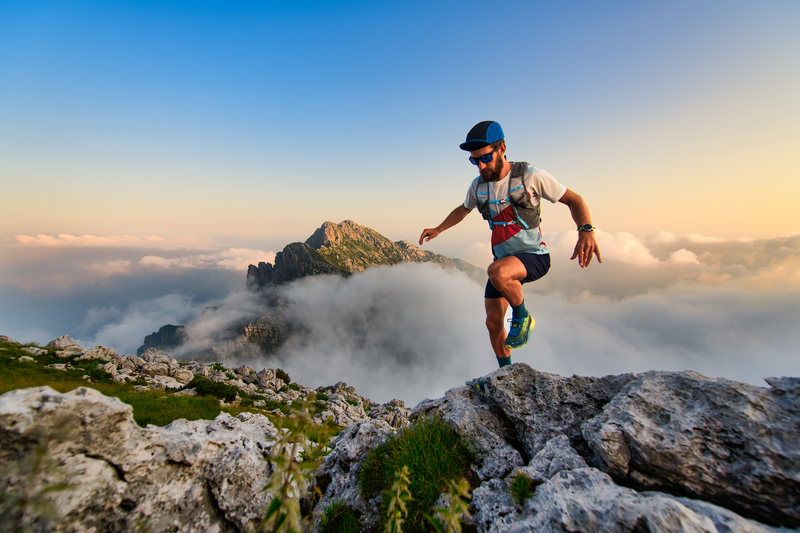
95% of researchers rate our articles as excellent or good
Learn more about the work of our research integrity team to safeguard the quality of each article we publish.
Find out more
MINI REVIEW article
Front. Behav. Econ. , 28 October 2024
Sec. Behavioral Microfoundations
Volume 3 - 2024 | https://doi.org/10.3389/frbhe.2024.1489159
The mini review assesses the value propositions of robo-advisors through the lens of behavioral finance. Despite their promise of data-driven, rational investment strategies, robo-advisors may not fully replicate the personalized service of human financial advisors or eliminate human biases in decision-making. A content analysis of 80 peer-reviewed articles and publications was conducted, focusing on the intersection of financial technology and behavioral finance. Literature was retrieved using The Chicago School University Library's OneSearch and the EBSCO host database, with key terms including “robo-advisor,” “investment behavior,” “risk tolerance,” “financial literacy,” and “affective trust.” The review identifies four key limitations of robo-advisors: (1) their inability to replicate the service-relationship of human advisors; (2) the presence of human bias in supposedly rational algorithms; (3) the inability to minimize market risk; and (4) their limited impact on improving users' financial literacy. Instead, robo-advisors temporarily compensate for a lack of financial knowledge through passive investment strategies. The findings suggest that integrating behavioral finance principles could enhance the predictive power of robo-advisors, though this would introduce additional complexities. The review calls for further research and regulatory measures to ensure that these technologies prioritize investor protection and financial literacy as they continue to evolve.
The financial sector is undergoing a large-scale transformation that is strongly characterized by the emergence of novel financial technology (FinTech) and its applications. With the rising accessibility of digital financial products, society's interest in participating in the capital market has continued to increase over the past years because of higher exposure and awareness of these new products. This is reflected by the increase of active stock holdings in U.S. households to by 2019 (Bleck, 2021). However, asset management is not done by private individuals alone, but instead accompanied by the support of professional financial advisory. In the digital age, this service is increasingly covered by online applications and so-called robo-advisors in particular who present a new form of competition and strategic advantage alike to traditional financial advisors. Robo-advisors provide data-driven, customized investment advice though automated online platforms in a low-cost manner (Beketov et al., 2018; Belanche et al., 2019; Garg et al., 2023; Hildebrand and Bergner, 2021; Sironi, 2016; Pietersen et al., 2021; Wexler and Oberlander, 2021). They do so by leveraging accessible market data, user insights, and customer preferences to derive tailored investment predictions and recommendations based on an advanced artificial intelligence model.
The compound annual growth rate (CAGR) of assets under robo-advisor management between 2023 and 2027 is predicted to be at 13.46% globally—tendency rising (Statista Market Insights, 2023). Today, Vanguard, Schwab Intelligent Portfolios, Betterment, or SoFi belong to the most renowned robo-advisor providers. On the one hand, it is important to understand the value proposition of robo-advisors as well as how their providers claim to realize this strategic advantage over traditional human financial advice. Therefore, it is pivotal to evaluate the interaction between customers and robo-advisors to understand the technology's functionality and means of application in a critical manner. Providers of robo-advisors claim to realize investment decisions “on the go,” whilst reducing investors' potential risks of biased decisions by providing individuals with experienced, data-driven advice. Yet, they cannot influence natural human attitudes, personal preferences, or the emotional states during an investment decision, e.g., responses to market dynamics or news. Put differently, robo-advisors try to “de-humanize” the investment process to minimize risks whilst maximizing financial returns. However, it is questionable to what extent robo-advisors can do so and whether it is beneficial.
In the literature, the rise of behavioral economics is characterized by the opposite trend: integrating, or rather “humanizing,” neoclassical economic models by replacing the rational homo economicus with the idea of a human being. According to this notion, behavior is not the consequence of perfect evaluation or utility maximization, but the product of affective states, cognition, information processing, and bounded rationality (Simon, 1955; Thaler, 2016). Herein, behavioral finance is a subset of behavioral economics that views financial phenomena from a psychological perspective and integrates behavioral dimensions into the economic principles of modern portfolio theory and asset pricing theorems (Moreira Costa et al., 2021; Sent, 2004; Shefrin, 2009; Maier-Paape and Zhu, 2018). Behavioral economics and behavioral finance are evolving fields that propose novel approaches to economics, whilst at the same time complementing and enhancing the predictive power of economic models through the integration of human dimensions to offer explanations for real-life scenarios.
