- 1Institute of Management (IBU), Karlsruhe Institute of Technology, Karlsruhe, Germany
- 2Bain & Company Germany, Inc., Frankfurt, Germany
- 3Institute of Production Science (wbk), Karlsruhe Institute of Technology, Karlsruhe, Germany
Introduction: Human-machine interaction has raised a lot of interest in various academic disciplines, but it is still unclear how human-human interaction is affected when robots join the team. Robotics has already been integral to manufacturing since the 1970s. With the integration of AI, however, they are increasingly working alongside humans in shared spaces.
Methods: We conducted an experiment in a learning factory to investigate how a change from a human-human work context to a hybrid human-robot work context affects participants' valuation of their production output as well as their pro-sociality among each other. Learning factories are learning, teaching, and research environments in engineering university departments. These factory environments allow control over the production environment and incentives for participants.
Results: Our experiment suggests that the robot's presence increases sharing behavior among human workers, but there is no evidence that rewards earned from production are valued differently.
Discussion: We discuss the implications of this approach for future studies on human-machine interaction.
1. Introduction
Human-machine interaction is becoming increasingly relevant in production environments across industries (Graetz and Michaels, 2018; Cheng et al., 2019). Thus, the adoption and use of computer and robotic technology results in–at times drastic–changes to employees' work environments. In the context of manufacturing, automation and artificial intelligence (AI) in combination with improved sensors allow so-called cobots (collaborative robots) to work closely and safely alongside humans (International Federation of Robotics, 2018).1 Such hybrid human-robot teams are relevant in the production workforce as they allow for improved efficiency and flexibility compared to fully automated or manually operated setups. Robots can work tirelessly, but changes in product design or the workflows in a production line can still most easily be adapted to by humans (see, e.g., Simões et al., 2022, for a review on creating shared human-robot workspaces for flexible production). Beyond production, hybrid human-robot teams are relevant in the fields of medicine, service, and logistics, where they assist in surgeries, patient care, customer service, and warehouse operations (Hornecker et al., 2020; Beuss et al., 2021; Carros et al., 2022; Burtch et al., 2023; CBS News, 2023). On the one hand, this raises a lot of interest in how humans and robots will work together effectively (Corgnet et al., 2019; Haesevoets et al., 2021) and which features of the robots affect the human workers' perceptions of the robots (Terzioğlu et al., 2020). On the other hand, despite human-machine interaction being an important subject for practitioners and researchers alike, it still needs to be determined how robots in hybrid human-robot teams affect human-human interaction. This is particularly relevant in the work context because it is known to create strong social incentives (Besley and Ghatak, 2018), norms (Danilov and Sliwka, 2017), and can serve as a socialization device (Ramalingam and Rauh, 2010).
The study of human-machine interaction in work environments has garnered increasing attention in recent years (Jussupow et al., 2020; Chugunova and Sele, 2022), focusing on the role of incentives (Corgnet et al., 2019), team interaction (Corgnet et al., 2019), and shared responsibility (Kirchkamp and Strobel, 2019). However, economic research with a more specific focus on robotics is relatively scarce. We see two main reasons for this scarcity. Firstly, there is an assumption that human-machine interaction is universal in that behavioral phenomena in human-computer interaction carry over to human-robot interactions or that attitudes toward robots elicited in surveys are meaningful when it comes to actual decisions. There is little evidence for tests of this assumption. Secondly, while robotics technology has been around for decades in the industry, controlled environments for experimental research have thus far not been available to behavioral researchers. Using field-in-the-lab experiments in learning factories (Kandler et al., 2021; Ströhlein et al., 2022) offers a promising experimental paradigm for this line of research.
An important question that can be investigated in this experimental paradigm is how prosociality between human coworkers, central to productivity and efficiency in firms (Besley and Ghatak, 2018), changes after introducing robots to the workplace. With an ever-changing work environment, it is increasingly vital for individuals to be adaptable and learn new skills quickly to stay competitive and meet the changing needs of their organizations. To a considerable extent, workers can do so by sharing skills and knowledge with their coworkers. Maintaining prosocial interaction while increasing the share of robots in production environments is thus essential but also demanding for organizations. We, therefore, investigate whether robotic team members affect the prosocial behavior among their human coworkers.
Another aspect that the introduction of robots could change together with the work context is the meaningfulness of the work carried out (Cassar and Meier, 2018). If they feel that they have no impact on the eventual team output, they might perceive the resulting income to be less valuable, which could, in turn, lead to a higher willingness to share it with others (Erkal et al., 2011; Gee et al., 2017). We want to test whether we can observe a reduction in people's valuation of their produced output, depending on whether they work in a hybrid human-robot or a pure human-human team.
We report evidence from a field-in-the-lab experiment, i.e., a controlled, incentivized experiment in a lab-like environment that contains essential elements from the field (Kandler et al., 2021). This setup allows studying the effects of robotics on human-human interaction in an environment that closely parallels natural production environments–a learning factory. In our experiment, two human participants operated two production stations at the beginning and end of a three-station production line to produce electronic motor components. The middle station was either operated by two robots or by a “transfer station” that performed the same steps but with the robots switched off and hidden. For each component, the human participants received a team piece rate. In addition to that monetary payment, they could earn a chocolate bar, i.e., a material, non-monetary incentive, if they individually completed their production step at least five times. After the production round, we elicited participants' willingness to accept (WTA) for selling this material/non-monetary part of their payoff, and they engaged in a bully game (see, e.g., Krupka and Weber, 2013). The WTA for the non-monetary part of their earnings allows us to test whether rewards earned in hybrid human-robot teams are valued less than in purely human production teams, whereas the bully game allows us to measure prosocial behavior between our treatments.
We find suggestive evidence that humans in hybrid human-robot teams are more prosocial toward each other when compared to the humans in pure human-human teams. Qualitatively, participants in our sample have a lower valuation for the material, non-monetary part of their earnings when they were part of a hybrid human-robot team compared to those in a pure human-human team. Still, this difference is not statistically significant and thus not the mechanism driving the greater extent of prosocial behavior. Investigating a range of controls levied in the post-experimental survey, it seems that human workers shifted responsibility. However, rather than shifting it to the robot, they instead shifted responsibility away from the robot, allocating relatively higher responsibility to themselves and the other human participant.
There is ample evidence that joint work on tasks creates more prosociality (Allport et al., 1954; Chen and Li, 2009; Stagnaro et al., 2017; Lowe, 2021). In contrast, introducing robotics into production lines can decrease the number of work interactions between workers and reduce the feeling of working together toward a common goal (Savela et al., 2021). Organizations must consider how to integrate these technologies into their production processes optimally. Our study is a first step to inform this consideration, focusing on the changing human-human interaction in such environments. In addition to demonstrating the feasibility of running lab-like experiments with state-of-the-art production robotics in learning factories, our primary goal is to understand whether the prosocial behavior between human workers changes when a robot is in the team. Our design allows us to test whether any such change is due to a changed valuation of the income earned, either with or without the external help of a robot. As a secondary and more exploratory objective, we want to understand how the robots' team membership changes human workers' attitudes toward technology and each other.
One key advantage of our methodology is the clarity of what the treatment is. An important design choice in experiments using virtual automated agents is how these are framed. The use of different frames to refer to automated agents can be problematic as it can trigger different concepts of the “machine” that participants are interacting with. For example, the use of the term “AI” (von Schenk et al., 2022) or “algorithm” (Dietvorst et al., 2015, 2018; Klockmann et al., 2022) can lead to participants having higher expectations of the machine's capabilities when compared to the use of terms like “computer” (Kirchkamp and Strobel, 2019) or “robot” (Veiga and Vorsatz, 2010). Yet, it is technically not always clear which term to use for the programmed automated agent. This can lead to different outcomes in the experiments, as participants may interact with these agents differently, depending on the framing (see, e.g., Hertz and Wiese, 2019, for the difference between “computer” and “robot”). The different cognitive concepts induced by the differences in the terminology could partly explain why the experimental evidence on human-machine interaction is still largely mixed (Jussupow et al., 2020; Chugunova and Sele, 2022). Our methodology allows us to avoid this ambiguity, as the robots are visible, and the interaction with them is experienced beyond simply observing the outcome of their work.
Our paper broadly relates to three strands of the literature: (i) human-machine or human-computer interaction, (ii) prosocial behavior with a specific focus on fair sharing, and, as we investigate the participants' valuation of their income, (iii) deservingness and the meaningfulness of work.
Research on human-machine interaction (Fried et al., 1972, using the antiquated term “Man-Machine Interaction”) and human-computer interaction (Carlisle, 1976) dates back to the 1970s. It has since largely focused on how the interfaces for these interactions affect the users' acceptance and ease of using them (Chin et al., 1988; Hoc, 2000). Due to an ever-increasing degree of computerization, automation, and robotization, the topic has attracted cognitive psychologists (Cross and Ramsey, 2021) and economists (Corgnet et al., 2019) alike.2
Jussupow et al. (2020) and Chugunova and Sele (2022) provide excellent literature surveys on the more recent studies within the social science methodological framework. Studies that have received particular attention are those relating to the phenomena of algorithm aversion (Dietvorst et al., 2015, 2018; Dietvorst and Bharti, 2019) and algorithm appreciation (Logg et al., 2019). The aforementioned literature surveys suggest that aversion is more pronounced in moral and social domains, whereas appreciation (and lower aversion) is more likely to be found when people have some degree of control over the automated agent. Savela et al. (2021) report evidence from a vignette study suggesting that humans in mixed human-robot teams have a lower in-group identification than those in purely human teams. Similarly, in another vignette study on service failures taken care of by either humans or robots, Leo and Huh (2020) report evidence suggesting that people attribute less responsibility toward the robot than the human because people perceive robots to have less control over the task. In the context of machine-mediated communication, Hohenstein and Jung (2020) show that when communication is unsuccessful in such situations, the AI is blamed for being coercive in the communication process. Thus, it functions like a moral crumple zone, i.e., other humans in the communication process are assigned less responsibility.
