- 1 Department of Neurobiology, University of Chicago, Chicago, IL, USA
- 2 Department of Psychology, Center for Neural Science, New York University, New York, NY, USA
- 3 Committee on Neurobiology, University of Chicago, Chicago, IL, USA
The impact of dopamine on adaptive behavior in a naturalistic environment is largely unexamined. Experimental work suggests that phasic dopamine is central to reinforcement learning whereas tonic dopamine may modulate performance without altering learning per se; however, this idea has not been developed formally or integrated with computational models of dopamine function. We quantitatively evaluate the role of tonic dopamine in these functions by studying the behavior of hyperdopaminergic DAT knockdown mice in an instrumental task in a semi-naturalistic homecage environment. In this “closed economy” paradigm, subjects earn all of their food by pressing either of two levers, but the relative cost for food on each lever shifts frequently. Compared to wild-type mice, hyperdopaminergic mice allocate more lever presses on high-cost levers, thus working harder to earn a given amount of food and maintain their body weight. However, both groups show a similarly quick reaction to shifts in lever cost, suggesting that the hyperdominergic mice are not slower at detecting changes, as with a learning deficit. We fit the lever choice data using reinforcement learning models to assess the distinction between acquisition and expression the models formalize. In these analyses, hyperdopaminergic mice displayed normal learning from recent reward history but diminished capacity to exploit this learning: a reduced coupling between choice and reward history. These data suggest that dopamine modulates the degree to which prior learning biases action selection and consequently alters the expression of learned, motivated behavior.
Introduction
The dopamine system plays a critical role in learning about rewards and performing behaviors that yield them (Berke and Hyman, 2000; Dayan and Balleine, 2002; Wise, 2004; Cagniard et al., 2006b; Daw and Doya, 2006; Salamone, 2006; Berridge, 2007; Day et al., 2007; Phillips et al., 2007; Schultz, 2007a; Belin and Everitt, 2008). Despite the ongoing debate on the precise role of dopamine in learning, motivation, and performance (Wise, 2004; Salamone, 2006; Berridge, 2007), the impact of hypothesized dopamine functions on adaptive behavior in a more (semi-) naturalistic environment is largely unexamined.
In natural environments, animals often have to choose between several actions, and the outcome of these actions may shift across time. As a consequence, the animal has to continually sample the environment and adjust its behavior in response to changing reward contingencies. To accomplish this, the animal must strike a balance between exploiting actions that have been previously rewarded and exploring previously disfavored actions to determine whether contingencies have changed. In the study of reinforcement learning (RL), the challenge of striking such a balance has been termed the explore-exploit dilemma, and formalizes an issue that lies at the heart of behavioral flexibility and adaptive learning (Sutton and Barto, 1998).
An implicit assumption in RL theories is that the learned value expectations determine action choice. Importantly, because of the explore-exploit dilemma, this control is not thought to be absolute: rather, choice in reinforcement learning tasks is characterized by a stochastic soft maximization (“softmax”) rule that allocates choices randomly, but with a bias toward the options believed to be richer (Daw et al., 2006). An important open question, however, is how the brain controls the degree to which choice is focused on apparently better options; that is, how much prior experience biases current action selection. This is commonly operationalized in RL models by a gain parameter (called “temperature”) that scales the effect of learned values on biases in action choice; however, though some hypotheses exist, its physiological instantiation is unknown (Doya, 2002 Daw et al., 2006; Cohen et al., 2007). In the present study, we consider the possibility that dopamine – and specifically, dopamine signaling at a tonic timescale – might be involved in controlling this aspect of behavioral expression and, as a result, modulate the balance between exploration and exploitation.
The hypothesized role of dopamine in learning about action values (Montague et al., 1996; Schultz et al., 1997) is based largely on recordings of phasic dopamine responses. However, dopamine neurons also exhibit a slower, more regular tonic background activity (Grace and Bunney, 1984b). Pharmacological and genetic experiments, which impact dopamine signaling at a tonic timescale, suggest a role for tonic dopamine in the expression rather than acquisition of motivated behavior (Cagniard et al., 2006a,b). To date, these experimental observations have not been analyzed in the context of computational reinforcement learning models, in a manner analogous to studies of phasic signaling, which has hampered efforts to formalize these results and to understand the relationship between theories of dopamine’s action in performance and learning (Berridge, 2007; Niv et al., 2007; Salamone, 2007). We take advantage of how the distinction between acquisition and expression is formalized in temporal difference RL models through the learning rate and temperature parameters, respectively, to quantitatively evaluate the impact of elevated tonic dopamine on choice behavior in the context of the computational model widely associated with phasic dopamine.
We used a homecage operant paradigm where mice earn their food entirely through lever pressing. In this “closed economy” (Rowland et al., 2008) with no access to food outside of the work environment, no experimenter induced food-restriction is needed; the amount of resources gained and spent reflect the animal’s behavioral strategy in adapting to its environment. In our paradigm, two levers yield food, but at different costs. At any one time, one lever is inexpensive (requiring few presses for a food) and another is expensive (requiring more presses). Which lever is expensive and which is inexpensive switches every 20-40 minutes.
We tested wild-type C57BL/6 mice and hyperdopaminergic dopamine-transporter knock down mice (DATkd) with reduced DA clearance and elevated extracellular tonic DA (Zhuang et al., 2001). Fitting the data to a reinforcement learning model, we find that altered dopamine modulates temperature – the explore-exploit parameter – resulting in decreased responsiveness to recent reward, without a change in learning rate, resulting in diminished behavioral flexibility in response to shifting environmental contingencies.
Materials and Methods
Animals
All mice were male between 10 and 12 weeks of age at the start of the experiment. Wild-type C57BL/6 mice were obtained from Jackson Laboratories. The dopamine transporter knock-down mice (DATkd) were from an established colony backcrossed with C57BL/6 more than ten generations. The DATkd have been previously described and characterized (Zhuang et al., 2001; Pecina et al., 2003; Cagniard et al., 2006a; Yin et al., 2006). All mice were housed under standard 12:12 light cycles. All animal procedures were approved by the Institutional Animal Care and Use Committee at The University of Chicago.
Behavior Setup and Housing
Mice were singly housed in standard cages equipped (Med-Associates, St. Albans, VT, USA) with two levers placed on one side of the cage approximately six inches apart with a food hopper between the levers. A pellet dispenser delivered 20 mg grain-based precision pellets (Bio-Serv, Frenchtown, NJ, USA) contingent on lever presses according to a programmed schedule. No other food was available. Water was available ad libitum. Upon initial placement in the operant homecages, three pellets were placed in the food hopper and the first 50 lever presses on either lever yielded a pellet (continuous reinforcement), after which a fixed ratio (FR) schedule was initiated. The cumulative lever press count for each lever was reset for both levers at each pellet delivery. All mice acquired the lever pressing response overnight. On the first day of FR (baseline), both levers operated on an FR20 schedule. On subsequent days, at any given time one lever was expensive and the other inexpensive lever. The inexpensive lever was always FR20. The expensive lever incremented by 20 each day from 40 to 200. Which lever was cheap and which expensive switched every 20–40 min. After the final FR200 increment, the program reverted to baseline conditions (FR20 both levers) for 3 days.
Data Collection and Analysis
All events – lever presses, pellet delivery, cost change between levers – were recorded and time-stamped using Med-PCIV software (Med-Associates, St. Albans, VT, USA). The data was then imported into MATLAB for analysis. Total consumption, high cost, low cost presses, ratio of low-cost to total, average cost per pellet, number of meals per day, average size of meals and duration of meals were calculated directly by the program operating the experiment (i.e., Figure 1 and Table 1). The onset of a meal was defined as the procurement of one pellet and the offset defined as the last pellet earned before 30 min elapsed without procuring a pellet. To calculate average lever pressing before and after episodes of cost switching between the levers, averaged across the experiment (Figure 2), all experimental days (i.e., with a cost differential between levers) were combined into a single dataset for each mouse. The time points for all cost switches were identified and a 10-min window (data recorded in 0.1 s bins) before and after each were averaged across switch episodes. The mean over all events was smoothed with a half-Gaussian filter using a weighted average kernel to retain original y-axis values from the data. The resulting smoothed data were averaged across mice within each genotype. To calculate runlength averaged across switch episodes, all lever presses within a run (consecutive presses on one lever without intervening presses on the other lever) were coded as the total length of the run (e.g., for a run of three presses, each would be coded as 3). Time bins in which no lever press occurred were coded with zero. When the mean across episodes was calculated, episodes without any pressing on either lever (e.g., mouse sleeping) were coded as not a number (NaN) and excluded from the mean. To make statistical comparisons of the above analyses, the raw data (.ie., not smoothed) across 0.1 s bins were collapsed into 20 one minute bins which were used as repeated measures in two-way ANOVAs. For single statistical comparisons, t-tests were used.
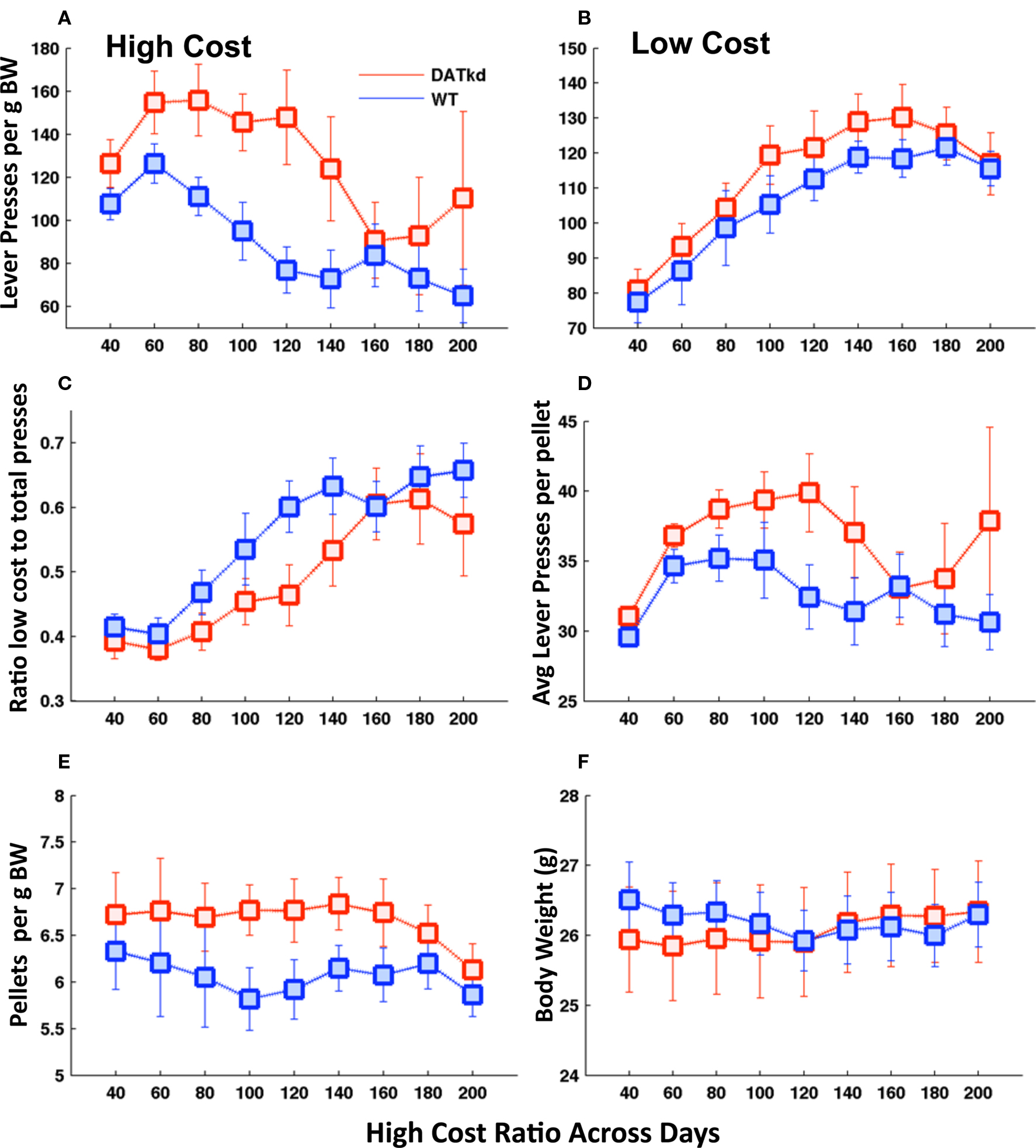
Figure 1. Lever pressing, consumption and body weight across experimental days. Average number of lever presses (LP) per gram of body weight on the (A) expensive lever (genotype, p < 0.01) and (B) inexpensive lever (genotype, NS). (C) Ratio of lever presses on the low cost lever to total lever presses (genotype, p = 0.121). (D) Average number of lever presses per pellet earned (genotype, p = 0.059). (E) Average number of pellets earned per day per gram of body weight (genotype, p = 0.025). (F) Daily body weight across experiment (genotype, NS). Error bars = S.E.M., N = 10.