The aim of this mini-review is to evaluate and contrast the value propositions of robo-advisors in the context of behavioral finance literature. Specifically, it focuses on the contrast between the data-driven, rationalized nature of robo-advisor technology and the insights derived from behavioral finance. The mini review proposes four key claims to structure its analysis. First, robo-advisors, being algorithmic systems, cannot replicate the nuanced service-relationship offered by human financial advisors. Second, despite their promise of objective and rational decision-making, robo-advisors cannot fully eliminate the human element, as the data they rely on inevitably contains subjective components. Third, robo-advisors are unable to mitigate market risks, such as the volatility of individual assets, due to the multitude of external factors that influence overall market conditions. Lastly, contrary to expectations, robo-advisors may not significantly enhance users' financial literacy. Instead, they tend to compensate for a lack of financial expertise by offering passive investment strategies that do not actively engage users in the learning process. This mini-review critically examines both the advantages and limitations of robo-advisors within this framework and concludes with a discussion of their broader implications for the financial industry. It is important to note that this mini review will not deep dive into advanced technological implications of the technology as it relates to machine learning, coding, or data analytics, nor will the review focus on ethical considerations that are of importance in this emerging field.
The researcher chose to write a mini review to provide a concise and condensed summary of the current state of research within this novel field of interest whilst also pointing out future directions of research and important developments (Bioelectronic Medicine, 2024). In the context of robo-advisor technology, a mini review allows to distill the essential findings or debates on the rising technology and its value proposition as well as current shortcomings, offering a clear, focused synthesis of the latest research in the field. By integrating contrasting perspectives, the mini review does not only summarize the current literature and points out existing gaps but integrates different perspectives, such as the interplay between robo-advisors' algorithmic logic and human behavioral finance. It is acknowledged that the review is not exhaustive of the entire body of literature existing in the field. Literature was retrieved using The Chicago School University Library's One Search and the EBSCO host database. Peer-reviewed articles and book entries between 1955 until 2024 were integrated into the analysis. Key words were chosen based on concepts that were assumed to influence service relationships and investment decisions. These included but were not limited to: “robo-advisor,” “investment behavior,” “risk tolerance,” “financial literacy,” and “affective trust.” Ethical considerations, regulatory issues, or advanced technological evaluations are out of the paper's scope.
Before evaluating the four outlined claims, it is important to understand what robo-advisors are, how they operate on a high-level, and which alleged human shortcomings the technology seeks to compensate for. The current state of the literature differentiates between static robo-advisors and conversational, also called generative, robo-advisors. For example, robo-advisors can be found on online banking platforms or digital investment hubs. Hereby, static robo-advisors will use questionnaire-driven risk assessments to define a customer's overarching risk profile, investment goals, or preferred asset classes as well as the amount and timeline of the envisioned investment. Based on the provided user data and the available market data, the robo-advisor will set up an investment strategy and corresponding portfolio for the customer to initiate the passive investment process (Alsabah et al., 2019; Beketov et al., 2018; Semko, 2019). Today's advanced technology allows for higher level of automation through artificial intelligence and algorithmic methods for portfolio optimization, which allows the robo-advisor to fully monitor and conduct the passive investment strategy on its own.
The newest generation of robo-advisors uses chatbot functionalities in addition to risk assessments to interact with new investors. These conversational robo-advisors integrate social cues, such as colloquial language or the use of emojis, to mimic a human-like two-way conversation (Hildebrand and Bergner, 2021; Thomaz et al., 2020). The advanced technology seeks to customize financial advice by creating a sense of relationship between the customer and financial institution. For example, providers hope that the use of emojis or user-adapted languages creates feelings of affective trust toward the robo-advisor, which is the outcome of turn-taking in an anthropologic chat design (Hildebrand and Bergner, 2021; Thomaz et al., 2020).
The technology behind robo-advisors shall not be the focus of this mini review, yet the core means of functionality shall be explained to understand how this FinTech application operates. According to a review of 219 robo-advisor systems, the following workflow pattern can be observed across robo-advisor applications: Following the user profiling and the review of available market data and financial products of the institution, suitable assets are selected and then allocated through Modern Portfolio Theory. To do so, the robo-advisor will use its foundational data model or any existing sample portfolio. The more individualization the better, the more complex the algorithm needs to be to ensure customer expectations are met and to allow for a continuous rebalancing of the created investment portfolio (Beketov et al., 2018; Semko, 2019). To enhance this process further, the use of artificial neural networks, a sub-domain of machine learning, may become the new state of the art approach to robo-advisor applications. Machine learning allows us to train the robo-advisor algorithm, leading to continuous improvement of the investment recommendations.
The continued evolution of this technology holds the promise that robo-advisors will potentially be able to perform the advising functionality that they have been designed for in the first place. It is expected that the application will improve gradually as more user feedback becomes available. The more data input the algorithm receives through the financial market environment as well as the clients' behavior, the more dynamic and sophisticated the data model becomes (Guresen and Kayakutlu, 2011; Semko, 2019). Hereby, neural networks use non-linear learning algorithms which simulate the activity of biological neurons to collect information and interconnects it continuously, posing the opportunity to detect and mitigate irrational behavioral patterns, such as biases, in human investment behavior that cannot be fully rebalanced through traditional Modern Portfolio Theory (Beketov et al., 2018; Guresen and Kayakutlu, 2011; Semko, 2019). Following this brief introduction to robo-advisors' functionality, the mini review will now evaluate the four proposed research claims by building on the current state of research.
Trust is an integral part of the formation of relationships. In the financial services industry, investing is considered a segment in which customers require knowledge that usually exceeds their personal know-how, making them dependent on skilled financial advisors to assist them in making informed financial choices. Investment banking is a personal field, requiring both high customer involvement and a strong foundation of trust. The term robo-advisor implies that a robot will provide a form of (financial) advice to the customer. How individuals respond to financial information does depend on multiple factors, such as how the information is being processed and portrayed, the perceived credibility of the message sender, as well as perceived level of affective trust toward the advisor. Advice, especially in the service industry, is delivered through speech and means of communication. It is a form of providing help that is either accepted or neglected (Torrey et al., 2013) and hence seems to go hand in hand with the concept of persuasion.