Besides the mere focus of our study on human-machine interaction, we also want to investigate how the presence of robots affects the participants' prosocial behavior, in particular, sharing. A well-established economic paradigm for these behaviors is the dictator game (Güth et al., 1982; Kahneman et al., 1986; Forsythe et al., 1994).3 A participant is in the role of the dictator and can share a fixed endowment between themselves and a passive receiver. A particular variant of the dictator game is the bully game (Krupka and Weber, 2013), in which both the dictator and the receiver are equipped with an initial endowment. Beyond splitting their own endowment between themselves and the receiver, in this variant, dictators can even take parts of the receivers' endowments, allowing us also to measure spiteful behavior (Liebrand and Van Run, 1985; Kimbrough and Reiss, 2012; Ayaita and Pull, 2022).
The closest study to ours is Corgnet et al. (2019), which, among other aspects, also analyzes how prosocial motives change in hybrid teams compared to traditional human work teams. They report evidence from a computerized lab experiment in which participants need to fill out matrices with patterns of three distinct colors. They either form a team consisting of three human players or two human players and a “robot.” Each team member has one specific color they can apply to the matrix, so teams need to work together to complete the task. They find lower performance in mixed teams with a robot than in purely human teams and explain this with a lack of altruism toward the robot, leading to a lower social incentive to be productive on behalf of the team. Our design builds on this setup but instead uses the production round as a pre-treatment before the elicitation of prosocial behavior and the participant's valuation of their earned reward. The experiment in Corgnet et al. (2019) was conducted in French, where robot can either be a wild card for various types of machines (e.g., web crawler translates to robot de l'indexation) or the translation of l'ordinateur, which can also be translated to computer. Nonetheless, even in other computerized studies run in English, the term robot is frequently used in instructions (e.g., Brewer et al., 2002; Veiga and Vorsatz, 2010). Calling a computer player a “robot”–or likewise an “algorithm,” “computer,” or “automated system”–is somewhat arbitrary. Our setting uses actual production robots visible to the participants, allowing us to use the term “robot” with much less ambiguity.
Another advantage of our approach is that it is a relatively meaningful task that participants engage in. Abstracting from more complex interactions in the workplace over prolonged periods of time, this parallels the nature of actual work, which is a source of meaning (Cassar and Meier, 2018). Compared to abstract real-effort tasks, this is particularly pronounced in jobs and tasks that produce a tangible output (Ariely et al., 2008; Nikolova and Cnossen, 2020). When a robot assists humans in this meaningful production, it could reduce the relative meaning of each worker's contribution to the overall output. If workers value their income relatively less in hybrid teams, they might be more willing to share parts of it with others. A piece of evidence that supports this is provided by Gee et al. (2017), who suggest that an increase in inequality has less impact on redistribution choices when income is earned through performance than through luck. Erkal et al. (2011) investigate the relationship between relative earnings and giving in a two-stage, real-effort experiment. They provide evidence that relative earnings can influence giving behavior and that this effect can be reduced by randomly determining earnings. Again, the degree to which earnings are generated through external factors influences the degree to which participants tend to give larger parts of their endowment away. More broadly, this raises the question of whether an endowment entirely earned through performance is valued more highly, as it is more meaningful to workers when compared to an income that is (at least partially) obtained with the help of an external factor, such as luck or a robot helping to generate the income.
The remainder of this paper is structured as follows. In Section 2, we briefly explain our general field-in-the-lab approach and how it is specifically conducive to research on human-machine and human-human interaction in the presence of machines. Section 3 describes our experimental design, the main variables of interest, as well as our hypotheses. We present our results in Section 4 and discuss them together with an interesting exploratory finding in Section 5. Section 6 concludes.
2. Field-in-the-lab methodology for behavioral human-robot research
As the experiment was conducted in a non-standard environment, i.e., neither a computerized lab experiment nor an online experiment administered solely through the browser, we briefly describe the learning factory environment where we ran the experiment and the advantages this environment has for research on human behavior when collaborating in hybrid human-robotic teams. The more general approach is described in Kandler et al. (2021).
The field-in-the-lab approach is an experimental method to create real-world settings in controlled environments that mimic the field.4 Kandler et al. (2021) suggest that so-called learning factories are ideal for running such studies. They are intended to teach students about the possibilities of production setups, lean management approaches, and the capabilities of digitization technologies in realistic factory settings (Abele et al., 2015). Typically, these factories have a topical focus in the sense that a specific product in a particular industry can be produced. Still, they are also designed to be malleable in the direction of the respective training courses convened. In the case of our study, the learning factory offered a line production of up to 10 production stations with a modular setup, i.e., individual stations could be replaced, moved, or left out of the production line. This allows building a layout tailored toward anonymity–by using visual covers and placing stations for humans apart from each other–and toward the concrete research question–by cutting out three stations of the entire line for the experiment and, depending on treatment, replacing one station with robots. Combined with data recording developed in oTree (Chen et al., 2016) or other input methods, this allows a methodologically clean experimental setup. As such, learning factories allow experimental economists to observe and measure the causal impact of various factors, such as the introduction of robotics, on human-human interactions and how this affects social incentives in the workplace. In addition, they allow us to assess the entire range of more traditional economic questions, such as the impact of different types of incentives and how they affect human behavior within the context of hybrid human-robotic teams. Such analyses are typically hard to conduct with happenstance or other observational data because this data type is often unavailable and lacks a precise measure of performance measure and social interaction.
Though laboratory experiments always contain a degree of abstraction conducive to testing hypotheses clearly and unambiguously, the research on human interactions with algorithms, computers, AI, or robotics and the interaction of humans among themselves in the presence of such technologies faces a central challenge. It is unclear whether lab participants understand the same thing if words like “algorithms,” “computers,” “AI,” or “robots” are used in writing instructions. In our approach, there is no ambiguity about the concept of a robot because it is visible, and participants can experience what it does and how exactly its actions affect the team outcome. Thus, besides recreating a setup that resembles real factories and production lines more closely, focusing on the specific aspects relevant to the research question is only one advantage of the field-in-the-lab approach. These infrastructures are available in many universities across the globe (Abele et al., 2015). They offer an opportunity for interdisciplinary research into human-machine and human-human interaction in the presence of machines with industry-standard robotics while maintaining substantial experimental control. Finally, the work in the learning factory produces a tangible and potentially meaningful product.
3. Experimental design
We begin by describing the production task. Then, we introduce our two treatments and subsequently describe the stages and procedures of the experiment.
3.1. The task and the flow of production
In every session, each participant was either in the role of Worker 1 or Worker 2. Their task was to produce a component for an electronic motor (see Figure 1). Motors of this type are typically used in cars for various purposes, such as window lifters, seat adjusters, or automated boot lids.
For each production step, Worker 1 used a station with a press (from hereon Station 1) to press two clips (Figure 2A) and two magnets (Figure 2B) into one pole housing (Figure 2C). Worker 1 was equipped with a sufficient supply of clips, magnets, and pole housings at Station 1. Worker 1's production step involved placing the clips and magnets into the designated, so-called “nests” of the press, placing the pole housing on top with the opening facing down, and executing the lever of the station's press to join the individual parts.
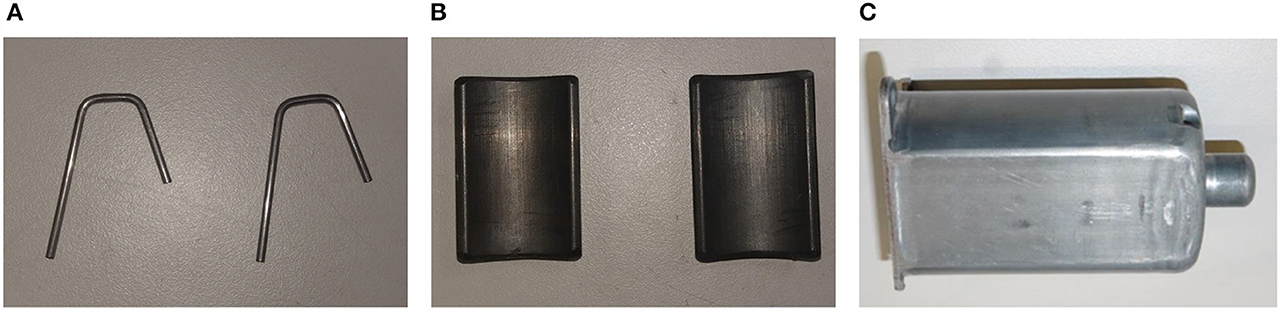
Figure 2. Production inputs for participants in the role of Worker 1 at Station 1. (A) Clips. (B) Magnets. (C) Pole housing.
After completing this production step, Worker 1 placed the resulting intermediate product onto a conveyor belt to hand it to Station TR (transfer or robot station).
At Station TR, an armature shaft with a ring magnet (Figure 3A) was placed into the prepared pole housing, and a brush holder (Figure 3B) was put on top, closing the pole housing and keeping the armature shaft in place.
Worker 2 at Station 2 took the resulting intermediate product after this step and screwed a worm gear (Figure 4A) onto the thread of the armature shaft. Subsequently, Worker 2 put the gearbox (Figure 4B) onto the pole housing and fastened it with two screws. Like Worker 1, Worker 2 was equipped with sufficient wrought parts (worm gears, gearboxes, and screws) to be able to produce throughout the production round.
From hereon, we will refer to a complete component as a final product. Once this final product was produced, Worker 2 placed it into a plastic box. The box, in turn, needed to be placed into a shelf with slides, where it was counted toward final production.5
3.2. Treatments
The production flow can be seen in Figures 5A, B. Station 2 was automated in both treatments, but it differed in the degree of automation and the visibility of the robots. For the sake of exposition, we introduce the Robot treatment first before describing the control group (NoRobot).
