- Department of Psychological and Behavioural Science, London School of Economics, London, United Kingdom
People are increasingly interacting with forms of artificial intelligence (AI). It is crucial to understand whether accepted evidence for human-human reciprocity holds true for human-bot interactions. In a pre-registered online experiment (N = 539) we first replicate recent studies, finding that the identity of a player's counterpart in a one-shot binary Trust Game has a significant effect on the rate of reciprocity, with bot counterparts receiving lower levels of returned amounts than human counterparts. We then explore whether individual differences in a player's personality traits—in particular Agreeableness, Extraversion, Honesty-Humility and Openness—moderate the effect of the identity of the player's counterpart on the rate of reciprocity. In line with literature on human-human interactions, participants exhibiting higher levels of Honesty-Humility, and to a lesser extent Agreeableness, are found to reciprocate more, regardless of the identity of their counterpart. No personality trait, however, moderates the effect of interacting with a bot. Finally, we consider whether general attitudes to AI affect the reciprocity but find no significant relationship.
Introduction
The growth in the sophistication of artificial intelligence1 (AI) have led some to proclaim the dawn of the Fourth Industrial Revolution (Schwab, 2017; Skilton and Hovsepian, 2018). AI has been identified as a crucial driver of future economic growth, with impacts being felt across labour markets, innovation, trade and productivity, among other facets of the so-called “robot economy” (Agrawal et al., 2019; Furman and Seamans, 2019). Human-AI interaction already generates efficiencies in a number of fields including industrial assembly (Faber et al., 2015; Villani et al., 2018) and mixed traffic (Nyholm and Smids, 2020). Our reliance on engagement with, and assistance from, bots and machines is growing across all the sectors of the economy (Makridakis, 2017; Rahwan et al., 2019; Dafoe et al., 2021; Chugunova and Sele, 2022).
Rahwan et al. (2019) note that studies of machine behaviour must integrate analysis of the social environments in which algorithms operate. Trust, reciprocity, cooperation, and altruism have long been identified as some of the bedrocks for human behaviour, economic growth and development, and social cohesion (Arrow, 1972; Andreoni, 1988; Camerer and Thaler, 1995; Fehr and Gächter, 2000, 2002; Charness and Rabin, 2002; Nowak, 2006; List, 2007; Algan and Cahuc, 2014). Trust and collaboration with bots will likely form a fundamental enabler for economic growth: it is reckoned that trust and reciprocity are essential aspects of the interactions between humans and bots (Lee and See, 2004; Lorenz et al., 2016; Sandoval et al., 2016; Sheridan, 2019; Schniter et al., 2020). It is thus crucial to understand whether accepted evidence for human-human trust and reciprocity holds true also for human-bot interactions.
While some recent studies suggest that humans show lower levels of reciprocity when interacting with bots as compared to human counterparts (Ishowo-Oloko et al., 2019; Karpus et al., 2021), others find no significant differences (Kirchkamp and Strobel, 2019). In summarising the stream of literature exploring human-machine interaction, March (2021) notes that the “overall finding is that subjects can cooperate with [computer players] as much as with other subjects, but this depends on the information about them” (p. 6).
We use a pre-registered between-subjects design to randomise participants into one of two experimental conditions: either playing with a human or a bot trustor counterpart in a one-shot binary Trust Game. The Trust Game (Berg et al., 1995) is perfectly suited to exploring positive reciprocity behaviour, and has been repeatedly used to test the relationship between numerous individual characteristics and trust and trustworthiness (Berg et al., 1995; Ortmann et al., 2000; Cox, 2004; Ashraf et al., 2006; Ermisch et al., 2009; Johnson and Mislin, 2011; Algan and Cahuc, 2014; Thielmann and Hilbig, 2015; Zhao and Smillie, 2015; Alós-Ferrer and Ritzberger, 2016; Alós-Ferrer and Farolfi, 2019). As a benchmark, in a human-human Trust Game, trustors generally send half of their endowment to trustees, receiving in return from trustees about a third of the pot multiplied by the experimenter (Johnson and Mislin, 2011; see further Figure 1 in Alós-Ferrer and Farolfi, 2019, for depictions of different formats of the Trust Game).
The dimensions of trust and reciprocity covered by the Trust Game are relevant to the ever-increasing range of human-machine interactions. Examples offered by March (2021) of strategic interactions utilising AI range from automated actors operating on behalf of humans in strategic settings (e.g., financial markets) to AI creating new strategic decisions (e.g., patients deciding whether to rely on diagnosis from AI or to seek a second, human, opinion). Further, automated agents are increasingly being used in e-commerce where trusting relationships are needed to facilitate economic transactions (Liu, 2010; Foehr and Germelmann, 2020). In addition, with automated agents being used to augment human teams, researchers are starting to explore how humans behave with automated agents acting as co-workers and teammates, as well as reactions from humans to delegated decision making from automated agents (Chugunova and Sele, 2022).
Chugunova and Sele (2022) discuss several potential factors which moderate responses to automated agents in social preferences games such as Dictator, Ultimatum, and Public Good Games, including emotional and social concerns, cultural backgrounds, and features of the experimental tasks and design. Other experimental studies have already looked at trust games between humans and bots (Mota et al., 2016; Oksanen et al., 2020; Schniter et al., 2020; Cominelli et al., 2021; Karpus et al., 2021). In this paper, we specifically focus on an aspect that has received comparatively less attention, namely whether positive reciprocity between humans and bots in the Trust Game is moderated by personality traits.
We first replicate the results of a previous study by Karpus et al. (2021) which highlighted a significantly lower rate of positive reciprocity where an AI trustor was paired with a human trustee. We then test whether personality traits of the human trustee moderate the interaction between human players and AI similarly to what has been documented for human-human Trust Games (Thielmann and Hilbig, 2015; Zhao and Smillie, 2015). The intersection between economic games, personality traits, and AI is an emerging avenue of research with surprisingly limited work conducted to date. We are not aware of any study investigating the role of personality traits as moderating factors in the context of an experimental Trust Game. In the context of other economic games, there is a recent study by Nielsen et al. (2021) on the impact of personality traits on contributions in the Dictator Game and the Public Goods Game with participants playing with humans or a computer. Given that personality traits and non-cognitive skills are playing a growing role in explaining economic behaviours and socio-economic outcomes (Heckman and Rubinstein, 2001; Stixrud and Urzua, 2006; Borghans et al., 2008; Denissen et al., 2018; Borghans and Schils, 2021), it is interesting to explore the role they can play in economic interactions with bots (Bashirpour Bonab et al., 2021). We also consider whether human attitudes towards AI affect the rate of reciprocity. Karpus et al. (2021) discussed a series of potential explanations including humans having a heightened competitive desire to outperform their AI counterpart, and humans perceiving machines as part of an out-group and therefore having fewer qualms about exploitation. Karpus et al. (2021), however, did not explore the role of personality traits nor of attitudes towards AI as potential explanatory factors for the lower rate of reciprocity with AI counterparts.
Against this background, we first examine whether the identity of a player's counterpart—either a human or machine—in the Trust Game, influences the rate of reciprocity, predicting that participants would exhibit a lower level of reciprocity with machine counterparts (Hypothesis 1, H1). Further, we posit that individual differences in a player's personality traits, in particular Agreeableness, Extraversion, Honesty-Humility and Openness, would moderate the effect of the identity of the player's counterpart on the rate of reciprocity (Hypothesis 2, H2). Finally, we expect that the player's general attitudes towards AI would moderate the effect of the identity of the player's counterpart on the rate of reciprocity (Hypothesis 3, H3).
The rest of the paper is organised as follows: Section 2 discusses the literature at the intersection between economic games, personality traits and AI, three fields that have only recently started to be connected; Section 3 describes the design and methods; Section 4 presents the results, while Section 5 discusses the main findings and briefly concludes.
Literature and background
Overview
Experimental economic games have long been used as a proxy to empirically test trust, reciprocity, cooperation, and altruism between humans (Camerer, 2003; Johnson and Mislin, 2011; Thielmann et al., 2020). Personality traits have been posited as one potential explanation for these behaviours, with higher levels of traits such as agreeableness found to be present in those who cooperate in social dilemma or public goods games (Pothos et al., 2011; Guilfoos and Kurtz, 2017; Müller and Schwieren, 2020). Personality traits have in turn been used to analyse human perceptions of robots, with agreeableness and openness found to predict positive evaluations of interactions with robots (Takayama and Pantofaru, 2009; Bernotat and Eyssel, 2017; Robert et al., 2020). Finally, economic games have most recently been used to test reciprocity between humans and AI, with mixed findings (Sandoval et al., 2016; Kirchkamp and Strobel, 2019; Karpus et al., 2021). Although a range of potential factors have been considered as moderating the responses to automated agents in social preference games, personality traits have only very recently been considered as moderating factors (Nielsen et al., 2021) and not yet in the context of the Trust Game.
Economic games and interactions with bots
Even as the first modern computers were being developed, researchers began to consider whether there is a difference between how people cooperate with humans and “human-like” computers, finding that people are more likely to break promises or cheat when dealing with a computer (Kiesler et al., 1996). As computers have grown in sophistication, so have experimental paradigms. From complex resource-sharing scenarios (Whiting et al., 2021) and immersive investment games (Zörner et al., 2021), to the creation of a reinforcement-learning algorithm that can signal to counterparts (Crandall et al., 2018), an entire field now exists to investigate the behavioural and logistical factors which affect human-bot cooperation (Rahwan et al., 2019; Chugunova and Sele, 2022).