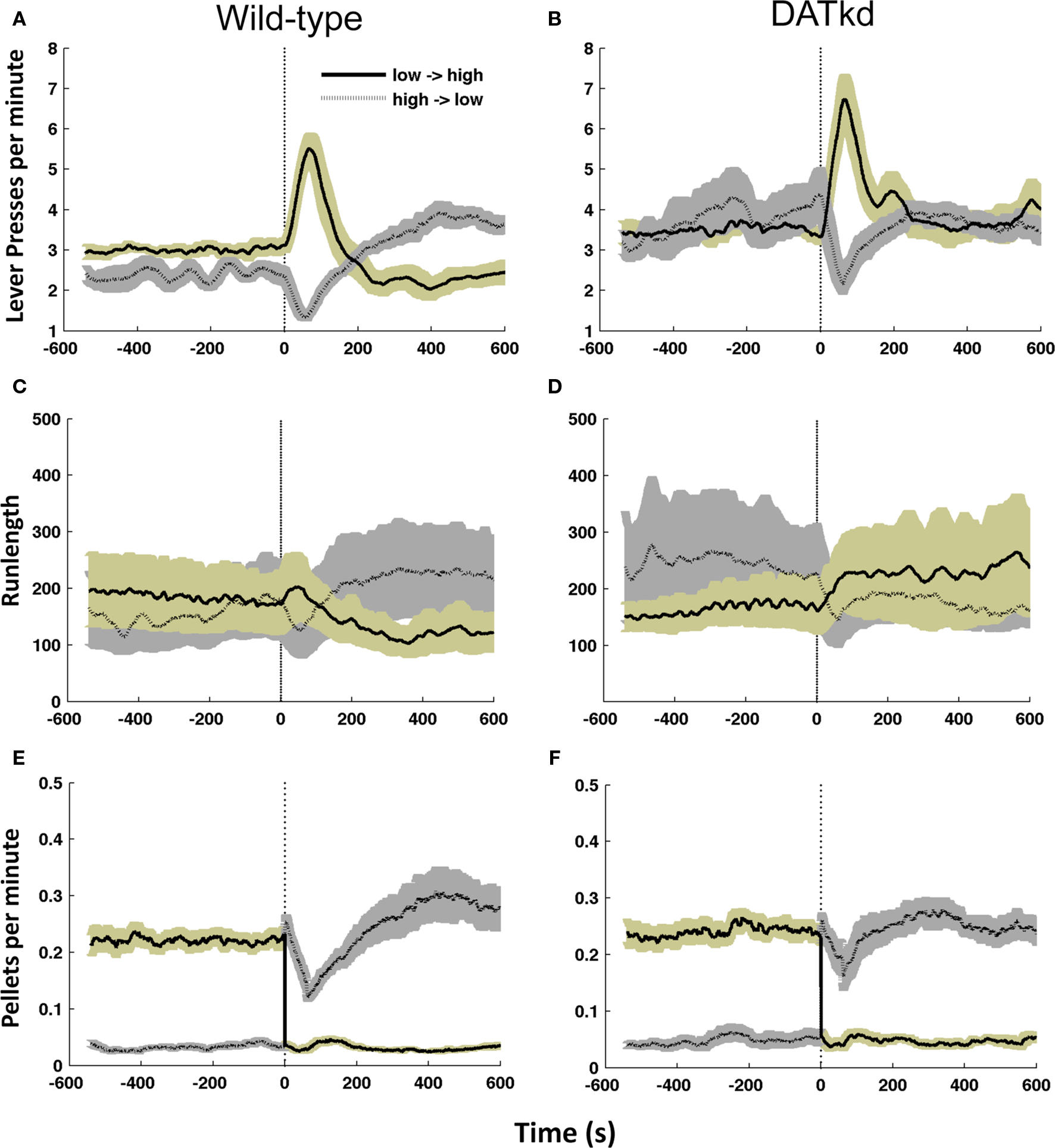
Figure 2. Mean allocation of effort and runlength on the high and low cost lever following the switch in reward contingency (dashed line). Mean lever presses per minute 10 min before and after reward contingency switch for (A) wild-type and (B) DATkd (genotype × lever × time, p < 0.0001). Mean runlength on each lever for (C) wild-type (D) DATkd (genotype × lever × time, p < 0.001). Mean rate of reinforcement across all contingency switches for (E) wild-type and (F) DATkd on the low → high cost lever (solid line, gold shading) and high → low cost lever (dotted line, gray shading) averaged across all episodes of contingency switches between levers (vertical dashed lines). Shading = S.E.M., N = 10.
Data Modeling
To model leverpress-by-leverpress how choices were impacted by rewarding feedback, we first removed temporal information from the dataset to express the data as a series of choices ct (=1 or −1 according to which was pressed) of either lever, and of accompanying rewards rt (=1, 0, or −1 where no reward was coded as 0 and a rewarded response on lever 1 or −1 was coded as 1 or −1, respectively). We characterized the choice sequences using two models, a more general logistic regression model (Lau and Glimcher, 2005) and a more specific model based on temporal difference learning (Sutton and Barto, 1998), and estimated the free parameters of these models for mice of each genotype.
In the regression model (Lau and Glimcher, 2005), the dependent variable was taken to be the binary choice variable c, and as explanatory variables for each t we included the N rewards preceding it, rt−N…t−1. Additionally, we included the prior leverpress (ct−1) to capture a tendency to stay or switch, and a bias variable (1) to capture fixed, overall preference for or against lever 1, for a total of N + 2 free parameters (regression weights expressing, for each explanatory variable, how it impacted the chance of choosing either lever). We used logistic regression to estimate maximum likelihood weights for each mouse’s choices separately, using the entire dataset concatenated across experimental days. We repeated the fit process for N = 1 − 100.
Error-driven reinforcement learning models such as temporal difference learning are closely related to a special case of the above model (Lau and Glimcher, 2005) with many fewer parameters, and we also fit the parameters of such a model to animals’ choice behavior. In particular, we assumed subjects maintain a value Vt for each lever, and for each choice updated the value of the chosen lever according to Vt + 1(ct) = Vt(ct) + αV•δt, where αV is a free learning rate parameter and the prediction error δt is the difference between the received and expected reward amounts, which in our notation can be written δt = abs(rt) − Vt(ct). Additionally, defining −ct as the option not chosen, we assumed this option is also updated according to Vt + 1(−ct) = Vt(−ct) + αV(0 − Vt(−ct)). (See Daw and Dayan, 2004; Corrado et al., 2005; Lau and Glimcher, 2005). Finally, we assumed subjects choose probabilistically according to a softmax choice rule, which is normally written:

Here the parameter β controls the degree to which choices are focused on the apparently best option. We refer to this parameter as the temperature, although it is technically the inverse temperature; the term originates in statistical mechanics where larger temperatures (here, smaller inverse temperatures) imply that particle velocities are more randomly distributed. In the second form of the equation, σ(z) is the logistic function 1/(1 + exp(−z)), highlighting the relationship between the RL model and logistic regression.
We augmented the model from Eq. 1 with additional bias terms, matching those used in the logistic regression model. Also, because the fits of the logistic regression model (see Results) suggested additional short-latency effects of reward on choice, we included an additional term to capture these effects:

Here, as in the logistic regression model, the parameters β1 and βc code biases for or against lever 1, and for or against sticking with the previous choice. St is a second, “short-latency” value function updated from received rewards using the same learning rules as Vt but with its own learning rate and temperature parameters, αs and βs. As for the logistic regression model, we fit the model of Eq. 2 to the choice and reward sequences for each mouse separately, in order to extract maximum likelihood estimates for the six free parameters (αV, βV, αs, βs, β1, and βc). For this, we searched for parameter estimates that maximized the log likelihood of the entire choice sequence (the sum over trials of the log of Eq. 2) using a non-linear function optimizer (fmincon from MATLAB optimization toolbox, Mathworks, Natick, MA, USA).
To measure goodness of model fit, we report a pseudo-r2 statistic (Camerer and Ho, 1999; Daw et al., 2006), defined as (R − L)/R, where R is the negative log likelihood of the data under random chance (the number of choices multiplied by −log(0.5)), and L is the negative log likelihood of the data under the model. To compare models, we used the Bayesian Information Criterion (Schwarz, 1978) to correct the raw likelihoods for the number of free parameters fit. Likelihoods and BIC scores were aggregated across mice. For comparing parameters between genotypes, we treated the parameter estimates as random variables instantiated once per animal then tested for between-group differences with two-sample t-tests. For visualization purposes, we plotted the mean coefficients for lagged reward from the logistic regression model with N = 100, averaged across animals within each genotype. For the reinforcement learning model, we computed the equivalent weights on lagged rewards implicit from Eq. 2 (for rewards τ trials ago, this is αV •βV•(1 – αV)τ–1 + αS βS•(1 – 1αS)τ–1, which can be obtained by iteratively substituting the update rules for V and S into Eq. 2, τ times), and again averaged these across animals.