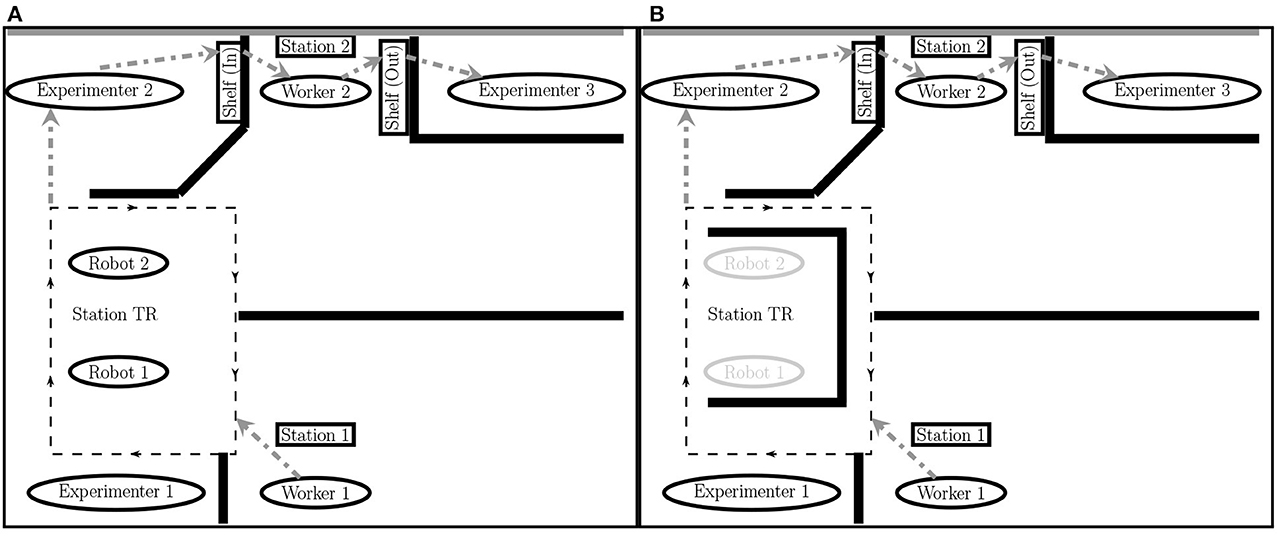
Figure 5. Setup of both treatments. (A) Robot treatment. (B) NoRobot treatment. Team members and experimenters are depicted with ellipses, stations, and shelves with rectangles. The light gray ellipses for the robots in (A) represent their switched-off state in this treatment. Station TR comprised both the robots and the conveyor belt in the Robot treatment and only the conveyor belt in the NoRobot treatment. Thick black lines represent visual covers, thick gray dashed arrows indicate the flow of production, thick gray lines represent solid walls, and the thin dashed line around Station TR depicts the conveyor belt with arrows indicating the direction of movement.
In the Robot treatment, shown in Figure 5A, Station 2 consisted of two KUKA KR 6 R900 robots (see Supplementary Figure 2 in Appendix E for a 3D model). These robots are pick-and-place robots that are equipped with light barriers as sensors for incoming intermediate products.6 Worker 1 placed each intermediate product on the conveyor belt after producing it. The conveyor belt transported the intermediate product to Robot 1. This robot placed an armature shaft (Figure 3A) with a mounted ring magnet (Figure 2B) in the intermediate product. It then automatically traveled to the next robot, which mounted the brush holder (Figure 3B) on the intermediate product. The robot then released the resulting intermediate product onto the conveyor belt, transporting it to Experimenter 2. Experimenter 2, after having it picked up from the conveyor belt, immediately placed the intermediate product into the shelf to the left of Worker 2. Processing at Station TR took 54 s for an intermediate product produced by Worker 1 before it arrived in the shelf to the left of Worker 2. Both workers could see the robots and the conveyor belt. However, they could not see each other or any of the experimenters.7
In the NoRobot treatment, shown in Figure 5B, the robots were switched off and surrounded by partition walls and thus were not visible to the participants.8 The conveyor belt operated outside these partition walls. It transported the intermediate product from Experimenter 1 to Experimenter 2. Thus, Station TR was still a (partially) automated station. Worker 1 placed each intermediate product on the conveyor belt after producing it. After Experimenter 2 picked up the intermediate product from the conveyor belt, a timer was started, and the intermediate product was processed by Experimenter 2 for Worker 2. When the timer showed 28 s, Experimenter 2 placed the intermediate product into the shelf to the left of Worker 2.9 Added to the time of the conveyor belt (26 s), this was the time the robots in the Robot treatment needed for their production step, which led to the same time gap between the completion of a work step of the participant at Station 1 and the provision of an intermediate product to the participant at Station 2.10
Due to the time that elapsed between the first part produced by Worker 1 and the first intermediate product that arrived at Worker 2, Worker 2 started their 10 min with a 90-s offset to Worker 1.
3.3. Stages
The experiment comprised four stages: Stage 1 consisted of instructions and a practice round, Stage 2 was the 10-min production round, Stage 3 consisted of two decision tasks, and Stage 4 was the post-experimental survey. In the following, we describe each stage in more detail.
In Stage 1, participants were brought to their stations and received general instructions about the experiment and specific instructions concerning the production step and their stations on a tablet computer. They also received instructions on how to handle their station.11 These instructions used GIF animation pictures.12 Subsequently, participants completed a practice round in which they had 3 min to produce a maximum of two intermediate products (Worker 1) or final products (Worker 2) with their station. Worker 2 was provided with two pre-mounted intermediate products to do so. Throughout the practice round, all instructions regarding the respective station and production steps were visible on the practice round screen. There were no incentives in this stage. After this practice round, participants had to answer a series of control questions before entering the production round, in which they carried out their production steps for the rewards.
Stage 2 consisted of the 10-min production round. We described the task and flow of production in the previous subsection. After 10 min, the production round ended, and Stage 2 concluded. Each participant received 65 ECU (corresponding to €0.65 at the end of the experiment) for each final product produced by their team and a chocolate bar if they individually produced more than five intermediate products (Worker 1) or final products (Worker 2). Thus, both workers earned the same monetary reward from the production round but earned the chocolate bar individually. We did so to avoid a single slow worker resulting in losing both observations for the ensuing Becker-deGroot-Marschak Mechanism (Becker et al., 1964, from hereon BDM).
For Stage 3, participants were brought to a table to allow the experimenters to clean and set up the stations for the next participants. In this stage, participants had to complete three decision tasks.
For the first decision task, the material part of their payment (the chocolate bar) was placed on their table. We used a 100 g milk chocolate bar from a popular brand that cost around € 1 at the time of the sessions.13 Subsequently, we elicited the participants' willingness to accept (WTA) for selling this item back to the experimenter using the BDM. Participants were asked to state a price r in the range of 0 to 200 ECU at which they would be willing to sell the chocolate bar. A random draw p from a uniform distribution between 0 and 200 ECU determined a price. If the participant's reservation price was lower than that draw (r < p), the chocolate bar remained at the table at the end of the experiment, and the participant received the price p randomly drawn by the computer. If the participant's reservation price was higher than or equal to that draw (r≥p), the participant would keep the chocolate bar at the end of the experiment and would not receive any additional ECU from this decision-task.14
In the second decision task, participants decided whether to give a part of their monetary payment to their human team member or to take some of their team member's monetary payment away. This is the bully variant of the dictator game, as described in Krupka and Weber (2013). Remember that both workers earned the same amount of ECU in the production round within teams. Yet, across production teams, the accumulated amounts of ECU differed as they produced different numbers of final products. Thus, this decision was programmed to be relative to the earned endowment from the production round to make it salient one more time that they worked toward a common goal in the preceding production round. The highest amount a participant could take away from their human team member was 50% of the earned endowment. The highest amount they could give to their team member was 100% of their earned income from the production round.15 They could choose any integer value between (and including) those ECU boundaries.16 At the end of the experiment, one worker's decision was randomly chosen with equal probability to be implemented for payoffs.
In the third and final decision task, we elicited social value orientation with the incentivized six-slider task described in Murphy et al. (2011). At the end of the experiment, one of the workers was chosen randomly with equal probabilities, and one of this worker's six sliders was randomly chosen with equal probabilities to be payoff-relevant.17
Stage 4 comprised a survey containing questions on context-related attitudes, the allocation of responsibility for the team output, conventional economic attitudes and preferences, and demographics. Participants received 250 ECU for this stage, irrespective of their responses.18
3.4. Procedures
The sessions were run at the Learning Factory for Global Production at the Institute of Production Science (wbk) at the Karlsruhe Institute of Technology (KIT). The experiment was conducted in German, where the word Roboter has very similar connotations to the English word robot. The exchange rate was 1ECU = €0.01. The average session lasted 41 min, and participants earned €13.03 on average (including the flat payment of €2.50 for the survey and the selling price in the BDM if participants sold), and the chocolate bar for producing more than five units in case participants did not sell it in the BDM.19 Participants from the KD2Lab Pool (KD2Lab, 2023) were recruited via hroot (Bock et al., 2014), and the experimental software was programmed using oTree (Chen et al., 2016). Due to the availability of only one experimental production line, i.e., one station for Workers 1 and 2, respectively, and only one station with KUKA Robots, we ran 24 sessions with two human participants for each treatment. This results in a total sample of 48 participants. Throughout the experiment, participants had a table bell they could ring in case they needed assistance or wanted to ask clarifying questions. Upon arrival, participants were immediately led to separate tables (spatially separated and surrounded by visual covers), and eventually, they exited the learning factory through different exits. Thus, our setup did not allow for interaction between workers before, during, or immediately after the experiment other than through the tasks described above.
3.5. Hypotheses
As argued above, evidence suggests that the change from purely human to hybrid human-machine teams can influence the social context of human interaction (Corgnet et al., 2019; Savela et al., 2021). Participants in our experiment are not colleagues for a prolonged amount of time. Thus, in line with previous research (Allport et al., 1954; Chen and Li, 2009; Stagnaro et al., 2017; Lowe, 2021), we implemented a production round where they had to work toward a common goal and made team performance salient. In this production round, we administered our treatment. From the perspective of any one of the workers, this also changes the salience of their coworker's human identity. With the robot in the (relative) out-group, the robots' presence in the team could strengthen the human team members' in-group identity (Akerlof and Kranton, 2000; Abbink and Harris, 2019), leading to increased prosociality between the human workers.20 Hypothesis 1. The robots' presence in a production line increases the share transferred in the bully game.21
Our literature discussion also suggests that when external factors are involved in attaining income, people change their willingness to share with others (Erkal et al., 2011; Gee et al., 2017). Thus, individuals may be more likely to share an income generated with the help of robots in a task. Since individuals do not feel as personally responsible for income generated through such external factors, one reason might be that they do not value it as highly and thus are more willing to share it with others. Our second research question is thus whether a worker's valuation for their production output changes depending on the team context. Work is a source of meaning (Cassar and Meier, 2018). Compared to abstract real-effort tasks, this is particularly pronounced in jobs and tasks that produce a tangible output (Ariely et al., 2008; Nikolova and Cnossen, 2020). We hypothesize that the robot in the team saliently diminishes the relative meaning of each worker's production step to the overall output. Therefore, we implemented a non-monetary part of income that could be earned in the production round to measure a change in participants' income valuation between treatments by eliciting their WTA for this part of their payoff. Hypothesis 2. The robots' presence in a production line reduces the WTA for the individually earned payoff.22
The experimental design and the hypotheses were preregistered at aspredicted.org.23
We are aware that beyond the valuation of the income earned, other factors, e.g., attitudes toward technology, experience with the production environment, and demographic factors, may play a role in the prosocial behavior, and thus leave the investigation of the controls we collected for exploratory investigation in the discussion of potential further mechanisms.