The current state of research on information processing does not adhere to one unifying theory but often differentiates between two distinct modes, reflected in dual-process theories. These modes, or systems, are characterized by different cognitive features. System 1 thinking is fast, intuitive, and based on past experiences, leading to automatic responses, while System 2 thinking requires more cognitive effort, engaging conscious, analytical reasoning (Evans and Stanovich, 2013; Frankish, 2010; Kahneman, 2011, 2003; Tversky and Kahneman, 1974). Although neither system is inherently superior, both can be susceptible to judgment errors. In financial decision-making, System 1 thinking may employ heuristics, using mental shortcuts to simplify the complex processing of financial information (De Bondt and Thaler, 1994; Petty and Cacioppo, 1979). Rather than providing a broad overview of dual-processing theories, the focus here is on how robo-advisors specifically engage these cognitive systems. Robo-advisors are designed to reduce reliance on the investor's System 1 thinking by automating many of the intuitive, heuristic-based decisions that investors might otherwise make. This minimizes the risk of hasty judgments and biases typically associated with System 1 processing. Instead, by streamlining the decision-making process, robo-advisors aim to free up cognitive resources, enabling investors to engage more fully in System 2 thinking. This deeper, more deliberate evaluation allows investors to better assess the risks and benefits of the proposed investment portfolios (Darškuviene and Lisauskiene, 2021; Jung et al., 2018). Consequently, the robo-advisor is designed to act as a cognitive aid, enhancing the investor's ability to make more informed, rational decisions without the cognitive overload often associated with manual financial analysis. For example, this is done by realizing passive investment strategies on behalf of the customer which merely requires hir or her agreement to initiate the investment process.
Yet, the interaction between a robo-advisor and a customer poses a new form of service relationship that is less characterized by social interaction and exchange, also referred to as turn-taking. A lack of human-to-human interaction yields the question of whether robo-advisors can form relationships. Also, it poses the question whether they can ultimately be considered a credible and trustful advisor. Information is perceived as attractive, valid, and lastly trustworthy if the sender is confident in the message's content and the expertise of the sender (Wu and Wang, 2011). However, credibility as an antecedent of trust is evaluated differently in robo-advisors as opposed to human financial advisors. The latter are measured against their perceived level of performance, the trust in the advisor and the financial institution, as well as the ability to provide accurate investment advice. In contrast, the credibility of robo-advisors is also determined by the level of trust toward the technology itself (Johnson and Grayson, 2005; Zhang et al., 2021). Yet, for the customer segment between the age of 18–35, the technology's data-driven nature has been found to favor credibility of robo-advisors. Older customer segments prefer the apparent interpersonal cues of the human financial advisor (Chua et al., 2023; Wu and Wang, 2011; Zhang et al., 2021).
It follows that different customer segments seem to put different emphasis on two dimensions of trust: cognitive trust and affective trust. Cognitive trust relates to the perceived level of competence and an individual's confidence therein (Hildebrand and Bergner, 2021; Johnson and Grayson, 2005), whilst affective trust describes a subjective, emotional dimension of trust between two or more parties that depends on the perceived level of social connection or the quality of relationship. However, affective trust remains the most integral part of a service relationship and determines a customer's willingness to put trust into the (financial) service provider (Hildebrand and Bergner, 2021). Ultimately, a customer's willingness to invest with the support of a robo-advisor again will depend on the achieved return on investment. Whether an investment is considered profitable or not depends on different factors: First, the investor will consider the total monetary gain that has been achieved. Second, the investor will evaluate to what extent the investment has contributed to achieving the personal investment goals. Because the process of following and acting on advice is completely reflected by the robo-advisor's passive investment strategy toward the customer's predefined investment goals, robo-advisors are rather an intelligent algorithm than an advisor. Financial advising is a profession in which trained financial advisors support individuals to reach their financial goals. For a fee, customers benefit from the advisor's expertise and allow them to manage their finances, e.g., by defining investment strategies or designing suitable portfolios (Aiken-Wisniewski et al., 2015; Napoletano, 2020). In contrast, an algorithm can be defined as logical steps or a computer program's execution path to reach a clearly defined goal. The performance of an algorithm is determined by its effectiveness and its reliability of results (Carstensen, 2024). However, this evaluation will depend on predefined performance indicators and the ability to measure reliability. In contrast, the prior findings underline that a successful service-relationship is determined by its ability to act on the expectations of the customer, and most importantly by the feeling of trust and social exchange which can only be achieved through continuous relationship-building in a human-to-human context. Therefore, the authors believes that robo-advisors should be considered algorithms, not true advisors.
Robo-advisors claim to be rationally acting agents that uphold complete rationality by leveraging data instead of human behaviorism or intuition. Yet, in real life, robo-advisors cannot uphold unbounded rationality due to inherent limitations in both data and algorithms. Whilst they process vast amounts of financial information rapidly, this does not guarantee that all investment options are fully considered or that future outcomes can be accurately predicted (Kalantari, 2010; Schwarz et al., 2022; Simon, 1955, 1991). In contrast, human investors face cognitive limitations that prevent them from being aware of all market possibilities or portfolio combinations. More specifically, investors often rely on mental shortcuts (heuristics) or their prior experience to simplify complex financial decisions, which can introduce biases (Costa et al., 2019; Petty and Cacioppo, 1990; Shefrin, 2009; Kahneman and Tversky, 1979; Kahneman, 2003; Tversky and Kahneman, 1974).
Robo-advisors themselves are limited by the quality of the data they process and the algorithms they use. Although they reduce reliance on human intuition, their predictive power is determined by the algorithms designed by humans, who are inherently influenced by their own cognitive biases. Every robo-advisor provider uses a unique algorithm created by individuals, which means the technology cannot be completely free from human affect, cognition, or opinion (Luo et al., 2024). Furthermore, robo-advisors base their predictions on historical market data—such as stock prices, company valuations, and macroeconomic trends—data that reflects both systematic market factors and individual behaviors. Therefore, despite the sophisticated processing power of robo-advisors, any recommendations they make may still carry biases from the data itself. Investment decisions are inherently decisions under uncertainty, and no form of financial advisory can guarantee perfect outcomes. Robo-advisors use algorithms grounded in Modern Portfolio Theory (MPT) to solve optimization problems, but these algorithms may not always align with the investor's goals. The “correctness” of financial decisions made by robo-advisors depends on the goals defined by their underlying algorithms, which may not always prioritize the profit-maximizing strategies expected by the investor (Beketov et al., 2018; Semko, 2019; Carstensen, 2024). As a result, while robo-advisors may process financial data accurately according to their models, they do not necessarily make optimal choices for the investor.
