- 1Department of Management, Faculty of Business Administration and Economics, Paderborn University, Paderborn, Germany
- 2Medical School OWL, Medical Assistance Systems, Bielefeld University, Bielefeld, Germany
- 3CITEC, Center for Cognitive Interaction Technology, Bielefeld University, Bielefeld, Germany
Introduction: Although decision support systems (DSS) that rely on artificial intelligence (AI) increasingly provide explanations to computer and data scientists about opaque features of the decision process, especially when it involves uncertainty, there is still only limited attention to making the process transparent to end users.
Methods: This paper compares four distinct explanation strategies employed by a DSS, represented by the social agent Floka, designed to assist end users in making decisions under uncertainty. Using an economic experiment with 742 participants who make lottery choices according to the Holt and Laury paradigm, we contrast two explanation strategies offering accurate information (transparent vs. guided) with two strategies prioritizing human-centered explanations (emotional vs. authoritarian) and a baseline (no explanation).
Results and discussion: Our findings indicate that a guided explanation strategy results in higher user reliance than a transparent strategy. Furthermore, our results suggest that user reliance is contingent on the chosen explanation strategy, and, in some instances, the absence of an explanation can also lead to increased user reliance.
1 Introduction
The application of artificial intelligence (AI) as a guidance source is extensive in various domains, including medical decision-making (Wang et al., 2019), finance (Binns et al., 2018), fraud detection (Cirqueira et al., 2020), and online advertising (Eslami et al., 2018). AI-powered decision support systems (DSS) are pivotal in assisting human users during the decision-making journey, offering advice to direct users toward their optimal selections. However, it is essential to note that the ultimate decision-making authority remains with the user. Recently, attention has been directed toward the AI-driven support mechanisms within Explainable AI (XAI) (Chazette and Schneider, 2020).
Adadi and Berrada (2018, p. 2) define XAI as “a research field that aims to make AI systems results more understandable to humans.” A major issue to date is the opacity of numerous algorithms. In other words, they cannot elucidate how they arrive at their decisions, such as identifying the specific features or input values that exert the most significant influence on the decision-making process. Even if one can effectively communicate and explain the importance of these features, users do not always process these explanations as intended, and their interpretations of AI explanations might not always align with logical or evidence-based reasoning (Bauer et al., 2023). Therefore, it is imperative to tailor explanations to suit the individual receiving them (Bronner, 2006). Much of the research is somewhat constrained by its focus on the viewpoints of data scientists and machine learning practitioners (Ren et al., 2016). Recent studies mainly concern technical solutions for XAI, such as the translation of Lime or Shapley values (Bauer et al., 2023). The question of what constitutes an effective explanation from a human-centered perspective warrants more extensive investigation (Riedl, 2019; Weitz et al., 2019).
In addition to technical solutions, the significance of interaction design is emphasized, particularly in the context of human-centered explanations that elucidate how the AI arrived at its advice (Laato et al., 2022). In a broader sense, two key challenges emerge in the communication between AI and humans: Firstly, the decision-making scenario may prove too complex for humans, entailing numerous parameters that lead to specific advice from the DSS. Secondly, the advice the DSS provides inherently involves risks, as specific parameters may contain stochastic elements whose characteristics remain uncertain or whose model may not cover all relevant characteristics. In such instances, effective communication must convey complexity and uncertainty, representing potential hazards for the human decision-maker. AI assistance becomes particularly valuable when decisions become uncertain or complex. In cases where decisions are made under uncertainty, it is crucial to convey this uncertainty, often through likelihoods or confidence intervals. Numerous approaches to explaining decisions have been explored, including those related to risk assessments in fields such as medicine (Schoonderwoerd et al., 2021), public administration (de Bruijn et al., 2022), and cybersecurity (Holder and Wang, 2021; Srivastava et al., 2022).
Given this background, the present study aims to understand the effects of various explanation strategies on user reliance within the context of XAI through experimental inquiry. Specifically:
1. Are there differences in user reliance after receiving advice from the DSS between:
• the two groups that received accurate explanation strategies (transparent vs. guided)?
• the two groups exposed to human-centered explanations (emotional vs. authoritarian)?
• groups receiving any explanation compared to a group that has not received any (control)?
2. How does an explanation strategy influence user reliance in general?
In our research, we employ a conventional economic experiment involving a lottery, which inherently embodies the stochastic nature of its outcomes. In this context, risk arises from the uncertainty concerning the lottery's outcome. Importantly, different strategies within this lottery present varying levels of risk. Enhancing the decision-making process within such a system can lead to significant improvements, as decision-makers often deviate from rational decision-making and their ideal level of risk preference. Individuals typically have a stable risk preference (Mata et al., 2018; Schildberg-Hörisch, 2018): Some enjoy taking risks, while others are averse to risk, resulting in psychological gains or losses. The appropriate risk level should align with the perceived stakes and the probability of experiencing losses. Consequently, the optimal decision becomes a function of an individual's expected utility and disutility, also considering the psychological costs or benefits of risk-taking. However, individuals cannot always select their optimal risk level from a choice set, e.g., because it is too complex or their emotions interfere (Fessler et al., 2004).
Through the inherent riskiness of the lottery scenario, the decision-making context becomes intertwined with ambiguity and uncertainty. Furthermore, personal preferences regarding risk may remain undisclosed to users and influenced by emotional factors. In such a scenario, AI can facilitate decision-making by offering guidance toward the optimal choice. The majority of the existing literature primarily emphasizes the technical aspect, aiming at elucidating the functionality of the system or the role of specific features. One of our contributions in this paper is how the concept of risk can be communicated effectively using different explanation strategies. Furthermore, we contribute to the research of data scientists and machine learning practitioners by deriving potential improvements for future DSS designs and formulating practical implications based on our findings. Our research also underscores the significance of operationalizing user reliance in this context. To this end, we consider three distinct behavioral responses drawn from the existing literature to capture user reliance: advice-taking (i.e., adhering to or following the advice), distance from the advice, and reactance. By including the latter two measures, our analysis contributes to the array of behavioral responses beyond simply following or not following advice. Our findings indicate that guided explanations result in greater user reliance than fully transparent explanations. Depending on the comparison with alternative strategies, even the absence of an explanation can lead to greater user reliance rather than full transparency or strong human centering. In the subsequent step, we investigate the user's perception of the advice. Our results reveal that explanations can lead to increased levels of user reliance, but they do not necessarily translate into higher levels of trust compared to scenarios with no explanation.
The remainder of this paper is structured as follows: In the section dedicated to related literature, we introduce an interdisciplinary approach to XAI, merging insights from computer science, psychology, and economics. Our focus is on effectively conveying risks and providing guidance in situations involving risk rather than explicating the functioning of the DSS. To this end, we explore four distinct explanation strategies tailored to the decision-maker, drawing from the existing literature. Subsequently, we delineate the standard experimental setup commonly employed in laboratory economic experiments. Finally, we present the results and discuss them with previous research findings.
2 Related work
2.1 Approaches for explaining AI advice
The desire to equip AI with the ability to explain why it has come to particular advice has led to a large body of research in AI and machine learning. Research on how different explanation strategies affect understanding, trust, and subsequent decision-making is still in its early stages. The fundamental idea behind providing explanations in decision-making scenarios is that humans can significantly benefit from having the advice explained (Schemmer et al., 2022). Current research emphasizes XAI (Miller, 2019; Rohlfing et al., 2020). A meta-analysis by Schemmer et al. (2022) reveals considerable heterogeneity in approaches. For example, in an online study on Mechanical Turk with 48 participants, Larasati et al. (2020) investigated the influence of four textual explanations on the explainee's trust in an AI medical support scenario. They found that all four explanation strategies impacted trust, with the strategy phrased in general terms leading to the lowest trust scores. Nevertheless, research on behavior in response to explanations shows a difference in participants' behavior when provided with an explanation compared to not receiving an explanation. In an experiment with 45 participants, van der Waa et al. (2021) investigated the effects of rule-based explanations, example-based explanations, and no explanation on persuasive power and task performance in a medical decision support context. Results indicate that advice with no explanation was less persuasive, i.e., participants followed the system's advice significantly less than participants who received rule-based or example-based explanations.
In general, current research results are very heterogeneous and the existing AI explanations could not always get the user to follow the advice (Schemmer et al., 2022). The literature on XAI and explainable human-robot interaction (HRI) further distinguishes between two generic explanation strategies: (1) Some explanation approaches that focus on technical solutions emphasize transparency, i.e., the accurate and complete presentation of information. (2) Recently, considering human nature has also become viable. However, this research focuses on explanations in human-computer interaction (HCI). In total, we will take a closer look at four explanation strategies. The first two strategies are motivated by the accurate information strategies used in classical XAI approaches like SHAP (SHapley Additive exPlanation) or LIME (Local Interpretable Model Agnostic Explanation). The latter two are more motivated by explanations in human-human interactions. We will discuss both types of strategies in the subsequent sections.
2.2 Accurate information strategies
One of the most widely used explanation methods in computer science is to achieve maximum completeness and accuracy of explanations. In this context, especially the designation known as feature importance rating plays a crucial role. That means that the approach depicts the primary features contributing to a classification. Aside from those features that support the classification, there are also potential features contradicting this category. However, their influence must be substantial to alter the model's classification into a different category. For example, explanations for this type are: “Because of features A and B, this item has been classified as class Y, even though feature C is an indicator against class Y.” Regarding recommender systems, Nunes and Jannach (2017, p. 12) propose the following explanation: “The recommended alternative has A and B as positive aspects, even though it has C as a negative aspect.” Generally, one can differentiate between local vs. global explanation approaches (Speith, 2022). Local approaches elucidate the classification of individual items, i.e., the reasoning for assigning a particular case to a specific class. One of the first local explanation strategies, LIME, was proposed by Ribeiro et al. (2016). Conversely, global approaches aim to comprehensively explain the whole model, i.e., focusing on the primary features that mainly influence the classification of most cases (Speith, 2022; Baniecki et al., 2023). A famous category of global explanation strategies involves perturbation methods such as SHAP (Cohen et al., 2005; Lundberg and Lee, 2017), or SAGE (Shapley Additive Global Importance) (Covert et al., 2021). The most pertinent features across the model can be determined by modifying or eliminating individual feature values and monitoring any resulting alteration in their predicted class. This leads to explanations such as: “The model predicts items belonging to class Y by considering the values of the features A and B.” Certain approaches can also be adapted to generate local explanations, such as SHAP. This provides the user with an interface for modifying the value of a particular feature and monitoring its impact on the classification.
Determining the optimal level of transparency in implementing feature-based explanations is an ongoing investigation (Miller, 2019). Contemporary research indicates that transparency influences reliance (Xu et al., 2014) and cognitive overload might be a factor to consider when contemplating advice-taking (You et al., 2022). Cognitive psychology posits that providing complete and transparent information can potentially lead to cognitive overload in individuals receiving the explanation. The same applies when humans face excessive options (Cramer et al., 2008). Previous research has demonstrated that cognitive overload may impact users' confidence (Hudon et al., 2021) and trust in the system (Schmidt et al., 2020) at the risk of users' reliance. In an online experimental study, You et al. (2022) investigated the influence of different levels of transparency on advice-taking. The results show that individuals exposed to a detailed representation are less inclined to follow advice than those provided with no or an aggregated representation. Considering prior studies on information overload, we posit that a more selective explanation strategy, emphasizing only the crucial aspects, might outperform full transparency. The present paper refers to this selective explanation strategy as “Guided.” We hypothesize that a guided explanation leads to higher user reliance than a fully transparent explanation (H1).