3.6. Main variables of interest and estimation strategy
Our primary interest is in the behavior in the bully game. We define the variable Share transferred that ranges from −50 to 100% for the amount transferred between the two workers according to the decision of each participant.24 That is, if a participant decided to take a part of the earnings from the other participant in the team, Share transferred would be negative. In contrast, it is positive if a participant decided to give a part of their own earnings to the other participant in the team. To investigate behavior at the extensive margin, we also create the variable Share categorical that is equal to 3 if Share transferred is strictly positive, equal to 2 if Share transferred is equal to zero, and equal to 1 if Share transferred is strictly negative. The variable Robot is our treatment dummy. It equals one if the participant was in the robot treatment and zero otherwise. We keep track of Individual production, the number of completed production steps by a participant in either role, and Team production, which is the number of final products produced by the production team.25 Worker 2 is a dummy equal to one if the participant was in the role of Worker 2 in the production line and zero otherwise, i.e., if the participant was in the role of Worker 1. Production in trial round is the number of work steps completed in the trial round. This number can only range from zero to two as this was the maximum number of intermediate products (Worker 1) or final products (Worker 2) participants could produce in the 3-min trial round.26
Our empirical strategy is as follows. For each hypothesis, we first report a two-sided Mann–Whitney U-test to compare the two treatments. We use the variable Share transferred for our first hypothesis on how the robot affects prosocial behavior and WTA for the second hypothesis on how the robot being in the team affects the valuation of the non-monetary part of the payoff.
We estimate regression specifications to control for demographics and the above survey measures. First, we regress the dependent variable on only the treatment dummy to show the pure treatment effect. We then add demographics in a second specification. In specification three, we control for Team production since the percentage point differences between treatments translate into different absolute amounts transferred, and the budget for this task depends on the amount earned. We also add Worker 2 to account for differences between the two roles and Production in trial round to account for differences in observed ability from the production round. In the fourth specification, we add all survey items related to attitudes applying directly to the environment in the production round. In the fifth and last specification, we add more general attitudes. We will refer to this specification as the saturated specification and base our discussions on findings mainly on this specification.
Unless mentioned otherwise, our results are robust to using the absolute number of shared ECUs. We can compare the participants' behavior with Share transferred. This way, means and coefficients can be interpreted as percentage points. We provide robustness checks using the absolute amounts in Appendix A. Also, we use heteroskedasticity-robust sandwich estimators (Eicker, 1967; Huber, 1967; White, 1980) in all regressions in the main text, but the results using cluster sandwich estimators (Rogers, 1993) on the team level can be found in Appendix C.
4. Results
Hypothesis 1 was that the presence of robots in a production line does not influence the share transferred in the bully game played with the earned endowment from the production round. Figure 6A reveals that we cannot reject this hypothesis without controlling for participant and team characteristics (p = 0.282, Mann–Whitney U-test).27 Qualitatively, contributions in the Robot treatment were 10.597% points higher than in the NoRobot treatment, translating into a difference of 100.542 ECU in absolute earnings.
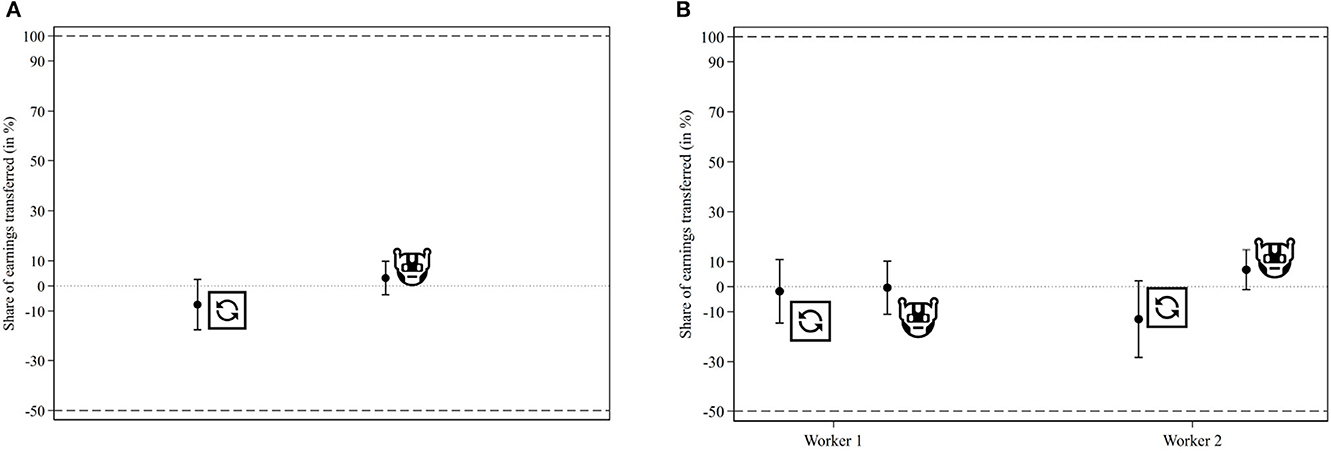
Figure 6. Share transferred in the bully game. (A) Across treatments. (B) Across treatments and roles. Dots represent means. Whiskers represent 95% confidence intervals. Dashed lines indicate the boundaries of admissible choices. The dotted line provides a visual reference at zero.
When considering behavior by roles in the production team in Figure 6B, we see that most of that aggregate difference was driven by the participants in the role of Worker 2. Here, the difference in the shares transferred is only 1.424% points for Worker 1 (p = 0.718, Mann–Whitney U-test), whereas it is 19.771% points for Worker 2 (p = 0.109, Mann–Whitney U-test).
When controlling for the covariates described in our empirical strategy, we see in Table 1 that the coefficient on our treatment dummy is positive, irrespective of our specification. In our last and preferred specification, participants transferred an 11.102% points higher amount to the other participant in the Robot compared to the NoRobot treatment.28
The distribution of shares transferred reveals that about a third of the participants did not transfer any earnings from or to the other participant in their team. In the NoRobot treatment, 37.50% of the participants took a part of the earnings from the other participant. As 29.17% of the participants in this treatment gave parts of their earnings to the other participant, the remaining 33.33% of participants in the NoRobot treatment chose neither to take a part of the earnings from the other participant nor to give parts of their earnings to the other participant (in other words, their Amount transferred or Share transferred was zero). In the Robot treatment, 25.00% of the participants took earnings from the other participants, whereas 41.67% gave parts of their earnings to the other participant. This leaves 33.33% of participants in the Robot treatment who chose an Amount transferred or Share transferred of zero. We checked whether the higher shares of participants choosing a strictly positive or negative transfer are driven by our treatment.29
Table 2 reports the results from ordered probit regressions on whether the Share transferred was strictly positive, equal to zero, or strictly negative. Though the treatment coefficient is not significant in all specifications, it is marginally significant in specifications (4) and (5). Thus, the treatment effect is partially due to differences in the decision on whether to transfer at all and in which direction and only partially due to the decision on how much to transfer. Result 1. We find suggestive evidence that participants behave more prosocially in the Robot than in the NoRobot treatment. In our data, this effect is partially driven by the extensive margin (whether they transfer any nonzero amount or not and in which direction).
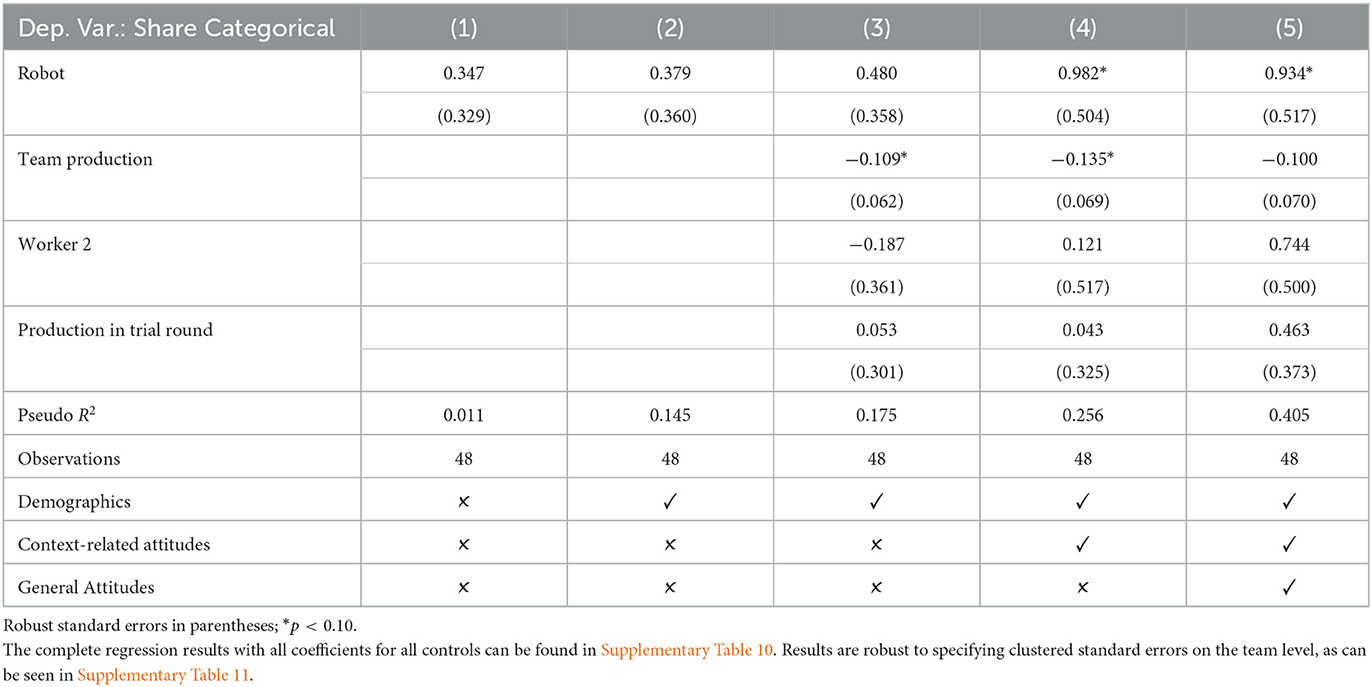
Table 2. Ordered Probit regressions on whether Share transferred was strictly positive (3), equal to zero (2), or strictly negative (1).