Lastly, the author argues that bounded rationality and human dimensions should be integrated into robo-advisor models. Simon (1955, 1991) introduced the concept of bounded rationality to address the limitations of neoclassical theory and abstract economic models. He emphasized that human behavior, while often considered “irrational,” provides valuable insights into real-life economic decisions. Rather than striving for unattainable perfect rationality, robo-advisors should aim to incorporate human factors—such as perception and affect—into their data models, which would better reflect the complexities of real-world investment scenarios. Bounded rationality can therefore be seen as a strength, helping to create models that more accurately mirror how investors behave.
In addition to unbiased rationality, robo-advisors also claim to reduce investment risks by providing passive investment strategies at low cost. Whilst other researchers have highlighted the shortcomings of robo-advisors to accurately understand and reflect investors' risk preferences in the passive investment strategies (Severino and Thierry, 2022), the author also argues that robo-advisors may not lower the overarching stock- or market risks. Instead, robo-advisors are designed to minimize the risk within a diversified portfolio by continuous rebalancing whilst seeking to meet the investor's financial goals (Beketov et al., 2018; Semko, 2019). In theory, robo-advisors aim to construct portfolios that balance high potential returns with the lowest possible volatility according to the investor's risk profile. However, investment decisions remain fundamentally decisions under uncertainty. Even with the advanced data-processing capabilities of algorithms, no model can fully account for all sources of uncertainty or eliminate the risk inherent in investment decisions. While robo-advisors may be more effective than human investors at balancing risk based on historical data, their ability to manage future risks remains limited by the unpredictable nature of markets and the inherent uncertainties of investing. Thus, while robo-advisors can enhance the accuracy and efficiency of risk management, they cannot overcome the fundamental unpredictability and complexity of financial markets (Elbannan, 2014; Sharpe, 1964).
Considering the above findings, it becomes apparent that an investor's stated low risk preference may lead to different rebalancing strategies depending on how the algorithm of the robo-advisor is designed and that a low-risk portfolio may also contain high-risk assets which are merely compensated by diversification. Further, the paragraph above has highlighted, that financial markets are also prone to external influences and human dynamics which lead to irregularities or sudden market fluctuations, which in turn trigger structural changes on the financial market (Luo et al., 2024). Volatility of a stock price is a potential risk, because it reflects the degree of price fluctuation. Some stocks are more volatile than others, yet volatility is a normal market phenomenon (Measuring and Managing Market Risk, 2024; Rosenberg, 2022). Often, the degree of volatility, expressed by the beta-value, is measured against a stock index to allow investors to drive meaningful comparisons.
In addition, the alpha value of a stock reflects its performance relative to a market index and indicates whether the stock is outperforming or underperforming. However, these values do not operate in isolation; they interact with other risk factors, creating a complex risk profile. For a robo-advisor to minimize the total risk of an investment, its algorithm would need to reduce both the individual risks of each asset and the risks arising from the interaction between these factors. This presents a significant limitation. According to the Capital Asset Pricing Model (CAPM), investment risk can be divided into two broad categories: individual stock risks and market risks (Elbannan, 2014; Sharpe, 1964). While robo-advisors can address stock-specific risks by selecting assets with low volatility, they cannot influence or mitigate the volatility itself. Stock-specific risks, such as those related to company performance, industry dynamics, or other idiosyncratic factors, remain present, and a robo-advisor's algorithm can only manage these risks by diversifying the portfolio and choosing assets based on the investor's risk tolerance. However, this management is reactive rather than preventive, meaning the algorithm cannot prevent stock-specific volatility from affecting the portfolio.
Furthermore, systematic market risk, which stems from macroeconomic factors, geopolitical events, and broad market fluctuations, poses a particular challenge to robo-advisors. Systematic risks are beyond the control of any advisor, human or robo, as they are inherent to the market itself. Although robo-advisors can balance portfolios in a way that aligns with an investor's risk preferences, they cannot mitigate these market-wide risks. The predictive power of robo-advisors, while valuable for navigating stock-specific risks, falls short in reducing exposure to the unpredictable nature of the overall market. In addition, interactive risk factors, or the way different risks interact within a portfolio, present another limitation for robo-advisors. Although they can optimize portfolios to match risk-return profiles, robo-advisors cannot fully account for the complex interactions between assets in real-time. These interactions, where the performance of one asset affects others, are difficult to predict and often behave in non-linear ways, especially during periods of market stress. As such, while a robo-advisor's algorithm can recommend portfolio adjustments based on historical correlations, it cannot completely forecast or manage the dynamic relationships between assets.
Lastly, robo-advisors often hold the promise of not requiring any financial knowledge to succeed on the investment market. Whilst robo-advisor applications simplify the access to investment opportunities and digitalize the process of investing in an increasingly user-friendly manner, they do not take over responsibility for the investment decision itself. Among other aspects, the investor first needs to determine her or his risk preference, investment goals, investment timeline, as well as the desired investment amount and intervals of investment to set up the passive investment portfolio. Whilst the robo-advisor algorithm will optimize and rebalance the portfolio according to the investor's user-profile, these initial investment criteria can be pivotal for investment success and do require an understanding of financial concepts. Objective financial literacy refers to an individual's ability to understand and apply financial concepts, which is often considered a key factor for stock market participation (Ansari et al., 2022; Lusardi and Mitchell, 2011; Raut, 2020; OECD, 2023). In contrast, subjective financial know-how, or overconfidence, refers to the beliefs an individual holds on her or his financial competence regardless of the actual level of know-how (Ahmad and Shah, 2022; Lewis, 2018; Mittal, 2022; Raut, 2020; Mittal et al., 2022; Kruger and Dunning, 1999).