2.3 Human-centered AI advice giving
Another key factor in XAI relates to emotions. Current research concerns incorporating emotions into human-machine interactions (Slovak et al., 2023). While existing methods enable the measurement of emotions, research into effective explanations is essential to integrate emotions into AI interaction seamlessly. In the field of HRI, the connection between emotions and trust has already been studied. In an experiment with 387 participants, Schniter et al. (2020) examined behavior and emotions within the context of a trust game. The study encompassed three distinct conditions, in which participants interacted with one of the following: another human, a robot, or both a robot and a human simultaneously. The results indicate that humans are inclined to undertake risks to engage with robots trustfully. However, they show different emotional responses during these interactions. Consequently, trust-based interactions involving humans and robots that influence other humans elicit more intense social-emotional reactions than trust-based interactions with robots alone. Therefore, machines can elicit and alter emotional states (Rosenthal-von der Pütten et al., 2013). Recent studies in the field of HCI concerning decision-making reveal that, in addition to the technology-centric view, a more human-centered perspective is also pertinent (Springer and Whittaker, 2020).
Within interpersonal interactions, an authoritarian communication style is quite common. Authoritarian frameworks have been subject to extensive examination in the context of human-human interactions, e.g., at work (Karambayya et al., 1992), in schools (Grasha, 1994), or in child education (Smetana and Asquith, 1994). Contemporary research also examines authoritarian frameworks in HRI. In a simulated emergency experiment employing a between-subjects design, Nayyar et al. (2020) investigated the impact of robot-delivered messages on participant's decision-making processes. They focused on the effects of four distinct message types characterized by varying levels of explainability. For example, the authors proposed placebo explanation strategies devoid of pertinent information, (e.g., the “because I'm a robot” approach), in contrast to either an authoritarian explanation, which included information regarding the robot's expertise, or an explanation containing the rationale behind the recommendation (e.g., “I know the shortest path to the exit”). The findings indicate a statistically significant increase in participants following the robot's guidance when the robot gave an authoritarian explanation vs. no explanation. In another study with 60 participants, Maggi et al. (2021) explored the influence of the social robot Pepper using three distinct interaction styles—friendly, authoritarian, and neutral—on cognitive performance during a psychometric assessment. The findings reveal a significant enhancement in cognitive performance associated with the authoritarian interaction style. In particular, the authoritarian interaction style yields superior cognitive performance, particularly in tasks that require high cognitive resources.
In human interactions, the presentation of emotional explanations indicates empathy and comprehension, which augments a person's sense of understanding. We assume that an AI explanation will be more positively received by the human explainee when tailored to human factors and integrated with human requirements (Weitz et al., 2019), i.e., considering non-factual aspects, such as emotions, in comparison to authoritarian explanation approaches that do not explicitly cater to human requisites. We anticipate that emotions will have a significant impact and hypothesize that an emotional explanation leads to higher user reliance than an authoritarian explanation (H2). This hypothesis is based on the assumption that relying on an authoritarian explanation depends on the context. Therefore, the authoritarian explanation could lead to higher user reliance in an emergency (Nayyar et al., 2020) or in case it is provided by a teacher at school (Grasha, 1994). But in situations without an emergency or a hierarchy between the explainer and the explainee, we assume that the emotional explanation leads to higher user reliance than the authoritarian explanation.
It has been proposed that the simultaneous presentation of different explanation methods enhances decision-makers' understanding. However, it remains unclear whether providing accurate information explanations (transparent or guided explanations) or human-centered explanations (addressing emotions or authoritarian strategies) exerts an increased adherence to human advice-taking. This unresolved question constitutes a point for exploration within the scope of the present paper.
2.4 Behavioral outcomes of XAI
Numerous research investigations assess the impact of AI on self-reported trust in advice provided by the AI. Typically, trust is determined by direct inquiries from users. In addition, other studies examine participant behavior by observing the extent to which the advice is followed. Whereas trust is frequently evaluated via self-reported assessments, user reliance is measured by the metric of advice-taking. Scharowski et al. (2022) further emphasize the relevance of the metrics employed in XAI research. The way in which researchers operationalize these metrics has consequences for the variable of interest. Failing to differentiate between these two metrics can confuse. Therefore, the authors define trust as a psychological construct encompassing a subjective attitude, whereas user reliance pertains to directly observable and objective behavior. Nevertheless, empirical findings exhibit variability. In an online testing environment designed to emulate a human-robot team task within a rescue setting, Wang et al. (2016) explored three distinct types of explanations alongside a control condition devoid of explanations. The primary objective was to examine the effects of these explanations on self-reported trust, understanding of the robot's decision-making process, and overall team performance. This investigation was conducted in a between-subject experimental design featuring robots of varying competency levels. The results indicate that the additional explanations enhance both trust and team performance. In particular, explanations that facilitated the decision-making process led to elevated ratings. When participants had difficulty discerning the derivation of the advice, this explanation received a low rating, comparable to receiving no explanation at all, particularly when the robot's abilities were limited. Most interestingly, although trust increased with the provision of explanations, the users' behavior, specifically their adherence to the robot's advice, remained unchanged regardless of whether explanations were given or not. In contrast, Cheng et al. (2019) found no discernible difference in trust between explanatory vs. non-explanatory conditions. Chong et al. (2022) highlighted that trust levels are contingent on the AI system's perceived expertise. Participants were guided by an AI in a chess game and were asked to rate their trust after each piece of advice. Trust declined sharply when harmful advice was deliberately given, while trust recovery through good advice was notably inert.
Research in AI and XAI occasionally utilizes the concepts of behavior and attitudes interchangeably, leading to potential confusion (Scharowski et al., 2022). By implementing a clinical DSS interface, Bussone et al. (2015) investigated the correlation between explanations, trust, and reliance. They conducted a user study using a between-subjects design. In addition to self-reported trust, the authors examined the influence of explanations on reliance by quantifying the occurrences in which users concurred with the system's diagnoses or appropriately determined their actions following the advice provided. In this context, an expansive elucidation of the diagnostic factors positively affected self-reported trust. This, however, also led to a tendency toward over-reliance. Conversely, more concise explanations resulted in issues with reliance. In contrast, Panigutti et al. (2022) investigated the impact of explanations within an AI-based clinical DSS on the decision-making processes of healthcare providers. They compared two scenarios, one in which the clinical DSS explains its advice and another in which it does not. Their investigation revealed a significant effect on advice-taking when the DSS decision was explained. Nevertheless, they could not observe a substantial disparity in explicit trust levels between the two conditions. Bayer et al. (2021) conducted an inquiry into XAI within an AI-based DSS designed to assist users in generating chess move suggestions. Their examination assessed the link between trust and advice-taking. While they could affirm a correlation between user intent and behavior within the context of AI-based DSS, it is worth noting that the effect was relatively modest. Therefore, the authors recommend that “[i]nvestigating intention is only a mediocre proxy for studying user behavior” (Bayer et al., 2021, p. 21).
Measuring user reliance is typically operationalized using the weight of advice measure (Bailey et al., 2022; Panigutti et al., 2022). The weight of advice captures the distance to advice, but not reactance. Reactance to AI is a prevalent problem (Ehrenbrink and Prezenski, 2017; Sheng and Chen, 2020), and a mere assessment of the mean weight of advice may prove insufficient in effectively capturing these complex response patterns. Furthermore, various explanation strategies may elicit distinct levels of reactance. Sankaran et al. (2021) revealed that providing comprehensive and fully transparent explanations of an AI system may mitigate reactance toward AI. In our paper, we refrain from utilizing the weight of advice. Still, we measure user reliance by monitoring individuals' adherence to the advice, responsiveness to the recommended course of action, and degree of conformity.
This paper discusses two precisely formulated hypotheses and two unresolved questions.
• H1: In the group of accurate information strategies, guided explanations lead to higher user reliance than fully transparent explanations.
• H2: Emotional explanation strategies lead to higher user reliance than authoritarian strategies in human-centered explanations.
• An open question is which explanation strategy from H1 and H2 works best overall. We aim to gain some first insights from our study.
• Another open question is which explanation strategies maximize user reliance, trust, satisfaction, and perceived quality.
We further assume that user reliance may not have an immediate connection to trust and that trust may be altered following an interaction. Consequently, we emphasize the analysis of advice-taking and distance from advice while separately examining the trust-related effects of various explanation strategies.
3 Methods
3.1 Study implementation and participants
We conducted a web-based interactive experiment between December 2022 and January 2023, for which we recruited participants via the Prolific platform. Our study focused on German and English native speakers from Europe and North America. Before the start of the experiment, a pretest was conducted to forestall any potential comprehension issues and ensure the readability of our materials. All participants received detailed information regarding data privacy. No preliminary details regarding the study's objectives or the social agent Floka were disclosed to the participants. Participants were randomly assigned to one of five conditions in a between-subjects experimental design: (1) Fully Transparent Explanation (“Transparent”), (2) Guided Explanation (“Guided”), (3) Explicit Emotional Debiasing Explanation (“Emotional”), (4) Authoritarian Explanation (“Authoritarian”), and (5) No Explanation (“Control”). In terms of procedures, all groups are standardized, with the sole variation in the explanation strategy specific to each condition. The distinct explanation strategies are presented in 3.2.3.
To ascertain participants' comprehension, we asked a series of questions. Due to issues related to the comprehension of the lottery task, we excluded 13 participants from the data set. Additionally, only those participants who completed the entire experiment were considered. Consequently, the final dataset comprises N = 742 participants. Overall, the average time required for participants to complete the experiment was 12.76 minutes. Each participant received an average compensation of 7.30 euros (SD = 1.29). The sample shows a well-balanced distribution of participants, with 20% assigned to the “Guided” treatment group, 21% to the “Authoritarian” treatment group, 20% to the “Emotional” treatment group, 18% to the “Transparent” treatment group, and 21% to the “Control” group. Half of the participants were female. The average age of the participants was 34 years (SD = 12).
3.2 Setup
The fundamental framework adheres to the standard risk-choice experiment (Holt and Laury, 2005). Decision-makers must select their preferred level of risk—ranging from relatively secure to relatively precarious options. Opting for the riskier alternative offers the possibility of higher net gains but, at the same time, involves a higher probability of earning less compared to the safer option. Participants are required to determine the risk level that aligns with their preferences. Prior research has consistently demonstrated substantial variability in risk attitudes within this decision-making situation. Some individuals associate taking high risks with elevated psychological costs, while others enjoy engaging in risk-prone behaviors. As a result, an optimal choice exists for each individual based on their unique risk disposition. Nonetheless, existing research also suggests that emotions may introduce a degree of ambiguity in the optimal decision-making process, potentially luring individuals away from selecting their most fitting risk level. This emotional influence may lead individuals to assume excessive or inadequate risk instead of making choices in alignment with their risk attitude (Conte et al., 2018).