Results
Wild-Type and DATkd Exhibit Similar Behavior when the Cost of Both Levers is Low
To assess for potential non-task related differences between the groups, baseline behavior was assessed during periods in which both levers yielded reward equally on a low-cost, FR20 schedule. Baseline measures were taken at the beginning and end of the experimental period. As there were no significant differences between pre- and post- experiment consumption (mean difference food consumed, 0.15g; t = 0.732, p = 0.4792, N = 6–7), they are combined in Table 1. No differences were observed in total consumption, total lever pressing, number of meals, meal size, meal duration or starting weights between the groups. Although hyperdopaminergic mice have been associated with greater motivation and willingness to work for reward when food-restricted (Cagniard et al., 2006a,b), we observe no difference in primary motivation for food or in the expenditure of energy (lever pressing) to obtain food under these initial, low cost conditions.
DATkd Mice Allocate More Effort to High-Cost Lever Pressing
During the experimental period there is always a cost differential between the levers and the assignment of low versus high cost to the left or right levers switches every 20–40 min. Figures 1A and B shows lever pressing on the high and low cost levers across the experiment. A full, repeated measure ANOVA with genotype and lever as independent variables reveals a significant main effect of genotype (F(1,18) = 17.13, p < 0.001) and a trend for genotype × lever interaction (F(1,18) = 3.43, p = 0.08) on lever pressing. Analyzing the levers separately, the DATkd mice expend more effort on the high cost lever than wild-type (Figure 1A, F(1,144) = 8.65, p < 0.01). There is no statistically significant difference in pressing on the low cost lever (Figure 1B, F(1,144) = 1.95, p = 0.179). This significant increase in high-cost pressing results in a trend toward diminished ratio of low cost versus total pressing (Figure 1C, F(1,144) = 2.64, p = 0.121) and, as a result, DATkd, on average, spend more effort lever pressing in order to earn one pellet than wild-type mice (Figure 1D, F(1,144) = 4.04, p = 0.059). Data in Figures 1A and B are normalized to body weight, i.e., lever presses per gram of body weight.
The DATkd mice consume more food (Figure 1E, F(1,144) = 5.94, p = 0.025) per gram of body weight without gaining more weight than wild-type (Figure 1F, F(1,144) = 0.01, p = 0.922), reflecting a less efficient behavioral strategy for maintaining energy balance. That is, the DATkd mice work harder and eat more to maintain the same body weight as wild-type. The increase in consumption does not reflect an overall higher basal activity level as there were no consumption or weight differences when the cost of both levers was low.
Wild-Type and DATkd Both Respond to Cost Switches between Levers but Employ Different Strategies for Maximizing Reward
There are several possible explanations of why the DATkd spend more effort working for food on the high-cost lever in order to maintain their body weight. They may have impaired learning and are not able to process reward information accurately and efficiently enough to respond to changes in reward contingencies between the levers. They may be more perseverative in their behavior, making it difficult for them to disengage one lever and engage another. This would not only result in wasted presses on the high cost lever, but would reduce sampling efficiency making them slower to recognize when the cost contingencies between levers have changed. To examine their behavioral strategies in greater detail, we analyzed lever pressing on the high and low cost levers before and after episodes of contingency switches between the levers.
Total Effort Allocation
Figures 2A and B show the average lever press rate on both levers 10 min prior to and after a switch in reward contingencies between the levers (vertical dashed line), averaged across the experiment. A significant difference is observed in the pattern of responding across contingency changes between the groups (Figures 2A and B; genotype main effect, F(1,342) = 17.11, p < 0.001; genotype × lever × time, F(19,342) = 2.53, p < 0.001). Prior to a switch in reward contingencies, wild-type mice exhibit pressing on both levers but clearly favor the inexpensive lever (Figure 2A; pre-switch main effect of lever, F(1,81) = 15.07, p = 0.0037). After cost contingencies switch, the wild-type show an initial burst of activity on what was once the low cost lever, but is now more expensive, followed by a decline in presses on this lever (Figure 2A; post-switch lever × time, F(9,81) = 72.518, p = 0.0001). After this burst, they increase their pressing on the newly established low cost lever, reversing their distribution of pressing in order to favor lower pressing per pellet (Figure 2A; last five bins only, lever main effect, F(1,36) = 10.726, p = 0.0096). The observed increase in pressing on the previously cheap but now expensive lever could reflect the animals’ recognition of the contingency change or arise simply as a consequence of continuing to press the previously preferred lever until it yields reward on the higher ratio. Figure 2E shows the rate of earned reinforcement 10 min prior to and following the shift in lever costs averaged across the experiment. After the contingency change, there is an immediate increase in earned rewards on the now cheap lever followed by a brief decrease before the mice establish a new preference shifting effort to the now cheap lever. This indicates that the burst on the previously expensive lever does not arise as mice simply complete the now higher ratio. Instead, the mice rapidly experience reward at the new contingencies but nonetheless return to the previously cheap lever and persist with it temporarily before shifting and establishing a new preference. This suggests the sharp increase in cheap now expensive lever presses following contingency changes is analogous to an extinction burst. These data demonstrate that wild-type mice have an overall preference for the low cost lever (Figure 2A; full lever × time, F(19,171) = 17.9, p < 0.0001) and an ability to recognize when the reward contingencies switch between levers. After a contingency change, the wild-type mice sample the new contingencies to establish the relative value of each lever and establish a new policy to exploit their updated knowledge until the next contingency switch.
In contrast, the DATkd do not show a preference for the low-cost lever prior to contingency changes (Figure 2B; pre-switch main effect of lever, F(1,81) = 0.176, p = 0.6848). However, they exhibit the same initial response to a change in cost contingencies as the wild-type (Figure 2B; post-switch lever × time, F(9,81) = 9.127, p < 0.001): an initial burst of activity on what was once the low cost lever, but is now more expensive. After this burst, the DATkd do not show a preference for one lever or another (Figure 2B; last five bins only, lever main effect, F(1,36) = 0.035, p = 0.8556). Figure 2F shows, that like the wild-type, the DAT mice also receive immediate reinforcement following the new contingencies, suggesting that the increase pressing on the previously cheap lever, as in wild-type, reflects an extinction burst. This indicates that the DATkd are sensitive to changes in reward contingencies and like wild-type sample the new contingencies to establish a new action policy (Figure 2B; full lever × time, F(19,171) = 3.39, p < 0.0001), ruling out the possibility that the DATkd are slower to recognize changes in the costs of the levers. However, despite their sensitivity to changes in the cost of rewards and the energetic advantage this knowledge could potentially provide if they were to exploit it, they do not preferentially press the inexpensive lever. Instead, they adopt an action policy of pressing both levers equally, despite the levers’ relative rates of return.
Run Length as an Index of Persistence
Measuring average lever press rates alone does not enable us to evaluate the pattern of switching between levers. To study this pattern, we analyzed run length – number of consecutive presses on a single lever before switching to the other lever (see Materials and Methods) – observing a significantly different pattern between the groups (Figures 2C and D; geno × lever × time, F(19,342) = 3.545, p < 0.0001). In wild-type, run length is consistent with the distribution of pressing observed in Figure 2A: the mice show greater run length on the low cost lever prior to the reward contingency switch between levers, followed by an extinction burst on the now high cost lever and a subsequent increase in run length with the now low cost lever (Figure 2C; lever × time, F(18,162) = 4.674, p < 0.0001). In contrast, prior to the reward contingency change, the DATkd show greater run length on the expensive lever. After the change in costs between levers, the DATkd decrease their run length on the new low cost lever and increase persistence on the new high cost lever resulting overall in no significant difference in pressing between the levers across time (Figure 2D; lever × time, F(18,162) = 0.317, p = 0.9967). This indicates the DATkd increase or decrease their persistence commensurate with the cost of both levers, rather than focusing long runs on the low cost lever. Again, this suggests that the hyperdopaminergic mice are sensitive to contingency changes and their persistence on the expensive lever, relative to wild-type, is not indiscriminate.
Rate of Responding and Post-Reinforcement Pauses Similar between Groups
Apparent differences in choice behavior between the genotypes might arise secondary to a more fundamental difference in motor performance. We analyzed several measures to assess this possibility and find little difference between the groups. There is no significant difference between groups in the rate of responding averaged across meal episodes (mean: WT 4.75 ± 0.173, DAT, 5.52 ± 0.236, genotype main effect, F(1,180) = 2.347, p = 0.1429, data not shown). Second, a histogram of inter-response times (IRTs) normalized as percentage of total IRTs shows no main effect of genotype (Figures 3A and B; F(1,162) = 3.155, p = 0.0925) though wild-type exhibit a slightly greater percentage of shorter IRTs (Figure 3A; genotype × bins F(9,162) = 2.67, p = 0.0065). These data suggests no great differences between the groups in rate of responding, though the wild-type may exhibit slightly more rapid, successive presses. Because subtle differences in pausing after reward may be lost in the IRT histogram, we specifically evaluated post-reinforcement pauses (PRPs). Figures 3C and D shows a histogram of PRPs for both groups with no significant differences observed. Together with no differences at baseline, these data indicate that generalized performance or vigor differences between the groups cannot account for the observed difference in behavioral choices and strategy.
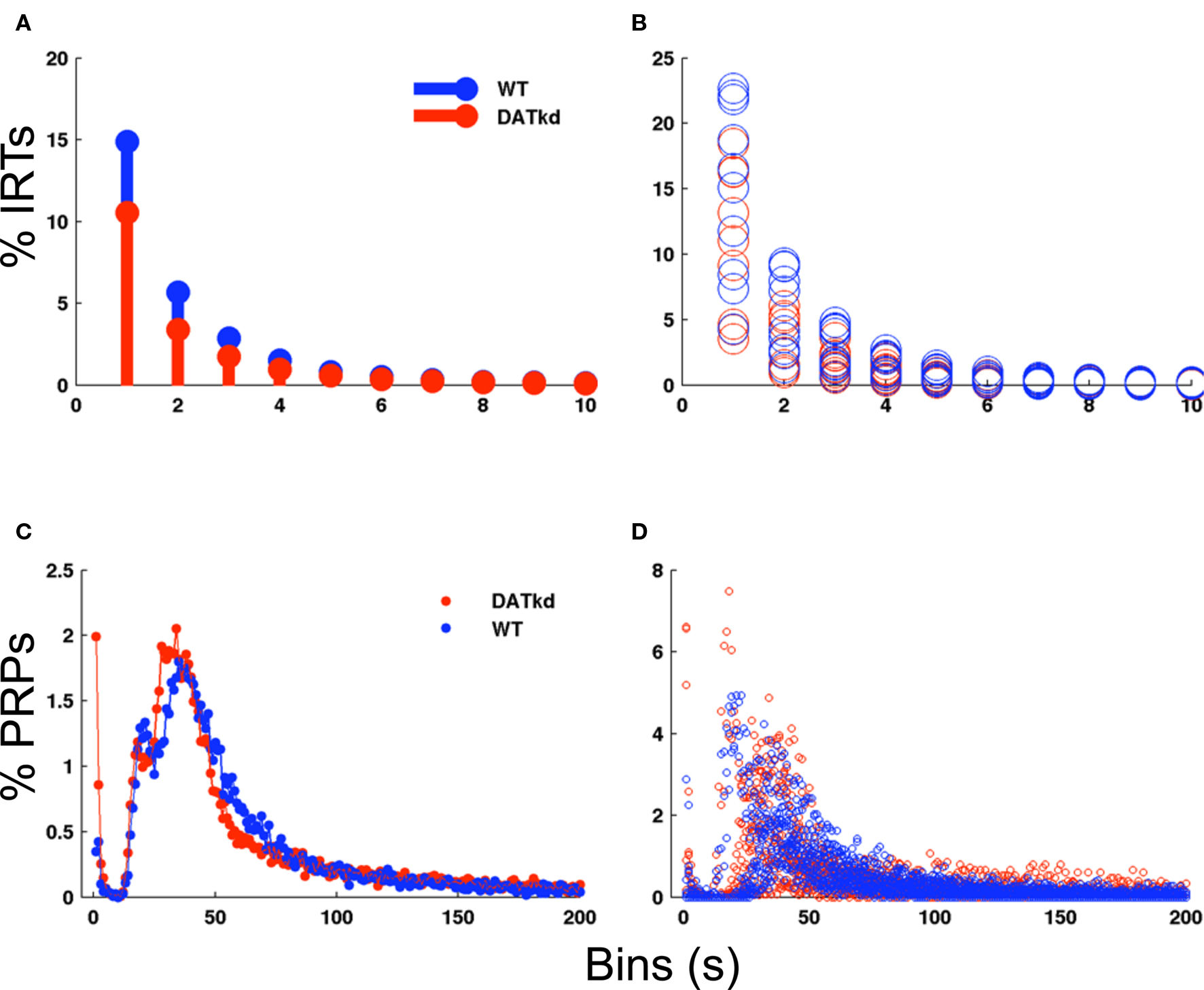
Figure 3. Inter-response times and post-reinforcement pauses across experiment. (A) Histogram of inter-response times (IRTs) in 1 s bins normalized to percentage of total IRTs for WT (blue bars) and DATkd (red bars) (genotype × bins, p = 0.0065). (B) Scatterplot of individual subject IRT histograms. (C) Histogram of post-reinforcement pauses (PRPs) in 1 s bins normalized to total PRPs for WT (blue trace) and DATkd (red trace) (genotype × bins, NS). (D) Scatterplot of individual subject PRP histograms. N = 10.