The distinction between these two types of literacy plays an important role in determining who is more likely to use robo-advisors and how effectively they can manage investments. For instance, a study by Isaia and Oggero (2022) found that individuals with higher objective financial literacy, those who have a solid grasp of financial concepts, are more likely to use robo-advisors than those with basic financial knowledge. This suggests that those who possess strong financial knowledge may recognize the benefits of using technology to assist in investment decisions. However, the study's focus on a specific demographic from Italy (n = 1,263) born between 1981 and 2010 limits the generalizability of its findings. In contrast, a study using data from the 2015 U.S. Financial Industry Regulatory Authority (FINRA) National Financial Capability Study (NFCS) offers a different perspective. This research found that individuals with high subjective financial literacy, meaning those who perceive themselves as financially knowledgeable, are more likely to use robo-advisors, even if their objective financial knowledge is limited. Interestingly, the study revealed a negative relationship between objective financial literacy and robo-advisor usage, suggesting that those with lower actual financial literacy may feel less confident in their abilities to manage investments independently and therefore turn to robo-advisors for assistance (Kim et al., 2019).
Either way, using robo-advisors makes individuals engage more with the investment market, yet does not contribute to their objective financial literacy because the technology takes over the investment process for them, nor does the robo-advisor provide customized knowledge resources during this process. Nevertheless, robo-advisors pose an alternative opportunity for rather inexperienced investors to participate in the stock market at a low-cost entry point. This allows them to make first experiences and maybe develop an interest in the field to further expand their skillset, i.e., their objective financial literacy (Tan, 2020). However, the author assumes that individuals with high subjective financial literacy and low objective financial literacy are at risk to overestimate their skills, if they experience their first moments of financial success through the help of robo-advisors. The importance of affective states has been highlighted repetitively in the behavioral finance literature. Accordingly, positive affect because of a financial gain has been associated with a reduction of risk aversion and increased risk-seeking behaviors in hope of further future gains (Kahneman and Tversky, 1984; Seo et al., 2010; Kahneman, 2003; Tversky and Kahneman, 1974). Therefore, robo-advisors might keep inexperienced investors from actively increasing their objective financial literacy because they already consider themselves to be highly skilled which in turn exposes them to higher investment risks and overconfidence.
The mini review has shown the potential and increasing influence of robo advisors. The technology shall serve as investors' digital supporters to pursue automated, individualized, passive investment strategies which create, manage and rebalance portfolios to maximize returns and protect their investments (Alsabah et al., 2019; Beketov et al., 2018; Semko, 2019). The author has critically reviewed the current state of research, particularly the blurred boundaries between robo-advisors and human advisors, where social cues and affective trust play a pivotal role in the service relationship. This is particularly relevant given the increasing use of human-like designs in generative robo-advisor chatbots (Hildebrand and Bergner, 2021; Thomaz et al., 2020).
A key limitation of robo-advisors lies in their reliance on data influenced by human behavior, which undermines their ability to act as fully rational agents. As algorithms are designed and built using data created by humans, they inevitably inherit human biases and cannot achieve pure rationality. This limitation is particularly apparent in the context of financial risk management. While robo-advisors may select low-volatility stocks according to an investor's risk profile, they cannot eliminate systematic market risks or the inherent volatility of financial markets (Elbannan, 2014; Rosenberg, 2022; Sharpe, 1964). These risks are driven by complex, interactive market effects and human behavior, which cannot be fully predicted by algorithms alone. Further, while robo-advisors are often positioned as tools that democratize investing, their role in addressing financial literacy remains underdeveloped. Rather than educating users or improving financial literacy, robo-advisors compensate for a lack of investor knowledge by relying on passive investment strategies. This can have unintended consequences, such as fostering overconfidence in investors who experience initial financial gains, potentially encouraging risk-seeking behavior (Kahneman and Tversky, 1979, 1984; Seo et al., 2010; Kahneman, 2003; Tversky and Kahneman, 1974). Such behaviors, especially among inexperienced investors, may pose a risk to long-term financial wellbeing.
The review ultimately raises a broader question: should the goal of robo-advisors be to “de-humanize” investment decisions in the pursuit of more objective, data-driven market predictions? Or, as behavioral finance suggests, would integrating human factors, such as emotions, perceptions, and social behaviors, lead to more accurate and sustainable economic models? The author encourages further reflection on whether incorporating human elements could enhance the predictability of financial outcomes, rather than relying solely on the rationality of algorithms. This integration may offer a more realistic approach to improving investment returns and mitigating risks in increasingly complex financial markets.
Ultimately, the mini review acknowledges the potential the robo-advisors hold and underlines their emerging nature of the technology which calls for continuous improvement. At the same time, the mini review outlines central misconceptions and current weaknesses of the robo-advisor technology to motivate other researchers and practitioners to adopt a holistic view of the problem and work toward new solutions.
The mini review allowed for a concise synthesis of the current state of literature as it relates to behavioral finance and the rise of robo-advisors until the year 2024. Future developments, ethical and regulatory concerns, or advanced technological evaluations were not considered in this review. Whilst the mini review draws on 80 peer-reviewed articles, publications, and validated data reports, the article does not claim a fully exhaustive review of the existing body of literature. In addition, the author acknowledges that both behavioral finance and the application of robo-advisors are still a novel field which limits the extent of available research today and underlines the potential of future research yet to come. Lastly, the mini review merely offers a meaningful synthesis of what we know about the intersection of these two fields today and makes assumptions about implications for individual investment behavior. The nature of the mini review does not include the development and testing of hypotheses to produce new knowledge through an experimental research design.