In our study, the main focus is on providing explanations. We have integrated a virtual agent that motivates participants through textual interactions to enhance the questionnaire completion process. The current version of the explanation process offers a single suggestion without the possibility of subsequent discussion, leaving the question of interactivity to future investigations. The chosen experimental setup was diligently designed to leverage a DSS that accounts for the impartial risk preferences of each participant (Anderson and Mellor, 2009). Drawing upon prior research, we are also aware of potential emotional biases inherent in this experimental setting, which were leveraged in the treatment (“Emotional”). Within our experimental framework, individuals completed a questionnaire to assess their risk attitudes. Following this assessment, participants made selections from the Holt and Laury lottery that aligned with their preferences. We subsequently assessed the alignment of their risk attitudes to their actual selection. If a participant's choice deviates from their optimal choice, indicating a disparity between their initial choice and risk preferences, the DSS intervenes by recommending a more suitable option. The success of the explaining process is contingent on its ability to guide the decision-makers toward selecting the optimal risk level based on their preferences. Conversely, if the explanation process proves ineffective, the decision-maker may persist with their original choice, opt for a suboptimal selection, or even exhibit signs of reactance by moving in the opposite direction of risk. Following advice, participants were instructed to make a subsequent lottery selection. We measured the extent to which they considered the provided advice based on the received explanations and conducted a between-participant analysis. Subsequently, we provide a more detailed overview of our methodological approach, and the complete questionnaire can be found in the Supplementary material “Questionnaire”.
3.2.1 Elicitation of risk preferences and emotional state
We assessed risk preferences and emotional states using established questionnaires. To determine an individual's personal risk level upon which the advice for a specific lottery is contingent, we used eleven questions from the German Socio-Economic Panel (GSOEP) (Sozialforschung, 2014). In selecting these eleven questions, we relied on their significant predictive power, as described by Fox and Tannenbaum (2011). We conducted median splits using data from the GSOEP to establish a straightforward scoring system. If an individual's response to a question was above the median, we assigned one point [or subtracted one point, depending on the direction of the risk relationship identified by Fox and Tannenbaum (2011)]. The final score was translated directly into the optimal risk level. This scoring system is an appropriate and functional indicator for guiding the personal risk assessment in our experimental design. In addition to assessing individual risk levels, we also evaluated the current emotional states of the respondents. The Modified Differential Emotions Scale (mDES) (Fredrickson, 2013) contains 20 questions in which participants rated their feelings on a 5-point Likert scale. Half of the questions are related to positive and the other half to negative emotions. Hence, the result derived from the mDES covers a possible range of 40 points each for positive and negative emotions.
After the initial questionnaire, participants were invited to engage in the Holt and Laury lottery twice. The first time, they participated without assistance, while the second time, an algorithm provided explanations corresponding to four experimental conditions. In the control group, the explanations were absent.
3.2.2 Decision task
In the study, participants' risk behavior was assessed by letting them choose between various Holt and Laury lottery scenarios. The standard tabular representation of the Holt and Laury lottery can be difficult to understand. Therefore, we opted for a visual representation—employing an urn and colored balls to illustrate the lottery—in order to enhance clarity (see Figure 1). This visualization illustrates the probability and value of each ball. Participants could choose between options (Lottery A and Lottery B) and were instructed to mark the point at which they preferred to transition from the left to the right side in the visual representation. No initial selection was given to prevent nudging. Furthermore, we provided a statement to inform the participants that they could win between 0.10 and 3.85 euros in addition to the regular compensation for their participation in the study. In this experimental design it is assumed that individuals inclined toward risk would choose option B in the first lottery, while risk-averse individuals would opt for option A. The transition point from option A to option B was used to indicate the participants' degree of risk aversion: a later transition indicates greater risk aversion.
3.2.3 Experimental manipulation
We aim to investigate the efficacy of various explanation strategies in fostering user reliance and providing optimal support to decision-makers. We assess the most beneficial decision by relying on an AI system's computation that considers the user's risk preference and thus determines the recommended risk selection. We want to gain insight into how XAI can cater to the distinct requirements of individuals, incorporating non-factual aspects and applying these explanation strategies to enhance decision-makers comprehension of uncertain situations. In our review of selected XAI approaches, we have provided a concise introduction to feature explanation approaches exemplified by techniques like LIME or SHAP. These methods elucidate the key features that have exerted the most influence on the AI system's decision. Typically, these approaches present a substantial number of features to the decision-maker, ranging from 10 to 20. However, even if these feature sets do not include all the features – which could number in the hundreds – the quantity of presented features makes it difficult for the decision-maker to process them in a way that enables informed decision-making. Instead of pursuing full transparency via an extensive set of features, our proposal pleads for the judicious selection of the most pertinent features, which are subsequently elucidated using natural language in a guided manner. This approach involves describing the influence of each selected feature for the decision-making process. Our overarching project aims to assist individuals who may find themselves in emotionally charged states that can influence their decision-making. We have also introduced a strategy explicitly designed to clarify the effects of specific emotional states. This strategy addresses the possibility that emotional factors may have influenced the user's first impulsive decision. We name this strategy explicit emotional debiasing. In addition, we have incorporated another distinct explanation strategy characterized by an authoritarian style. This configuration leads to four unique explanation strategies, extended with a fifth condition without explanations. Our overarching goal is to concentrate on local rather than global model explanations to provide tailored support for a specific decision-making scenario.
Following the initial phase of our interactive experiment, we introduce our AI visualization, Floka, which provides the explanations. Our research project's fundamental hypothesis revolves around the notion that an adequate level of understanding and effectiveness in explaining AI can only be achieved through collaborative, dialogic co-construction (Rohlfing et al., 2020). This requires the engagement of two agents who collaboratively strive to construct explanations sufficiently, actively contributing through questions and elaborations. It also requires establishing and maintaining a partner model, in which the explainer consistently tracks and adapts their explanations based on the explainee's evolving level of understanding. This process hinges on a dialog between two agents and highlights the necessity of an AI-based agent with the capability to engage in social interaction with the explainee. Hence, we decided to incorporate Floka into this study, a visually improved virtual version of its predecessor called Flobi (Lütkebohle et al., 2010). Flobi is characterized as an anthropomorphic robot head that unifies the features of a mature, serious-looking adult visage with endearing baby face attributes, known to enhance perceptions of trustworthiness (Hegel et al., 2010; Lütkebohle et al., 2010). Among others, these features include a miniature head, a petite nose and chin, sizeable round eyes, and characteristically low positioning of the eyes, nose, and mouth on the face. In a systematic review, Song and Luximon (2020) concluded that features corresponding to the baby schema, such as large, round eyes, provide an evolutionary advantage regarding the perceived trustworthiness of human facial characteristics. Floka introduces itself as a social and intelligent agent in this introductory phase. Subsequently, Floka offers participants guidance regarding their optimal choices tailored to their risk profiles. Participants were randomly assigned either to one of the four treatment groups or to the control group.
An overview of the four treatment groups and the additional control group “Control” is provided in Table 1. Our formulation of these explanation strategies was inspired by the recommendations outlined by Amershi et al. (2019), who derived these strategies from human-AI interaction design guidelines. Our approach focuses on methods best suited for designs within the field of HCI. These include incorporating contextually relevant information and introducing the social agent when participants have already had the opportunity to interact with the task independently. The first explanation strategy, referred to as “Guided,” has been adapted from Schoonderwoerd et al. (2021) and incorporates a fusion of supporting and conflicting information and comparisons to other examples. This strategy effectively streamlines the information and employs individual elements to guide the participant toward the appropriate decision. In detail, this strategy comprises three arguments, each relating to different risk directions. A statement is a sentence associated with a specific item and its direction. For instance, if a participant has indicated a tendency for risk-taking in a workplace scenario, an argument that goes in a risk-oriented direction might be articulated as follows: “You are more likely to take risks at work. You are also more likely to take risks in general.” The first two arguments relate to placing the advice within the lottery range. If the advice corresponds to lotteries one to three (see Figure 1), it is considered risky. Consequently, the first and second arguments are tailored to reinforce the willingness to take risks. If the advice corresponds to lotteries four to seven, one argument encourages risk-taking, while the other endorses risk-averse behavior. Lastly, both arguments support risk aversion if the advised lottery is between eight and ten. The content of the third argument depends on the direction in which the participant has moved away from their initial decision.
The “Authoritarian” strategy dispenses with an explanation and makes an authoritarian statement instead: “Another decision is inappropriate!”. This strategy is derived from human-human interactions and explains why the participant follows Floka's advice with minimal cognitive effort.
In laboratory research, Schütze et al. (2023) found empirical evidence that emotions influence risk-related decision-making. Consequently, we included an explanation strategy centered on emotional factors. This strategy does not utilize the collected risk data, instead it draws on emotional states to provide explanations. As with other strategies, the collected risk data serves as the basis for classification into a recommended risk level. The “Emotional” strategy uses the outcomes of the mDES scale to compute emotional tendencies that are used to generate an explanation. If the emotional tendencies lean more toward negativity than positivity, the resulting explanation will be “Your emotional state leans more to the negative side at the moment. Your emotions can lead to a biased assessment of your risk, so you should reconsider your lottery choice.” The rationale underlying this explanation strategy is that individuals are encouraged to follow Floka's advice depending on their prevailing emotional state, as emotions can alter the perception of risk explanation (Rosenthal-von der Pütten et al., 2013).
Regarding the “Transparent” explanation strategy, we followed the approach outlined by Schoonderwoerd et al. (2021). In this approach, all items derived from the questionnaire that were used to compute the risk level were presented together with an explanation of the impact of each response on these items. This approach assumes that participants understand how Floka arrives at its results and that their trust in the calculation motivates them to follow the advice.
The last group serves as a control group. No further explanations are given except that the advice is based on calculating the personality profile. Overall, Table 1 visually represents the explanation strategies and Floka's corresponding statements.
After providing explanations and advice, participants were allowed to modify their initial decision as part of the lottery. It is important to note that nothing was preselected. However, both the first decision and Floka's advice were highlighted for the participant. The participant was then required to actively make a new decision. The final decision was incentive-driven, with compensation depending upon the choice made within the lottery and a subsequent random draw.
3.2.4 Dependent variable
To ascertain the effectiveness of each explanation strategy in our research, we adopt a methodology in line with prior research, utilizing an individual's behavior—user reliance— as an objective metric for evaluating trust. We distinguish between user reliance, an objective measure, and trust, a subjective measure based on self-reported assessments (Lai et al., 2021). Additionally, we emphasize operationalization. On the one hand, we assess advice-taking by examining whether individuals act following the advice provided (Bussone et al., 2015; Levy et al., 2021; Wang and Yin, 2021). However, user reliance encompasses not only the general tendency to follow advice but also the degree of accuracy in following the advice and the question of whether it triggers reactance in certain individuals (Poursabzi-Sangdeh et al., 2021). Therefore, we also measure the disparity between the taken advice, the second choice and signs of reactance on behalf of the respondent (see Section 3.3 for a profound description of the distance from advice and reactance).
3.2.5 Questionnaire and attention check
Following the completion of the experiment, we asked for additional questionnaire-based information. Our web-based interactive experiment included demographic information. Prior studies have established that various factors influence advice-taking in computer-based environments (Jussupow et al., 2020; Chugunova and Sele, 2022; Mahmud et al., 2022). Thus, we also included an examination of participants' educational background and their interaction with technical systems, assessed by the Affinity for Technology Interaction (ATI) Scale (see Franke et al., 2019, α =.87). An example from this measure is “I like to occupy myself in greater detail with technical systems.” Participants provided ratings on a scale ranging from 0, “completely disagree”, to 6, “completely agree.” Furthermore, we included the subjective perception of trust – and for the sake of completeness – we also considered participants' satisfaction with the system and the quality of advice in our study. In evaluating Floka's explanation, we used the adapted trust (α =.91) and satisfaction (α =.87) scales designed for XAI by Shin (2021). Participants were queried using a 7-point Likert scale (1 = do not agree at all; 7 = agree completely). An item representing the trust assessment scale is: “I trust Floka's recommendations.” “In general, I am happy with Floka's content.” constitutes an example from the satisfaction query. We also asked questions about the perceived quality of the advice (Gino et al., 2012), α =.90), with participants indicating to what extent they agree with the statements (1 = very unlikely; 7 = very likely). One exemplary statement is the following: “Floka's advice was likely to be accurate.” Besides, we included two attention checks in our study to ensure the reliability of participants' responses. Participants were given explicit instructions to attentively read the text before providing their answers. All participants in our study answered both attention checks correctly.