DATkd Show Effort Distribution Similar to Wild-Type when Cost Differential is Stationary
There are several potential explanations to the behavioral results described. The DATkd mice may be insensitive to costs and/or might derive some intrinsic value from lever pressing itself. To test these, we conducted a similar experiment with a cheap and expensive lever but which lever was cheap and expensive remained constant. We observe no significant differences between the groups in the stationary version of the paradigm (Figures 4A–D). This clearly indicates that the DATkd do not derive an intrinsic value from lever pressing. More importantly, though the results in the switching paradigm are consistent with a reduced sensitivity to cost in the DATkd, this experiment indicates that they are not indifferent to cost. Thus, their apparent reduced sensitivity to cost in the switching paradigm arises as a consequence of how they use reward (and cost) history to determine their behavioral strategy in a dynamic environment and not as a result of generalized indifference to cost.
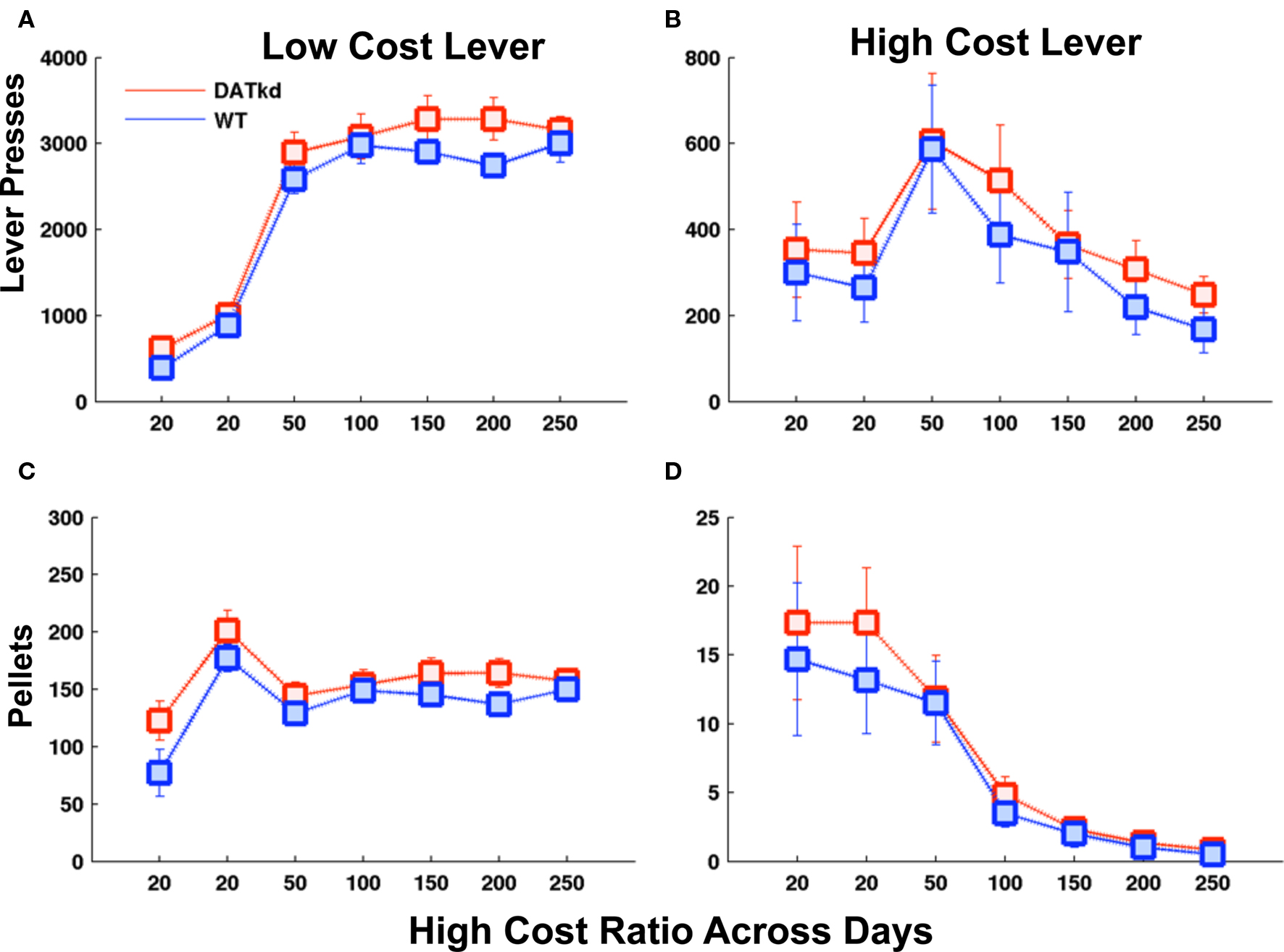
Figure 4. Effort and earned rewards when the price of the high and low cost levers does not switch. Average lever presses on the (A) low cost and (B) high cost lever and average pellets earned on (C) low and (D) high cost levers as the price of the high cost lever increases across days. No significant genotype differences across panels. Error bars = S.E.M, N = 6.
DATkd Lever Choice Is Less Influenced by Recent Reward than Wild-Type
The aggregate behavioral measures examined so far arise from cumulative, choice-by-choice decision-making. Animals must allocate their lever presses guided by recent rewarding outcomes, which are the only feedback that signals the periodic changes in cost contingencies. To understand how animals adapted their lever pressing, choice-by-choice, in response to reward outcomes and history, we fit behavior with reinforcement learning models that predict lever choice as a function of past experience (e.g., Lau and Glimcher, 2005). For this analysis, we considered only which levers were chosen in what order, and not the actual timing of lever presses. In this way, we were able to abstract away the temporal patterning of the behavior and analyze the choice between levers in a manner consistent with previous work on tasks in which choices occurred in discrete trials rather than ongoing free-operant responses (Sugrue et al., 2004; Lau and Glimcher, 2005; Daw et al., 2006). We used two models adapted from that literature, first a general logistic regression model that tests the overall form of the learning constrained by few assumptions (Lau and Glimcher, 2005) and, suggested by these fits, a more specific model based on temporal difference learning (Sutton and Barto, 1998). Parameters estimated from the fit of the more specific model characterize different aspects of the learning, and these were compared between genotypes.
First, logistic regression was used to predict choices as a function of the rewards received (or not) for recent previous lever presses, along with additional predictive variables to capture biases (see Materials and Methods). Figure 5A depicts the regression coefficients for rewards received from 1 to 100 lever presses previously, in predicting the current lever press. Coefficients (y-axis) greater than zero indicate that a reward tends to promote staying on the lever that produced it, while coefficients less than zero indicate that rewards instead promote switching. A standard error-driven reinforcement learning model (such as Eq. 1 from Materials and Methods) is equivalent to the logistic regression model with reward history coefficients that are everywhere positive, largest for the most recent rewards and with the effect of reward declining exponentially with delay (Lau and Glimcher, 2005). The coefficients illustrated in Figure 5A instead were sharply negative for the most recent reward, indicating a strong tendency to switch to the other lever. This effect decayed quickly and was replaced by the opposite tendency to stay on the lever that recently yielded reward.