Financial technology, and artificial intelligence in particular, are continuing to advance and develop at a high pace. Therefore, it will be of utmost importance to monitor these developments from a technological, anthropologic, ethical, and psychological point of view. One can assume that advanced robo-advisor applications that do not only evoke the feeling of cognitive trust but also create a social connection by mimicking humanoid behaviors will establish affective trust and ultimately succeed on the market. However, to be of interest to a larger customer base, financial services organizations need to provide greater transparency on the technology behind this FinTech application. For example, by using simple language and user-friendly explanations, human financial advisors may be able to convince investors of their digitalized counterpart by explaining both the advantages and risks that are associated with the technology.
In addition, financial organizations may learn from customer feedback and improve their robo-advisor applications by integrating further functionalities that not only make online investments more easily, but also more human-like to establish a stable feeling of trust and elevate the service relationship between the institution and the investor. In addition, the mini review has highlighted the importance of integrating human dimensions into existing data models and algorithms to elevate their predictive power. Surely, this implies an even higher degree of complexity yet might untap hidden potential of the application. Hereby, the capability of robo-advisors should not only be evaluated through the eyes of financial services organizations, but also from an investors' standpoint. Individuals need to understand how their personal attitudes and behaviors toward both digitalized finance services and investing in general impact their investment choices, and which benefits and risks are associated with that. For example, this includes self-awareness of one's objective financial literacy as opposed to one perceived financial literacy to determine whether an investment should be done merely by the help of a robo-advisor, or whether additional professional advice is of value.
The evolving technological landscape also gives rise to ethical questions and the need for transparent regulations that protect consumers and proactively promote financial wellbeing. Future research is well-advised to focus its efforts on understanding how individuals perceive and react to different forms of financial advice and how their behaviors impact their perceived level of risk and affective trust considering their financial literacy. In addition, future research should focus on supporting policy makers to design the laws and regulations required to foster a safe promotion, application, and advancement of digital financial services.
This mini review has critically examined the evolving role of robo-advisors by evaluating four statements that relate to both interactive market effects and human effects as underlined by the behavioral finance literature. While the advantages of robo-advisors are undeniable, so are its shortcomings and persisting limitations. Hence, the findings of the mini review suggest that the integration of behavioral finance principles could enhance the efficacy and predictive power of robo-advisors, though this would likely introduce additional complexities. Furthermore, the review stresses the importance of transparency and ethical considerations in the development and regulation of these technologies, that should be explored by future research to work toward a balanced approach that prioritizes investor protection and financial literacy. As the landscape of financial technology continues to advance, it is imperative that future research and policy development adopt a holistic perspective, addressing both the opportunities and challenges presented by robo-advisors. Ultimately, the intersection of technological innovation and behavioral finance will continue to play a pivotal role in shaping the future of digital financial services, with significant implications for portfolio management on the one hand and investors' financial wellbeing on the other hand.
KE: Conceptualization, Investigation, Methodology, Project administration, Writing – original draft, Writing – review & editing. ES: Methodology, Supervision, Validation, Writing – review & editing.
The author(s) declare that no financial support was received for the research, authorship, and/or publication of this article.
I would like to express my sincere gratitude to Elizabeth Schwab who supported this process through her ideas, guidance, and words of wisdom.
The authors declare that the research was conducted in the absence of any commercial or financial relationships that could be construed as a potential conflict of interest.
All claims expressed in this article are solely those of the authors and do not necessarily represent those of their affiliated organizations, or those of the publisher, the editors and the reviewers. Any product that may be evaluated in this article, or claim that may be made by its manufacturer, is not guaranteed or endorsed by the publisher.
Ahmad, M., and Shah, S. Z. A. (2022). Overconfidence heuristic-driven bias in investment decision-making and performance: mediating effects of risk perception and moderating effects of financial literacy. J. Econ. Admin. Sci. 38, 60–90. doi: 10.1108/JEAS-07-2020-0116
Aiken-Wisniewski, S. A., Johnson, A., Larson, J., and Barkemeyer, J. (2015). A preliminary report of advisor perceptions of advising and of a profession. NACADA J. 35, 60–70. doi: 10.12930/NACADA-14-020
Alsabah, H., Capponi, A., Octavio Ruiz, L., and Stern, M. (2019). Robo-advising: learning investors' risk preferences via portfolio choices. ArXiv.Org. doi: 10.1093/jjfinec/nbz040
Ansari, Y., Albarrak, M. S., Sherfudeen, N., and Aman, A. (2022). A study of financial literacy of investors-a bibliometric analysis. Int. J. Fin. Stud. 10:36. doi: 10.3390/ijfs10020036
Beketov, M., Lehmann, K., and Wittke, M. (2018). Robo advisors: quantitative methods inside the robots. J. Asset Manag. 19, 363–370. doi: 10.1057/s41260-018-0092-9
Belanche, D., Casaló, L. V., and Flavián, C. (2019). Artificial Intelligence in FinTech: understanding robo-advisors adoption among customers. Industr. Manag. Data Syst. 119, 1411–1430. doi: 10.1108/IMDS-08-2018-0368
Bioelectronic Medicine (2024). BioMed Central. Available at: https://bioelecmed.biomedcentral.com/submission-guidelines/preparing-your-manuscript/mini-review#:~:text=Mini%2Dreviews%20provide%20a%20concise (accessed July 26, 2024).
Bleck, A. (2021). Aktionärsquote USA: 4 Investment-Regeln für mehr Rendite. NorthernFinance. Available at: https://northern.finance/investieren-lernen/investment-regeln/ (accessed December 29, 2023).
Carstensen, J. (2024). Advanced Mathematics—Computational Mathematics (CompMath) [Review of Advanced Mathematics—Computational Mathematics (CompMath)]. Universität Kiel. Available at: https://www.tf.uni-kiel.de/matwis/amat/comp_math/kap_1/backbone/r_se5.html (accessed August 9, 2024).