Some studies find that humans cooperate less with machines than other humans across a range of economic games including the Prisoner's Dilemma (Sandoval et al., 2016; Ishowo-Oloko et al., 2019) and the mini-Ultimatum Game (Sandoval et al., 2016). When the true identity of a human player's counterpart was revealed to be a bot in a repeated Prisoner's Dilemma, cooperation rates dropped significantly, despite cooperation generating higher profits (Ishowo-Oloko et al., 2019). Participants playing the mini-Ultimatum Game were found to collaborate more with humans than bots, although there was no significant difference in the negative reciprocity shown to either counterpart in a different stage of the game. In a series of experiments using two-player games including the Chicken Game and the Trust Game, Karpus et al. (2021) found that while human participants exhibited similar levels of trust towards their AI counterparts as they did human counterparts, they did not reciprocate trusting behaviour from AI counterparts, instead exploiting the AI more than humans. Less pro-social and more self-serving responses towards AI partners in experimental games have also been found by Moon and Nass (1998), Moon (2003), de Melo et al. (2016), Adam et al. (2018), Farjam and Kirchkamp (2018), Corgnet et al. (2019), Erlei et al. (2020), Köbis et al. (2021), and Klockmann et al. (2022). On the other hand, Kirchkamp and Strobel (2019), using a modified Dictator Game to investigate whether humans perceive a decision shared with a computer differently from one shared with a human, found no significant differences in the number of selfish choices made when working with a human or machine counterpart. Our H1 is motivated by this first stream of literature: we examine whether the identity of a player's counterpart—either a human or machine—in the Trust Game, influences the rate of reciprocity, predicting that participants would exhibit a lower level of reciprocity with machine counterparts (Hypothesis 1, H1).
Personality traits and economic games
Personality traits have been shown to predict outcomes as well as, or better than, economic preferences for a range of key variables such as job persistence and credit scores (Rustichini et al., 2016). Importantly, personality can be modelled as a stable trait into economic decisions (Cobb-Clark and Schurer, 2012). Further, the structure of personality traits appear to be generalised across a diverse range of cultures (McCrae and Costa, 1997; García et al., 2022), at least in Western, educated, industrialised, rich and democratic (WEIRD) societies (Henrich et al., 2010; Dijk and De Dreu, 2020), with some studies using personality trait models to conduct cross-cultural comparisons (Morelli et al., 2020).
Two major models have been used to analyse the interaction between personality traits and outcomes in economic games. The Five-Factor Model (FFM) features Agreeableness, Conscientiousness, Extraversion, Neuroticism, and Openness to Experience) (Digman, 1990; McCrae and Costa, 1997). The HEXACO Model (Lee and Ashton, 2004, 2018) features a six-factor structure which is conceptually different from the FFM (Thielmann et al., 2021), covering Honesty-Humility, Emotionality, EXtraversion, Agreeableness, Conscientiousness, and Openness to Experience2 (see Appendix A.1 for a detailed breakdown of the traits).
Personality traits have been shown to affect reciprocity and prosocial behaviour, both generally and more specifically in the context of economic games (Proto et al., 2019; Sofianos, 2022). In a study of contextual performance, agreeableness in particular was found to explain cooperative behaviour in team tasks (LePine and Van Dyne, 2001). In a re-analyses of previous studies, Honesty-Humility but not Agreeableness was found to predict active cooperation (i.e., non-exploitation), with the latter instead linked to reactive cooperation (i.e., reciprocity, non-retaliation) (Hilbig et al., 2013). In the context of economic games, findings suggest Honesty-Humility, Agreeableness and, to a lesser extent, Openness are traits present in those who cooperate in social dilemma or public goods games (Pothos et al., 2011; Kagel and Mcgee, 2014; Guilfoos and Kurtz, 2017; Müller and Schwieren, 2020; see more generally Thielmann et al., 2020; Lawn et al., 2021).
Within the context of the Trust Game, there are comparatively few studies (compared to those focusing on the behaviour of player 1) looking at the influence of personality traits on trustee behaviour (Thielmann and Hilbig, 2015). Those that do, however, suggest a significant role of agreeableness and openness (Ben-Ner and Halldorsson, 2010; Becker et al., 2012). However, Müller and Schwieren (2020), applying the FFM to interpret behaviour in a Trust Game, concluded that while personality contributed to explaining the behaviour of player 1 (with agreeableness and openness having a significant positive impact on the amount of money sent by the trustor), the behaviour of player 2 was instead mostly affected by the behaviour of the player 1. Their findings lend evidence to the strong situation hypothesis (Mischel, 1977), which suggests that personality contributes more to decisions in ambiguous situations (deemed “weak”) compared to when there are situational triggers (deemed “strong”), such as the actions of player 1, which instead form the basis of a decision (see also Zhao and Smillie, 2015). While this presents a potentially interesting moderating effect of personality traits, the overall empirical basis for the overarching theory has been called into question (Cooper and Withey, 2009).
For studies involving the analysis of the actions of the trustee in the Trust Game, Thielmann et al. (2020) show at least a small or a medium positive correlation between Honesty-Humility and reciprocating behaviour (meta-analytic r = 0.22, p < 0.001, k = 7), with weaker correlations for FFM Agreeableness (r = 0.13, P < 0.001, k = 28) and HEXACO Agreeableness (r = 0.11, P < 0.001, k = 7) (p. 54). Falling within the top ~40% of effect sizes reported in personality psychology (i.e., r > 0.21, Gignac and Szodorai, 2016), the question of whether this link between Honesty-Humility and reciprocity holds when interacting with bots bears investigation.
The impact of personality traits on human interactions with bots is an open question. A recent study considered whether giving behaviour in economic games reflects true prosocial preferences or instead confusion on the part of the participants. In tackling this question, Nielsen et al. (2021) analysed the impact of personality traits on contributions in the Dictator Game and the Public Goods Game with participants playing with humans or a computer. Interestingly, Honesty-Humility was found to be significantly related to allocations to both types of counterparts (r = 0.21, p < 0.001 for humans, and r = 0.11, p = 0.019 for bots) in a two-player Dictator Game, but not to contributions in a two-player Public Goods Game (r = 0.10, p = 0.085 for humans, and r = 0.04, p = 0.431 for bots)3. No evidence is available for playing experimental trust games with bots in this context, something which motivates our H2: we posit that individual differences in a player's personality traits, in particular Agreeableness, Extraversion, Honesty-Humility and Openness, would affect reciprocity in a human-bot trust game and would moderate the effect of the identity of the player's counterpart on the rate of reciprocity (Hypothesis 2, H2).
Interactions with bots and personality traits
Following a wide-ranging review, Robert et al. (2020) confirm that “personality has been identified as a vital factor in understanding the quality of human-robot interactions” (p.1). Although the review focuses on interactions between humans and embodied-personality robots, it provides useful direction, both in terms of the relevance of personality to human perceptions of robots, and more specifically identifying traits (whether of the human or embodied by the bot) that may influence reciprocity.
Researchers typically seem to use the FFM to examine robot personality (Chee et al., 2012; Hwang et al., 2013; Robert et al., 2020) with agreeableness and openness predicting positive evaluations of interactions with robots (Takayama and Pantofaru, 2009; Bernotat and Eyssel, 2017; Robert et al., 2020). In one study, people who exhibited higher levels of agreeableness felt more comfortable being closer to a robot in a behavioural experiment about personal space than those who had lower levels of agreeableness (Takayama and Pantofaru, 2009), although in another study traits were found to have null effect on approach distance (Syrdal et al., 2007). Robert et al. (2020) find that extraversion is the most commonly explored trait in terms of understanding the impact of personality on perception of robots, with one study suggesting that individuals high in extraversion are more likely to afford bots with a higher level of trust (Haring et al., 2013). These other findings, none of which, again, refer to experimental trust games, further motivate our H2.
Our Hypothesis 3 is arguably more speculative and, rather than on the previous literature, was based mainly on our intuition that general attitudes towards AI could potentially explain reciprocity towards bots in an experimental trust game. We expected that the player's general attitudes towards AI would moderate the effect of the identity of the player's counterpart on the rate of reciprocity (Hypothesis 3, H3).
Design and procedures
To understand whether and how the identity of the counterpart affects the rate of reciprocity, we used a between-subjects design to randomly allocate participants into one of two experimental conditions: either playing with a human or a bot trustor counterpart in a binary Trust Game. Participants also provided demographic information as well as answering questionnaires on the HEXACO and General Attitudes towards Artificial Intelligence Scale (GAAIS) measures to support the analysis of the role of traits and attitudes on the rate of reciprocity. In what follows, we briefly describe the experimental design, the measures, and the sample.
Experimental design
Participants and questionnaire
The study was conducted online with participants drawn from Prolific Academic (prolific.co) (Zhou and Fishbach, 2016; Arechar et al., 2018; Sauter et al., 2020) (see Appendix A.3). The Gorilla Experiment Builder (app.gorilla.sc) (Anwyl-Irvine et al., 2020) (Gorilla) was used to design and host the experiment, and to collect data.
The 60-item English HEXACO Personality Inventory-Revised (PI-R) (Ashton and Lee, 2009), which has been used extensively in analysing the outcomes of economic games and also in mapping human interactions with bots (Robert et al., 2020; Thielmann et al., 2020), was used as the base traits questionnaire. Questions relating to Emotionality and Conscientiousness were removed (see Appendix A.1 for questionnaire and scoring key) to reduce the time spent by, and fatigue of, participants in completing the entire experiment. The literature indicated that Emotionality and Conscientiousness had been found to be of limited relevance to human-machine interactions across a range of settings. Responses were provided by participants on a five-point Likert-scale ranging from Strongly Disagree to Strongly Agree. In the estimations we use the raw responses to the five-point Likert-scale questions in each HEXACO subscale. In the Supplementary material we also report, as robustness checks, the results replicating the analyses using standardised measures of the responses in the subscales.