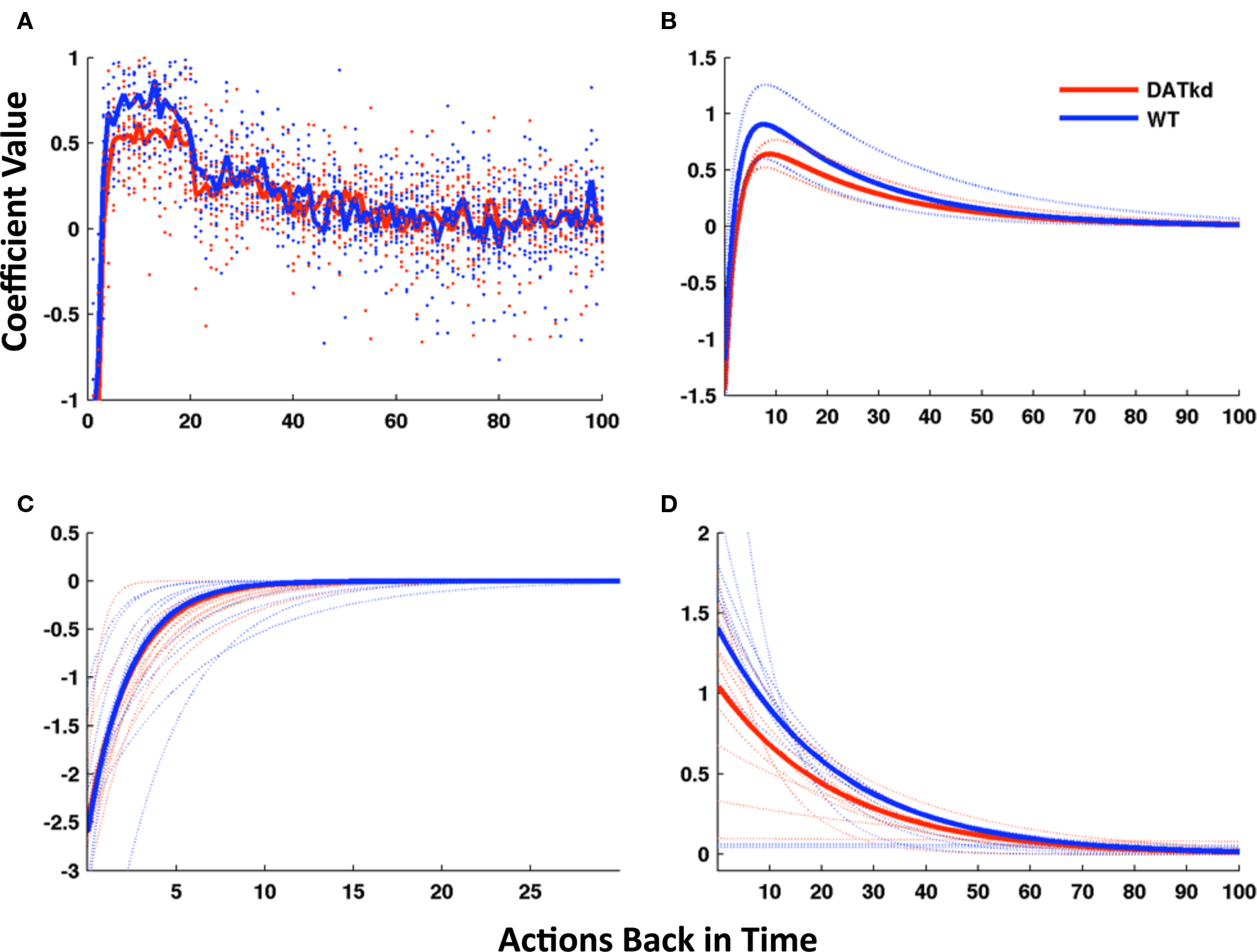
Figure 5. Model of reward function and persistence on high and low cost lever averaged across reward procurement. (A) Reward history as 100 discrete parameters representing 100 actions (rewarded or not) back in time, solid line represents group averages superimposed on a scatterplot of individual subjects (wild-type, blue; DATkd, red). (B) Reward as a continuous function comprised of two exponentials (4 parameters). Though the function incorporates the effect of reward infinitely back in time, only the first 100 actions back are shown. Light traces show curves plotted ± standard error of parameters within groups. (C,D) The two exponentials of the model plotted separately. Solid lines represent model using group means of parameters and light traces represent individual subjects. See Table 2 for statistics. N = 10.
We reasoned that instead of reward dependency following a single exponential curve, as in a standard reinforcement learning model, the response to reward appeared to be well characterized by the superposition of two exponentials, a short-latency tendency to switch initially overwhelming a more traditional, longer-latency value learning process.
We therefore fit the animals’ choices with an augmented error-driven learning model (Eq. 2), which included a standard value learning process accompanied by a second, short-latency process plus bias terms. This is equivalent to constraining the reward history coefficients from the logistic regression model to follow a curve described by the sum of two exponentials. Figure 5B displays the reward dependency curves implied by the best-fitting parameters of this reduced model to the choice data, in the same manner as those from the regression model; they appear to capture the major features of the original fits while somewhat “cleaning up” the noise. Although the reinforcement learning model had far fewer free parameters than the regression model (six per animal), it fit the choice data nearly as well (negative log likelihood, aggregated over animals, 1.156e + 5; pseudo-r2, 0.83). In order to compare the goodness of fit taking into account the number of parameters optimized, we used the Bayesian Information Criterion (BIC; Schwarz, 1978) to penalize data likelihoods for the number of free parameters. According to this score, the best of the regression models, trading off fit and complexity, was that for N = 20 (the number of rewards back in time for which coefficients were fit; 22 free parameters per animal, negative log likelihood, 1.168e + 5, pseudo-r20.83). The 6-parameter reinforcement learning model thus fit the data better (smaller negative log likelihood) than this model, even before correcting for the fact that it had about 1/4 the number of free parameters. (The difference in BIC-corrected likelihoods was 4.81e + 4 in favor of the simpler model, which constitutes “very strong” evidence according to the guidelines of Kass and Raftery, 1995). In all, these results suggest that the choice data were well characterized by the 6-parameter reinforcement learning model.
Finally, having developed, fit and validated a computational characterization of the choice behavior, we used the estimates of the model’s free parameters to compare the learning process between genotypes. Table 2 presents fitted parameters for each group and statistical comparisons. These comparisons show a selective difference in the parameter βV, which was smaller in the DATkd mice (t = 3.1, p < 0.01). This is the temperature parameter for the value learning process, which controls the extent to which learning about values guides action choice. This is consistent with the aggregate findings (Figures 1 and 2) that they distribute effort more evenly across both levers, resulting in more high cost lever presses and an overall less cost-effective behavioral strategy. By contrast, the remaining parameters of the model did not differ. These results suggest that the effect of the DAT knockdown was specific to the value learning process and not to the short-latency switching part of the model (Figures 5C and D, two exponentials plotted separately) or the other bias terms. Within value learning, the genotype difference was specific to the temperature parameter rather than the learning rate parameter αV, which characterizes how readily values adapt to feedback. This selective difference between groups is also apparent in Figures 5B and C, where the tendency toward a short latency switch following a reward appears similar between groups, but the subsequent countervailing tendency to return to a lever that has delivered reward appears blunted (Figures 5B and D, lower peak). Although this tendency is scaled down in the DATkd mice, the time course by which rewards exert their effect, i.e., the timescale of decay of the function, which captures the learning rate parameter, appears unchanged. Together, these results indicate that the DATkd mice, choice-by-choice, adapt their choices to recent rewards with a similar temporal profile, but that recent rewards exhibit an overall less profound influence on their behavior, resulting in diminished coupling between temporally local rates of reinforcement and decision-making.
Discussion
Though dopamine has been studied for decades, its impact on adaptive behavior in complex, naturalistic environments can difficult to infer in the absence of paradigms designed specifically to examine adaptation to environmental conditions. The paradigm used here trades the highly controlled approach of traditional behavior testing for a semi-naturalistic design that generates a rich dataset against which different models and hypotheses can be examined (and generated) and in the process eliminates many difficult to address confounds such as the impact of food restriction, handling, time of testing, and so on.
In the present study, we used a closed-economy, homecage paradigm to ask if elevated tonic dopamine alters the animals’ flexible adaptation to changing environmental reward contingencies. When shifting reward contingencies between the levers is introduced, wild-type mice distribute more effort to the currently less expensive lever, increasing yield for energy expended. In contrast, the hyperdopaminergic mice distribute their effort approximately equally between the levers, apparently less influenced by the relative cost of the two levers. As a consequence, on average they expend more effort for each pellet earned than wild-type mice. In this paradigm, however, little is gained by this effort. Data from low-cost baseline, when both levers function at the same cost, and from a non-switching version of the task, indicate that the differences observed between genotypes cannot be attributed to differences in baseline consumption, generalized effects of activity level, differences in motor performance, or an intrinsic valuation of lever pressing. Rather, the observed difference arises specifically as a consequence of on-going adaptation to a dynamic environment.
Discerning Alterations in Reinforcement Learning (Acquisition) from Changes in Motivation (Expression)
A fundamental debate is whether dopamine influences behavior through reinforcement learning or by modulating the expression of motivated behavior (Wise, 2004; Salamone, 2006; Berridge, 2007). Accumulating data support both perspectives; however, distinguishing the relative contribution of learning versus expression to adaptive behavior and integrating these two roles into a comprehensive framework remain elusive. To disentangle these two potential influences on adaptive behavior, we ask how dopamine alters the updating and utilization of incentive values in decision-making, on a choice-by-choice basis, in response to shifting environmental contingencies and reward outcomes. By fitting the data to the computational model at the heart of reinforcement learning theories of dopamine (Montague et al., 1996; Schultz et al., 1997; Sutton and Barto, 1998), we find that elevated tonic dopamine does not alter learning, as reflected in the learning rate parameter, but does alter the expression of that learning, as reflected by the temperature parameter, which modulates the degree to which prior reward biases action selection. Surprisingly, the DATkd mice are less influenced by recent reward resulting in diminished coupling between on-going reward information and behavioral choice.
It has been suggested that tonic and phasic dopamine may serve different functions (Schultz, 2007b), with tonic contributing to the scaling of motivated behavior (Cagniard et al., 2006b; Berridge, 2007; Salamone, 2007) while phasic provides a prediction error signal critical to learning (Schultz et al., 1993, 1997; Schultz and Dickinson, 2000). Consistent with previous work (Zhuang et al., 2001; Cagniard et al., 2006a,b; Yin et al., 2006), the current study supports this view as the DATkd mice retain phasic dopamine activity (Zhuang et al., 2001; Cagniard et al., 2006b) and show no alterations in learning. In contrast, we show for the first time that tonic dopamine can alter the temperature parameter in a temporal difference RL model, which suggests a mechanism by which the expression of motivated behavior may be modulated or scaled by dopamine within a common framework with its role in reinforcement learning.
Functional Accounts of Dopamine
In contrast to theories that focus on dopamine’s role in reward learning, associated with phasic activity (but see Gutkin et al., 2006; Palmiter, 2008; Zweifel et al., 2009), tonic dopamine has been associated with motivational accounts of dopamine function whereby dopamine increases an animal’s energy expenditure toward a goal. The effects of dopamine on motivation have been characterized as enhanced incentive or “wanting”(Berridge, 2007), decreased sensitivity to cost (Aberman and Salamone, 1999; Salamone et al., 2001; Mingote et al., 2005), “scaling” of reinforced responding (Cagniard et al., 2006b) or as a mediator of “vigor” (Lyons and Robbins, 1975; Taylor and Robbins, 1984; Niv et al., 2007).
In one attempt to formalize these ideas and reconcile them with RL models of phasic dopamine, Niv et al. (2007) proposed that instrumental actions actually involve two separate decisions: what to do (the choice between actions), and when (or how vigorously) to do it. They suggested, moreover, that phasic dopamine might affect choice of “what to do” via learning while tonic dopamine would modulate the vigor of the chosen action, as an expression effect. In the present study, the DATkd genotype show altered choices between levers, suggesting that tonic dopamine can, independent of learning, affect choice of what to do as well as the vigor with which a choice is pursued (see also Salamone et al., 2003).
The most straightforward and mechanistic interpretation of the data is that tonic dopamine modulates the gain in action selection mechanisms (Servan-Schreiber et al., 1990; Braver et al., 1999). Dopamine affects cellular and synaptic processes widely throughout the brain (Hsu et al., 1995; Kiyatkin and Rebec, 1996; Flores-Hernandez et al., 1997; Nicola et al., 2000; Cepeda et al., 2001; Horvitz, 2002; Reynolds and Wickens, 2002; Bamford et al., 2004; Goto and Grace, 2005a, b; Calabresi et al., 2007; Wu et al., 2007; Kheirbek et al., 2008; Wickens, 2009), especially in the striatum, believed to be central in action selection (Mogenson et al., 1980; Mink, 1996; Redgrave et al., 1999). Activation of D2 receptors on corticostriatal terminals has been shown to filter cortical input (Cepeda et al., 2001; Bamford et al., 2004) and activation of D1 receptors on striatal medium spiny neurons (MSNs) can provide a gain function by altering the threshold for switching from the down-state to the up-state while facilitating responsiveness of those MSNs already in the up-state (Nicola et al., 2000). Consequently, dopamine is positioned to modulate the processing of information flowing through the striatum by modulating both plasticity and gain (or temperature), reflecting a dopaminergic role in learning and expression of learning, respectively (Braver et al., 1999). This hypothesis, that tonic dopamine modulates gain on corticostriatal processing thereby regulating the temperature at which learned expected values influence action selection, would explain how tonic dopamine could affect both choice of “what to do” and the “scaling” of the expression of learned, reinforced behavioral responses.
