thwin, M. T., Deisseroth, K., and Kreitzer, A. C. (2010). Regulation of Parkinsonian motor behaviours by optogenetic control of basal ganglia circuitry. Nature 466, 622–626.
Kreitzer, A. C., and Malenka, R. C. (2007). Endocannabinoid-mediated rescue of striatal LTD and motor deficits in Parkinson’s disease models. Nature 445, 643–647.
Lauwereyns, J., Watanabe, K., Coe, B., and Hikosaka, O. (2002). A neural correlate of response bias in monkey caudate nucleus. Nature 418, 413–417.
Lei, W., Jiao, Y., Del Mar, N., and Reiner, A. (2004). Evidence for differential cortical input to direct pathway versus indirect pathway striatal projection neurons in rats. J. Neurosci. 24, 8289–8299.
Lo, C. C., and Wang, X. J. (2006). Cortico-basal ganglia circuit mechanism for a decision threshold in reaction time tasks. Nat. Neurosci. 9, 956–963.
Mallol, R., Barros-Loscertales, A., Lopez, M., Belloch, V., Parcet, M. A., and Avila, C. (2007). Compensatory cortical mechanisms in Parkinson’s disease evidenced with fMRI during the performance of pre-learned sequential movements. Brain Res. 1147, 265–271.
Matsumoto, M., and Hikosaka, O. (2007). Lateral habenula as a source of negative reward signals in dopamine neurons. Nature 447, 1111–1115.
Mehta, M. A., Montgomery, A. J., Kitamura, Y., and Grasby, P. M. (2008). Dopamine D2 receptor occupancy levels of acute sulpiride challenges that produce working memory and learning impairments in healthy volunteers. Psychopharmacology (Berl.) 196, 157–165.
Montague, P. R., Dayan, P., and Sejnowski, T. J. (1996). A framework for mesencephalic dopamine systems based on predictive Hebbian learning. J. Neurosci. 16, 1936–1947.
Morris, G., Arkadir, D., Nevet, A., Vaadia, E., and Bergman, H. (2004). Coincident but distinct messages of midbrain dopamine and striatal tonically active neurons. Neuron 43, 133–143.
Muslimovic, D., Post, B., Speelman, J. D., and Schmand, B. (2007). Motor procedural learning in Parkinson’s disease. Brain 130, 2887–2897.
Nakamura, K., and Hikosaka, O. (2006). Role of dopamine in the primate caudate nucleus in reward modulation of saccades. J. Neurosci. 26, 5360–5369.
Nakamura, K., Matsumoto, M., and Hikosaka, O. (2008). Reward-dependent modulation of neuronal activity in the primate dorsal raphe nucleus. J. Neurosci. 28, 5331–5343.
Oyama, K., Hernadi, I., Iijima, T., and Tsutsui, K. (2010). Reward prediction error coding in dorsal striatal neurons. J. Neurosci. 30, 11447–11457.
Pessiglione, M., Seymour, B., Flandin, G., Dolan, R. J., and Frith, C. D. (2006). Dopamine-dependent prediction errors underpin reward-seeking behaviour in humans. Nature 442, 1042–1045.
Picconi, B., Centonze, D., Hakansson, K., Bernardi, G., Greengard, P., Fisone, G., Cenci, M. A., and Calabresi, P. (2003). Loss of bidirectional striatal synaptic plasticity in L-DOPA-induced dyskinesia. Nat. Neurosci. 6, 501–506.
Pizzagalli, D. A., Evins, A. E., Schetter, E. C., Frank, M. J., Pajtas, P. E., Santesso, D. L., and Culhane, M. (2008). Single dose of a dopamine agonist impairs reinforcement learning in humans: behavioral evidence from a laboratory-based measure of reward responsiveness. Psychopharmacology (Berl.) 196, 221–232.
Reynolds, J. N., Hyland, B. I., and Wickens, J. R. (2001). A cellular mechanism of reward-related learning. Nature 413, 67–70.
Richfield, E. K., Penney, J. B., and Young, A. B. (1989). Anatomical and affinity state comparisons between dopamine D1 and D2 receptors in the rat central nervous system. Neuroscience 30, 767–777.
Robinson, D. A. (1972). Eye movements evoked by collicular stimulation in the alert monkey. Vision Res. 12, 1795–1808.
Samejima, K., Ueda, Y., Doya, K., and Kimura, M. (2005). Representation of action-specific reward values in the striatum. Science 310, 1337–1340.
Sato, M., and Hikosaka, O. (2002). Role of primate substantia nigra pars reticulata in reward-oriented saccadic eye movement. J. Neurosci. 22, 2363–2373.
Schall, J. D., Hanes, D. P., Thompson, K. G., and King, D. J. (1995). Saccade target selection in frontal eye field of macaque. I. Visual and premovement activation. J. Neurosci. 15, 6905–6918.
Schultz, W. (2006). Behavioral theories and the neurophysiology of reward. Annu. Rev. Psychol. 57, 87–115.