To test H3, participants completed the GAAIS (Schepman and Rodway, 2020) (see Appendix A.2 for questionnaire and scoring key) which operates on the same five-point Likert-scale as the HEXACO PI-R. Validated through exploratory factor analysis and displaying good psychometric properties, the GAAIS was developed to measure attitudes towards AI in different contexts. The GAAIS features independent (although correlated) Positive and Negative subscales. The higher the participant's score on each scale, the more positive their attitude towards AI. As with the HEXACO subscale, in the estimations we use the raw responses to the GAAIS five-point Likert-scale questions and in the Supplementary material we also report, as robustness checks, the results replicating the analyses using standardised measures of the responses in the subscales.
Experimental paradigm
We used the same experimental paradigm used by Karpus et al. (2021). This experimental paradigm also meets the criteria for a trust situation set out by Alós-Ferrer and Farolfi (2019), occurring when: (a) the trustor's decision to trust is voluntary; (b) there is a time lag between the choices made by the trustor and trustee; (c) the possibility for the trustee to abuse or honour the reciprocity (i.e., trust) occurs if and only if the trustee does indeed show reciprocity; d) the trustor is left worse off (when compared to a situation where the trustor did not show trust) if the trustee decides to abuse the reciprocity; and (e) trust should be optimal, in the sense of maximising the sum of the payoffs.
Figure 1 sets out a visual representation of the incentive-compatible paradigm used in this study. Participants acting as the trustee (i.e., player 2) were informed in the instructions that their counterpart (the trustor, player 1) was either a human or bot. They were then informed that, during their turn, their counterpart had chosen to play , that is a trusting move giving both parties the chance to potentially earn more than the 30 credits each that would be due to each player if the counterpart had played the alternative strategy
. To avoid any confusion, it was made explicit that this meant that the participant's counterpart had chosen not to play
but the participant was left to interpret what this meant in terms of the overall pot available for distribution. The trustee then had two options to choose from. They could either play
, that is, a reciprocating move rewarding the trustor's trust, giving each player a return of 70 credits each; or play
, that is a strategy betraying the trustor's trust, leaving the trustee with 100 credits and the trustor with 0.
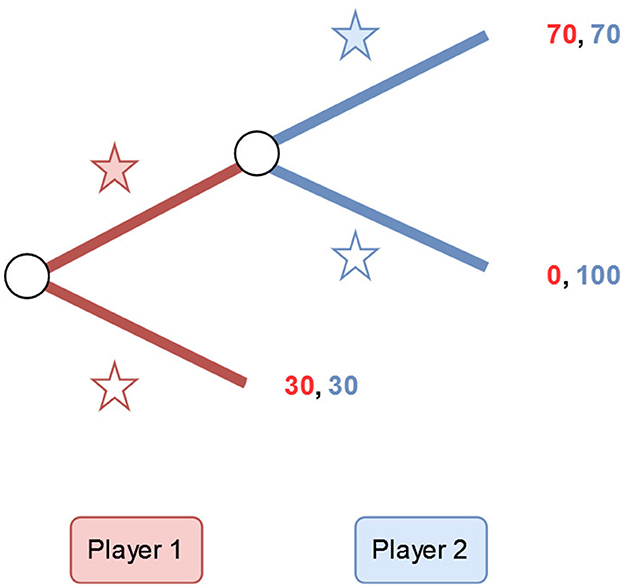
Figure 1. Diagram of the options available to participants and associated payoffs in the binary Trust Game used in this study.
In our study, reciprocity is therefore operationalised as the willingness of the human trustee to play in response to being informed that their counterpart, either human or bot, had played
. The overall rate of reciprocity is calculated by dividing the number of times the human player plays
in a condition by the number of human players assigned to that condition.
We used the strategy method to implement the Trust Game as an asynchronous game of strategic interaction in an online experiment (Brandts and Charness, 2000, 2011; Brosig et al., 2003; Burks et al., 2003; Oxoby and McLeish, 2004; Bardsley et al., 2009; Casari and Cason, 2009), implementing what March (2021) calls a “Human-like [computer player]” which mimics the behaviour of human subjects in a previous experiment. We used a simple one-shot Trust Game to avoid confounding factors related to learning, feedback, income, or reputation building effects (Goeree and Holt, 2001, 2004), and because a one-shot game better reflects first encounter situations with bots where human decision-makers have limited information about the bot counterpart. For the same reason we used a generic and neutral presentation of the bot counterpart.
Before participants in the experiment were asked to make their choices as the second player, we asked separate samples of participants to make their choices as the first players in the binary Trust Game. Some players selected to play and others
. For the human condition, we selected those players from the separate sample who chose to play
to be matched as player 1 to the player 2 participants. For the bot condition, we selected the same AI algorithm originally used by Karpus et al. (2021) which proposed to play
to be matched as player 1 to all the player 2 participants. This asynchronous, ex-ante pairing procedure is the same procedure employed by Karpus et al. (2021) and in other experiments in economic game experiments (for example, Shapiro, 2009; Cox et al., 2017). Participants received a base payment of £1.50 for completing the study, an effective rate of £6 per hour in line with the minimum wage in the UK. They were also eligible to receive a bonus allocated by the randomised payment. Each credit earned in the game was worth £0.02 for the purposes of any bonus payment. The option to betray the trust of the counterpart and to earn 100 credits was therefore the most profitable move for the participant. Matched player 1s whose original offers were selected to be used in the experiment were also paid a bonus depending on the corresponding moves of player 2.
In line with the largest share of the studies recently reviewed by von Schenk et al. (2023) (132 studies out of 160), no specific information was given to players 2 about whether machine players 1 also received payoffs. This corresponds to the “no information” condition in the experiment by von Schenk et al. (2023). This was done also to make our experimental interaction with bots as close as possible to real-world situations where usually there is no information about possible compensations to machines: in another recent review of the literature, March (2021) notes that “full information about AI that humans interact with is unlikely to apply in the real world” (p. 13), whereas Oksanen et al. (2020) argue that in experimental games it is important to give as few cues as possible about the nature of robots and AI because in real-world online behaviours “various cues are left out” (p. 8).
The only significant departures from the original protocol used by Karpus et al. (2021) was the introduction of a randomised lottery to decide which participants would be paid the amount earned in the study (with 1 credit = £0.02) in order to make the decision incentive-compatible, along with a change in the description of whether or not the machine would receive a payoff. Further information about the participant pairing procedure, instructions provided regarding the nature of the participant's counterpart and the compensation procedure can be found in Appendix. A.3.
Sample size and power
Informed by the analysis fully set out in Appendix A.4, a conservative, small effect size of d = 0.25 (Cohen, 2013) was set as the minimal difference to be detected between the study groups (Gignac and Szodorai, 2016; Funder and Ozer, 2019). Appendix A.4. also contains details of our a priori power analysis (Glennerster and Takavarasha, 2013; Munafò et al., 2017; Lakens, 2021): using G*Power 3.1 (Faul et al., 2009) it indicated a sample size of 265 participants per condition would be needed to detect a small effect of d ≥ 0.25 at α = 0.05 with appropriate statistical power (1-β = 0.80).
Control variables
In addition to the personality and attitude measures noted above, additional robustness checks were conducted using a range of covariates which the literature suggested might interact with, or moderate, the rate of reciprocity. Age (Oksanen et al., 2020), gender (Spiel et al., 2019), experience with game theory games of strategic interaction (Chandler et al., 2014), and religiosity were all used by Karpus et al. (2021) as covariates. An additional covariate, experience interacting with bots, was included based on research that suggests that the outcome of experiments involving machines can be affected by a novelty effect on those who have not previously interacted with a bot (Chandler et al., 2014).
Ethics and pre-registration
The study complied with the London School of Economics and Political Science Research Ethics Policy and Procedures (reference: 49465). There was no deception, and all participants provided their explicit informed consent. The study was pre-registered in OSF4 There were no material deviations from the pre-registered protocol, save that, in analysing H2, personality traits were not categorised by percentiles (see below) and the study was stopped once the minimum power threshold was reached.
Results
Sample
Five hundred and thirty nine5 participants completed the study. Supplementary Table A1 confirms the general balance of the observable characteristics of the participants between the two experimental conditions, with additional commentary on data analysis and dropouts. Table 1 reports the characteristics of the whole sample.
Reciprocity with human and machine counterparts (H1)
Participants reciprocated significantly more in the human condition than in the bot condition (see Figure 2). When participants, acting in the role of the trustee (i.e., Player 2) were told that the trustor (i.e., Player 1) was a human, they reciprocated 58% of the time, while the rate of reciprocity was 42% when they were told that the trustor was a bot (see Figure 2).
A chi-square test for difference in proportions shows a significant relationship between the identity of the counterpart and the rate of reciprocation [χ2 (1, N = 539) = 13.403, p < 0.001]: consistent with results from recent studies, people are more likely to reciprocate a human counterpart than a bot counterpart when playing the Trust Game.
In the pre-registration, age and experience with game theory were selected as a potentially relevant co-variates for robustness analysis. However, it turns out that there was a generally negligible or weak association between the rate of reciprocity and the control variables (see Supplementary Table A2). This finding is in line with the results obtained by Karpus et al. (2021) which suggest that the only significant factor behind trust was the identity of a player's counterpart. Experience with bots, the other control selected for our study, was similarly not significant.
A set of probit regression models was then used to analyse the robustness of the treatment effect on reciprocation across multiple specifications controlling for different sets of co-variates (see Supplementary Table A3). The result from Model 1 (p < 0.001) confirms that the identity of a player's counterpart has a significant effect on the rate of reciprocation. The effect of the identity of the counterpart is robust across all the specifications including controls (Models 2–7), with marginal effects consistently indicating that, even controlling for co-variates, participants randomly assigned to the bot condition are between 15% and 16% less likely to reciprocate than participants in the human condition (see Table 2). Age is the only co-variate which has a small and (marginally) statistically significant impact on the rate of reciprocity (p = 0.041).