Hypothesis 2 relates to one potential mechanism to explain this finding. Participants in the Robot treatment potentially valued the earnings generated from the production round less than those in the NoRobot treatment because these earnings were generated with the robots' assistance and not solely through their own work. The absence of such an effect would, in turn, indicate that being in a mixed human-robot team does not affect the workers' perceived value of the individually earned reward. Looking at Figure 7A, we see that participants in the Robot treatment stated a 19.167 ECU (16.04%) lower WTA for the chocolate bar than participants in the NoRobot treatment. Still, this difference is not statistically significant (p = 0.298, Mann-Whitney U test).30
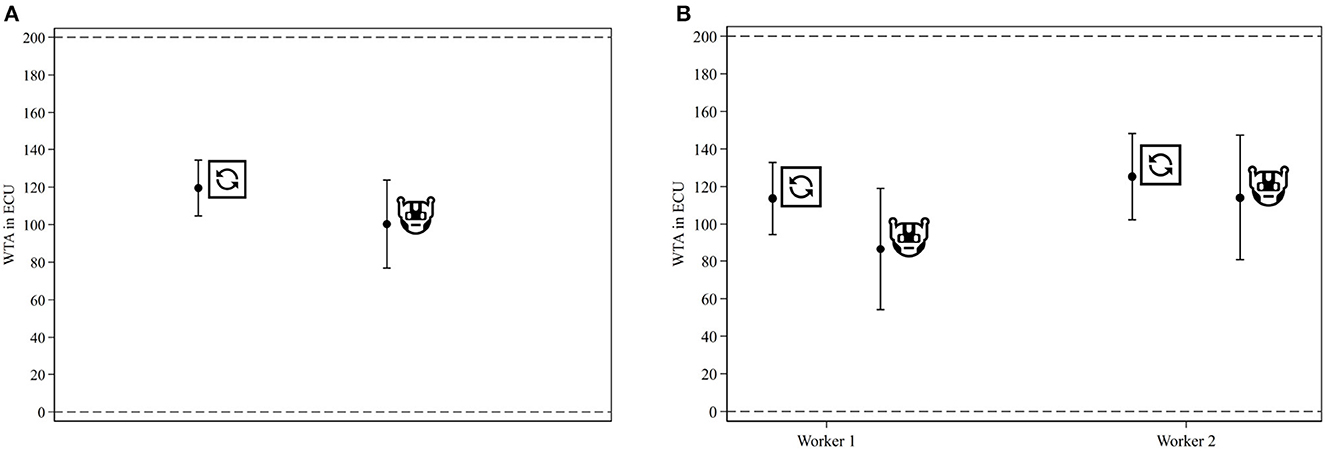
Figure 7. WTA for the chocolate bar. (A) Across treatments. (B) Across treatments and roles. Dots represent means. Whiskers represent 95% confidence intervals. Dashed lines indicate the boundaries of admissible choices.
The direction of the difference is the same for Worker 1 (Δ = 27.083 ECU or 23.83%, p = 0.384, Mann Whitney U test) and Worker 2 (Δ = 11.250 ECU or 8.98%, p = 0.743, Mann Whitney U test), which can also be seen from Figure 7B.
In line with what can be seen from the figure, the regression results reported in Table 3 corroborate that there is no treatment difference in the WTA for the non-monetary part of earnings. Yet, the coefficient is negative in all specifications, ranging between 14.584 ECU and 24.703 ECU.
Result 2. We do not find evidence for a difference in the WTA for the non-monetary part of earnings from the production round.
This concludes our investigation into our preregistered hypotheses.
5. Discussion
We found mild evidence for more prosocial behavior in the Robot treatment compared to the NoRobot treatment. We hypothesized that a lower valuation for the earned income could have led to this result. While the WTA for the chocolate bar was, on average, lower in the Robot treatment, that treatment difference was not statistically significant. Given the low number of observations, we cannot interpret this as evidence for a null result. In the following, we discuss this limitation together with other shortcomings of our design and present one interesting finding from our sample that could be interesting for future research.31
5.1. Limitations of the experiment
We report results from a relatively small sample. This sample size was chosen due to the intensive data elicitation process that required a significant number of experimenters, labor hours of assistants, and their focus when counting components. Thus, to ensure the experiment could be adequately controlled and data collection went smoothly, we opted for a straightforward design with only two treatments and, thus, a smaller sample. Therefore, our experiment is a good starting point for investigating human-human interaction in the presence of physical and visible robots in a natural manufacturing context. This opens the potential to, among other things, investigate whether algorithm aversion (Dietvorst et al., 2015, 2018) or appreciation (Logg et al., 2019) carry over to physical robots or whether our results on the allocation of responsibility are robust to the provision of incentives for shifting responsibility to the robots.
Given this small sample size, however, any effects would need to be rather large to be picked up by statistical tests. Our experiment employed a comparably light treatment difference from an economist's perspective. We kept monetary and non-monetary incentives identical across treatments, and, whereas our treatment was administered in the production round, we measured treatment differences in the subsequent stage that, in itself, did not differ across treatments. Yet, the post hoc statistical power for the tests of our two hypotheses is arguably too low to make our results conclusive.32 In combination with correcting for multiple comparisons (List et al., 2019) and the resulting statistical implications for the results of this paper, our experiment should be seen as a starting point, demonstrating that economic experiments in ecologically valid but yet fairly controlled environments, namely learning factories, are feasible.
Participants received the chocolate bar if their individual performance crossed a threshold. We implemented it this way to allow for sampling Worker 1s even if Worker 2 was too slow to cross that threshold. Yet, this performance is actually independent of the robots' productivity, at least for Worker 1. This might explain why we see a slightly higher WTA for Worker 2 in Table 3, even though only statistically significant in specification (4), which is not the fully saturated specification. On the other hand, Figure 7A instead seems to suggest that any potential treatment effect would be lower for Worker 2 than Worker 1, leading us to believe that the same elicitation based on a threshold for the group performance would not have lead to a greater difference than the one we reported.
Our participant sample consisted predominantly of students with some connection to engineering and manufacturing subjects or STEM fields. As such, they are more exposed to robotics and artificial intelligence and are likely to be keener to use technology. More generally, students are relatively young and interact more regularly with new digital technologies in their private lives than other strata of society. Even though our participant pool is constant across treatments, this would be problematic if it affected how strongly participants perceive the Robot treatment to differ from the NoRobot treatment. In fact, it seems plausible that we would observe larger effects on people outside the context of a technical university for whom production robots would be novel and unusual.33
We cannot discuss generalizable claims from our small sample, but we can discuss how hopeful one can be to obtain generalizable results in future studies with larger samples and potentially in other countries. In this study, we used a German sample, i.e., we ran our experiment in a country with a relatively high share of GDP attributed to the secondary or manufacturing sector. Bartneck et al. (2005) though show that there are no large differences in robotic attitudes to other industrialized countries, e.g., the US, Japan, or the Netherlands, when it comes to interaction with a robot. We see this as an indication that researchers can more broadly gain valuable insights from using learning factories for studies on human-robot interaction and human-human interaction in robot-augmented setups.
Finally, our setup did not allow for a trade-off between quality and quantity. The production task for both Worker 1 and Worker 2 was very simple, and bad quality and non-completion (i.e., simply handing the raw/input materials to the shelves) was almost indistinguishable. As such, we only counted pieces of “good” quality because incomplete intermediate products could not be processed any further. However, as the production steps were so simple, we essentially never observed a product of “bad” quality.
5.2. The allocation of responsibility in hybrid human-robot teams
We asked participants to state who or what was responsible for team production not being greater than it actually was. The bully game decision might have resulted from responsibility being shifted away from one participant to the other participant, the robot, or the transfer station, respectively. Thus, a shift in how workers allocate responsibility between themselves and the robots (Kirchkamp and Strobel, 2019) or even blame-shifting (Bartling and Fischbacher, 2012; Oexl and Grossman, 2013) could be a mechanism explaining the increased amounts transferred in the Robot treatment.34 Overall, between a participant and the respective human coworker, there was no pronounced difference in the allocation of responsibility between the two treatments (p = 0.439, Mann-Whitney U test). This still holds when we consider the two roles separately (p = 0.939 for Worker 1 and p = 0.262 for Worker 2, Mann-Whitney U tests). This can also be seen in Figure 8.
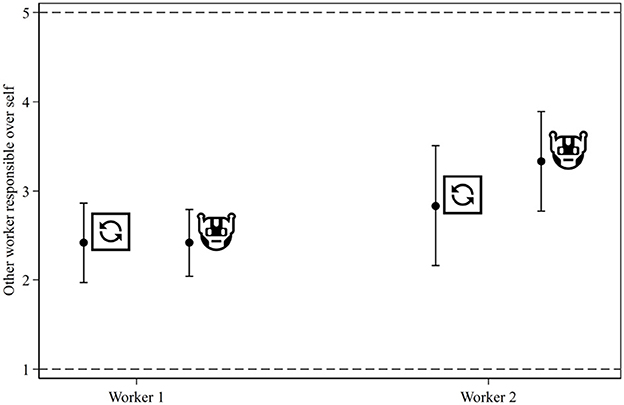
Figure 8. Degree to which participants allocated responsibility to the other participant (high value) as compared to themselves (low value) for not having produced more components. Dots represent means. Whiskers represent 95% confidence intervals. Dashed lines indicate the boundaries of admissible choices.
When we consider how responsibility was divided between a participant and the transfer station (NoRobot treatment) or the robot (Robot treatment) in Figure 9, we see that Station TR was allocated less responsibility in the Robot treatment than in the NoRobot treatment. This is in line with Leo and Huh (2020), who also found that humans allocate more responsibility (or as the authors call it, “blame”) for a mistake or bad outcome to themselves than to robots. This difference is not statistically significant overall (p = 0.101, Mann-Whitney U test). Still, looking at the two roles separately, we find a marginally statistically significant treatment difference only for Worker 1 (p = 0.072 for Worker 1 and p = 0.434 for Worker 2, Mann-Whitney U tests). This might have been driven by the fact that Worker 1s could watch the robot throughout Stage 2 in their peripheral view. In contrast, Worker 2 would only see the robot when turning to get another intermediate product for their production step.