3.2.6 Payment
In the final stage, participants were shown their earnings for the first time as they witnessed a random draw from an urn that ultimately decided their payment. Subsequently, the color of the ball was then also decided at random, leading to the calculation of the payout, which was then presented to the participant.
3.3 Analysis
To test the hypotheses posited in 2.4, the collected data is being analyzed and evaluated using STATA 17.0. For the graphical illustration, R version 4.2.2 is used. Our initial focus centers on examining the data from participants who followed Floka's advice. For this purpose, we generated a binary variable “advice-taking.” Suppose a participant changes their decision after receiving Floka's advice by adjusting or surpassing it. In that case, it is categorized as “1,” signifying the participant's adherence to the advice. We consider a case as “missing” when the first decision aligns precisely with the advice, and the second decision remains unaltered. This distinction is implemented in order to avoid a misinterpretation between adhering to the original decision and actively following the direction of the advice. If the decision remains unaltered or is not corrected to an opposing choice, it is designated as “0.” Figure 2 illustrates cases categorized as “0,” “1,” or as a “missing.” Furthermore, we evaluate the difference between advice and choice as a numerical metric, quantifying the distance from advice. Consequently, we generate a new variable, “distance from advice,” computed as the absolute difference between the second decision and the advice: DistancefromAdvice = |SecondDecision−Advice|
4 Results
Successful advice for users enables humans to choose a more appropriate individual risk level. In the scope of our study, we measure advice-taking, distance from advice, and signs of reactance. Our findings are presented in multiple sections to enable a comprehensive presentation. In 4.1, we deal with data from participants who followed Floka's advice, examining the relationship between the type of explanation and advice-taking and the distance from advice. Subsequently, we focus on reactance (4.2). In 4.3, we explore the influence of various factors on advice-taking using a multivariate probit model. In a subsequent step, we also analyze the factors accounting for the distance from advice. Section 4.4 provides an overview of our findings. The final 4.5 addresses participants' ratings of the perceived quality of the advice, trust in Floka's advice and satisfaction with Floka. We present the relevant results in a structured manner comprising three steps: Initially, we examine the ratings among distinct subgroups. Secondly, we look at the treatment groups and the subgroups. Finally, we compare the treatment groups and the control group.
In an initial assessment, we look at the distribution of reactions within the four treatment groups and the control group (Figure 3). We use the first and second choices to calculate a decision direction depending on the advice.
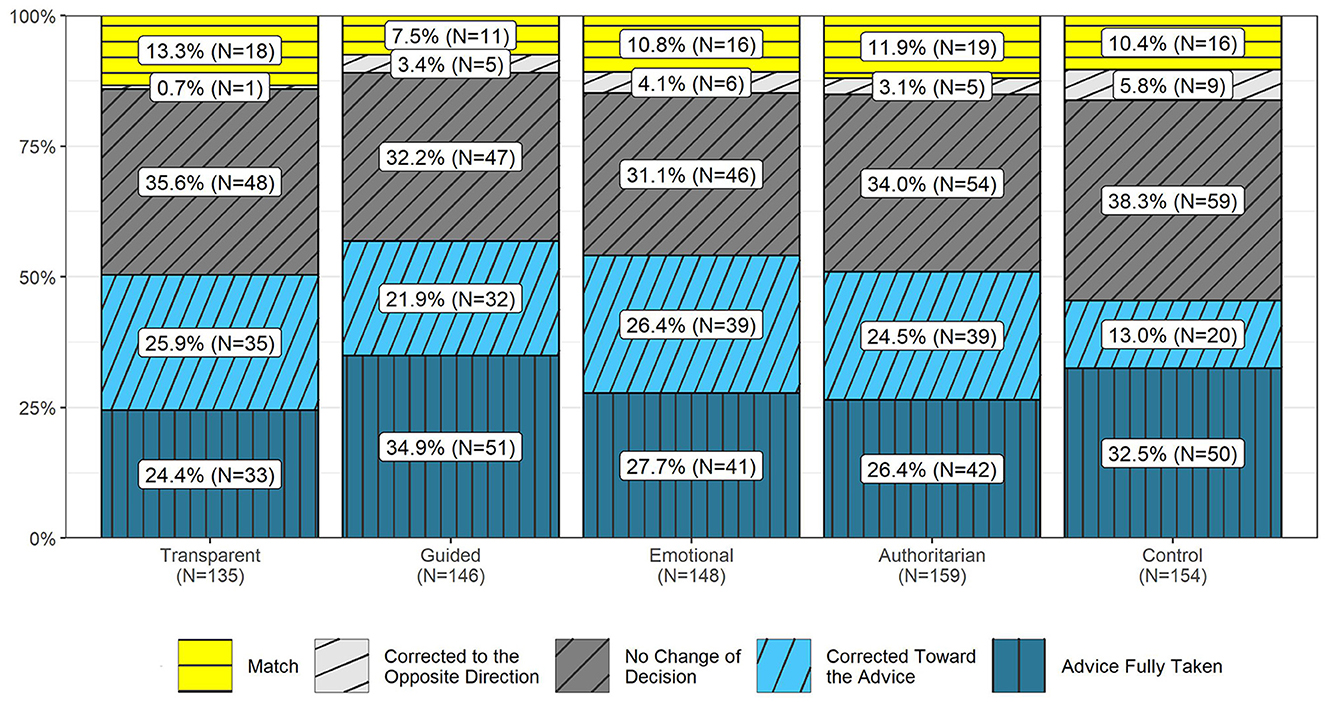
Figure 3. Percentage of the direction of the second decision, depending on the advice and explanation.
The results are presented as follows: (1) Advice fully taken occurs if the second decision aligns with Floka's advice. (2) Corrected toward the direction consistent with the advice, encompassing corrections approaching the advice and extending beyond the advice. (3) No change of decision (e.g., when the second decision is the same as the first), and (4) Decisions contradicting Floka's advice (e.g., decisions moving in the opposite direction). (5) Match where the first decision, the advice, and the second decision are identical.
Figure 3 visualizes that the explanation strategies “Guided” and “Emotional” lead to the highest level of adoption of Floka's advice (blue shades represent advice-taking; shades of gray represent advice-rejecting). The “Transparent” explanation strategy results in very few participants revising their decision in the opposite direction, which indicates minimal reactance. Specifically, only one participant corrected their decision in the opposite direction.
4.1 Advice-taking
First, we analyze participants who followed Floka's advice across treatment groups and the control group. This analysis evaluates the advice-taking frequencies (as illustrated in Figure 4), in which we combine the group of full advice-taking and the group of participants who partially aligned with it or even surpassed Floka's advice. In total, N = 382 participants are observed to have followed Floka's advice. As depicted in Figure 4, all treatment groups exhibit a notably higher proportion of individuals who followed the advice compared to the control group. This implies that participants exposed to some kind of explanation change their initial decision toward Floka's suggested direction, irrespective of whether that entails opting for a more or less risk-prone choice. Notably, the advice-taking rate in the treatment group “Guided” is the highest, with 61.48% of the participants following Floka's advice. Furthermore, the “Emotional” treatment group reveals a substantial advice-taking rate of 60.61%. Subsequently, the examination proceeds to the two remaining treatment groups, where participants in the “Transparent” condition take advice at a 58.12% rate and in the “Authoritarian” at a 57.86% rate, respectively. On the contrary, the control group, which did not receive any explanation input, shows the lowest rate of advice-taking at a 50.72% rate. Several chi-square tests of independence were performed to examine the relation between the explanation strategy and advice-taking (refer to Table Appendix 2 in the Supplementary material “Manipulation check and additional tables” for additional details). The results indicate a statistically significant difference in the frequency of advice-taking between the treatment group “Guided” and the “Control” group, χ2(1) = 1.42, p = 0.073. Examining the confidence interval for the estimated chi-square value reveals its inclusion of zero. Consequently, the available evidence is insufficient to substantiate the existence of a statistically significant distinction. Further chi-square tests of independence show no significantly different odds of advice-taking.
The choice to examine dichotomies represents just one potential operationalization of advice-taking. In addition to the binary analysis of advice-taking, we are equally interested in evaluating the precision with which individuals followed the advice within each experimental group. When individuals follow Floka's advice, the difference between their second choice and Floka's advice should ideally be zero. As displayed in Figure 3, it is evident that some individuals followed Floka's advice, although not always entirely, and in some cases, they even exceeded the advice recommended by Floka. Therefore, our analysis expands to the evaluation of the difference between the provided advice and the decision made. Among those who generally take the advice, we see, based on both mean and median, that the most substantial variations are evident within the “Transparent” treatment group (Mdn = 1.00, M = 1.24, SD = 1.54, as depicted in Table 2). The difference between the second decision and the advice given is lowest among those who generally followed the advice in the “Control” group (Mdn = 0.00, M = 0.66, SD = 1.37) and the “Guided” group (Mdn = 0.00, M = 0.88, SD = 1.40). Since the normal distribution assumption for residuals was not fulfilled, we conducted a set of two-sample Mann-Whitney U tests to investigate potential statistical differences between the treatment and control groups. The results are presented in Table 2. The “Control” group shows a significantly lower distance from advice, indicating a higher degree of advice-taking, when compared to the “Transparent” group (z = 2.87, p = 0.004), the “Emotional” group (z = 2.43, p = 0.015), and the “Authoritarian” group (z = 2.20, p = 0.027). Moreover, the results show that the “Guided” group displays a significantly lower distance from advice than the “Transparent” group (z = −1.68, p = 0.095). No significant difference in the distance from advice is observed between the groups “Guided” and “Control.” The results suggest that participants who do not receive any explanation input tend to follow the advice more closely but not fully.
4.2 Reactance
Explanations can both facilitate advice-taking and potentially provoke reactance in particular individuals. Rejecting advice may lead to the user sticking to their initial decision or moving in the opposite direction. Thus, reactance differs from ignoring the advice. While both groups reject the advice, only the latter group shows reactance. We compute the absolute difference value for individuals who reject the advice (refer to the formula in Section 3.3). Table 3 shows the median, mean, and the results of all Mann-Whitney U tests. When considering participants who reject the advice, the results indicate that the “Guided” group (Mdn = 2.00) exhibits a significantly lower distance, indicating their closer alignment with the advice than the “Transparent” group (Mdn = 4.00, z = −2.42, p = 0.015). In addition, receiving the “Emotional” treatment (Mdn = 2.50) results in a significantly lower distance from advice compared to the “Transparent” treatment (Mdn = 4.00, z = −1.82, p = 0.069).