Effect of personality types on reciprocity with human and machine counterparts (H2)
There was reasonable variation in the scores for personality traits, as also highlighted by acceptable to good alpha and omega coefficients for all the scales (Dunn et al., 2014; Hayes and Coutts, 2020, see Table 3 for descriptive statistics). A randomisation check confirms balance of personality traits between experimental conditions (see Supplementary Table A4). Supplementary Figures A10–A13 provide a visual summary of the distribution of the traits between the responses. Correlation coefficients for the personality traits and the choice made by the participants in the game can be found in Supplementary Table A5.
A set of probit regression models was used to analyse the effect of the personality traits on the rate of reciprocation across the conditions (see Supplementary Table A8). Personality traits were analysed based on the raw scale (i.e., 1 to 5) as the literature does not indicate any consensually validated categories, for example a recognised level at which a person could be said to have a “high” or “low” level of a particular trait. However, in robustness checks reported in the Supplementary material, we also replicated all the analyses using standardised scores of the personality traits subscales, finding substantially similar results to the ones reported below.
The effect of the identity of the counterpart is robust across all the specifications including personality traits as controls (Models 8–12), with marginal effects consistently indicating that, even controlling for personality traits, participants randomly assigned to the bot condition are between 15% and 16% less likely to reciprocate than participants in the human condition (see Table 4).
An analysis of the marginal effects from the probit models suggests that, controlling for the experimental condition, participants who exhibit one more point in the Honesty-Humility scale are, on average, 11.5% more likely to cooperate, and those that exhibit one more point in the Agreeableness scale are, on average, 6.8% more likely to show such reciprocity (see Table 4). The results reveal that the personality traits that most affect the actions of the trustee in a Trust Game between humans are also relevant in games between humans and bots, although the proportions of variance explained by the models are relatively low.
We also conduct moderation analysis to formally test whether personality traits moderate the effect of the bot experimental condition. Using a moderation-of-process design, we conduct further regression analyses looking at the interactions between the bot condition and each of the personality traits. The regressions, which include the interaction terms together with the relevant main effects of the experimental condition and the personality traits, show that the estimated coefficients of all the interaction terms are not statistically significant at conventional levels (Table 5). This indicates that none of the personality traits moderate the effect of the bot condition or, in other words, that none of the personality traits play any different role in human-bot interactions compared to human-human interactions.
Effect of general attitudes towards AI on reciprocity with human and machine counterparts (H3)
There was reasonable variation also in the scores for the GAAIS subscales, as also confirmed by good alpha and omega coefficients for all the subscales [(Dunn et al., 2014; Hayes and Coutts, 2020), see Supplementary Table A9 for descriptive statistics]. A randomisation check confirmed a balance of GAAIS scales between conditions (see Supplementary Table A10). Supplementary Figures A14, A15 provide a visual summary of the distribution of the GAAIS scales between the responses.
Correlation analysis between the subscales and the choice made by the participants in the game (see Supplementary Table A11), reveal negligible and not significant correlation between the GAAIS subscales and the response of the participants. The strong and significant correlation between the subscales themselves was expected as the higher the score on each subscale, the more positive the attitude (Schepman and Rodway, 2020). A set of probit regression models (see Supplementary Table A13) confirms that H3 was not supported by the data. The effect of the identity of the counterpart is robust also across all the specifications including GAAIS scales as controls (Models 13–15 in Supplementary Table A13), with marginal effects consistently indicating that, even controlling for them, participants randomly assigned to the bot condition are about 15.5% less likely to reciprocate than participants in the human condition. In robustness checks reported in the Supplementary material, we also replicated all the analyses using standardised scores of the GAAIS subscales, finding substantially similar results to the ones reported above.
Discussion
The tendency to reciprocate shown by humans playing economic games with other humans is well established. As we move towards a new age where interactions with AI are rewiring entire sectors of the economy and the society, it is vital to understand whether and how human-bot interaction differs from the findings of human-human studies (Rahwan et al., 2019; March, 2021; Chugunova and Sele, 2022). Over and above the debates about what it means to be “fair” to bots (Ishowo-Oloko et al., 2019), there are stark practical consequences to humans not trusting bots. Machines which are both subject to reciprocal behaviour from humans and capable of using such data to inform future behaviour may learn to mimic such an approach in subsequent interactions (Karpus et al., 2021). Such an outcome would inevitably reduce or entirely dissipate the efficiency gains generated by introducing AI in economic interactions. In this study we assess whether human respondents reciprocate less towards bots than other humans, and we explore the role of personality traits and attitudes towards AI.
Our first hypothesis (H1) was that participants would exhibit a lower level of reciprocity with machine counterparts. Consistent with recent studies, we confirm that people are more likely to reciprocate a human counterpart than a bot counterpart when playing the Trust Game. Our results thus contribute to the growing pool of evidence that reveals a new framework of reference is required when considering interactions between humans and bots. While some have suggested machines may need to hide their non-human identity for the sake of efficiency (Ishowo-Oloko et al., 2019), this position is less than optimal in terms of transparency and may end up leading to lower levels of reciprocity with other humans if the identity of a counterpart is uncertain (Karpus et al., 2021).
We expected that individual differences in personality traits, in particular Agreeableness, Extraversion, Honesty-Humility and Openness, would moderate the effect of the identity of the player's counterpart on the rate of reciprocation (H2). Our hypothesis was mainly based on existing literature around human-human interactions. While there was no significance in the effect of Extraversion and Openness on the rate of reciprocation, participants who exhibited higher levels of Honesty-Humility and, to a lesser extent, Agreeableness were more likely to reciprocate regardless of the identity of their counterpart. This result mirrors the findings from studies of human-human interactions, where Honesty-Humility and Agreeableness have been shown to contribute to higher levels of reciprocity. However, and importantly, no personality trait moderates the effect of interacting with a bot when deciding to reciprocate. These findings around personality traits might be used as a potentially helpful new lens through which to investigate human-bot interactions.
The impact of personality traits on trustee behaviour in our study complement the findings from Müller and Schwieren (2020). As outlined above, the study, focusing on human-human interactions, found no correlation between the behaviour of the trustee and any personality factors from the FFM, with the authors concluding that if players find themselves in a strong position, personality factors do not explain behavioural differences. Instead they concluded that it was the amount sent by the trustor that predicted trustee behaviour. The experimental paradigm used by Müller and Schwieren (2020) allowed participants to return a range of amounts, which may perhaps explain the differences in outcome. Participants in our study faced a stark binary decision and we acknowledge that a paradigm which offers a higher degree of granularity in returns may have yielded different results.
Personality traits are already considered significant in affecting outcomes of social and economic interactions between humans (Cobb-Clark and Schurer, 2012; Rustichini et al., 2016; Proto et al., 2019; Sofianos, 2022). Given our findings, the personality traits of users could be taken into account in developing more effective interactions between humans and computers (Alves et al., 2020): for example, researchers are considering how elements of a user interface can be influenced by personality traits (Al-Samarraie et al., 2018), with the aim of designing more personalised systems that lead to more effective engagement from users. The findings from our study could be used to inspire AI interaction design that activates, or caters to, user traits of Honesty-Humility and Agreeableness which may in turn promote reciprocity. While not all potential users will have high levels of such traits, working with those who do may create allies and ambassadors for the wider use of bots.
Our final hypothesis was that general attitudes towards AI would moderate the effect of the identity of the player's counterpart on the rate of reciprocity. Contrary to our expectations, this hypothesis was not supported by the experimental data. It is possible that while people do not hold general negative views towards AI, they may take the opportunity in the moment to exploit AI despite their general beliefs.
We also acknowledge some limitations of our study.
First, we acknowledge that the combination of using the asynchronous strategy method with the different and asymmetric practical solutions employed to generate binary Trust Game offers from the human and machine players 1, and to administer their payments, makes our experimental procedure complex and cumbersome. While participants were informed about the whole procedure, it is possible that participants may have been confused about the monetary consequences of their choices on both their own payoffs and the payoffs for their matched player 1. If this was the case, there may well be multiple interpretations of our results. Although our preferred interpretation of the results is differences in levels of reciprocity, there may be other factors at play such as participants' concerns about efficiency, social pressure, or self-serving bias, as discussed in detail by Chugunova and Sele (2022).
Further, the degree to which humans cooperate with machines depends on the implementation of, and information about, payoffs for the machine (von Schenk et al., 2023; see more generally also March, 2021). Using a Dictator Game and a Reciprocity Game, von Schenk et al. (2023) ran a between-subjects study manipulating the identity of the counterpart (human and machine), and the description of payoffs, including explanations that the programmer of the algorithm or an independent third person (token player) would keep payoffs earned by the machine, the computer earning and keeping money itself, and providing no information about payoffs. They found that people show signs of reciprocity towards humans and towards machines where humans (either the programmer or the token player) earn the payoffs, but that this reciprocity falls aways when there are no human beneficiaries or no information about the payoffs is provided. The findings regarding lower reciprocity towards machines in our study may have been affected by our payoff instructions that are similar to the “no information” condition in von Schenk et al. (2023). Our study contributes to this evidence by documenting that personality traits do not moderate the impact of the machine identity counterpart in a Trust Game. Further studies are needed to fully investigate the impact of personality traits across the various payoff conditions used by von Schenk et al. (2023).