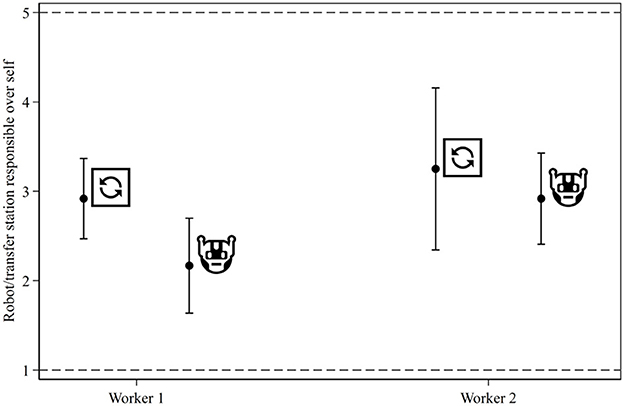
Figure 9. Degree to which participants allocated responsibility to the transfer station/robot (high value) compared to themselves (low value) for not having produced more components. Dots represent means. Whiskers represent 95% confidence intervals. Dashed lines indicate the boundaries of admissible choices.
Consider Figure 10. When we asked participants how they would divide responsibility between the transfer station (NoRobot treatment) or the robot (Robot treatment) on the one side and the human coworker on the other, we found that the participants allocated more responsibility to the other participant than the robot.
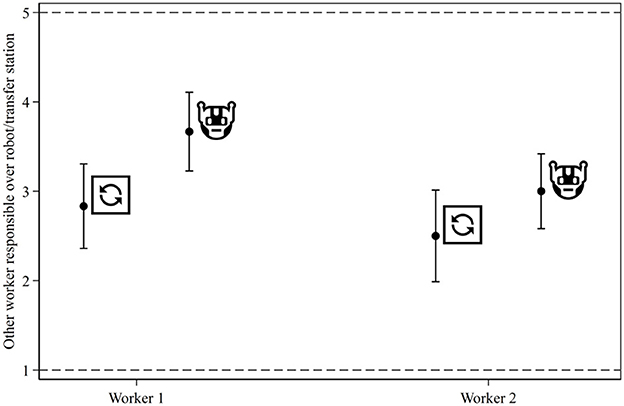
Figure 10. Degree to which participants allocated responsibility to the transfer station/robot (high value) compared to the other participant (low value) for not having produced more components. Dots represent means. Whiskers represent 95% confidence intervals. Dashed lines indicate the boundaries of admissible choices.
This difference is statistically significant (p = 0.014, Mann-Whitney U test). Looking at the two roles separately, we also find this statistically significant treatment difference for Worker 1 but not Worker 2 (p = 0.034 for Worker 1 and p = 0.168 for Worker 2, Mann-Whitney U tests). As with the previous response to the allocation of responsibility, this might be due to different visual exposure to the robot between the roles.
Note that these variables were included in the Context-related attitudes in our regression specifications where we saw the largest effect both in size and statistical significance of the treatment coefficient (see Tables 1, 2).
6. Conclusion
We report evidence from a field-in-the-lab experiment in which we varied the team composition from a human-human team to a hybrid human-robot team. We find suggestive evidence that the robots in our experiment changed the social context of the work interaction, leading to more prosocial behavior among the human workers in the bully game. We find no statistically significant evidence that the valuation for earned income differs between our treatments. Our data suggests that the participants blamed themselves and the other participant in their team more than the robot for not having produced more in the production round. This has important implications for future research into the diffusion of responsibility in hybrid human-robot teams. The fact that they do not use the robots as scapegoats for productivity issues shows a relatively high acceptance of robots in the task. The negative reading is that people might rely too strongly on the robots' performance and overly search for responsibility in their human coworkers. This could create tensions in the long run. Future studies, investigating how social pressure during the production round and prolonged and more complex interaction in hybrid teams affect these behaviors, potentially in settings in which workers need to make more autonomous decisions during production, could answer these questions.
Beyond the study of social interactions between humans and income valuation in hybrid human-robot teams, our field-in-the-lab approach (Kandler et al., 2021) offers a promising methodology for studying various aspects of human-machine interaction in work environments, including issues related to “robotic aversion,” a more direct investigation of the allocation of responsibility in hybrid human-robot teams, and the optimal design of hybrid-team work environments. A key advantage of this approach is its ability to replicate real-world conditions and processes in a controlled laboratory setting. It allows researchers to manipulate variables of interest and measure the impact on human behavior and performance. For example, when studying “robotic aversion,” researchers could manipulate a robotic co-worker's autonomy level and measure the impact on human attitudes and behavior toward the robot. Another advantage of the field-in-the-lab approach is its ability to capture dynamic interactions between humans and machines over time. By running experiments over multiple rounds or sessions, researchers can track how attitudes and behaviors evolve as individuals become more familiar with their robotic co-workers. This is particularly relevant for studying issues related to shared responsibility and blame-shifting, as these behaviors may change as humans become more accustomed to working with robots.
Data availability statement
The raw data supporting the conclusions of this article will be made available by the authors, without undue reservation.
Ethics statement
Ethical approval was not required for the studies involving humans because ethical approval was not generally required as long as personal data could not be used to identify individuals, which is not the case for our anonymized study. The studies were conducted in accordance with the local legislation and institutional requirements. The participants provided their written informed consent to participate in this study.
Author contributions
PG, BR, and LS designed the experiment, wrote the instructions, and ran the experimental sessions. PG and BR programmed the experimental code and ran the statistical analysis. LS prepared the setup in the learning factory. All authors were involved in the manuscript preparation, contributed to its revision, and read and approved the submitted version.
Funding
PG and LS gratefully acknowledge funding from the IFREE Small Grants Program. All authors acknowledge support from the KIT-Publication Fund of the Karlsruhe Institute of Technology.
Acknowledgments
All authors would like to thank Agnes Bäker, Petra Nieken, Christina Strobel, Karoline Ströhlein, Sven Walther, and the participants of the HeiKaMaXy workshop in March 2023 at Heidelberg University for helpful comments and Nimet Bozkurt, Patrizia Dück, Baptiste Obry, Sergiu Panainte, Julia Schreier, and Rebecca Zimmer for their excellent research assistance.
Conflict of interest
BR is employed by Bain & Company Germany, Inc., Germany. His employment has begun after data elicitation.
The remaining authors declare that the research was conducted in the absence of any commercial or financial relationships that could be construed as a potential conflict of interest.
The author PG declared that they were an editorial board member of Frontiers, at the time of submission. This had no impact on the peer review process and the final decision.
Publisher's note
All claims expressed in this article are solely those of the authors and do not necessarily represent those of their affiliated organizations, or those of the publisher, the editors and the reviewers. Any product that may be evaluated in this article, or claim that may be made by its manufacturer, is not guaranteed or endorsed by the publisher.
Supplementary material
The Supplementary Material for this article can be found online at: https://www.frontiersin.org/articles/10.3389/frbhe.2023.1220563/full#supplementary-material
Footnotes
1. ^Specifically, the International Federation of Robotics (2018) defines collaborative robots in the following way: “The International Federation of Robotics defines two types of robot designed for collaborative use. One group covers robots designed for collaborative use that comply with the International Organization for Standards norm 10218-1 which specifies requirements and guidelines for the inherent safe design, protective measures and information for use of industrial robots. The other group covers robots designed for collaborative use that do not satisfy the requirements of ISO 10218-1. This does not imply that these robots are unsafe. They may follow different safety standards, for example national or in-house standards”.
2. ^See March (2021) for a review of experiments using computer players.
3. ^See Engel (2011) or Cochard et al. (2021) for extensive meta-studies.
4. ^Note that this is different from the lab-in-the-field approach (Gneezy and Imas, 2017) or artifactual field experiments (Harrison and List, 2004), which refer to experiments that are lab-like but use a non-standard subject pool. In contrast, field-in-the-lab experiments (Kandler et al., 2021) use standard subject pools in malleable field-like environments like learning factories.
5. ^Note that for both workers, it was hardly possible to hand in intermediate or final products in a bad quality. Bad quality and non-completion (i.e., simply handing the raw/input materials into the shelves) were almost indistinguishable. As such, we only counted pieces of good quality as incomplete intermediate products could not be processed any further. This essentially never happened in the production round.
6. ^Note that these robots, though resembling a human arm, have not been further anthropomorphized, which is known to improve the human perception of robots (Terzioğlu et al., 2020).
7. ^Due to the setup in the learning factory, we had to implement the layout such that Worker 1 had the robots in their peripheral view throughout, whereas Worker 2 would only see them if they turned, e.g., for picking up a part from the shelf to their left.
8. ^Put differently, we did not deceive participants by using the robots in both treatments at Station TR.
9. ^The raw time of the conveyor belt to transport the intermediate product from experimenter 1 to experimenter 2 is 26 s, thus adding to 54 s with experimenter 2's timer.
10. ^Note that in the NoRobot treatment, we told participants in the role of Worker 1 that we would provide Worker 2 with an intermediate product that is equipped with an armature shaft and a brush holder 54 s after they turn in their intermediate product. We neither told them that this was done by Experimenter 2 nor did we claim that it is the identical intermediate product. This allowed us to also use pre-mounted intermediate products for Worker 2 in this treatment.
11. ^Participants also received a brief description of what the other participant was doing and that the other participant would receive the same incentives.
12. ^The static photos used in the figures of this article are identical to the ones used in the instructions.
13. ^A picture can be found in Appendix B, together with the instructions.
14. ^Additional to instructions on the mechanism, participants could also gain an intuition with a virtual tool on the preceding instructions page. They could enter a fictional WTA, and a fictitious price would be randomly drawn from between 0 and 200 ECU. Subsequently, the resulting outcome was described. Participants could do this as often as they liked.
15. ^Given that we used the team production as stakes, this guaranteed that no participant could earn a negative payoff from this task.
16. ^Similar to the BDM, participants could familiarize themselves with that decision and try different potential amounts. A slider was displayed with the actually possible amounts as the endpoints. For any amount chosen, the consequences of that choice for both participants were shown, assuming that the participant was randomly assigned the role of the dictator. Participants could only make their actual decision after having chosen to leave this page.