4.3 Multivariate results
The following section examines whether control variables can account for the primary effect. Table 4 presents a probit regression model with “advice-taking” (0= “Reject,” 1= “Take”) as the dependent variable. In addition to the independent treatment variables, we incorporate controls for the initial choice, differences between initial choice and advice, age, gender, affinity for technology interaction (ATI), and education. In the subsequent part of the paragraph, we refer to marginal effects at the mean (as indicated in Table 4 using square brackets). After introducing the control variables, the analysis reveals that participants in the “Guided” treatment group are 11% more likely to take advice from Floka than the control group “Control.” None of the other treatment groups show statistically significant differences from the “Control” group. Further, individuals with less risky initial choices are 3% less likely to take advice. Participants with a greater difference between initial choice and advice are 5% more inclined to follow Floka's advice. Additionally, participants with any form of education, particularly those with a STEM-oriented education, are significantly more likely to take Floka's advice (16% and 13%, respectively) than individuals without formal education.
In the second column, we employ the absolute difference from advice as the dependent variable (the formula is detailed in Section 3.3). This variable can be any integer value between 0 (indicating no difference) and 7 (representing the maximum potential difference). The ordered probit model presented in Table 4 shows that, given other factors, explanation strategies do not significantly influence the distance from advice. The less risky the initial choice and the higher the differences between the first decision and the advice, the greater the likelihood of increased distance from advice.
4.4 Summary of the hypotheses testing
As we have examined the influence of an explanation strategy on user reliance by assessing three distinct operationalizations (refer to 4.1: Advice-taking and distance from advice; and 4.2: Reactance), we summarize the findings of our tests. Table 5 displays an overview.
Hypothesis 1 specifically postulates that “Guided” explanations lead to more user reliance than “Transparent” ones within the accurate information strategies group. We find support for this hypothesis: In pairwise comparisons, “Guided” explanations lead to significantly less distance from advice and less reactance than “Transparent” explanation strategies. This effect is more substantial for reactance. Hypothesis 2 states that “Emotional” explanation strategies lead to higher user reliance than “Authoritarian” explanations within human-centered explanations. However, we cannot support this hypothesis: Although the descriptive data suggests that “Emotional” explanations lead to more user reliance than “Authoritarian” explanations, advice-taking, difference from advice, and reactance do not reveal significant differences between the two groups. Concerning the open question about the superior performance of explanation strategies – specifically, accurate information strategies vs human-centered explanation strategies – our findings indicate which strategy outperforms the other. In this context, the measure of user reliance is crucial. In our paper, we have defined user reliance as advice-taking and the difference between advice and decision as reactance. None of the explanation strategies exhibits significant superiority over the other strategies in all pairwise comparisons or all user reliance measures. The probit regression analysis reveals that “Guided” leads to a significantly higher likelihood of advice-taking than “Control.” Controlling for various variables, the analysis shows that people in the “Guided” treatment group are 11% more likely to take advice from Floka than people in the “Control” group. In the probit regression analysis, no statistically significant differences were found between the control and other treatment groups. When solely considering the distance from advice as a measure of user reliance (see Table 2), we see that receiving no explanation can also be well received, as it becomes apparent that “Control” shows significantly less distance from advice compared to “Transparent,” “Emotional,” and “Authoritarian.” Further, the distinction between individuals who followed and rejected the advice is pertinent. For instance, an additional finding indicates that individuals who rejected the advice and were assigned to the “Emotional” group showed significantly lower reactance than participants in the “Transparent” group. This effect is not observed for those who followed the advice.
4.5 Quality of the advice, trust, and satisfaction with Floka
Depending on the explanation strategy, perceptions of Floka's advice quality may vary among individuals. Therefore, we compare perceived advice quality, trust in Floka's advice, and satisfaction with Floka across the different explanation treatments and among distinct subgroups.
First, we categorize individuals into three groups: Those who took Floka's advice (“Take”) and those who rejected Floka's advice (“Reject”). The third group includes those individuals who had initially chosen their optimal risk level in the first decision, subsequently received confirmation from Floka, and stuck to their initial decision, aligning with Floka's advice. We have excluded this group from our previous analysis since they neither took nor rejected Floka's advice. However, they interacted with Floka, indicating a degree of trust in the system. In this analysis, we include this group, denoted as “Match,” to clarify that they, quite possibly by coincidence, have already chosen the optimal level in their first decision.
Figure 5 displays the medians of all variables across the three groups. Two-sample Mann-Whitney U Tests were conducted to determine whether the subgroups differed in quality, trust, and satisfaction. The results, as detailed in Table 6, indicate that the subgroup “Match” has significantly higher ratings for the quality of advice than the other groups. Moreover, the subgroup “Take” demonstrates significantly higher quality ratings than the subgroup “Reject.” The results of the Mann-Whitney U tests indicate a significant difference for trust between the subgroups “Match” and “Reject” and between “Take” and “Reject,” respectively. In terms of satisfaction ratings, all conducted tests show a significant difference with comparisons such as “Match” vs. “Take,” “Match” vs. “Reject,” “Take” vs. “Reject.”
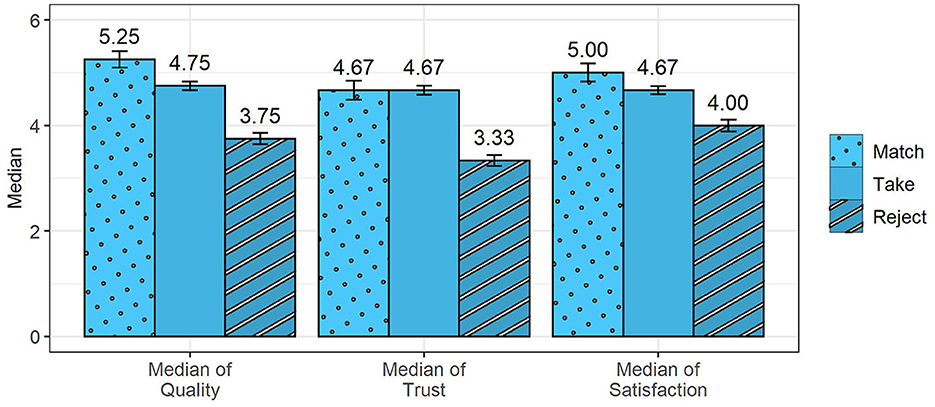
Figure 5. Median of quality, trust, satisfaction by subgroups (error bars = standard error of the median).
Secondly, to examine differences in quality, trust, and satisfaction among the treatment groups between the subgroups “Match,” “Take,” and “Reject,” we conduct further Mann-Whitney U tests by individually comparing each treatment group. Figure 6 displays the medians for each subgroup. The results of the Mann-Whitney U Tests are provided in the Supplementary material “Manipulation check and additional tables” Table Appendix 3–Table Appendix 11. Across all treatment groups, the subgroups “Match” and “Take” consistently show significantly higher values for quality, trust, and satisfaction compared to the subgroup “Reject.” Regarding quality, the subgroup “Match” when contrasted with “Take” shows significantly higher values in the treatment groups “Transparent” “Guided,” and “Authoritarian”. The two subgroups “Match” and “Take” differ significantly from each other in trust and satisfaction solely within the treatment group “Authoritarian.”
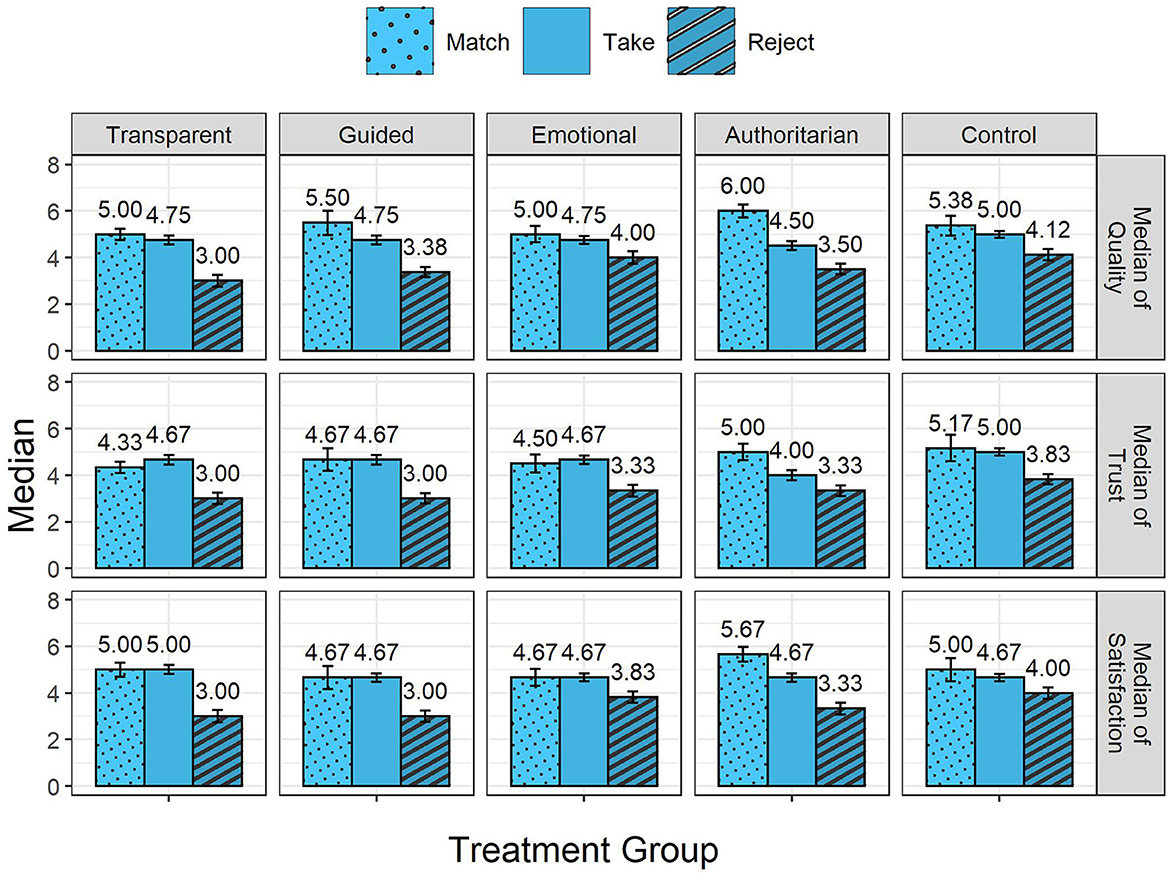
Figure 6. Median of quality, trust, and satisfaction by treatments and subgroups (error bars = standard error of the median).
Additional finding: Individuals who feel confirmed by or follow the advice have higher quality, trust, and satisfaction scores within and between treatment groups than those who reject the advice.
Finally, we perform pairwise comparisons to examine differences in quality, trust, and satisfaction between the treatment and control groups for each subgroup. The median values are displayed in Figure 6, and a set of Mann-Whitney U Test results can be found in the Supplementary material “Manipulation check and additional tables” Table Appendix 12. Remarkably, individuals who have followed the advice and are part of the “Control” group show significantly higher trust scores than the treatment groups “Guided,” “Emotional,” and “Authoritarian.” Individuals who reject the advice and belong to the “Control” group show significantly higher perceived quality, trust, and satisfaction ratings than individuals in the treatment groups. Table 7 illustrates our findings pertaining to our second open question.