As noted above, like many other studies on economic games and most online experiments, we have used a pool of participants from a western developed country. Given there are undoubtedly cultural and societal factors which influence trust and reciprocity both more generally (Bohnet et al., 2008; Fehr, 2009) and in the context of economic games more specifically (Henrich et al., 2005), and given the global proliferation of AI, this presents a significant limitation to the generalisability of the findings. A future series of experiments should consider replication of the study using participant pools that are culturally distant (Muthukrishna et al., 2020; Banerjee et al., 2021) from the present sample.
We consider only the outcome of a specific economic game, the Trust Game, and indeed only the actions of humans acting as the second player. Although Karpus et al. (2021) have used a range of games to test the outcome of identity on the rate of cooperation and reciprocity, there is further work to be done to assess the impact of personality traits on such outcomes. For example, while Honesty-Humility has been shown to promote active cooperation, Agreeableness is instead the tendency towards reactive cooperation and therefore may manifests itself as non-retaliation (Hilbig et al., 2013). The impact of such traits on cooperation may therefore be different, especially in repeated games (Al-Ubaydli et al., 2016). Indeed, while we focused on a one-shot interaction without communication, it is likely that there will be richer interactions with bot counterparts in real life situations which involve multiple interactions or longer-term engagements (Crandall et al., 2018). The use of different sequential moves games such as the ultimatum game would allow for testing similar hypotheses also for negative reciprocity—that is, punishment of uncooperative behaviour. The research on the role of personality traits in experimental ultimatum games among human-human participants is mixed, and, together with existing findings from human-bots ultimatum games, provides an intriguing area for further exploration for human-machine interactions (Perugini et al., 2003; Lee and Ashton, 2012; Sandoval et al., 2016; Zhao et al., 2016; de Melo et al., 2019).
Finally, there is currently debate on whether, and to which extent, experimental social preferences games predict real-life social behaviours (Galizzi and Navarro-Martinez, 2019). To try to address this external validity question, we conducted this study online, in the same environment where one expects a bot encounter to take place in the real world. However, it is fair to acknowledge that the scenario was still heavily controlled and stylised. To consider whether the outcomes regarding reciprocity manifest in real-world scenarios, it would be helpful to next design a natural field experiment where different groups of participants are observed while naturally interacting with bots (Harrison and List, 2004). For example, a participant may be asked to deal or work with a chatbot to complete a series of additional tasks (Følstad et al., 2021).
The most innovative finding from our study is the evidence that personality traits are just as much a factor in influencing reciprocity between human-bot counterparts as they are in human-human settings. Personality traits do not affect reciprocity differently when interacting with a bot compared to another human partner. This study breaks new ground by extending the outcomes of the human-human-focused literature into the growing field of human-machine interaction, adding the personality traits as a new lens through which to view reciprocity with bots. This new finding is critical to our understanding of human-bot interaction in the real world, with implications for human-robot interaction design.
Our paper creates the foundation for a series of further research. In the field of human-robot interaction, researchers are already investigating human perceptions of, and engagements with, embodied robots, both more generally (Zörner et al., 2021) and as across cultures (Haring et al., 2014). With personality playing such a large role in how humans interact with bots (Robert et al., 2020; Paetzel-Prüsmann et al., 2021), it would be interesting to see whether the outcomes of the present study differ when humans play against embodied robots. If the Fourth Industrial Revolution is truly upon us, the work to understand the underlying basis for human-bot reciprocity is just beginning.
Data availability statement
The datasets presented in this study can be found in online repositories. The names of the repository/repositories and accession number(s) can be found below: https://osf.io/48jgv/?view_only=28d14f4e206541928f3da53fcb0602d7.
Ethics statement
The studies involving humans were approved by London School of Economics and Political Science (reference: 49465). The studies were conducted in accordance with the local legislation and institutional requirements. The participants provided their written informed consent to participate in this study.
Author contributions
NU and MG conceptualised the study and its design, contributed substantially to reviewing and editing the manuscript, and approved the final version for submission. NU developed and implemented the study design, conducted the pilot testing, ran the data collection, conducted formal and quantitative analysis, and drafted the first version of the manuscript. MG supervised the process. All authors contributed to the article and approved the submitted version.
Conflict of interest
NU is Director of Behavioural Insights at the Ropes & Gray Insights Lab.
The remaining author declares that the research was conducted in the absence of any commercial or financial relationships that could be construed as a potential conflict of interest.
Publisher's note
All claims expressed in this article are solely those of the authors and do not necessarily represent those of their affiliated organizations, or those of the publisher, the editors and the reviewers. Any product that may be evaluated in this article, or claim that may be made by its manufacturer, is not guaranteed or endorsed by the publisher.
Supplementary material
The Supplementary Material for this article can be found online at: https://www.frontiersin.org/articles/10.3389/frbhe.2023.1164259/full#supplementary-material
Footnotes
1. ^We use quite interchangeably the terms “AI”, “bot”, “robot”, and “machine” to mean “a machine that has the ability to solve problems that are usually dealt with by…humans…with…natural intelligence” (Carriço, 2018, p. 30; see further, Andresen, 2002).
2. ^Italicised references to traits in this paper refer to the factors of the HEXACO Model. Terms used for discussion of a more general concept are not capitalised or italicised. Openness to Experience is shortened to Openness for the remainder of this paper.
3. ^The mixed result for the four-player Public Goods Game is less relevant for this study but worth reporting (r=.17, p=.003 for humans but r=.01, p=.794 for bots).
4. ^https://osf.io/48jgv/?view_only=28d14f4e206541928f3da53fcb0602d7
5. ^An entry by one participant reporting their age to be “3” was re-coded as missing data.
References
Adam, M. T. P., Teubner, T., and Gimpel, H. (2018). No rage against the machine: how computer agents mitigate human emotional processes in electronic negotiations. Group Dec. Negot. 27, 543–571. doi: 10.1007/s10726-018-9579-5
Agrawal, A., Gans, J., and Goldfarb, A. (eds)., (2019). The Economics of Artificial Intelligence: An Agenda. Chicago, IL: University of Chicago Press (National Bureau of Economic Research Conference Report). Available online at: https://press.uchicago.edu/ucp/books/book/chicago/E/bo35780726.html (accessed August 25, 2022).
Algan, Y., and Cahuc, P. (2014). “Chapter 2—Trust, Growth, and Well-Being: New Evidence and Policy Implications,” in Handbook of Economic Growth, eds P. Aghion and S.N. Durlauf (Elsevier), pp. 49–120.
Alós-Ferrer, C., and Farolfi, F. (2019). Trust games and beyond. Front. Neurosci 13, 887. doi: 10.3389/fnins.2019.00887
Alós-Ferrer, C., and Ritzberger, K. (2016). The Theory of Extensive Form Games. 1st edn. (Springer Series in Game Theory).
Al-Samarraie, H., Sarsam, S. M., Alzahrani, A. I., and Alalwan, N. (2018). Personality and individual differences: the potential of using preferences for visual stimuli to predict the Big Five traits. Cogn. Technol. Work 20, 337–349. doi: 10.1007/s10111-018-0470-6
Al-Ubaydli, O., Jones, G., and Weel, J. (2016). Average player traits as predictors of cooperation in a repeated prisoner's dilemma. J. Behav. Exp. Econ. 64, 50–60. doi: 10.1016/j.socec.10005
Alves, T., Natálio, J., Henriques-Calado, J., and Gama, S. (2020). Incorporating personality in user interface design: a review. Personal. Individ. Diff. 155, 109709. doi: 10.1016/j.paid.2019.109709
Andreoni, J. (1988). Privately provided public goods in a large economy: the limits of altruism. J. Public Econ. 35, 57–73. doi: 10.1016/0047-2727(88)90061-8
Andresen, S. L. (2002). John McCarthy: father of AI. Intelligent Sys. IEEE, 17, 84–85. doi: 10.1109/MIS.2002.1039837
Anwyl-Irvine, A. L., Massonnié, J., Flitton, A., Kirkham, N., and Evershed, J. K. (2020). Gorilla in our midst: an online behavioral experiment builder. Behav. Res. Methods 52, 388–407. doi: 10.3758/s13428-019-01237-x
Arechar, A. A., Gächter, S., and Molleman, L. (2018). Conducting interactive experiments online. Exp. Econ. 21, 99–131. doi: 10.1007/s10683-017-9527-2
Ashraf, N., Bohnet, I., and Piankov, N. (2006). Decomposing trust and trustworthiness. Exp. Econ. 9, 193–208. doi: 10.1007/s10683-006-9122-4
Ashton, M. C., and Lee, K. (2009). The HEXACO-60: a short measure of the major dimensions of personality. J. Personal. Assess. 91, 340–345. doi: 10.1080/00223890902935878
Banerjee, S., Galizzi, M. M., and Hortala-Vallve, R. (2021). Trusting the trust game: an external validity analysis with a UK representative sample. Games 12, 66. doi: 10.3390/g12030066
Bardsley, N., Cubitt, R., Loomes, G., Moffatt, P., Starmer, C., and Sugden, R. (2009). Experimental Economics: Rethinking the Rules, Experimental Economics. Princeton University Press. doi: 10.1515/9781400831432
Bashirpour Bonab, A., Rudko, I., and Bellini, F. (2021). “A review and a proposal about socio-economic impacts of artificial intelligence,” in Business Revolution in a Digital Era: 14th International Conference on Business Excellence, ICBE 2020, Bucharest, Romania 2021 (Springer International Publishing) (pp. 251-270). doi: 10.1007./978-3-030-59972-0_18
Becker, A., Deckers, T., Dohmen, T., Falk, A., and Kosse, F. (2012). The Relationship between Economic Preferences and Psychological Personality Measures. SSRN Scholarly Paper ID 2042458. Rochester, NY: Social Science Research Network. Available at: https://papers.ssrn.com/abstract=2042458 (accessed October 3, 2021).