Chua, A. Y. K., Pal, A., and Banerjee, S. (2023). AI-enabled investment advice: will users buy it? Comput. Hum. Behav. 138:107481. doi: 10.1016/j.chb.2022.107481
Costa, D. F., Carvalho, F. de M., and Moreira, B. C. de M. (2019). Behavioral economics and behavioral finance: a bibliometric analysis of the scientific fields. J. Econ. Surv. 33, 3–24. doi: 10.1111/joes.12262
Darškuviene, V., and Lisauskiene, N. (2021). Linking the robo-advisors phenomenon and behavioral biases in investment management: an interdisciplinary literature review and research agenda. Org. Mark. Emerg. Econ. 12, 459–477. doi: 10.15388/omee.2021.12.65
De Bondt, W. F. M., and Thaler, R. H. (1994). Financial decision-making in markets and firms: a behavioral perspective. NBER Work. Pap. Ser. 1994:4777. doi: 10.3386/w4777
Elbannan, M. A. (2014). The capital asset pricing model: an overview of the theory. Int. J. Econ. Fin. 7:216. doi: 10.5539/ijef.v7n1p216
Evans, J., and Stanovich, K. E. (2013). Dual-process theories of higher cognition: advancing the debate. Perspect. Psychol. Sci. 8, 223–241. doi: 10.1177/1745691612460685
Frankish, K. (2010). Dual-process and dual-system theories of reasoning. Philos. Compass 5, 914–926. doi: 10.1111/j.1747-9991.2010.00330.x
Garg, G., Shamshad, M., Gauhar, N., Tabash, M. I., Hamouri, B., and Daniel, L. N. (2023). A bibliometric analysis of fintech trends: an empirical investigation. Int. J. Fin. Stud. 11:79. doi: 10.3390/ijfs11020079
Guresen, E., and Kayakutlu, G. (2011). Definition of artificial neural networks with comparison to other networks. Proc. Comput. Sci. 3, 426–433. doi: 10.1016/j.procs.2010.12.071
Hildebrand, C., and Bergner, A. (2021). Conversational robo advisors as surrogates of trust: onboarding experience, firm perception, and consumer financial decision making. J. Acad. Market. Sci. 49, 659–676. doi: 10.1007/s11747-020-00753-z
Isaia, E., and Oggero, N. (2022). The potential use of robo-advisors among the young generation: evidence from Italy. Fin. Res. Lett. 48:103046. doi: 10.1016/j.frl.2022.103046
Johnson, D., and Grayson, K. (2005). Cognitive and affective trust in service relationships. J. Bus. Res. 58, 500–507. doi: 10.1016/S0148-2963(03)00140-1
Jung, D., Dorner, V., Weinhardt, C., and Pusmaz, H. (2018). Designing a robo-advisor for risk-averse, low-budget consumers. Electr. Mark. 28, 367–380. doi: 10.1007/s12525-017-0279-9
Kahneman, D. (2003). A perspective on judgment and choice: mapping bounded rationality. Am. Psychol. 58, 697–720. doi: 10.1037/0003-066X.58.9.697
Kahneman, D., and Tversky, A. (1979). Prospect theory: An analysis of decision under risk. Econometrica 47, 263–291. doi: 10.2307/1914185
Kahneman, D., and Tversky, A. (1984). Choices, values, and frames. Am. Psychol. 39, 341–350. doi: 10.1037/0003-066X.39.4.341
Kalantari, B. (2010). Herbert A. Simon on making decisions: enduring insights and bounded rationality. J. Manag. Hist. 16, 509–520. doi: 10.1108/17511341011073988
Kim, S. D., Cotwright, M., and Chatterjee, S. (2019). Who are robo-advisor users? J. Fin. Iss. 18, 33–50. doi: 10.58886/jfi.v18i2.2225
Kruger, J., and Dunning, D. (1999). Unskilled and unaware of it: how difficulties in recognizing one's own incompetence lead to inflated self-assessments. J. Personal. Soc. Psychol. 77, 1121–1134. doi: 10.1037/0022-3514.77.6.1121
Lewis, D. R. (2018). The perils of overconfidence: why many consumers fail to seek advice when they really should. J. Fin. Serv. Market. 23, 104–111. doi: 10.1057/s41264-018-0048-7
Luo, Y., Li, X., Yu, W., Huang, K., Yang, Y., and Huang, Y. (2024). Research on human dynamics characteristics under large-scale stock data perturbation. North Am. J. Econ. Fin. 70, 102070–102070. doi: 10.1016/j.najef.2023.102070
Lusardi, A., and Mitchell, O. S. (2011). Financial literacy around the world: an overview. J. Pension Econ. Fin. 10, 497–508. doi: 10.1017/S1474747211000448
Maier-Paape, S., and Zhu, Q. J. (2018). A general framework for portfolio theory. Part I: theory and various models. Risks 6, 1–35. doi: 10.3390/risks6020053
Measuring and Managing Market Risk (2024). CFA Institute. Available at: https://www.cfainstitute.org/en/membership/professional-development/refresher-readings/measuring-managing-market-risk#:~:text=Market%20risk%20is%20the%20risk (accessed August 28, 2024).
Mittal, S., Bhattacharya, S., and Mandal, S. (2022). Characteristics analysis of behavioral portfolio theory in the Markowitz portfolio theory framework. Manag. Fin. 48, 277–288. doi: 10.1108/MF-05-2021-0208
Mittal, S. K. (2022). Behavior biases and investment decision: theoretical and research framework. Qualit. Res. Fin. Mark. 14, 213–228. doi: 10.1108/QRFM-09-2017-0085
Moreira Costa, V., De Sá Teixeira, N. A., Cordeiro Santos, A., and Santos, E. (2021). When more is less in financial decision-making: financial literacy magnifies framing effects. Psychol. Res. 85, 2036–2046. doi: 10.1007/s00426-020-01372-7
Napoletano, E. (2020). What Is A Financial Advisor? How Do They Work? Forbes Advisor. Available at: https://www.forbes.com/advisor/investing/financial-advisor/what-is-a-financial-advisor/
OECD (2023). OECD/INFE 2023 International Survey of Adult Financial Literacy, OECD Business and Finance Policy Papers, No. 39. Paris: OECD Publishing.