5 Discussion
Using four unique explanation strategies, we examine approaches to address individual needs, accounting for non-factual aspects to enhance the comprehension of the decision-maker and user reliance in uncertain situations. Within our first hypothesis, we investigated whether the “Guided” explanation leads to more user reliance than the “Transparent” explanation within the accurate information strategies group. In our scenario, the participants in the “Guided” condition show significantly less distance from the systems' advice and less reactance than participants in the “Transparent” condition. Thus, our first hypothesis can be supported. Our research outcomes substantiate earlier findings by You et al. (2022), emphasizing that providing selective explanations, which highlight essential components, increase user reliance compared to the explanations offered by the “Transparent” group. We assume that information overload is a viable contributing factor within our specific context, potentially resulting in a diminished understanding in the “Transparent” explanation group. Our results suggest that less information than provided in the “Transparent” explanation strategy was required for understanding and following the advice. To elaborate further, the “Guided” strategy reduces the potential for an information overload because pertinent and accurate information is provided concurrently. Our approach involved customizing explanations for each individual, ensuring comprehensibility for all. We selectively used information to guide participants toward the recommended decision (Schoonderwoerd et al., 2021). While we randomly selected attributes for each participant in the “Guided” explanation strategy of the present study, a more thorough selection would be desirable in future work. This represents a noteworthy advancement toward achieving personalization in order to increase user reliance and satisfaction further, as mentioned by Shin and Park (2019). The question of determining the appropriate level of transparency remains subject to an ongoing inquiry. Our results provide a promising foundation for further investigations in this area.
With our second hypothesis, we investigated whether the “Emotional” explanation strategy leads to higher user reliance than the “Authoritarian” strategy within the human-centered explanation group. Since our scenario did not involve an emergency context, we assumed that the “Emotional” strategy should perform better. However, participants in the emotional condition do not rely significantly more on the systems' advice than participants in the “Authoritarian” condition. Thus, our second hypothesis could not be supported. We attribute this outcome to the contextual nature of the two strategies. For instance, when a human finds themselves in a scenario in which negative emotions are clearly expressed, receiving an emotional explanation is likely to be more favorably received because it helps to regulate emotions and mitigates the risk of potential cognitive distortions for the individual. Regarding our open question concerning the optimal explanation strategy, our results indicate that not every strategy yields a heightened user reliance. This result aligns with the proposition by Schemmer et al. (2022), that the absence of explanation is not in general exceeded by explanation of any kind. To be more specific, only “Guided” explanations contribute to greater user reliance. Receiving no explanation at all outperforms several types of explanations for AI decisions, i.e., “Transparent,” “Emotional,” and “Authoritarian”. This observation may explain why Schemmer et al. (2022) reported ambiguous outcomes in comparing XAI and AI. A possible explanation for the observation that the “Emotional” and “Authoritarian” explanation strategies are even less successful than the “Control” group is that the first two strategies are of a highly individualized nature. Consequently, it is plausible that individuals did not attach emotional significance to the situation because they considered the “Emotional” explanation strategy inappropriate. Similarly, individuals may have perceived the “Authoritarian” strategy as patronizing or simply incorrect. Research results by Wang et al. (2016) emphasize the importance of information quantity in a rescue scenario in which advice was provided. Especially when participants did not know how the advice was generated, its quality ratings were as negative as in a scenario in which they did not get any explanation. In our research, we intentionally chose the concise and decisive strategy denoted as “Authoritarian” to mitigate the risk of overwhelming individuals with excessive information overload. Thus, it is conceivable that in emergencies, where the need for comprehensive understanding is heightened, providing information following this strategy may result in a more favorable reception than its application in a risk-based scenario (Wang et al., 2016). We conclude that future explanation strategies should be more carefully tailored to the specific contextual requirements.
While our paper primarily focuses on user reliance as an objective measure of individual behavior, we also queried perceived quality, subjective trust, and satisfaction in our second open question. Furthermore, we conducted a subgroup analysis on three subgroups (“Match,” “Take,” and “Reject”). Individuals belonging to the “Match” subgroup, who had already selected their optimal risk level in advance, did not differ significantly regarding their self-reported trust compared to the treatment and control groups. This result is consistent with the observations made by Cheng et al. (2019) and Panigutti et al. (2022), who observed no difference in self-reported trust between explanatory and non-explanatory conditions. In contrast, Wang et al. (2016) reported significant differences in self-reported trust, while distinctions in behavior, specifically following the advice, were not statistically significant. These results endorse Lai et al. (2021) in their call for a more precise differentiation between trust and reliance. Consistent with Scharowski et al. (2022), our results show the necessity to distinguish between these two terms in XAI. Moreover, we postulate that assessing user reliance is a multifaceted construct encompassing various dimensions of human behavior, such as advice-taking, distance from advice, and reactance. Incorporating this multifacetedness into the analysis allows for the extraction of comprehensive insights and the facilitation of meaningful comparisons among different studies in the field of XAI.
Our results reveal interesting links to research in leadership styles, particularly to studies investigating advice-taking behavior within contexts characterized by asymmetric roles. Boulu-Reshef et al. (2020) analyzed advice-taking as well as “follower contribution,” a term denoting active engagement and participation in the decision-making process of “followers” within a leader-follower team. The authors compared two leadership styles: an empowering style, which grants decision-making authority to the follower, and a directive style, which focuses on achieving compliance. Most interestingly, neither of these leadership styles provides any explanations. Instead, the empowering style conveys “a willingness to share power and responsibility with the group” whereas the directive style “convey[s] a willingness to provide clear guidance and expectations to followers” (Boulu-Reshef et al., 2020, p. 4). The empowering style yielded significantly more advice-taking and follower contributions than the directive style. Thus, sharing power and responsibility appears to activate heightened engagement and a stronger commitment to a common objective. This aligns with our underlying hypothesis that the facilitation of co-constructive explanations is essential to ensure the active participation of both partners in achieving understanding and making informed decisions. These observations pave the way for future research, investigating whether additional strategies that emphasize commitment to a joint objective and grant decision-making authority and responsibility to the explainee can enhance the quality of explanation processes and results.
Furthermore, although we have not explicitly addressed the matter of under- and over-reliance in our study, we found a noteworthy trend that merits more extensive investigation: In three conditions (“Transparent,” “Emotional,” and “Authoritarian”), participants who followed the advice show higher distances from the exact value recommended by Floka. This observation indicates that under-reliance, characterized by a failure to follow the advice when it is correct, and over-reliance, marked by unconditional compliance to advice, could pose risks. Furthermore, it highlights that the mere presence of an explanation prompts users to assess the provided advice critically. This aligns with findings by Bussone et al. (2015), who show a high degree of trust in an AI system, and users are inclined to over-rely on the system's advice. In contrast, those who distrust the system tend to rely on their knowledge, even if it is not satisfactory. Moreover, research in the medical domain shows that over-reliance on AI-based advice can result in deficient decision-making, potentially leading to incorrect choices when the AI-based advice is wrong (Jacobs et al., 2021). Conversely, even when the advice is correct, it may not be embraced (Bussone et al., 2015). Bauer et al. (2023) have found a possible contributing factor to over-reliance by showing that explanations provided by an AI DSS can increase confirmation biases in comparison to an AI advice without explanations. Thereby, users are more likely to follow the advice that aligns with their beliefs. This cognitive bias can spill over into new domains, where explanations from a previous case may no longer be applicable. To mitigate the deteriorating effect of over-reliance, Jacobs et al. (2021) suggest adapting the explanation strategies in DSS to the clinician's experience with such AI systems. In our research hypothesis, we broadened our scope beyond the adaptation strategies to include the dynamic characteristics of the user. In line with Rohlfing et al. (2020), we suggest that XAI systems integrate a partner model capable of monitoring the cognitive and emotional state of the human recipient with regard to explanations. This partner model would enable the system to tailor explanations to the partner's immediate needs and cognitive capability. Our guiding strategy represents an initial step toward developing an adaptive explanation strategy. In this strategy, argument selection is guided by evaluating the user's pre-existing knowledge. While this strategy is created as a human-centered method in general, it also holds promise to counteract over-reliance as it can provide arguments for and against AI advice. In addition, in the context of the “Emotional” explanation strategy, we are working on a meta-level by enabling the human recipient of the explanation to reflect upon possible biases of their decision-making. This could function as a method to mitigate tendencies toward over-reliance. Further research is needed to develop and evaluate refined strategies to enhance understanding and user reliance while mitigating the risks of both under-reliance and over-reliance.
While this study offers valuable insights into the impact of various explanation strategies on user reliance within the field of XAI research, it is essential to acknowledge certain limitations that warrant consideration in future investigations. Firstly, the currently minimal co-construction of the explanation should be mentioned. In this study, the interaction between the participants and the social agent solely involved presenting the respective explanation strategy, limiting the opportunity for participants to pose questions. Future designs should enhance interactivity, allowing for the co-construction of explanations with respondents, thereby addressing individual concerns. Another limitation stems from the participants' exposure to a specific risk decision scenario, with explanation strategies tailored specifically to the Holt and Laury lottery. How these strategies would work in a different decision context remains for future research. Future research should explore applying the explanation strategies outlined in this paper in diverse risk situations, considering the unique personalities of participants and the contextual variables at play. Exploring the combination of several explanation strategies is also important to investigate.
6 Conclusion and outlook
We conducted an online experiment to examine four distinct explanation strategies in risk-related decision-making with the assistance of a social agent named Floka. The primary objective was to identify the explanation strategy leading to higher user reliance. In our specific context, we found that providing extensive information and presenting diverse explanations encompassing varying degrees of information content and scope are not necessarily associated with improved understanding or increased user reliance. Our results indicate that, as exemplified by the “Transparent” strategy, the potential for information overload may lead to reduced user reliance in contrast to an explanation characterized by minimal information, as evident in the “Guided” strategy group. In addition, participants did not appreciate the “Authoritarian” and “Emotional” strategies. We attribute this to the two strategies' highly individual and context-dependent nature, which might have been perceived as inappropriate. Furthermore, our results highlight a difference between self-reported trust and user reliance as behavioral indicators. Our study emphasizes the advantages of using diverse measures to quantify user reliance. The differentiated perspective on user reliance provides reinforcing or complementary rather than contradictory findings. Moreover, it demonstrates the relevance for future research, highlighting the aspect that user reliance cannot be accurately measured solely based on advice-taking.
Our results can be applied in implementing a DSS that establishes a connection between the technical component and the individual human user, considering non-factual aspects to increase understanding and user reliance. For this purpose, we introduced a “Guided” explanation strategy tailored to human users. Our observations show that the “Guided” explanation strategy outperforms alternative strategies in uncertain decision-making scenarios. Many other explanation strategies, especially the “Transparent” strategy and those rooted in human-centered strategies (“Emotional” and “Authoritarian”), perform remarkably poor compared to strategies where no explanations were provided. Future work could examine how context influences the selection of strategy, e.g., whether the optimal explanation strategy depends on the task, the explainee, or situational factors. For instance, different strategies can be helpful in different situations, e.g., “Authoritarian” strategies may be useful in emergencies where quick action must be taken. Also, one may assume that the high emotional involvement of the explainee requires different explanation strategies than the low emotional involvement. Furthermore, a combination or an extension could be beneficial, e.g., starting with the “Guided” strategy and only providing further information when necessary until all features are explained and the “Transparent” strategy has been implemented. In interactive settings, changing the explanation strategy may also depend on user feedback, e.g. questions of the explainee or non-verbal cues of non-understanding.
Data availability statement
The raw data supporting the conclusions of this article will be made available by the authors, without undue reservation.
Ethics statement
The studies involving humans were approved by the University of Paderborn Institutional Review Board. The studies were conducted in accordance with the local legislation and institutional requirements. The participants provided their written informed consent to participate in this study.