Insofar as functional aspects of behavior, such as incentive and cost (or exploration, performance, uncertainty, and so on) are processed through the striatum, a temperature/gain regulation function of dopamine would alter these functional aspects of behavior. However, the functional effects and the underlying mechanism need not be co-extensive. Depending upon the input, task or specific anatomical region manipulated, a temperature modulation function might have seemingly distinct functional effects on behavior (Braver et al., 1999). Though response selection in striatum is particularly associated with its dorsolateral region and incentive processing with ventral regions, the nucleus accumbens in particular (Humphries and Prescott, 2010; Nicola, 2007), in the present study, we cannot discern which striatal compartment contributes to the observed phenotype. Determining the unique contribution of the ventral and dorsal striatum to behavioral flexibility will require further studies.
The notion that dopamine may change the expression of motivated behavior by altering the gain operating on the processing of either cost or incentive is consistent with previous theories of dopaminergic function (Salamone and Correa, 2002; Berridge, 2007). However, discerning whether dopamine operates on costs, incentive value or both may ultimately require greater understanding of the precise neural representation of these functional constructs.
For example, Rushworth and colleagues (Rudebeck et al., 2006) have suggested that tracking of delay- and effort-based costs are mediated by the orbitofrontal and anterior cingulate cortices, respectively, both of which project to the ventral striatum. Shidara and colleagues (Shidara et al., 1998, 2005; Shidara and Richmond, 2004) provide data that the anterior cingulate processes reward expectancy and that the ventral striatum tracks progress toward a reward. Presumably such information maintains focus on a goal, favoring task-related action selection during the exertion of effort or across a temporal delay. This would give rise to an apparent reduced sensitivity to costs though the underlying mechanism would be an enhanced representation of progress toward a goal. A mechanism such as this would equally support dopamine theories of enhanced incentive and reduced sensitivity to costs, both of which arise as a consequence of dopaminergic modulation of gain in corticostriatal processing of information modulating action selection. Importantly, though, in this view dopamine is not modulating incentive value or cost sensitivity per se, but the gain in action selection processing which alters the influence of incentive or costs on behavioral choice.
Dopamine and the Regulation of Exploration and Exploitation
It is curious that increased tonic dopamine diminishes coupling between choice and reward history when one might expect an enhanced gain function to make an organism more sensitive to recent reward and to marginal contrasts between putative values of two choices. However, the effects of changing concentrations of dopamine in various brain regions associated with different functions have been often characterized by an inverted U shaped curve (Seamans et al., 1998; Williams and Dayan, 2005; Delaveau et al., 2007; Vijayraghavan et al., 2007; Clatworthy et al., 2009; Monte-Silva et al., 2009; Schellekens et al., 2010) such that too much dopamine may effectively reduce gain as observed on the behavioral level. One reason for this might be saturation in realistic neural representations: although in the model, gain can be increased without bound, in the brain, too much dopamine might ultimately wash out fine discriminations due to saturation. As a consequence, only middle ranges of extracellular dopamine would provide optimal gain for exploiting prior learning. In contrast, low dopamine would diminish exploitation resulting in generalized, non-goal- and task-related exploration while high dopamine would facilitate exploration between established, goal- and task-related options.
Because it modulates the connection between value and choice, the gain mechanism embodied by the softmax temperature in reinforcement learning models is often identified with regulating the balance between exploration and exploitation. If tonic dopamine affects this temperature, then it might, functionally, be involved in regulating exploration by modulating the degree to which prior learning biases action selection; that is, by controlling the degree of exploitation. Dopamine may not be unique in modulating the balance between exploration and exploitation; other accounts have associated exploration with top-down control from anterior frontal cortex (Daw et al., 2006) and/or with temperature regulation by another monoamine neuromodulator, norepinephrine (Aston-Jones and Cohen, 2005a,b).
Dopamine and Behavioral Flexibility
The ability to flexibly deploy and modify learned behaviors in response to a changing environment is critical to adaptation. Though the PFC is widely associated with behavioral flexibility, considerable data suggest that flexibility arises from a cortico-striatal circuit in which both cortical and subcortical regions contribute important components to flexible behavior (Cools et al., 2004; Frank and Claus, 2006; Lo and Wang, 2006; Hazy et al., 2007; Floresco et al., 2009; Haluk and Floresco, 2009; Pennartz et al., 2009; Kehagia et al., 2010). In the present study, it is possible that changed dopamine in the PFC contributed to the observed phenotype. Xu et al. (2009) recently reported that DAT knock-out mice (DATko) lack LTP in prefrontal pyramidal cells. However, the knock-out line used in that study and the knock-down used here differ significantly making it difficult to draw inferences from one line to the other. The DATko phenotype is more severe and complicated with developmental abnormalities, including growth retardation, pituitary hypoplasia, lactation deficits, and high mortality (Bosse et al., 1997), none of which occur in the knock-down line used here (Zhuang et al., 2001). More importantly, the DATko, consistent with a loss of PFC LTP, show learning, and memory deficits (Giros et al., 1996; Gainetdinov et al., 1999; Morice et al., 2007; Weiss et al., 2007; Dzirasa et al., 2009). In contrast, learning has been shown to be normal in the DATkd (Cagniard et al., 2006a,b; Yin et al., 2006), including in the present study. Moreover, the weight of evidence suggest that dopamine reuptake in the PFC is mediated primarily by the norepinephrine transporter (NET) rather than DAT, suggesting that a knockdown of DAT would not significantly alter the kinetics of reuptake in the PFC (Sesack et al., 1998; Mundorf et al., 2001; Moron et al., 2002). In contrast, the changes in dopamine dynamics in the striatum are pronounced and well documented (Zhuang et al., 2001; Cagniard et al., 2006b).
It is unlikely that behavioral flexibility is localized specifically to any single anatomical region; rather, flexibility is likely an emergent property arising from interdependent interaction between structures within circuits. For example, Kellendonk et al. (2006) demonstrate that overexpression of D2 receptors in the striatum can alter PFC function. From this perspective, we would expect that the PFC does contribute to the observed phenotype because it is an integral component of the corticostriatal circuit mediating choice behavior. In the present study, however, the weight of evidence supports the notion that potential changes in PFC function arise as a consequence of alterations in dopaminergic tone in the striatum rather than in the PFC directly, consistent with the widely held view that the striatum critically mediates reward learning and action selection. To this we add the suggestion that striatal dopamine may contribute to behavioral flexibility by modulating the degree to which prior learning is or is not exploited.
Distinguishing the Contribution of Tonic and Phasic Dopamine
Dopamine cells have been characterized as having two primary modes (Grace and Bunney, 1984a,b), tonic (slow, irregular pacemaker activity), and phasic (short bursts of high frequency spikes). Experimentally isolating and manipulating these to investigate their putatively distinct functions remains a significant challenge. When DAT expression is reduced, the amplitude of dopamine release from evoked stimulation is reduced to 25% of wild-type (Zhuang et al., 2001). Despite this reduced release, the effect on tonic dopamine is robust and clear, resulting in both increased rate of tonic activity and elevated extracellular dopamine in the striatum (Zhuang et al., 2001; Cagniard et al., 2006b). In contrast, phasic activity itself remains unaltered (Cagniard et al., 2006b).
Though phasic activity itself remains intact, the impact of reduced amplitude of release during that activity is uncertain. That is, it is possible that reduced dopamine during phasic release might underlie the observed phenotype rather than increased tonic activity. The weight of evidence argues against this. Phasic activity is most widely associated with mediating a prediction error during reward learning (Schultz et al., 1997), with evidence that the magnitude of phasic activity correlates to the magnitude of unexpected reward (Tobler et al., 2005). However, we observe no alterations in reward learning. Dopamine has also been associated with energizing and mobilizing reward oriented appetitive behavior, but we observe no reduction in motivation and effort.
Bergman and colleagues (Joshua et al., 2009) suggests that phasic dopamine activity itself may be composed of two components: a fast phase that serves an activational function and a more prolonged, slow phase that modulates plasticity. It is intriguing to consider that a reduction in the amplitude of putative fast phase activity may result in less activation and gain of learned values, effectively reducing the bias of prior learning on choice, as observed here. However, in the present study the mice have extensive experience with the lever and reward contingencies. The literature on phasic dopamine suggests that bursting should occur primarily during unexpected outcomes, such as contingency switches in this task. However, it is precisely around these switches that the WT and DATkd behavior is similar while differences in choice are observed primarily during the stable periods between contingency switches. Thus, though we cannot conclusively rule out a potential role for reduced amplitude of phasic release in the phenotype observed here, the weight of evidence points to the pronounced changes in tonic dopamine as the critical factor.
Though dopamine is often associated with greater motivation, willingness to work, and persistence in pursuing a goal, the present study suggests a potential trade-off between such enhanced motivation and flexibility. The relative value of persistence and flexibility will depend upon the environment. Consequently, polymorphisms in genes regulating dopamine function (D’Souza and Craig, 2008; Frank et al., 2009; Le Foll et al., 2009; Marco-Pallares et al., 2009) may have evolved from evolutionary pressures arising from different environments. In some environments, extraordinary persistence (exploitation of prior learning) may be essential for survival. In other environments, exploration is essential and persistence with a previously, but not currently, successful action wastes energy. Genetic diversity in dopamine function may afford enhanced adaptive survival by providing a range of phylogenetic solutions to the problem of determining the degree to which an organism should base future behavior on past outcomes, a vexing challenge in adaption for any organism.
Conflict of Interest Statement
The authors declare that the research was conducted in the absence of any commercial or financial relationships that could be construed as a potential conflict of interest.
Acknowledgments
This work was supported by NIDA, DA25875 (Jeff A. Beeler), and NIMH, MH066216 (Xiaoxi Zhuang), a Scholar Award from the McKnight Foundation (Nathaniel Daw) and a NARSAD Young Investigator Award (Nathaniel Daw).
References
Aberman, J. E., and Salamone, J. D. (1999). Nucleus accumbens dopamine depletions make rats more sensitive to high ratio requirements but do not impair primary food reinforcement. Neuroscience 92, 545–552.
Aston-Jones, G., and Cohen, J. D. (2005a). Adaptive gain and the role of the locus coeruleus-norepinephrine system in optimal performance. J. Comp. Neurol. 493, 99–110.
Aston-Jones, G., and Cohen, J. D. (2005b). An integrative theory of locus coeruleus-norepinephrine function: adaptive gain and optimal performance. Annu. Rev. Neurosci. 28, 403–450.