Ben-Ner, A., and Halldorsson, F. (2010). Trusting and trustworthiness: what are they, how to measure them, and what affects them. J. Econ. Psychol. 31, 64–79. doi: 10.1016/j.joep.10001
Berg, J., Dickhaut, J., and McCabe, K. (1995). Trust, reciprocity, and social history. Games Econ. Behav. 10, 122–142. doi: 10.1006/game.1995.1027
Bernotat, J., and Eyssel, F. A. (2017). A robot at home—How affect, technology commitment, and personality traits influence user experience in an intelligent Robotics Apartment. Proceedings of the 26th IEEE International Symposium on Robot and Human Interactive Communication (RO-MAN). [Preprint]. Available online at: https://pub.uni-bielefeld.de/record/2914078 (accessed October 3, 2021).
Bohnet, I., Greig, F., Herrmann, B., and Zeckhauser, R. (2008). Betrayal aversion: evidence from Brazil, China, Oman, Switzerland, Turkey, and the United States. Am. Econ. Rev. 98, 294–310. doi: 10.1257/aer.98.1.294
Borghans, L., Duckworth, A. L., Heckman, J. J., and Ter Weel, B. (2008). The economics and psychology of personal traits. J. Human Res. 43, 17. doi: 10.1353./jhr.2008.0017
Borghans, L., and Schils, T. (2021). “Chapter 20—An economic approach to modeling personality,” in Measuring and Modeling Persons and Situations, ed D. Wood. (Academic Press), pp. 675–697.
Brandts, J., and Charness, G. (2000). Hot vs. cold: sequential responses and preference stability in experimental games. Exp. Econ. 2, 227–238. doi: 10.1023/A:1009962612354
Brandts, J., and Charness, G. (2011). The strategy vs. the direct-response method: a first survey of experimental comparisons. Exp. Econ. 14, 375–398. doi: 10.1007/s10683-011-9272-x
Brosig, J., Weimann, J., and Yang, C. L. (2003). The hot vs. cold effect in a simple bargaining experiment. Exp. Econ. 6, 75–90. doi: 10.1023/A:1024204826499
Burks, S. V., Carpenter, J. P., and Verhoogen, E. (2003). Playing both roles in the trust game. J. Econ. Behav. Org. 51, 195–216. doi: 10.1016/S0167-2681(02)00093-8
Camerer, C. F. (2003). Behavioral Game Theory: Experiments in Strategic Interaction. New York, NY, US: Russell Sage Foundation (Behavioral game theory: Experiments in strategic interaction), pp. xv, 550.
Camerer, C. F., and Thaler, R. H. (1995). Anomalies: ultimatums, dictators and manners. J. Econ. Perspect. 9, 209–219. doi: 10.1257/jep.9.2.209
Carriço, G. (2018). The EU and artificial intelligence: a human-centred perspective. Eur. View 17, 29–36. doi: 10.1177/1781685818764821
Casari, M., and Cason, T. (2009). The strategy method lowers measured trustworthy behavior. Econ. Lett. 103, 157–159. doi: 10.1016/j.econlet.2009.03.012
Chandler, J., Mueller, P., and Paolacci, G. (2014). Non-naïveté among Amazon mechanical turk workers: consequences and solutions for behavioral researchers. Behavior Research Methods 46, 112–130. doi: 10.3758/s13428-013-0365-7
Charness, G., and Rabin, M. (2002). Understanding social preferences with simple tests. Quart. J. Econ. 117, 817–869. doi: 10.1162/003355302760193904
Chee, B. T. T., Taezoon, P., Xu, Q., Ng, J., and Tan, O. (2012). Personality of social robots perceived through the appearance. Work, 41, 272–276. doi: 10.3233./WOR-2012-0168-272
Chugunova, M., and Sele, D. (2022). We and It: An interdisciplinary review of the experimental evidence on how humans interact with machines. J. Behav. Exp. Econom. 99, 101897.
Cobb-Clark, D. A., and Schurer, S. (2012). The stability of big-five personality traits. Econ. Lett. 115, 11–15. doi: 10.1016/j.econlet.11015
Cohen, J. (2013). Statistical Power Analysis for the Behavioral Sciences. New York, NY: Academic Press.
Cominelli, L., Feri, F., Garofalo, R., Giannetti, C., Meléndez-Jiménez, M. A., Greco, A., et al. (2021). Promises and trust in human–robot interaction. Sci. Rep. 11, 9687. doi: 10.1038/s41598-021-88622-9
Cooper, W. H., and Withey, M. J. (2009). The strong situation hypothesis. Personal. Soc. Psychol. Rev. 13, 62–72. doi: 10.1177/1088868308329378
Corgnet, B., Hernán-Gonzalez, R., and Mateo, R. (2019). Rac(g)e Against the Machine? Social Incentives When Humans Meet Robots. Working Papers. Available online at: https://ideas.repec.org//p/hal/wpaper/halshs-01994021.html (accessed April 18, 2023).
Cox, C., Karam, A., and Murphy, R. (2017). Social preferences and cooperation in simple social dilemma games. J. Behav. Exp. Econom. 69, 1–3. doi: 10.1016/j.socec.2017.05.002
Cox, J. C. (2004). How to identify trust and reciprocity. Games Econ. Behav. 46, 260–281. doi: 10.1016/S0899-8256(03)00119-2
Crandall, J. W., Oudah, M. Tennom, Ishowo-Oloko, F., Abdallah, S., Bonnefon, J. F., et al. (2018). Cooperating with machines. Nat. Commun. 9, 8. doi: 10.1038./s41467-017-02597-8
Dafoe, A., Bachrach, Y., Hadfield, G., Horvitz, E., Larson, K., Graepel, T., et al. (2021). Cooperative AI: machines must learn to find common ground. Nature 593, 33–36. doi: 10.1038/d41586-021-01170-0
de Melo, C., Marsella, S., and Gratch, J. (2019). Human cooperation when acting through autonomous machines. PNAS Proceed. Nat. Acad. Sci. USA, 116, 3482–3487. doi: 10.1073/pnas.1817656116
de Melo, C. M., Marsella, S., and Gratch, J. (2016). People do not feel guilty about exploiting machines. ACM Transact. Comp. Human Interact. 23, 1–17. doi: 10.1145/2890495
Denissen, J. J., Bleidorn, W., Hennecke, M., Luhmann, M., Orth, U., Specht, J., et al. (2018). Uncovering the power of personality to shape income. Psychol. Sci. 29, 3–13. doi: 10.1177/0956797617724435.
Digman, J. M. (1990). Personality structure: emergence of the five-factor model. Ann. Review Psychol. 41, 417–440. doi: 10.1146/annurev.ps.41.020190.002221
Dijk, E., and De Dreu, C. (2020). Experimental games and social decision making. Ann.Review Psychol. 72, 718. doi: 10.1146./annurev-psych-081420-110718
Dunn, T. J., Baguley, T., and Brunsden, V. (2014). From alpha to omega: a practical solution to the pervasive problem of internal consistency estimation. Br. J. Psychol. 4, 46. doi: 10.1111./bjop.12046
Erlei, A., Nekdem, F., Meub, L., Anand, A., and Gadiraju, U. (2020). Impact of algorithmic decision making on human behavior: evidence from ultimatum bargaining. In Proceedings of the AAAI Conference on Human Computation and Crowdsourcing (8, 43–52.).
Ermisch, J., Gambetta, D., Laurie, H., Siedler, T., and Noah Uhrig, S. C. (2009). Measuring people's trust. J. Royal Stat. Soc. Series A (Stati. Soc.), 172, 749–769. doi: 10.1111/j.1467-985X.2009.00591.x
Faber, M., Bützler, J., and Schlick, C. M. (2015). (2015). Human-robot cooperation in future production systems: analysis of requirements for designing an ergonomic work system. Proced. Manufact. 3, 510–517. doi: 10.1016/j.promfg.07215
Farjam, M., and Kirchkamp, O. (2018). Bubbles in hybrid markets: how expectations about algorithmic trading affect human trading. J. Econ. Behav. Organ. 146, 248–269. doi: 10.1016/j.jebo.11011
Faul, F., Erdfelder, E., Buchner, A., and Lang, A. G. (2009). Statistical power analyses using G*Power 3.1: tests for correlation and regression analyses. Behav. Res. Methods 41, 1149–1160. doi: 10.3758/BRM.41.4.1149
Fehr, E. (2009). (2009). On the economics and biology of trust. J. Eur. Econ. 7, 235–266. doi: 10.1162/JEEA.72–3.235
Fehr, E., and Gächter, S. (2000). Cooperation and punishment in public goods experiments. Am. Econ. Rev. 90, 980–994. doi: 10.1257/aer.90.4.980
Fehr, E., and Gächter, S. (2002). Altruistic punishment in humans. Nature 415, 137–140. doi: 10.1038/415137a
Foehr, J., and Germelmann, C. C. (2020). Alexa, can I trust you? Exploring consumer paths to trust in smart voice-interaction technologies. J. Assoc. Cons. Res. 5, 181–205. doi: 10.1086/707731
Følstad, A., Araujo, T., Law, E. L. C., Brandtzaeg, P. B., Papadopoulos, S., Reis, L., et al. (2021). Future directions for chatbot research: an interdisciplinary research agenda. Computing 103, 2915–2942. doi: 10.1007/s00607-021-01016-7
Funder, D. C., and Ozer, D. J. (2019). Evaluating effect size in psychological research: sense and nonsense. Adv. Methods Pract. Psychol. Sci. 2, 156–168. doi: 10.1177/2515245919847202
Furman, J., and Seamans, R. (2019). AI and the economy. Innov. Policy Econ. 19, 161–191. doi: 10.1086/699936
Galizzi, M. M., and Navarro-Martinez, D. (2019). On the external validity of social preference games: a systematic lab-field study. Manag. Sci. 65, 976–1002. doi: 10.1287/mnsc.2017.2908
García, L. F., Aluja, A., Rossier, J., Ostendorf, F., Glicksohn, J., Oumar, B., et al. (2022). Exploring the stability of HEXACO-60 structure and the association of gender, age, and social position with personality traits across 18 countries. J. Personal. 90, 256–276. doi: 10.1111/jopy.12664
Gignac, G. E., and Szodorai, E. T. (2016). Effect size guidelines for individual differences researchers. Personal. Indiv. Diff. 102, 74–78. doi: 10.1016/j.paid.06069
Glennerster, R., and Takavarasha, K. (2013). Running Randomized Evaluations: A Practical Guide. Illustrated edition. Princeton: Princeton University Press.