Author contributions
OL: Conceptualization, Data curation, Formal analysis, Investigation, Methodology, Visualization, Writing – original draft, Writing – review & editing. BR: Conceptualization, Methodology, Supervision, Writing – original draft, Writing – review & editing. CS: Conceptualization, Investigation, Methodology, Software, Visualization, Writing – original draft, Writing – review & editing. KT: Conceptualization, Formal analysis, Funding acquisition, Methodology, Supervision, Writing – original draft, Writing – review & editing. BW: Conceptualization, Funding acquisition, Supervision, Writing – original draft, Writing – review & editing.
Funding
The author(s) declare financial support was received for the research, authorship, and/or publication of this article. This study was supported by a grant from the German research foundation (Deutsche Forschungsgemeinschaft, DFG) TRR 318/1 2021 – 438445824, which the authors gratefully acknowledge.
Conflict of interest
The authors declare that the research was conducted in the absence of any commercial or financial relationships that could be construed as a potential conflict of interest.
Publisher's note
All claims expressed in this article are solely those of the authors and do not necessarily represent those of their affiliated organizations, or those of the publisher, the editors and the reviewers. Any product that may be evaluated in this article, or claim that may be made by its manufacturer, is not guaranteed or endorsed by the publisher.
Supplementary material
The Supplementary Material for this article can be found online at: https://www.frontiersin.org/articles/10.3389/frbhe.2024.1377075/full#supplementary-material
References
Adadi, A., and Berrada, M. (2018). Peeking inside the black-box: a survey on explainable artificial intelligence (XAI). IEEE Access 6, 52138–52160. doi: 10.1109/ACCESS.2018.2870052
Amershi, S., Weld, D., Vorvoreanu, M., Fourney, A., Nushi, B., Collisson, P., et al. (2019). “Guidelines for human-ai interaction,” in Proceedings of the 2019 CHI Conference on Human Factors in Computing Systems (New York, NY: Association for Computing Machinery), 1–13. doi: 10.1145/3290605.3300233
Anderson, L. R., and Mellor, J. M. (2009). Are risk preferences stable? Comparing an experimental measure with a validated survey-based measure. J. Risk Uncertain. 39, 137–160. doi: 10.1007/s11166-009-9075-z
Bailey, P. E., Leon, T., Ebner, N. C., Moustafa, A. A., and Weidemann, G. (2022). A meta-analysis of the weight of advice in decision-making. Curr. Psychol. 42, 24516–24541. doi: 10.1007/s12144-022-03573-2
Baniecki, H., Parzych, D., and Biecek, P. (2023). The grammar of interactive explanatory model analysis. Data Min. Knowl. Discov. 1–37. doi: 10.1007/s10618-023-00924-w
Bauer, K., von Zahn, M., and Hinz, O. (2023). Expl (ai) ned: the impact of explainable artificial intelligence on users information processing. Inform. Syst. Res. doi: 10.1287/isre.2023.1199
Bayer, S., Gimpel, H., and Markgraf, M. (2021). The role of domain expertise in trusting and following explainable AI decision support systems. J. Deci. Syst. 32, 110–138. doi: 10.1080/12460125.2021.1958505
Binns, R., Van Kleek, M., Veale, M., Lyngs, U., Zhao, J., and Shadbolt, N. (2018). “'it's reducing a human being to a percentage' perceptions of justice in algorithmic decisions,” in Proceedings of the 2018 Chi Conference on Human Factors in Computing Systems, 1–14.
Boulu-Reshef, B., Holt, C. A., Rodgers, M. S., and Thomas-Hunt, M. C. (2020). The impact of leader communication on free-riding: an incentivized experiment with empowering and directive styles. Leadersh. Q. 31, 101351. doi: 10.1016/j.leaqua.2019.101351
Bronner, S. J. (2006). Folk logic: interpretation and explanation in folkloristics. West. Folk. 65, 401–433.
Bussone, A., Stumpf, S., and O'Sullivan, D. (2015). “The role of explanations on trust and reliance in clinical decision support systems,” in 2015 International Conference on Healthcare Informatics (Dallas, TX: IEEE), 160–169.
Chazette, L., and Schneider, K. (2020). Explainability as a non-functional requirement: challenges and recommendations. Requirem. Eng. 25, 493–514. doi: 10.1007/s00766-020-00333-1
Cheng, H.-F., Wang, R., Zhang, Z., O'Connell, F., Gray, T., Harper, F. M., et al. (2019). “Explaining decision-making algorithms through UI: Strategies to help non-expert stakeholders,” in Proceedings of the 2019 CHI Conference on Human Factors in Computing Systems (New York, NY: Association for Computing Machinery), 1–12. doi: 10.1145/3290605.3300789
Chong, L., Zhang, G., Goucher-Lambert, K., Kotovsky, K., and Cagan, J. (2022). Human confidence in artificial intelligence and in themselves: the evolution and impact of confidence on adoption of ai advice. Comput. Human Behav. 127:107018. doi: 10.1016/j.chb.2021.107018
Chugunova, M., and Sele, D. (2022). We and It: An interdisciplinary review of the experimental evidence on how humans interact with machines. J. Behav. Exp. Econ. 99, 101897. doi: 10.1016/j.socec.2022.101897
Cirqueira, D., Nedbal, D., Helfert, M., and Bezbradica, M. (2020). “Scenario-based requirements elicitation for user-centric explainable AI: a case in fraud detection,” in Machine Learning and Knowledge Extraction: 4th IFIP TC 5, TC 12, WG 8.4, WG 8.9, WG 12.9 International Cross-Domain Conference, CD-MAKE 2020, Dublin, Ireland, August 25-28, 2020, Proceedings 4 (Dublin: Springer), 321–341.
Cohen, S., Ruppin, E., and Dror, G. (2005). Feature selection based on the shapley value. Proc. IJCAI. 5, 665–670.
Conte, A., Levati, M. V., and Nardi, C. (2018). Risk preferences and the role of emotions. Economica 85, 305–328. doi: 10.1111/ecca.12209
Covert, I. C., Lundberg, S., and Lee, S.-I. (2021). Explaining by removing: a unified framework for model explanation. J. Mach. Learn. Res. 22, 9477–9566.
Cramer, H., Evers, V., Ramlal, S., Van Someren, M., Rutledge, L., Stash, N., et al. (2008). The effects of transparency on trust in and acceptance of a content-based art recommender. User model. User-Adapt. Interact. 18, 455–496. doi: 10.1007/s11257-008-9051-3
de Bruijn, H., Warnier, M., and Janssen, M. (2022). The perils and pitfalls of explainable ai: Strategies for explaining algorithmic decision-making. Gov. Inf. Q. 39, 101666. doi: 10.1016/j.giq.2021.101666
Ehrenbrink, P., and Prezenski, S. (2017). “Causes of psychological reactance in human-computer interaction: a literature review and survey,” in Proceedings of the European Conference on Cognitive Ergonomics (New York, NY: Association for Computing Machinery), 137–144. doi: 10.1145/3121283.3121304
Eslami, M., Krishna Kumaran, S. R., Sandvig, C., and Karahalios, K. (2018). “Communicating algorithmic process in online behavioral advertising,” in Proceedings of the 2018 CHI Conference on Human Factors in Computing Systems (New York, NY: Association for Computing Machinery), 1–13. doi: 10.1145/3173574.3174006
Fessler, D. M., Pillsworth, E. G., and Flamson, T. J. (2004). Angry men and disgusted women: An evolutionary approach to the influence of emotions on risk taking. Organ. Behav. Hum. Decis. Process. 95, 107–123. doi: 10.1016/j.obhdp.2004.06.006
Fox, C. R., and Tannenbaum, D. (2011). The elusive search for stable risk preferences. Front. Psychol. 2, 298. doi: 10.3389/fpsyg.2011.00298
Franke, T., Attig, C., and Wessel, D. (2019). A personal resource for technology interaction: development and validation of the affinity for technology interaction (ati) scale. Int. J. Hum. Comput. Interact. 35, 456–467. doi: 10.1080/10447318.2018.1456150
Fredrickson, B. L. (2013). Positive emotions broaden and build. Adv. Exp. Social Psychol. 47, 1–53. doi: 10.1016/B978-0-12-407236-7.00001-2
Gino, F., Brooks, A. W., and Schweitzer, M. E. (2012). Anxiety, advice, and the ability to discern: feeling anxious motivates individuals to seek and use advice. J. Pers. Soc. Psychol. 102, 497. doi: 10.1037/a0026413
Grasha, A. F. (1994). A matter of style: the teacher as expert, formal authority, personal model, facilitator, and delegator. College Teach. 42, 142–149. doi: 10.1080/87567555.1994.9926845
Hegel, F., Eyssel, F., and Wrede, B. (2010). “The social robot flobi: Key concepts of industrial design,” in 19th International Symposium in Robot and Human Interactive Communication (Viareggio: IEEE), 107–112.
Holder, E., and Wang, N. (2021). Explainable artificial intelligence (XAI) interactively working with humans as a junior cyber analyst. Human-Intellig. Syst. Integrat. 3, 139–153. doi: 10.1007/s42454-020-00021-z
Holt, C. A., and Laury, S. K. (2005). Risk aversion and incentive effects: new data without order effects. Am. Econ. Rev. 95, 902–904. doi: 10.1257/0002828054201459
Hudon, A., Demazure, T., Karran, A., Léger, P.-M., and Sénécal, S. (2021). “Explainable artificial intelligence (XAI): how the visualization of ai predictions affects user cognitive load and confidence,” in Information Systems and Neuroscience: NeuroIS Retreat 2021 (Cham: Springer), 237–246.
Jacobs, M., Pradier, M. F., McCoy, T. H. Jr, Perlis, R. H., Doshi-Velez, F., and Gajos, K. Z. (2021). How machine-learning recommendations influence clinician treatment selections: the example of antidepressant selection. Transl. Psychiatry 11, 108. doi: 10.1038/s41398-021-01224-x
Jussupow, E., Benbasat, I., and Heinzl, A. (2020). Why Are We Averse Towards Algorithms? A Comprehensive Literature Review on Algorithm Aversion.
Karambayya, R., Brett, J. M., and Lytle, A. (1992). Effects of formal authority and experience on third-party roles, outcomes, and perceptions of fairness. Acad. Manage. J. 35, 426–438. doi: 10.5465/256381
Lai, V., Chen, C., Liao, Q. V., Smith-Renner, A., and Tan, C. (2021). Towards a science of human-ai decision making: a survey of empirical studies. arXiv. doi: 10.48550/arXiv.2112.11471
Laato, S., Tiainen, M., Najmul Islam, A., and Mäntymäki, M. (2022). How to explain ai systems to end users: a systematic literature review and research agenda. Int. Res. 32, 1–31. doi: 10.1108/INTR-08-2021-0600
Larasati, R., De Liddo, A., and Motta, E. (2020). “The effect of explanation styles on user's trust,” in 2020 Workshop on Explainable Smart Systems for Algorithmic Transparency in Emerging Technologies. Available online at: https://oro.open.ac.uk/70421/
Levy, A., Agrawal, M., Satyanarayan, A., and Sontag, D. (2021). “Assessing the impact of automated suggestions on decision making: domain experts mediate model errors but take less initiative,” in Proceedings of the 2021 CHI Conference on Human Factors in Computing Systems, 1-13.