17. ^All random draws during the experiment were independent of each other.
18. ^The experimental instructions for all stages can be found in Appendix B.
19. ^No participant failed to cross the threshold of five intermediate products (Worker 1) or final products (Worker 2). This was intentional to obtain sufficient data for our analysis. The experimental software would have skipped the BDM task in that case.
20. ^The presence of machine players in economic paradigms is also known to result in more rational behavior (March, 2021). In our context, that would mean higher amounts taken in the bully game. Yet, the literature reviewed focuses mainly on how humans act toward the machine players and not the human players.
21. ^The hypothesis in our preregistration was formulated in terms of the null hypothesis: “The presence of robots in a production line does not influence the share transferred in the bully game”.
22. ^The hypothesis in our preregistration was formulated in terms of the null hypothesis: “The presence of robots in a production line does not influence the WTA for the individually earned endowment”.
23. ^The preregistration number is #87128, and the document can be found at https://aspredicted.org/NVY_4CZ.
24. ^Since teams have produced different amounts and thus earned different budgets for the bully game, we stated our hypothesis regarding the share that participants transferred rather than absolute amounts. The Amount transferred ranged from −650 ECU to 325 ECU, and we report regressions using this variable as the dependent variable in Supplementary Table 8 in Appendix A as a robustness check.
25. ^Note that Individual production and Team production are identical for Worker 2 because this worker finished the intermediate products to final products counted toward team production.
26. ^A complete description of the remaining control variables can be found in Appendix A.
27. ^If we use Amount transferred this does not change (p = 0.270, Mann–Whitney U-test). For both tests, we summed up the share or amount transferred within each team and ran the test with twelve independent observations per treatment. This test result is also qualitatively identical when accounting for clustering on the team level as suggested by Rosner et al. (2006) and Jiang et al. (2017) (p = 0.227).
28. ^Consider Supplementary Table 8 in Appendix A for regressions on the absolute amounts transferred.
29. ^Note that we considered zero-inflated and other two-step procedures, but they are not suitable to our data.
30. ^This test result is qualitatively identical when accounting for clustering on the team level as suggested by Rosner et al. (2006) and Jiang et al. (2017) (0.495).
31. ^A set of further exploratory analyses on the survey responses can be found in Appendix B.
32. ^For Hypotheses 1, our post hoc statistical power is at 44.12% for the non-parametric test, and for the corresponding regression analysis, this figure is at 64.06%. For Hypothesis 2, these figures are 30.59 and 26.56%, respectively.
33. ^We compared the means of age and gender in our sample as well as the distribution of study subjects to the summary statistics of the subject pool at the time of the experiment as well as to another experimental dataset of colleagues and found no systematic differences. Thus, as far as we can infer from these characteristics, the invitation to the learning factory did not attract a specific, tech-savvy subset of the subject pool.
34. ^The way we measure perceived blame or responsibility in the survey is similar to Kirchkamp and Strobel (2019), Hohenstein and Jung (2020), and Leo and Huh (2020).
References
Abbink, K., and Harris, D. (2019). In-group favouritism and out-group discrimination in naturally occurring groups. PLoS ONE 14, e0221616. doi: 10.1371/journal.pone.0221616
Abele, E., Metternich, J., Tisch, M., Chryssolouris, G., Sihn, W., ElMaraghy, H., et al. (2015). Learning factories for research, education, and training. Procedia CIRP, 32, 1–6. doi: 10.1016/j.procir.2015.02.187
Akerlof, G. A., and Kranton, R. E. (2000). Economics and identity. Q. J. Econ. 115, 715–753. doi: 10.1162/003355300554881
Allport, G. W., Clark, K., and Pettigrew, T. (1954). The Nature of Prejudice. Reading, MA: Addison-wesley.
Ariely, D., Kamenica, E., and Prelec, D. (2008). Man's search for meaning: the case of legos. J. Econ. Behav. Organ. 67, 671–677. doi: 10.1016/j.jebo.2008.01.004
Ayaita, A., and Pull, K. (2022). Positional preferences and narcissism: evidence from ‘money burning' dictator games. Appl. Econ. Lett. 29, 267–271. doi: 10.1080/13504851.2020.1863320
Bartling, B., and Fischbacher, U. (2012). Shifting the blame: on delegation and responsibility. Rev. Econ. Stud. 79, 67–87. doi: 10.1093/restud/rdr023
Bartneck, C., Nomura, T., Kanda, T., Suzuki, T., and Kato, K. (2005). “Cultural differences in attitudes towards robots,” in Proceedings of the AISB Symposium on Robot Companions: Hard Problems and Open Challenges in Human-Robot Interaction (Hatfield).
Becker, G. M., DeGroot, M. H., and Marschak, J. (1964). Measuring utility by a single-response sequential method. Behav. Sci. 9, 226–232. doi: 10.1002/bs.3830090304
Besley, T., and Ghatak, M. (2018). Prosocial motivation and incentives. Annu. Rev. Econom. 10, 411–438. doi: 10.1146/annurev-economics-063016-103739
Beuss, F., Schmatz, F., Stepputat, M., Nokodian, F., Fluegge, W., Frerich, B., et al. (2021). Cobots in maxillofacial surgery-challenges for workplace design and the human-machine-interface. Procedia CIRP 100, 488–493. doi: 10.1016/j.procir.2021.05.108
Bock, O., Baetge, I., and Nicklisch, A. (2014). hroot: Hamburg registration and organization online tool. Eur. Econ. Rev. 71, 117–120. doi: 10.1016/j.euroecorev.2014.07.003
Brewer, P. J., Huang, M., Nelson, B., and Plott, C. R. (2002). On the behavioral foundations of the law of supply and demand: human convergence and robot randomness. Exp. Econ. 5, 179–208. doi: 10.1023/A:1020871917917
Burtch, G., Greenwood, B. N., and Ravindran, K. (2023). Lucy and the chocolate factory: warehouse robotics and worker safety. SSRN Working Paper. doi: 10.2139/ssrn.4389032
Carlisle, J. H. (1976). “Evaluating the impact of office automation on top management communication,” in AFIPS '76 (New York, NY: ACM). doi: 10.1145/1499799.1499885
Carros, F., Schwaninger, I., Preussner, A., Randall, D., Wieching, R., Fitzpatrick, G., et al. (2022). “Care workers making use of robots: results of a three-month study on human-robot interaction within a care home,” in Proceedings of the 2022 CHI Conference on Human Factors in Computing Systems (New York, NY: ACM), 1–15. doi: 10.1145/3491102.3517435
Cassar, L., and Meier, S. (2018). Nonmonetary incentives and the implications of work as a source of meaning. J. Econ. Perspect. 32, 215–238. doi: 10.1257/jep.32.3.215
CBS News (2023). Are Robot Waiters the Wave of the Future? Some Restaurants Say Yes. Available online at: https://www.cbsnews.com/news/robot-waiters-restaurants-future/ (accessed September 12, 2023).
Chen, D. L., Schonger, M., and Wickens, C. (2016). oTree—an open-source platform for laboratory, online, and field experiments. J. Behav. Exp. Finance 9, 88–97. doi: 10.1016/j.jbef.2015.12.001
Chen, Y., and Li, S. X. (2009). Group identity and social preferences. Am. Econ. Rev. 99, 431–457. doi: 10.1257/aer.99.1.431
Cheng, H., Jia, R., Li, D., and Li, H. (2019). The rise of robots in china. J. Econ. Perspect. 33, 71–88. doi: 10.1257/jep.33.2.71
Chin, J. P., Diehl, V. A., and Norman, K. L. (1988). “Development of an instrument measuring user satisfaction of the human-computer interface,” in Proceedings of the SIGCHI Conference on Human Factors in Computing Systems (New York, NY: ACM), 213–218. doi: 10.1145/57167.57203
Chugunova, M., and Sele, D. (2022). We and it: an interdisciplinary review of the experimental evidence on human-machine interaction. J. Behav. Exp. Econ. 99, 101897. doi: 10.1016/j.socec.2022.101897
Cochard, F., Le Gallo, J., Georgantzis, N., and Tisserand, J. C. (2021). Social preferences across different populations: meta-analyses on the ultimatum game and dictator game. J. Behav. Exp. Econ. 90, 101613. doi: 10.1016/j.socec.2020.101613
Corgnet, B., Hernán-Gonzalez, R., and Mateo, R. (2019). Peer effects in an automated world. Labour Econ. 102455. doi: 10.1016/j.labeco.2023.102455
Cross, E. S., and Ramsey, R. (2021). Mind meets machine: towards a cognitive science of human-machine interactions. Trends Cogn. Sci. 25, 200–212. doi: 10.1016/j.tics.2020.11.009
Danilov, A., and Sliwka, D. (2017). Can contracts signal social norms? Experimental evidence. Manage. Sci. 63, 459–476. doi: 10.1287/mnsc.2015.2336
Dietvorst, B. J., Simmons, J. P., and Massey, C. (2015). Algorithm aversion: people erroneously avoid algorithms after seeing them err. J. Exp. Psychol. Gen. 144, 114. doi: 10.1037/xge0000033
Dietvorst, B. J., Simmons, J. P., and Massey, C. (2018). Overcoming algorithm aversion: eople will use imperfect algorithms if they can (even slightly) modify them. Manage. Sci. 64, 1155–1170. doi: 10.1287/mnsc.2016.2643
Dietvorst, B. J., and Bharti, S. (2019). Risk seeking preferences lead consumers to reject algorithms in uncertain domains. ACR North Am. Adv. 78–81.
Eicker, F. (1967). “Limit theorems for regressions with unequal and dependent errors,” in Proceedings of the Fifth Berkeley Symposium on Mathematical Statistics and Probability, number 1 (Berkeley, CA: University of California Press), 59–82.