Goeree, J. K., and Holt, C. A. (2001). Ten little treasures of game theory and ten intuitive contradictions. Am. Econ. Review, 91, 1402–1422. doi: 10.1257/aer.91.5.1402
Goeree, J. K., and Holt, C. A. (2004). A model of noisy introspection. Games Econ. Behav. 46, 365–382. doi: 10.1016/S0899-8256(03)00145-3
Guilfoos, T., and Kurtz, K. J. (2017). (2017). Evaluating the role of personality trait information in social dilemmas. J. Behav. Exp. Econ. 68119–129. doi: 10.1016/j.socec.04006
Haring, K., Matsumoto, Y., and Watanabe, K. (2013). How Do People Perceive and Trust a Lifelike Robot: 2013 World Congress on Engineering and Computer Science, WCECS 2013. 2013, 425–430.
Haring, K. S., Silvera-Tawil, D., Matsumoto, Y., Velonaki, M., and Watanabe, K. (2014). Perception of an Android Robot in Japan and Australia. Cross-Cultural Comp. 4, 166–175. doi: 10.1007./978-3-319-11973-1_17
Harrison, G. W., and List, J. A. (2004). Field experiments. J. Econ. Lit. 42, 1009–1055. doi: 10.1257/0022051043004577
Hayes, A. F., and Coutts, J. J. (2020). Use omega rather than cronbach's alpha for estimating reliability. Commun. Methods Meas. 14, pp. 1–24. doi: 10.1080/19320201718629
Heckman, J. J., and Rubinstein, Y. (2001). The importance of non-cognitive skills: lessons from the GED testing program. Am. Econ. Rev. 91, 145–149. doi: 10.1257/aer.91.2.145
Henrich, J., Boyd, R., Bowles, S., Camerer, C., Fehr, E., Gintis, H., et al. (2005). “Economic man” in cross-cultural perspective: behavioral experiments in 15 small-scale societies. Behav. Brain Sci. 28, 795–815. doi: 10.1017/S0140525X05000142
Henrich, J., Heine, S. J., and Norenzayan, A. (2010). Most people are not WEIRD. Nature 466, 29–29. doi: 10.1038/466029a
Hilbig, B. E., Zettler, I., Leist, F., and Heydasch, T. (2013). It takes two: honesty–Humility and Agreeableness differentially predict active vs. reactive cooperation. Personal. Indiv. Diff. 54, 598–603. doi: 10.1016/j.paid.11008
Hwang, J., Park, T., and Hwang, W. (2013). The effects of overall robot shape on the emotions invoked in users and the perceived personalities of robot. Appl. Ergon. 44, 459–471. doi: 10.1016/j.apergo.10010
Ishowo-Oloko, F., Bonnefon, J. F., Soroye, Z., Crandall, J., Rahwan, I., Rahwan, T., et al. (2019). Behavioural evidence for a transparency–efficiency tradeoff in human–machine cooperation. Nat. Mach. Intell. 1, 517–521. doi: 10.1038/s42256-019-0113-5
Johnson, N. D., and Mislin, A. A. (2011). Trust games: a meta-analysis. J. Econ. Psychol. 32, 865–889. doi: 10.1016/j.joep.05007
Kagel, J., and Mcgee, P. (2014). Personality and cooperation in finitely repeated prisoner's dilemma games. Econ. Lett. 124, 274–277. doi: 10.1016/j.econlet.05034
Karpus, J., Krüger, A., Verba, J. T., Bahrami, B., and Deroy, O. (2021). Algorithm exploitation: humans are keen to exploit benevolent AI. iScience 24, 102679. doi: 10.1016/j.isci.2021.102679
Kiesler, S., Sproull, L., and Waters, K. (1996). A prisoner's dilemma experiment on cooperation with people and human-like computers. J. Personal. Soc. Psychol. 70, 47–65. doi: 10.1037//0022-3514.70.1.47
Kirchkamp, O., and Strobel, C. (2019). (2019). Sharing responsibility with a machine. J. Behav. Exp. Econ. 80, 25–33. doi: 10.1016/j.socec.02010
Klockmann, V., Von Schenk, A., and Villeval, M. C. (2022). (2022). Artificial intelligence, ethics, and intergenerational responsibility. J. Econ. Behav. Org. 203, 284–317. doi: 10.1016/j.jebo.09010
Köbis, N., Bonnefon, J. F., and Rahwan, I. (2021). Bad machines corrupt good morals. Nat. Human Behav. 5, 679–685. doi: 10.1038/s41562-021-01128-2
Lawn, E. C., Zhao, K., Laham, S. M., and Smillie, L. D. (2021). Prosociality beyond big five agreeableness and HEXACO honesty-humility: is openness/intellect associated with cooperativeness in the public goods game?. Eur. J. Personal. 5, 1. doi: 10.25384./SAGE.c.5498187.v1
Lee, J. D., and See, K. A. (2004). Trust in automation: designing for appropriate reliance. Human Fact. 46, 50–80. doi: 10.1518/hfes.46.1.50_30392
Lee, K., and Ashton, M. C. (2004). Psychometric properties of the HEXACO personality inventory. Multiv. Behav. Res. 39, 329–358. doi: 10.1207/s15327906mbr3902_8
Lee, K., and Ashton, M. C. (2012). Getting mad and getting even: agreeableness and Honesty-Humility as predictors of revenge intentions. Personal. Indiv. Diff. 52, 596–600. doi: 10.1016/j.paid.12004
Lee, K., and Ashton, M. C. (2018). Psychometric properties of the HEXACO-100. Assessment. 25, 543–556. doi: 10.1177/1073191116659134
LePine, J. A., and Van Dyne, L. (2001). Voice and cooperative behavior as contrasting forms of contextual performance: evidence of differential relationships with Big Five personality characteristics and cognitive ability. J. Appl. Psychol. 86, 326–336. doi: 10.1037/0021-9010.86.2.326
List, J. A. (2007). On the interpretation of giving in dictator games. J. Polit. Econ. 115, 482–493. doi: 10.1086/519249
Liu, C. (2010). “Human-machine trust interaction: a technical overview.” in Trust modeling and Management in Digital Environments: From Social Concept to System Development. Hershey, PA, US: Information Science Reference/IGI Global, pp. 471–486.
Lorenz, T., Weiss, A., and Hirche, S. (2016). Synchrony and reciprocity: key mechanisms for social companion robots in therapy and care. Int. J. Soc. Robot. 8, 8. doi: 10.1007./s12369-015-0325-8
Makridakis, S. (2017). (2017). The Forthcoming Artificial Intelligence (AI). Revolut. Impact Soc. Fir Fut. 4, 6. doi: 10.1016/j.futures.03006
March, C. (2021). Strategic interactions between humans and artificial intelligence: lessons from experiments with computer players. J. Econ. Psychol. 87, 102426. doi: 10.1016/j.joep.2021.102426
McCrae, R. R., and Costa, P. T. (1997). Personality trait structure as a human universal. Am. Psychol. 52, 509–516. doi: 10.1037//0003-066x.52.5.509
Mischel, W. (1977). “The interaction of person and situation,” in Personality at the Crossroads: Current Issues in Interactional Psychology, eds Magnusson, D., and Endler, N. S. Hillsdale, (New Jersey: Lawrence Erlbaum Associates), pp. 333–352.