Petty, R. E., and Cacioppo, J. T. (1979). Issue involvement can increase or decrease persuasion by enhancing message-relevant cognitive responses. J. Personal. Soc. Psychol. 37, 1915–1926. doi: 10.1037/0022-3514.37.10.1915
Petty, R. E., and Cacioppo, J. T. (1990). Involvement and persuasion: tradition versus integration. Psychol. Bullet. 107, 367–374. doi: 10.1037/0033-2909.107.3.367
Pietersen, J., Ferreira-Schenk, S., and Dickason, Z. (2021). Are robots taking over? technological advancements and investor risk tolerance. Acta Universitatis Danubius. Economica 17, 7–27.
Raut, R. K. (2020). Past behaviour, financial literacy and investment decision-making process of individual investors. Int. J. Emerg. Mark. 15, 1243–1263. doi: 10.1108/IJOEM-07-2018-0379
Rosenberg, E. (2022). The Difference Between Volatility and Risk [Review of the Difference Between Volatility and Risk]. Prudential. Available at: https://www.prudential.com/financial-education/difference-between-volatility-and-risk#:~:text=A%20beta%20below%201.0%20means,20means,squared%2C%20and%20the%20Sharpe%20ratio (accessed August 10, 2024).
Schwarz, G., Christensen, T., and Zhu, X. (2022). Bounded rationality, satisficing, artificial intelligence, and decision-making in public organizations: the contributions of Herbert Simon. Publ. Admin. Rev. 82, 902–904. doi: 10.1111/puar.13540
Semko, R. (2019). Machine learning for robo-advisors: testing for neurons specialization. Invest. Manag. Fin. Innov. 16, 205–214. doi: 10.21511/imfi.16(4).2019.18
Sent, E.-M. (2004). Behavioral economics: how psychology made its (limited) way back into economics. Hist. Polit. Econ. 36, 735–760. doi: 10.1215/00182702-36-4-735
Seo, M.-G., Goldfarb, B., and Barrett, L. F. (2010). Affect and the framing effect within individuals over time: risk taking in a dynamic investment simulation. Acad. Manag. J. 53, 411–431. doi: 10.5465/amj.2010.49389383
Severino, F., and Thierry, S. (2022). Robo-advisors: a big data challenge. Big Data Fin. 7, 115–131. doi: 10.1007/978-3-031-12240-8_7
Sharpe, W. F. (1964). Capital asset prices: a theory of market equilibrium under conditions of risk. J. Fin. 19, 425–442. doi: 10.1111/j.1540-6261.1964.tb02865.x
Simon, H. A. (1955). A behavioral model of rational choice. Quart. J. Econ. 69, 99–118. doi: 10.2307/1884852
Simon, H. A. (1991). Bounded rationality and organizational learning. Org. Sci. 2, 125–134. doi: 10.1287/orsc.2.1.125
Sironi, P. (2016). FinTech Innovation: From Robo-Advisors to Goal Based Investing and Gamification. Hoboken, NJ: Wiley.
Statista Market Insights (2023). Robo-Advisors—Worldwide. Statista Market Forecast. Statista. Available at: https://www.statista.com/outlook/fmo/wealth-management/digital-investment/robo-advisors/worldwide (accessed August 1, 2023).
Tan, G. K. S. (2020). Robo-advisors and the financialization of lay investors. Geoforum 117, 46–60. doi: 10.1016/j.geoforum.2020.09.004
Thaler, R. H. (2016). Behavioral economics: Past, present, and future. Am. Econ. Rev. 106, 1577–1600.
Thomaz, F., Salge, C., Karahanna, E., and Hulland, J. (2020). Learning from the Dark Web: leveraging conversational agents in the era of hyper-privacy to enhance marketing. J. Acad. Market. Sci. 48, 43–63. doi: 10.1007/s11747-019-00704-3
Torrey, C., Fussell, S., and Kiesler, S. (2013). How a Robot Should Give Advice (Tokyo: IEEE), 275–282.
Tversky, A., and Kahneman, D. (1974). Judgment under uncertainty: heuristics and biases. Science 185, 1124–1131. doi: 10.1126/science.185.4157.1124
Wexler, M. N., and Oberlander, J. (2021). Robo-advisors (RAs): the programmed self-service market for professional advice. J. Serv. Theor. Pract. 31, 351–365. doi: 10.1108/JSTP-07-2020-0153
Wu, P. C. S., and Wang, Y.-C. (2011). The influences of electronic word-of-mouth message appeal and message source credibility on brand attitude. Asia Pacific J. Market. Logist. 23, 448–472. doi: 10.1108/13555851111165020
Keywords: behavioral finance, robo-advisor, investment behavior, risk, affective trust, financial literacy, artificial intelligence
Citation: Eichler KS and Schwab E (2024) Evaluating robo-advisors through behavioral finance: a critical review of technology potential, rationality, and investor expectations. Front. Behav. Econ. 3:1489159. doi: 10.3389/frbhe.2024.1489159
Received: 31 August 2024; Accepted: 07 October 2024;
Published: 28 October 2024.
Edited by:
A. M. Elsawah, Beijing Normal University–Hong Kong Baptist University United International College, ChinaReviewed by:
Luca Polonio, Università degli studi di Milano Bicocca, ItalyCopyright © 2024 Eichler and Schwab. This is an open-access article distributed under the terms of the Creative Commons Attribution License (CC BY). The use, distribution or reproduction in other forums is permitted, provided the original author(s) and the copyright owner(s) are credited and that the original publication in this journal is cited, in accordance with accepted academic practice. No use, distribution or reproduction is permitted which does not comply with these terms.
*Correspondence: Kim Sandy Eichler, a2VpY2hsZXJAZWdvLnRoZWNoaWNhZ29zY2hvb2wuZWR1
Disclaimer: All claims expressed in this article are solely those of the authors and do not necessarily represent those of their affiliated organizations, or those of the publisher, the editors and the reviewers. Any product that may be evaluated in this article or claim that may be made by its manufacturer is not guaranteed or endorsed by the publisher.
Research integrity at Frontiers
Learn more about the work of our research integrity team to safeguard the quality of each article we publish.