Engel, C. (2011). Dictator games: a meta study. Exp. Econ. 14, 583–610. doi: 10.1007/s10683-011-9283-7
Erkal, N., Gangadharan, L., and Nikiforakis, N. (2011). Relative earnings and giving in a real-effort experiment. Am. Econ. Rev. 101, 3330–3348. doi: 10.1257/aer.101.7.3330
Forsythe, R., Horowitz, J. L., Savin, N. E., and Sefton, M. (1994). Fairness in simple bargaining experiments. Games Econ. Behav. 6, 347–369. doi: 10.1006/game.1994.1021
Fried, J., Weitman, M., and Davis, M. K. (1972). Man-machine interaction and absenteeism. J. Appl. Psychol. 56, 428. doi: 10.1037/h0033591
Gee, L. K., Migueis, M., and Parsa, S. (2017). Redistributive choices and increasing income inequality: experimental evidence for income as a signal of deservingness. Exp. Econ. 20, 894–923. doi: 10.1007/s10683-017-9516-5
Gneezy, U., and Imas, A. (2017). “Lab in the field: measuring preferences in the wild,” in Handbook of Economic Field Experiments, Vol. 1, eds A. V., Banerjee, and E. Duflo, (Amsterdam: Elsevier), 439–464. doi: 10.1016/bs.hefe.2016.08.003
Graetz, G., and Michaels, G. (2018). Robots at work. Rev. Econ. Stat. 100, 753–768. doi: 10.1162/rest_a_00754
Güth, W., Schmittberger, R., and Schwarze, B. (1982). An experimental analysis of ultimatum bargaining. J. Econ. Behav. Organ. 3, 367–388. doi: 10.1016/0167-2681(82)90011-7
Haesevoets, T., De Cremer, D., Dierckx, K., and Van Hiel, A. (2021). Human-machine collaboration in managerial decision making. Comput. Hum. Behav. 119, 106730. doi: 10.1016/j.chb.2021.106730
Harrison, G. W., and List, J. A. (2004). Field experiments. J. Econ. Lit. 42, 1009–1055. doi: 10.1257/0022051043004577
Hertz, N., and Wiese, E. (2019). Good advice is beyond all price, but what if it comes from a machine? J. Exp. Psychol. Appl. 25, 386. doi: 10.1037/xap0000205
Hoc, J.-M. (2000). From human-machine interaction to human-machine cooperation. Ergonomics 43, 833–843. doi: 10.1080/001401300409044
Hohenstein, J., and Jung, M. (2020). Ai as a moral crumple zone: the effects of ai-mediated communication on attribution and trust. Comput. Human Behav. 106, 106190. doi: 10.1016/j.chb.2019.106190
Hornecker, E., Bischof, A., Graf, P., Franzkowiak, L., and Krüger, N. (2020). “The interactive enactment of care technologies and its implications for human-robot-interaction in care,” in Proceedings of the 11th Nordic Conference on Human-Computer Interaction: Shaping Experiences, Shaping Society (New York, NY: ACM), 1–11. doi: 10.1145/3419249.3420103
Huber, P. J. (1967). “The behavior of maximum likelihood estimates under nonstandard conditions,” in Proceedings of the fifth Berkeley Symposium on Mathematical Statistics and Probability, number 1, eds L. M. Le Cam, and J. Neyman (Berkeley, CA: University of California Press), 221–233.
International Federation of Robotics (2018). Demystifying collaborative industrial robots. Positioning Paper, Available online at: https://web.archive.org/web/20190823143255/https://ifr.org/downloads/papers/IFR_Demystifying_Collaborative_Robots.pdf (accessed October 20, 2023).
Jiang, Y., He, X., Lee, M.-L. T., Rosner, B., and Yan, J. (2017). Wilcoxon rank-based tests for clustered data with R package clusrank. arXiv. [preprint]. arxiv: 1706.03409. doi: 10.48550/arXiv.1706.03409
Jussupow, E., Benbasat, I., and Heinzl, A. (2020). “Why are we averse towards algorithms? A comprehensive literature review on algorithm aversion,” in Proceedings of the 28th European Conference on Information Systems (ECIS), An Online AIS Conference. Available online at: https://aisel.aisnet.org/ecis2020_rp/168
Kahneman, D., Knetsch, J. L., and Thaler, R. H. (1986). Fairness and the assumptions of economics. J. Bus. 59, 285–300. doi: 10.1086/296367
Kandler, M., Schäfer, L., Gorny, P. M., Lanza, G., Nieken, P., and Ströhlein, K. (2021). “Learning factory labs as field-in-the-lab environments-an experimental concept for human-centred production research,” in Proceedings of the Conference on Learning Factories (CLF). Rochester, NY: Elsevier.
KD2Lab (2023). Karlsruhe Decision and Design Lab. Available online at: https://www.kd2lab.kit.edu/index.php (accessed May 08, 2023).
Kimbrough, E., and Reiss, J. (2012). Measuring the distribution of spitefulness. PLoS ONE 7, e41812. doi: 10.1371/journal.pone.0041812
Kirchkamp, O., and Strobel, C. (2019). Sharing responsibility with a machine. J. Behav. Exp. Econ. 80, 25–33. doi: 10.1016/j.socec.2019.02.010
Klockmann, V., Von Schenk, A., and Villeval, M. C. (2022). Artificial intelligence, ethics, and intergenerational responsibility. J. Econ. Behav. Organ. 203, 284–317. doi: 10.1016/j.jebo.2022.09.010
Krupka, E. L., and Weber, R. A. (2013). Identifying social norms using coordination games: why does dictator game sharing vary? J. Eur. Econ. Assoc. 11, 495–524. doi: 10.1111/jeea.12006
Leo, X., and Huh, Y. E. (2020). Who gets the blame for service failures? Attribution of responsibility toward robot versus human service providers and service firms. Comput. Human Behav. 113, 106520. doi: 10.1016/j.chb.2020.106520
Liebrand, W. B., and Van Run, G. J. (1985). The effects of social motives on behavior in social dilemmas in two cultures. J. Exp. Soc. Psychol. 21, 86–102. doi: 10.1016/0022-1031(85)90008-3
List, J. A., Shaikh, A. M., and Xu, Y. (2019). Multiple hypothesis testing in experimental economics. Exp. Econ. 22, 773–793. doi: 10.1007/s10683-018-09597-5
Logg, J. M., Minson, J. A., and Moore, D. A. (2019). Algorithm appreciation: people prefer algorithmic to human judgment. Organ. Behav. Hum. Decis. Process. 151, 90–103. doi: 10.1016/j.obhdp.2018.12.005
Lowe, M. (2021). Types of contact: a field experiment on collaborative and adversarial caste integration. Am. Econ. Rev. 111, 1807–1844. doi: 10.1257/aer.20191780
March, C. (2021). Strategic interactions between humans and artificial intelligence: lessons from experiments with computer players. J. Econ. Psychol. 87, 102426. doi: 10.1016/j.joep.2021.102426
Murphy, R. O., Ackermann, K. A., and Handgraaf, M. J. (2011). Measuring social value orientation. Judgm. Decis. Mak. 6, 771–781. doi: 10.1017/S1930297500004204
Nikolova, M., and Cnossen, F. (2020). What makes work meaningful and why economists should care about it. Labour Econ. 65, 101847. doi: 10.1016/j.labeco.2020.101847
Oexl, R., and Grossman, Z. J. (2013). Shifting the blame to a powerless intermediary. Exp. Econ. 16, 306–312. doi: 10.1007/s10683-012-9335-7
Ramalingam, A., and Rauh, M. T. (2010). The firm as a socialization device. Manage. Sci. 56, 2191–2206. doi: 10.1287/mnsc.1100.1239
Rosner, B., Glynn, R. J., and Lee, M.-L. T. (2006). The Wilcoxon signed rank test for paired comparisons of clustered data. Biometrics 62, 185–192. doi: 10.1111/j.1541-0420.2005.00389.x
Savela, N., Kaakinen, M., Ellonen, N., and Oksanen, A. (2021). Sharing a work team with robots: the negative effect of robot co-workers on in-group identification with the work team. Comput. Hum. Behav. 115, 106585. doi: 10.1016/j.chb.2020.106585
Simões, A. C., Pinto, A., Santos, J., Pinheiro, S., and Romero, D. (2022). Designing human-robot collaboration (HRC) workspaces in industrial settings: a systematic literature review. J. Manuf. Syst. 62, 28–43. doi: 10.1016/j.jmsy.2021.11.007
Stagnaro, M. N., Arechar, A. A., and Rand, D. G. (2017). From good institutions to generous citizens: top-down incentives to cooperate promote subsequent prosociality but not norm enforcement. Cognition 167, 212–254. doi: 10.1016/j.cognition.2017.01.017
Ströhlein, K., Gorny, P. M., Kandler, M., Schäfer, L., Nieken, P., and Lanza, G. (2022). “Decision experiments in the learning factory: a proof of concept,” in Proceedings of the Conference on Learning Factories (CLF). Rochester, NY: Elsevier.
Terzioğlu, Y., Mutlu, B., and Şahin, E. (2020). “Designing social cues for collaborative robots: the role of gaze and breathing in human-robot collaboration,” in Proceedings of the 2020 ACM/IEEE International Conference on Human-Robot Interaction, 343–357. doi: 10.1145/3319502.3374829
Veiga, H., and Vorsatz, M. (2010). Information aggregation in experimental asset markets in the presence of a manipulator. Exp. Econ. 13, 379–398. doi: 10.1007/s10683-010-9247-3
von Schenk, A., Klockmann, V., Bonnefon, J.-F., Rahwan, I., and Köbis, N. (2022). Lie detection algorithms attract few users but vastly increase accusation rates. arXiv. [preprint]. arxiv:2212.04277. doi: 10.48550/arXiv.2212.04277
Keywords: robotics, human-machine interaction, experimental methodology, prosocial behavior, learning factory
Citation: Gorny PM, Renner B and Schäfer L (2023) Prosocial behavior among human workers in robot-augmented production teams—A field-in-the-lab experiment. Front. Behav. Econ. 2:1220563. doi: 10.3389/frbhe.2023.1220563
Received: 10 May 2023; Accepted: 10 October 2023;
Published: 06 November 2023.
Edited by:
Marina Chugunova, Max Planck Institute for Innovation and Competition, GermanyReviewed by:
Brice Corgnet, École de Management de Lyon, FranceVictor Klockmann, Julius Maximilian University of Würzburg, Germany
Copyright © 2023 Gorny, Renner and Schäfer. This is an open-access article distributed under the terms of the Creative Commons Attribution License (CC BY). The use, distribution or reproduction in other forums is permitted, provided the original author(s) and the copyright owner(s) are credited and that the original publication in this journal is cited, in accordance with accepted academic practice. No use, distribution or reproduction is permitted which does not comply with these terms.
*Correspondence: Paul M. Gorny, paul.gorny@kit.edu