Moon, Y. (2003). Don't Blame the computer: when self-disclosure moderates the self-serving bias. J. Cons. Psychol. 13, 125–137. doi: 10.1207/S15327663JCP13-1and2_11
Moon, Y., and Nass, C. (1998). Are computers scapegoats? Attributions of responsibility in human–computer interaction. Int. J. Human-Comp. Stud. 49, 79–94. doi: 10.1006/ijhc.1998.0199
Morelli, M., Chirumbolo, A., Bianchi, D., Baiocco, R., Cattelino, E., Laghi, F., et al. (2020). The role of HEXACO personality traits in different kinds of sexting: a cross-cultural study in 10 countries. Comp. Human Behav. 113, 106502. doi: 10.1016./j.chb.2020.106502/
Mota, R. C. R., Rea, D. J., Le Tran, A., Young, J. E., Sharlin, E., Sousa, M. C., et al. (2016). “Playing the ‘trust game'with robots: Social strategies and experiences,” in 2016 25th IEEE International Symposium on Robot and Human Interactive Communication (RO-MAN) (IEEE), pp. 519-524. doi: 10.1109./ROMAN.2016.7745167
Müller, J., and Schwieren, C. (2020). Big Five personality factors in the Trust Game. J. Business Econ. 5, 90. doi: 10.1007./s11573-019-00928-3
Munafò, M. R., Nosek, B. A., Bishop, D. V., Button, K. S., Chambers, C. D., et al. (2017). A manifesto for reproducible science. Nat. Human Behav. 1, 1–9. doi: 10.1038/s41562-016-0021
Muthukrishna, M., Bell, A. V., Henrich, J., Curtin, C. M., Gedranovich, A., McInerney, J., et al. (2020). Beyond Western, educated, industrial, rich, and democratic (WEIRD). Psychology: measuring and mapping scales of cultural and psychological distance. Psychol. Sci. 31, 678–701. doi: 10.1177/0956797620916782
Nielsen, Y. A., Thielmann, I., Zettler, I., and Pfattheicher, S. (2021). Sharing money with humans vs. computers: on the role of honesty-humility and (non-)social preferences. Soc. Psychol. Personal. Sci. 5, 19485506211055624. doi: 10.1177./19485506211055622
Nowak, M. A. (2006). Five rules for the evolution of cooperation. Science 314, 1560–1563. doi: 10.1126/science.1133755
Nyholm, S., and Smids, J. (2020). Automated cars meet human drivers: responsible human-robot coordination and the ethics of mixed traffic. Ethics Inform. Technol. 22, 335–344. doi: 10.1007/s10676-018-9445-9
Oksanen, A., Savela, N., Latikka, R., and Koivula, A. (2020). Trust toward robots and artificial intelligence: an experimental approach to human–technology interactions online. Front. Psychol. 11, 8256. doi: 10.3389./fpsyg.2020.568256
Ortmann, A., Fitzgerald, J., and Boeing, C. (2000). Trust, reciprocity, and social history: a re-examination. Exp. Econ. 3, 81–100. doi: 10.1023/A:1009946125005
Oxoby, R. J., and McLeish, K. N. (2004). Sequential decision and strategy vector methods in ultimatum bargaining: evidence on the strength of other-regarding behavior. Econ. Lett. 84, 399–405. doi: 10.1016/j.econlet.03011
Paetzel-Prüsmann, M., Perugia, G., and Castellano, G. (2021). The influence of robot personality on the development of uncanny feelings. Comp. Human. 120, 106756. doi: 10.1016/j.chb.2021.106756
Perugini, M., Gallucci, M., Persaghi, F., and Ercolani, A. P. (2003). The personal norm of reciprocity. Euro. J. Pers. 17, 251–283. doi: 10.1002/per.474
Pothos, E. M., Perry, G., Corr, P. J., Matthew, M. R., and Busemeyer, J. R. (2011). Understanding cooperation in the Prisoner's Dilemma game. Personal. Indiv. Diff. 51, pp. 210–215. doi: 10.1016/j.paid.05002
Proto, E., Rustichini, A., and Sofianos, A. (2019). Intelligence, personality, and gains from cooperation in repeated interactions. J. Polit. Econ. 127, 1351–1390. doi: 10.1086/701355
Rahwan, I., Cebrian, M., Obradovich, N., Bongard, J., Bonnefon, J. F., Breazeal, C., et al. (2019). Machine behaviour. Nature 568, 477–486. doi: 10.1038/s41586-019-1138-y
Robert, L. P Jr., Alahmad, R., Esterwood, C., Kim, S., You, S., and Zhang, Q. (2020). A review of personality in human-robot interactions. Foundation. Trend. Infm. Syst. 4, 107–212. doi: 10.1561/2900000018
Rustichini, A., DeYoung, C. G., Anderson, J. E., and Burks, S. V. (2016). (2016). Toward the integration of personality theory and decision theory in explaining economic behavior: an experimental investigation. J. Behav. Exp. Econ. 64, 122–137. doi: 10.1016/j.socec.04019
Sandoval, E. B., Brandstetter, J., Obaid, M., and Bartneck, C. (2016). Reciprocity in human-robot interaction: a quantitative approach through the prisoner's dilemma and the ultimatum game. Int. J. Soc. Robot. 8, 303–317. doi: 10.1007/s12369-015-0323-x
Sauter, M., Draschkow, D., and Mack, W. (2020). Building, hosting and recruiting: a brief introduction to running behavioral experiments online. Brain Sci. 10, 251. doi: 10.3390/brainsci10040251
Schepman, A., and Rodway, P. (2020). Initial validation of the general attitudes toward artificial intelligence scale. Comp. Human Behav. Rep 1, 100014. doi: 10.1016/j.chbr.2020.100014
Schniter, E., Shields, T. W., and Sznycer, D. (2020). Trust in humans and robots: economically similar but emotionally different. J. Econ. Psychol. 78, 102253. doi: 10.1016/j.joep.2020.102253
Schwab, K. (2017). The Fourth Industrial Revolution: Klaus Schwab. 1st edition. London, UK u. a: Portfolio Penguin.
Shapiro, D. A. (2009). The role of utility interdependence in public good experiments. Int. J. Game Theory. 38, 81–106. doi: 10.1007/s00182-008-0141-6
Sheridan, T. B. (2019). Extending three existing models to analysis of trust in automation: signal detection, statistical parameter estimation, and model-based control. Human Factors 61, 1162–1170. doi: 10.1177/0018720819829951
Skilton, M., and Hovsepian, F. (2018). The 4th industrial revolution: responding to the impact of artificial intelligence on business. Foresight 21, 318–319. doi: 10.1007./978-3-319-62479-2
Sofianos, A. (2022). Self-reported and revealed trust: experimental evidence. J. Econ. Psychol. 88, 102451. doi: 10.1016/j.joep.2021.102451
Spiel, K., Haimson, O., and Lottridge, D. (2019). How to do better with gender on surveys: a guide for HCI researchers. Interactions 26, 62–65. doi: 10.1145/3338283
Stixrud, J., and Urzua, S. (2006). The effects of cognitive and non-cognitive abilities on labor market outcomes and social behavior. J. Labor Econo. 24, 411–482. doi: 10.1086/504455
Syrdal, D. S., Dautenhahn, K., Woods, S. N., Walters, M. L., and Koay, K. L. (2007). Looking Good? Appearance Preferences and Robot Personality Inferences at Zero Acquaintance. pp. 86–92. Available online at: https://researchprofiles.herts.ac.uk/en/publications/looking-good-appearance-preferences-and-robot-personality-inferen
Takayama, L., and Pantofaru, C. (2009). “Influences on proxemic behaviors in human-robot interaction,” in 2009 IEEE/RSJ International Conference on Intelligent Robots and Systems. 2009 IEEE/RSJ International Conference on Intelligent Robots and Systems, pp. 5495–5502. doi: 10.1109./IROS.2009.5354145
Thielmann, I., and Hilbig, B. E. (2015). The traits one can trust: dissecting reciprocity and kindness as determinants of trustworthy behavior. Personal. Soc. Psychol. Bullet. 41, 1523–1536. doi: 10.1177/0146167215600530
Thielmann, I., Moshagen, M., Hilbig, B., and Zettler, I. (2021). On the comparability of basic personality models: meta-analytic correspondence, scope, and orthogonality of the big five and HEXACO dimensions. Eur. J. Personal. 4, 08902070211026793. doi: 10.1177./08902070211026793
Thielmann, I., Spadaro, G., and Balliet, D. (2020). Personality and prosocial behavior: a theoretical framework and meta-analysis. Psychol. Bullet. 146, 30–90. doi: 10.1037/bul0000217
Villani, V., Pini, F., Leali, F., and Secchi, C. (2018). (2018). Survey on human–robot collaboration in industrial settings: safety, intuitive interfaces and applications. Mechatronics 55, 248–266. doi: 10.1016/j.mechatronics.02009
von Schenk, A., Klockmann, V., and Köbis, N. (2023). Social Preferences Towards Humans And Machines: A Systematic Experiment on the Role of Machine Payoffs. Rochester, NY. Available online at: https://ssrn.com/abstract=4145868
Whiting, T., Gautam, A., Tye, J., Simmons, M., Henstrom, J., Oudah, M., et al. (2021). Confronting barriers to human-robot cooperation: balancing efficiency and risk in machine behavior. iScience 24, 101963. doi: 10.1016/j.isci.2020.101963
Zhao, K., Ferguson, E., and Smillie, L. D. (2016). Prosocial personality traits differentially predict egalitarianism, generosity, and reciprocity in economic games. Front. Psychol. 7. doi: 10.3389./fpsyg.2016.01137
Zhao, K., and Smillie, L. D. (2015). The role of interpersonal traits in social decision making: exploring sources of behavioral heterogeneity in economic games. Personal. Soc. Psychol. Rev. 19, 277–302. doi: 10.1177/1088868314553709
Zhou, H., and Fishbach, A. (2016). The pitfall of experimenting on the web: how unattended selective attrition leads to surprising (yet false). research conclusions. J. Personal. Soc. Psychol. 111, 493–504. doi: 10.1037/pspa0000056
Keywords: human-AI interaction, reciprocity, personality traits, trust game, human-human and human-machine interactions
Citation: Upadhyaya N and Galizzi MM (2023) In bot we trust? Personality traits and reciprocity in human-bot trust games. Front. Behav. Econ. 2:1164259. doi: 10.3389/frbhe.2023.1164259
Received: 12 February 2023; Accepted: 07 August 2023;
Published: 28 August 2023.
Edited by:
Marina Chugunova, Max Planck Institute for Innovation and Competition, GermanyReviewed by:
Alicia Von Schenk, Julius Maximilian University of Würzburg, GermanyNils Köbis, Max Planck Institute for Human Development, Germany
Copyright © 2023 Upadhyaya and Galizzi. This is an open-access article distributed under the terms of the Creative Commons Attribution License (CC BY). The use, distribution or reproduction in other forums is permitted, provided the original author(s) and the copyright owner(s) are credited and that the original publication in this journal is cited, in accordance with accepted academic practice. No use, distribution or reproduction is permitted which does not comply with these terms.
*Correspondence: Nitish Upadhyaya, nitish.upadhyaya@ropesgray.com