Schultz, W., Apicella, P., Scarnati, E., and Ljungberg, T. (1992). Neuronal activity in monkey ventral striatum related to the expectation of reward. J. Neurosci. 12, 4595–4610.
Schultz, W., Dayan, P., and Montague, P. R. (1997). A neural substrate of prediction and reward. Science 275, 1593–1599.
Schwarzschild, M. A., Agnati, L., Fuxe, K., Chen, J. F., and Morelli, M. (2006). Targeting adenosine A2A receptors in Parkinson’s disease. Trends Neurosci. 29, 647–654.
Shen, W., Flajolet, M., Greengard, P., and Surmeier, D. J. (2008). Dichotomous dopaminergic control of striatal synaptic plasticity. Science 321, 848–851.
Shimo, Y., and Hikosaka, O. (2001). Role of tonically active neurons in primate caudate in reward-oriented saccadic eye movement. J. Neurosci. 21, 7804–7814.
Sommer, M. A., and Wurtz, R. H. (2001). Frontal eye field sends delay activity related to movement, memory, and vision to the superior colliculus. J. Neurophysiol. 85, 1673–1685.
Surmeier, D. J., Ding, J., Day, M., Wang, Z., and Shen, W. (2007). D1 and D2 dopamine-receptor modulation of striatal glutamatergic signaling in striatal medium spiny neurons. Trends Neurosci. 30, 228–235.
Takikawa, Y., Kawagoe, R., and Hikosaka, O. (2002). Reward-dependent spatial selectivity of anticipatory activity in monkey caudate neurons. J. Neurophysiol. 87, 508–515.
Takikawa, Y., Kawagoe, R., and Hikosaka, O. (2004). A possible role of midbrain dopamine neurons in short- and long-term adaptation of saccades to position-reward mapping. J. Neurophysiol. 92, 2520–2529.
Tereshchenko, L. V., Yudin, A. G., Kuznetsov, Y., Latanov, A. V., and Shul’govskii, V. V. (2002). Disturbances of saccadic eye movements in monkeys during development of MPTP-induced syndrome. Bull. Exp. Biol. Med. 133, 182–184.
Vermersch, A. I., Rivaud, S., Vidailhet, M., Bonnet, A. M., Gaymard, B., Agid, Y., and Pierrot-Deseilligny, C. (1994). Sequences of memory-guided saccades in Parkinson’s disease. Ann. Neurol. 35, 487–490.
Voon, V., Pessiglione, M., Brezing, C., Gallea, C., Fernandez, H. H., Dolan, R. J., and Hallett, M. (2010). Mechanisms underlying dopamine-mediated reward bias in compulsive behaviors. Neuron 65, 135–142.
Wang, Z., Kai, L., Day, M., Ronesi, J., Yin, H. H., Ding, J., Tkatch, T., Lovinger, D. M., and Surmeier, D. J. (2006). Dopaminergic control of corticostriatal long-term synaptic depression in medium spiny neurons is mediated by cholinergic interneurons. Neuron 50, 443–452.
Watanabe, K., and Hikosaka, O. (2005). Immediate changes in anticipatory activity of caudate neurons associated with reversal of position-reward contingency. J. Neurophysiol. 94, 1879–1887.
Watanabe, K., Lauwereyns, J., and Hikosaka, O. (2003). Neural correlates of rewarded and unrewarded eye movements in the primate caudate nucleus. J. Neurosci. 23, 10052–10057.
White, O. B., Saint-Cyr, J. A., Tomlinson, R. D., and Sharpe, J. A. (1983). Ocular motor deficits in Parkinson’s disease. II. Control of the saccadic and smooth pursuit systems. Brain 106(Pt 3), 571–587.
Keywords: LTP, LTD, model, saccade, latency, reaction time, reward, motivation
Citation: Hong S and Hikosaka O (2011) Dopamine-mediated learning and switching in cortico-striatal circuit explain behavioral changes in reinforcement learning. Front. Behav. Neurosci. 5:15. doi: 10.3389/fnbeh.2011.00015
Received: 19 October 2010;
Accepted: 09 March 2011;
Published online: 21 March 2011.
Edited by:
Paul E. M. Phillips, University of Washington, USAReviewed by:
Kenji Doya, Okinawa Institute of Science and Technology, JapanCopyright: © 2011 Hong and Hikosaka. This is an open-access article subject to an exclusive license agreement between the authors and Frontiers Media SA, which permits unrestricted use, distribution, and reproduction in any medium, provided the original authors and source are credited.
*Correspondence: Simon Hong, Laboratory of Sensorimotor Research, National Eye Institute, National Institutes of Health, 49 Convent Drive, Bethesda, MD 20892, USA. e-mail:aG9uZ3lAbmVpLm5paC5nb3Y=
Disclaimer: All claims expressed in this article are solely those of the authors and do not necessarily represent those of their affiliated organizations, or those of the publisher, the editors and the reviewers. Any product that may be evaluated in this article or claim that may be made by its manufacturer is not guaranteed or endorsed by the publisher.
Research integrity at Frontiers
Learn more about the work of our research integrity team to safeguard the quality of each article we publish.