Bamford, N. S., Zhang, H., Schmitz, Y., Wu, N. P., Cepeda, C., Levine, M. S., Schmauss, C., Zakharenko, S. S., Zablow, L., and Sulzer, D. (2004). Heterosynaptic dopamine neurotransmission selects sets of corticostriatal terminals. Neuron 42, 653–663.
Belin, D., and Everitt, B. J. (2008). Cocaine seeking habits depend upon dopamine-dependent serial connectivity linking the ventral with the dorsal striatum. Neuron 57, 432–441.
Berke, J. D., and Hyman, S. E. (2000). Addiction, dopamine, and the molecular mechanisms of memory. Neuron 25, 515–532.
Berridge, K. C., (2007). The debate over dopamine’s role in reward: the case for incentive salience. Psychopharmacology (Berl.) 191, 391–431.
Bosse, R., Fumagalli, F., Jaber, M., Giros, B., Gainetdinov, R. R., Wetsel, W. C., Missale, C., and Caron, M. G. (1997). Anterior pituitary hypoplasia and dwarfism in mice lacking the dopamine transporter. Neuron 19, 127–138.
Braver, T. S., Barch, D. M., and Cohen, J. D. (1999). Cognition and control in schizophrenia: a computational model of dopamine and prefrontal function. Biol. Psychiatry 46, 312–328.
Cagniard, B., Balsam, P. D., Brunner, D., and Zhuang, X. (2006a). Mice with chronically elevated dopamine exhibit enhanced motivation, but not learning, for a food reward. Neuropsychopharmacology 31, 1362–1370.
Cagniard, B., Beeler, J. A., Britt, J. P., McGehee, D. S., Marinelli, M., and Zhuang, X. (2006b). Dopamine scales performance in the absence of new learning. Neuron 51, 541–547.
Calabresi, P., Picconi, B., Tozzi, A., and Di Filippo, M. (2007). Dopamine-mediated regulation of corticostriatal synaptic plasticity. Trends Neurosci. 30, 211–219.
Camerer, C., and Ho, T.-H. (1999). Experience-weighted attraction learning in normal form games. Econometrica 67, 827–874.
Cepeda, C., Hurst, R. S., Altemus, K. L., Flores-Hernandez, J., Calvert, C. R., Jokel, E. S., Grandy, D. K., Low, M. J., Rubinstein, M., Ariano, M. A., and Levine, M. S. (2001). Facilitated glutamatergic transmission in the striatum of D2 dopamine receptor-deficient mice. J. Neurophysiol. 85, 659–670.
Clatworthy, P. L., Lewis, S. J., Brichard, L., Hong, Y. T., Izquierdo, D., Clark, L., Cools, R., Aigbirhio, F. I., Baron, J. C., Fryer, T. D., and Robbins, T. W. (2009). Dopamine release in dissociable striatal subregions predicts the different effects of oral methylphenidate on reversal learning and spatial working memory. J. Neurosci. 29, 4690–4696.
Cohen, J. D., McClure, S. M., and Yu, A. J. (2007). Should I stay or should I go? How the human brain manages the trade-off between exploitation and exploration. Philos. Trans. R. Soc. Lond. 362, 933–942.
Cools, R., Clark, L., and Robbins, T. W. (2004). Differential responses in human striatum and prefrontal cortex to changes in object and rule relevance. J. Neurosci. 24, 1129–1135.
Corrado, G. S., Sugrue, L. P., Seung, H. S., and Newsome, W. T. (2005). Linear-nonlinear-Poisson models of primate choice dynamics. J. Exp. Anal. Behav. 84, 581–617.
D’Souza, U. M., and Craig, I. W. (2008). Functional genetic polymorphisms in serotonin and dopamine gene systems and their significance in behavioural disorders. Prog. Brain Res. 172, 73–98.
Daw, N. D., and Doya, K. (2006). The computational neurobiology of learning and reward. Curr. Opin. Neurobiol. 16, 199–204.
Daw, N. D., O’Doherty, J. P., Dayan, P., Seymour, B., and Dolan, R. J. (2006). Cortical substrates for exploratory decisions in humans. Nature 441, 876–879.
Day, J. J., Roitman, M. F., Wightman, R. M., and Carelli, R. M. (2007). Associative learning mediates dynamic shifts in dopamine signaling in the nucleus accumbens. Nat. Neurosci. 10, 1020–1028.
Dayan, P., and Balleine, B. W. (2002). Reward, motivation, and reinforcement learning. Neuron 36, 285–298.
Delaveau, P., Salgado-Pineda, P., Micallef-Roll, J., and Blin, O. (2007). Amygdala activation modulated by levodopa during emotional recognition processing in healthy volunteers: a double-blind, placebo-controlled study. J. Clin. Psychopharmacol. 27, 692–697.
Dzirasa, K., Ramsey, A. J., Takahashi, D. Y., Stapleton, J., Potes, J. M., Williams, J. K., Gainetdinov, R. R., Sameshima, K., Caron, M. G., and Nicolelis, M. A. (2009). Hyperdopaminergia and NMDA receptor hypofunction disrupt neural phase signaling. J. Neurosci. 29, 8215–8224.
Flores-Hernandez, J., Galarraga, E., and Bargas, J. (1997). Dopamine selects glutamatergic inputs to neostriatal neurons. Synapse (New York, NY) 25, 185–195.
Floresco, S. B., Zhang, Y., and Enomoto, T. (2009). Neural circuits subserving behavioral flexibility and their relevance to schizophrenia. Behav. Brain Res. 204, 396–409.
Frank, M. J., and Claus, E. D. (2006). Anatomy of a decision: striato-orbitofrontal interactions in reinforcement learning, decision making, and reversal. Psychol. Rev. 113, 300–326.
Frank, M. J., Doll, B. B., Oas-Terpstra, J., and Moreno, F. (2009). Prefrontal and striatal dopaminergic genes predict individual differences in exploration and exploitation. Nat. Neurosci. 12, 1062–1068.
Gainetdinov, R. R., Wetsel, W. C., Jones, S. R., Levin, E. D., Jaber, M., and Caron, M. G. (1999). Role of serotonin in the paradoxical calming effect of psychostimulants on hyperactivity. Science (New York, NY) 283, 397–401.
Giros, B., Jaber, M., Jones, S. R., Wightman, R. M., and Caron, M. G. (1996). Hyperlocomotion and indifference to cocaine and amphetamine in mice lacking the dopamine transporter. Nature 379, 606–612.
Goto, Y., and Grace, A. A. (2005a). Dopamine-dependent interactions between limbic and prefrontal cortical plasticity in the nucleus accumbens: disruption by cocaine sensitization. Neuron 47, 255–266.
Goto, Y., and Grace, A. A. (2005b). Dopaminergic modulation of limbic and cortical drive of nucleus accumbens in goal-directed behavior. Nat. Neurosci. 8, 805–812.
Grace, A. A., and Bunney, B. S. (1984a). The control of firing pattern in nigral dopamine neurons: burst firing. J. Neurosci. 4, 2877–2890.
Grace, A. A., and Bunney, B. S. (1984b). The control of firing pattern in nigral dopamine neurons: single spike firing. J. Neurosci. 4, 2866–2876.
Gutkin, B. S., Dehaene, S., and Changeux, J. P. (2006). A neurocomputational hypothesis for nicotine addiction. Proc. Natl. Acad. Sci. U.S.A. 103, 1106–1111.
Haluk, D. M., and Floresco, S. B. (2009). Ventral striatal dopamine modulation of different forms of behavioral flexibility. Neuropsychopharmacology 34, 2041–2052.
Hazy, T. E., Frank, M. J., and O’Reilly, R. C. (2007). Towards an executive without a homunculus: computational models of the prefrontal cortex/basal ganglia system. Philos. Trans. R. Soc. Lond. B. Biol. Sci. 362, 1601–1613.
Horvitz, J. C., (2002). Dopamine gating of glutamatergic sensorimotor and incentive motivational input signals to the striatum. Behav. Brain Res. 137, 65–74.
Hsu, K. S., Huang, C. C., Yang, C. H., and Gean, P. W. (1995). Presynaptic D2 dopaminergic receptors mediate inhibition of excitatory synaptic transmission in rat neostriatum. Brain Res. 690, 264–268.
Humphries, M. D., and Prescott, T. J. (2010). The ventral basal ganglia, a selection mechanism at the crossroads of space, strategy, and reward. Prog. Neurobiol. 90, 385–417.
Joshua, M., Adler, A., and Bergman, H. (2009). The dynamics of dopamine in control of motor behavior. Curr. Opin. Neurobiol. 19, 615–620.
Kehagia, A. A., Murray, G. K., and Robbins, T. W. (2010). Learning and cognitive flexibility: frontostriatal function and monoaminergic modulation. Curr. Opin. Neurobiol. 20, 199–204.
Kellendonk, C., Simpson, E. H., Polan, H. J., Malleret, G., Vronskaya, S., Winiger, V., Moore, H., and Kandel, E. R. (2006). Transient and selective overexpression of dopamine D2 receptors in the striatum causes persistent abnormalities in prefrontal cortex functioning. Neuron 49, 603–615.
Kheirbek, M. A., Beeler, J. A., Ishikawa, Y., and Zhuang, X. (2008). A cAMP pathway underlying reward prediction in associative learning. J. Neurosci. 28, 11401–11408.
Kiyatkin, E. A., and Rebec, G. V. (1996). Dopaminergic modulation of glutamate-induced excitations of neurons in the neostriatum and nucleus accumbens of awake, unrestrained rats. J. Neurophysiol. 75, 142–153.
Lau, B., and Glimcher, P. W. (2005). Dynamic response-by-response models of matching behavior in rhesus monkeys. J. Exp. Anal. Behav. 84, 555–579.
Le Foll, B., Gallo, A., Le Strat, Y., Lu, L., and Gorwood, P. (2009). Genetics of dopamine receptors and drug addiction: a comprehensive review. Behav. Pharmacol. 20, 1–17.
Lo, C. C., and Wang, X. J. (2006). Cortico-basal ganglia circuit mechanism for a decision threshold in reaction time tasks. Nat. Neurosci. 9, 956–963.
Lyons, M., and Robbins, T. W. (1975) “The action of central nervous system stimulant drugs: a general theory concerning amphetamine effects,” in Current Developments in Psychopharmacology, Vol. 2, ed W. Essman, (New York: Spectrum), 79–163.
Marco-Pallares, J., Cucurell, D., Cunillera, T., Kramer, U. M., Camara, E., Nager, W., Bauer, P., Schule, R., Schols, L., Munte, T. F., and Rodriguez-Fornells, A. (2009). Genetic variability in the dopamine system (dopamine receptor D4, catechol-O-methyltransferase) modulates neurophysiological responses to gains and losses. Biol. Psychiatry. 66, 154–161.
Mingote, S., Weber, S. M., Ishiwari, K., Correa, M., and Salamone, J. D. (2005). Ratio and time requirements on operant schedules: effort-related effects of nucleus accumbens dopamine depletions. Eur. J. Neurosci. 21, 1749–1757.
Mink, J. W., (1996). The basal ganglia: focused selection and inhibition of competing motor programs. Prog. Neurobiol. 50, 381–425.