Lundberg, S. M., and Lee, S.-I. (2017). A unified approach to interpreting model predictions. Adv. Neural Inf. Process. Syst. 30.
Lütkebohle, I., Hegel, F., Schulz, S., Hackel, M., Wrede, B., Wachsmuth, S., et al. (2010). “The bielefeld anthropomorphic robot head “flobi,” in 2010 IEEE International Conference on Robotics and Automation (Anchorage, AK: IEEE), 3384–3391.
Maggi, G., Dell'Aquila, E., Cucciniello, I., and Rossi, S. (2021). “Don't get distracted!”: the role of social robots' interaction style on users' cognitive performance, acceptance, and non-compliant behavior. Int. J. Social Robot. 13, 2057–2069. doi: 10.1007/s12369-020-00702-4
Mahmud, H., Islam, A. N., Ahmed, S. I., and Smolander, K. (2022). What influences algorithmic decision-making? A systematic literature review on algorithm aversion. Technol. Forecast. Soc. Change 175, 121390. doi: 10.1016/j.techfore.2021.121390
Mata, R., Frey, R., Richter, D., Schupp, J., and Hertwig, R. (2018). Risk preference: a view from psychology. J. Econ. Persp. 32, 155–172. doi: 10.1257/jep.32.2.155
Miller, T. (2019). Explanation in artificial intelligence: Insights from the social sciences. Artif. Intell. 267, 1–38. doi: 10.1016/j.artint.2018.07.007
Nayyar, M., Zoloty, Z., McFarland, C., and Wagner, A. R. (2020). “Exploring the effect of explanations during robot-guided emergency evacuation,” in Social Robotics: 12th International Conference, ICSR 2020, Golden, CO, USA, November 14-18, 2020, Proceedings 12 (Cham: Springer), 13–22.
Nunes, I., and Jannach, D. (2017). A systematic review and taxonomy of explanations in decision support and recommender systems. User Model. User-adapt. Interact. 27, 393–444. doi: 10.1007/s11257-017-9195-0
Panigutti, C., Beretta, A., Giannotti, F., and Pedreschi, D. (2022). “Understanding the impact of explanations on advice-taking: a user study for ai-based clinical decision support systems,” in Proceedings of the 2022 CHI Conference on Human Factors in Computing Systems, CHI '22, New York, NY, USA: Association for Computing Machinery. doi: 10.1145/3491102.3502104
Poursabzi-Sangdeh, F., Goldstein, D. G., Hofman, J. M., Wortman Vaughan, J. W., and Wallach, H. (2021). “Manipulating and measuring model interpretability,” in Proceedings of the 2021 CHI Conference on Human Factors in Computing Systems (New York, NY: Association for Computing Machinery), 1–52. doi: 10.1145/3411764.3445315
Ren, D., Amershi, S., Lee, B., Suh, J., and Williams, J. D. (2016). Squares: supporting interactive performance analysis for multiclass classifiers. IEEE Trans. Vis. Comput. Graph. 23(1):61–70. doi: 10.1109/TVCG.2016.2598828
Ribeiro, M. T., Singh, S., and Guestrin, C. (2016). “Why should i trust you? Explaining the predictions of any classifier,” in Proceedings of the 22nd ACM SIGKDD International Conference on Knowledge Discovery and Data Mining (New York, NY: Association for Computing Machinery), 1135–1144. doi: 10.1145/2939672.2939778
Riedl, M. O. (2019). Human-centered artificial intelligence and machine learning. Hum. Behav. Emerg. Technol. 1, 33–36. doi: 10.1002/hbe2.117
Rohlfing, K. J., Cimiano, P., Scharlau, I., Matzner, T., Buhl, H. M., Buschmeier, H., et al. (2020). Explanation as a social practice: toward a conceptual framework for the social design of ai systems. IEEE Trans. Cognit. Dev. Syst. 13, 717–728. doi: 10.1109/TCDS.2020.3044366
Rosenthal-von der Pütten, A. M., Krämer, N. C., Hoffmann, L., Sobieraj, S., and Eimler, S. C. (2013). An experimental study on emotional reactions towards a robot. Int. J. Social Robot. 5, 17–34. doi: 10.1007/s12369-012-0173-8
Sankaran, S., Zhang, C., Aarts, H., and Markopoulos, P. (2021). Exploring peoples' perception of autonomy and reactance in everyday ai interactions. Front. Psychol. 12, 713074. doi: 10.3389/fpsyg.2021.713074
Scharowski, N., Perrig, S. A., von Felten, N., and Brühlmann, F. (2022). Trust and reliance in xai-distinguishing between attitudinal and behavioral measures. arXiv. doi: 10.48550/arXiv.2203.12318
Schemmer, M., Hemmer, P., Nitsche, M., Kühl, N., and Vössing, M. (2022). “A meta-analysis of the utility of explainable artificial intelligence in human-ai decision-making,” in Proceedings of the 2022 AAAI/ACM Conference on AI, Ethics, and Society (New York, NY: Association for Computing Machinery), 617–626. doi: 10.1145/3514094.3534128
Schildberg-Hörisch, H. (2018). Are risk preferences stable? J. Econ. Persp. 32, 135–154. doi: 10.1257/jep.32.2.135
Schmidt, P., Biessmann, F., and Teubner, T. (2020). Transparency and trust in artificial intelligence systems. J. Deci. Syst. 29, 260–278. doi: 10.1080/12460125.2020.1819094
Schniter, E., Shields, T. W., and Sznycer, D. (2020). Trust in humans and robots: economically similar but emotionally different. J. Econ. Psychol. 78:102253. doi: 10.1016/j.joep.2020.102253
Schoonderwoerd, T. A., Jorritsma, W., Neerincx, M. A., and Van Den Bosch, K. (2021). Human-centered XAI: developing design patterns for explanations of clinical decision support systems. Int. J. Hum. Comput. Stud. 154, 102684. doi: 10.1016/j.ijhcs.2021.102684
Schütze, C., Lammert, O., Richter, B., Thommes, K., and Wrede, B. (2023). “Emotional debiasing explanations for decisions in HCI,” in The Proceedings of the 4th International Conference on Artificial Intelligence in HCI, an affiliated conference of HCII 2023: Human-Computer Interaction: International Conference, Proceedings (Cham: Springer International Publishing).
Sheng, H., and Chen, Y. (2020). “An empirical study on factors influencing users' psychological reactance to artificial intelligence applications,” in 2020 7th International Conference on Information Science and Control Engineering (ICISCE) (Changsha: IEEE), 234–237.
Shin, D. (2021). The effects of explainability and causability on perception, trust, and acceptance: implications for explainable AI. Int. J. Hum. Comput. Stud. 146:102551. doi: 10.1016/j.ijhcs.2020.102551
Shin, D., and Park, Y. J. (2019). Role of fairness, accountability, and transparency in algorithmic affordance. Comput. Human Behav. 98:277–284. doi: 10.1016/j.chb.2019.04.019
Slovak, P., Antle, A., Theofanopoulou, N., Daudén Roquet, C., Gross, J., and Isbister, K. (2023). Designing for emotion regulation interventions: an agenda for hci theory and research. ACM Trans. Comput. Hum. Interact. 30, 1–51. doi: 10.1145/3569898
Smetana, J. G., and Asquith, P. (1994). Adolescents' and parents' conceptions of parental authority and personal autonomy. Child Dev. 65, 1147–1162. doi: 10.1111/j.1467-8624.1994.tb00809.x
Song, Y., and Luximon, Y. (2020). Trust in ai agent: a systematic review of facial anthropomorphic trustworthiness for social robot design. Sensors 20, 5087. doi: 10.3390/s20185087
Sozialforschung (2014). SOEP 2014 – Erhebungsinstrumente 2014 (Welle 31) des Sozio-oekonomischen Panels: Personenfragebogen, Altstichproben. SOEP Survey Papers 235: Series A. Berlin: DIW/SOEP.
Speith, T. (2022). “A review of taxonomies of explainable artificial intelligence (XAI) methods,” in 2022 ACM Conference on Fairness, Accountability, and Transparency, FAccT '22 (New York, NY, USA: Association for Computing Machinery), 2239–2250.
Springer, A., and Whittaker, S. (2020). Progressive disclosure: When, why, and how do users want algorithmic transparency information? ACM Trans. Interact. Intellig. Syst. (TiiS) 10, 1–32. doi: 10.1145/3374218
Srivastava, G., Jhaveri, R. H., Bhattacharya, S., Pandya, S., Maddikunta, P. K. R., Yenduri, G., et al. (2022). Xai for cybersecurity: state of the art, challenges, open issues and future directions. arXiv. doi: 10.48550/arXiv.2206.03585
van der Waa, J., Nieuwburg, E., Cremers, A., and Neerincx, M. (2021). Evaluating xai: A comparison of rule-based and example-based explanations. Artif. Intell. 291, 103404. doi: 10.1016/j.artint.2020.103404
Wang, D., Yang, Q., Abdul, A., and Lim, B. Y. (2019). “Designing theory-driven user-centric explainable AI,” in Proceedings of the 2019 CHI Conference on Human Factors in Computing Systems (New York, NY: Association for Computing Machinery), 1–15. doi: 10.1145/3290605.3300831
Wang, N., Pynadath, D. V., and Hill, S. G. (2016). “The impact of pomdp-generated explanations on trust and performance in human-robot teams,” in Proceedings of the 2016 International Conference on Autonomous Agents & Multiagent Systems, 997–1005.
Wang, X., and Yin, M. (2021). “Are explanations helpful? A comparative study of the effects of explanations in ai-assisted decision-making,” in 26th International Conference on Intelligent User Interfaces (New York, NY: Association for Computing Machinery), 318–328. doi: 10.1145/3397481.3450650
Weitz, K., Schiller, D., Schlagowski, R., Huber, T., and André, E. (2019). “Do you trust me? Increasing user-trust by integrating virtual agents in explainable ai interaction design,” in Proceedings of the 19th ACM International Conference on Intelligent Virtual Agents (New York, NY: Association for Computing Machinery), 7–9. doi: 10.1145/3308532.3329441
Xu, J., Benbasat, I., and Cenfetelli, R. T. (2014). The nature and consequences of trade-off transparency in the context of recommendation agents. MIS Quart. 38, 379–406. doi: 10.25300/MISQ/2014/38.2.03
Keywords: human-centered XAI, human-computer interaction, empirical studies in HCI, explanation strategies, explainability, user reliance, decision-making under uncertainty, decision support system
Citation: Lammert O, Richter B, Schütze C, Thommes K and Wrede B (2024) Humans in XAI: increased reliance in decision-making under uncertainty by using explanation strategies. Front. Behav. Econ. 3:1377075. doi: 10.3389/frbhe.2024.1377075
Received: 26 January 2024; Accepted: 12 February 2024;
Published: 08 March 2024.
Edited by:
Nicola Lacetera, University of Toronto, CanadaReviewed by:
Mario Macis, Johns Hopkins University, United StatesGuglielmo Briscese, The University of Chicago, United States
Copyright © 2024 Lammert, Richter, Schütze, Thommes and Wrede. This is an open-access article distributed under the terms of the Creative Commons Attribution License (CC BY). The use, distribution or reproduction in other forums is permitted, provided the original author(s) and the copyright owner(s) are credited and that the original publication in this journal is cited, in accordance with accepted academic practice. No use, distribution or reproduction is permitted which does not comply with these terms.
*Correspondence: Olesja Lammert, olesja.lammert@uni-paderborn.de