Mogenson, G. J., Jones, D. L., and Yim, C. Y. (1980). From motivation to action: functional interface between the limbic system and the motor system. Prog. Neurobiol. 14, 69–97.
Montague, P. R., Dayan, P., and Sejnowski, T. J. (1996). A framework for mesencephalic dopamine systems based on predictive Hebbian learning. J. Neurosci. 16, 1936–1947.
Monte-Silva, K., Kuo, M. F., Thirugnanasambandam, N., Liebetanz, D., Paulus, W., and Nitsche, M. A. (2009). Dose-dependent inverted U-shaped effect of dopamine (D2-like) receptor activation on focal and nonfocal plasticity in humans. J. Neurosci. 29, 6124–6131.
Morice, E., Billard, J. M., Denis, C., Mathieu, F., Betancur, C., Epelbaum, J., Giros, B., and Nosten-Bertrand, M. (2007). Parallel loss of hippocampal LTD and cognitive flexibility in a genetic model of hyperdopaminergia. Neuropsychopharmacology 32, 2108–2116.
Moron, J. A., Brockington, A., Wise, R. A., Rocha, B. A., and Hope, B. T. (2002). Dopamine uptake through the norepinephrine transporter in brain regions with low levels of the dopamine transporter: evidence from knock-out mouse lines. J. Neurosci. 22, 389–395.
Mundorf, M. L., Joseph, J. D., Austin, C. M., Caron, M. G., and Wightman, R. M. (2001). Catecholamine release and uptake in the mouse prefrontal cortex. J. Neurochem. 79, 130–142.
Nicola, S. M., (2007). The nucleus accumbens as part of a basal ganglia action selection circuit. Psychopharmacology (Berl.) 191, 521–550.
Nicola, S. M., Surmeier, J., and Malenka, R. C. (2000). Dopaminergic modulation of neuronal excitability in the striatum and nucleus accumbens. Annu. Rev. Neurosci. 23, 185–215.
Niv, Y., Daw, N. D., Joel, D., and Dayan, P. (2007). Tonic dopamine: opportunity costs and the control of response vigor. Psychopharmacology (Berl.) 191, 507–520.
Palmiter, R. D., (2008). Dopamine signaling in the dorsal striatum is essential for motivated behaviors: lessons from dopamine-deficient mice. Ann. N. Y. Acad. Sci. 1129, 35–46.
Pecina, S., Cagniard, B., Berridge, K. C., Aldridge, J. W., and Zhuang, X. (2003). Hyperdopaminergic mutant mice have higher “wanting” but not “liking” for sweet rewards. J. Neurosci. 23, 9395–9402.
Pennartz, C. M., Berke, J. D., Graybiel, A. M., Ito, R., Lansink, C. S., van der Meer, M., Redish, A. D., Smith, K. S., and Voorn, P. (2009). Corticostriatal Interactions during Learning, Memory Processing, and Decision Making. J. Neurosci. 29, 12831–12838.
Phillips, P. E., Walton, M. E., and Jhou, T. C. (2007). Calculating utility: preclinical evidence for cost-benefit analysis by mesolimbic dopamine. Psychopharmacology (Berl.) 191, 483–495.
Redgrave, P., Prescott, T. J., and Gurney, K. (1999). The basal ganglia: a vertebrate solution to the selection problem? Neuroscience 89, 1009–1023.
Reynolds, J. N., and Wickens, J. R. (2002). Dopamine-dependent plasticity of corticostriatal synapses. Neural. Netw. 15, 507–521.
Rowland, N. E., Vaughan, C. H., Mathes, C. M., and Mitra, A. (2008). Feeding behavior, obesity, and neuroeconomics. Physiol. Behav. 93, 97–109.
Rudebeck, P. H., Walton, M. E., Smyth, A. N., Bannerman, D. M., and Rushworth, M. F. (2006). Separate neural pathways process different decision costs. Nat. Neurosci. 9, 1161–1168.
Salamone, J. D., (2006). Will the last person who uses the term reward’ please turn out the lights? Comments on processes related to reinforcement, learning, motivation and effort. Addict. Biol. 11, 43–44.
Salamone, J. D., (2007). Functions of mesolimbic dopamine: changing concepts and shifting paradigms. Psychopharmacology (Berl.) 191, 389.
Salamone, J. D., and Correa, M. (2002). Motivational views of reinforcement: implications for understanding the behavioral functions of nucleus accumbens dopamine. Behav. Brain Res. 137, 3–25.
Salamone, J. D., Correa, M., Mingote, S., and Weber, S. M. (2003). Nucleus accumbens dopamine and the regulation of effort in food-seeking behavior: implications for studies of natural motivation, psychiatry, and drug abuse. J. Pharmacol. Exp. Ther. 305, 1–8.
Salamone, J. D., Wisniecki, A., Carlson, B. B., and Correa, M. (2001). Nucleus accumbens dopamine depletions make animals highly sensitive to high fixed ratio requirements but do not impair primary food reinforcement. Neuroscience 105, 863–870.
Schellekens, A. F., Grootens, K. P., Neef, C., Movig, K. L., Buitelaar, J. K., Ellenbroek, B., and Verkes, R. J. (2010). Effect of apomorphine on cognitive performance and sensorimotor gating in humans. Psychopharmacology 207, 559–569.
Schultz, W. (2007b). Multiple dopamine functions at different time courses. Annu. Rev. Neurosci. 30, 259–288.
Schultz, W., Apicella, P., and Ljungberg, T. (1993). Responses of monkey dopamine neurons to reward and conditioned stimuli during successive steps of learning a delayed response task. J. Neurosci. 13, 900–913.
Schultz, W., Dayan, P., and Montague, P. R. (1997). A neural substrate of prediction and reward. Science (New York, NY) 275, 1593–1599.
Schultz, W., and Dickinson, A. (2000). Neuronal coding of prediction errors. Annu. Rev. Neurosci. 23, 473–500.
Seamans, J. K., Floresco, S. B., and Phillips, A. G. (1998). D1 receptor modulation of hippocampal-prefrontal cortical circuits integrating spatial memory with executive functions in the rat. J. Neurosci. 18, 1613–1621.
Servan-Schreiber, D., Printz, H., and Cohen, J. D. (1990). A network model of catecholamine effects: gain, signal-to-noise ratio, and behavior. Science (New York, NY) 249, 892–895.
Sesack, S. R., Hawrylak, V. A., Guido, M. A., and Levey, A. I. (1998). Cellular and subcellular localization of the dopamine transporter in rat cortex. Adv. Pharmacol. (San Diego, CA) 42, 171–174.
Shidara, M., Aigner, T. G., and Richmond, B. J. (1998). Neuronal signals in the monkey ventral striatum related to progress through a predictable series of trials. J. Neurosci. 18, 2613–2625.
Shidara, M., Mizuhiki, T., and Richmond, B. J. (2005). Neuronal firing in anterior cingulate neurons changes modes across trials in single states of multitrial reward schedules. Exp. Brain Res. 163, 242–245.
Shidara, M., and Richmond, B. J. (2004). Differential encoding of information about progress through multi-trial reward schedules by three groups of ventral striatal neurons. Neurosci. Res. 49, 307–314.
Sugrue, L. P., Corrado, G. S., and Newsome, W. T. (2004). Matching behavior and the representation of value in the parietal cortex. Science (New York, NY) 304, 1782–1787.
Sutton, R., and Barto, A. (1998). Reinforcement Learning: An Introduction. Cambridge, MA: MIT Press.
Taylor, J. R., and Robbins, T. W. (1984). Enhanced behavioural control by conditioned reinforcers following microinjections of d-amphetamine into the nucleus accumbens. Psychopharmacology (Berl.) 84, 405–412.
Tobler, P. N., Fiorillo, C. D., and Schultz, W. (2005). Adaptive coding of reward value by dopamine neurons. Science (New York, NY) 307, 1642–1645.
Vijayraghavan, S., Wang, M., Birnbaum, S. G., Williams, G. V., and Arnsten, A. F. (2007). Inverted-U dopamine D1 receptor actions on prefrontal neurons engaged in working memory. Nat. Neurosci. 10, 376–384.
Weiss, S., Nosten-Bertrand, M., McIntosh, J. M., Giros, B., and Martres, M. P. (2007). Nicotine improves cognitive deficits of dopamine transporter knockout mice without long-term tolerance. Neuropsychopharmacology 32, 2465–2478.
Williams, J., and Dayan, P. (2005). Dopamine, learning, and impulsivity: a biological account of attention-deficit/hyperactivity disorder. J. Child Adolesc. Psychopharmacol. 15, 160–179; discussion 157–169.
Wu, N., Cepeda, C., Zhuang, X., and Levine, M. S. (2007). Altered corticostriatal neurotransmission and modulation in dopamine transporter knock-down mice. J. Neurophysiol. 98, 423–432.
Xu, T. X., Sotnikova, T. D., Liang, C., Zhang, J., Jung, J. U., Spealman, R. D., Gainetdinov, R. R., and Yao, W. D. (2009). Hyperdopaminergic tone erodes prefrontal long-term potential via a D2 receptor-operated protein phosphatase gate. J. Neurosci. 29, 14086–14099.
Yin, H. H., Zhuang, X., and Balleine, B. W. (2006). Instrumental learning in hyperdopaminergic mice. Neurobiol. Learn. Mem. 85, 283–288.
Zhuang, X., Oosting, R. S., Jones, S. R., Gainetdinov, R. R., Miller, G. W., Caron, M. G., and Hen, R. (2001). Hyperactivity and impaired response habituation in hyperdopaminergic mice. Proc. Natl. Acad. Sci. U.S.A. 98, 1982–1987.
Zweifel, L. S., Parker, J. G., Lobb, C. J., Rainwater, A., Wall, V. Z., Fadok, J. P., Darvas, M., Kim, M. J., Mizumori, S. J., Paladini, C. A., Phillips, P. E., and Palmiter, R. D. (2009). Disruption of NMDAR-dependent burst firing by dopamine neurons provides selective assessment of phasic dopamine-dependent behavior. Proc. Natl. Acad. Sci. U.S.A. 106, 7281–7288.
Keywords: dopamine, reinforcement learning, DAT knock-down, explore-exploit, behavioral flexibility, environmental adaptation
Citation: Beeler JA, Daw N, Frazier CRM and Zhuang X (2010) Tonic dopamine modulates exploitation of reward learning. Front. Behav. Neurosci. 4:170. doi: 10.3389/fnbeh.2010.00170
Received: 13 July 2010;
Accepted: 11 October
2010;
Published online: 04 November 2010.
Edited by:
Julietta U. Frey, Leibniz Institute for Neurobiology, GermanyReviewed by:
Satoru Otani, University of Paris VI, FranceKatharina A. Braun, OttoVonGuericke University, Germany
Copyright: © 2010 Beeler, Daw, Frazier and Zhuang. This is an open-access article subject to an exclusive license agreement between the authors and the Frontiers Research Foundation, which permits unrestricted use, distribution, and reproduction in any medium, provided the original authors and source are credited.
*Correspondence: Jeff Beeler, Department of Neurobiology, The University of Chicago, 924 E 57th Street R222, Chicago, IL 60637, USA. e-mail:amFiZWVsZXJAdWNoaWNhZ28uZWR1