- 1Institute for Health Services Research and Health Economics, Centre for Health and Society, Medical Faculty and University Hospital Düsseldorf, Heinrich-Heine-University Düsseldorf, Düsseldorf, Germany
- 2CINCH (Competent in Competition and Health), Essen, Germany
- 3Institut für Mittelstandsforschung (IfM) Bonn, Bonn, Germany
- 4University of Dortmund (TU), Dortmund, Germany
- 5ETH Zürich, Zurich, Switzerland
This paper investigates how the design of health insurance plans influences individuals' treatment decisions. We derive several hypotheses how the framing of incentives in a plan affects behavior and test these hypotheses in a laboratory experiment. Our results show that rebates are more effective in containing costs, as individuals request significantly less inefficient low-value care under rebates than under cost-sharing. We elicit individuals' degree of loss aversion but find no evidence that loss aversion influences utilization rates. Instead, our results suggest that relative thinking and the difficulty of understanding the dynamic incentive structure drive the design effect.
JEL: I13, I18, D02, D91
1. Introduction
The increasing share of health-care expenditures relative to Gross Domestic Product in most industrialized countries raises questions about the efficient use of societal resources in the health-care market. Economists have pointed out that health insurance leads individuals to request more care than they would if individuals were uninsured and had to pay the full price of care. To reduce this market inefficiency, commonly referred to as moral hazard, economists have long stressed the importance of designing appropriate incentives in health-insurance plans to increase the efficiency of care.1
Cost-sharing through deductibles and co-insurance is regarded as the standard approach to reduce medical spending, and evidence proves its effectiveness in the field.2 Other evidence, however, raises questions about the efficiency of these tools. First, field evidence suggests that cost-sharing reduces health care regardless of the value of care (Brot-Goldberg et al., 2017) and disproportionally affects low-income households (Nilsson and Paul, 2018).3 The efficient use of resources requires a reduction in inefficient care without a reduction of efficient care. Second, dynamic incentives in non-linear contracts undermine the effectiveness of cost-sharing provisions (Keeler et al., 1977; Ellis, 1986). While there is conflicting evidence on the extent to which individuals incorporate dynamic considerations4, these considerations pose a serious challenge to any incentive scheme.
So far, alternative incentive schemes for decreasing moral hazard, such as rebates, have received less attention in the literature.5 Rebates substitute the negative incentives of cost-sharing with a positive incentive scheme by offering a bonus payment that increases if less care is used (Zweifel, 1987). From a theoretical perspective, rebates should provide the same incentives to individuals as deductibles. A vast amount of previous research has analyzed how the framing of incentives shapes individuals choices.6 An important strand of this literature shows that the framing of contracts can affect effort provision.7 In a recent study, Diederich et al. (2021) also find that the framing of donation schemes affects donation behavior. Concerning health insurance contracts, field evidence by Remmerswaal et al. (2019) and Hayen et al. (2021) suggests that cost-sharing leads to lower utilization of health care than rebates. Unfortunately, these studies differ on what causes the different response, and specifically, to what extent it constitutes a mere framing effect as suggested by Johnson et al. (1993).8 Additionally, it remains an open question whether and how the difference in effectiveness of the two incentive schemes translates into possible differences in efficiency.
We contribute to the existing literature by studying the impact of the health insurance design on individuals' utilization of inefficient health care, i.e., low-value health care for which—absent any insurance—the individual costs of treatment exceed the individual benefit. Our experimental approach comes with two major advantages in comparison to the existing literature that relies on field data. First, it enables us to isolate the pure effect of framing insurance incentives as either cost-sharing or rebate. Second, it allows us to unambiguously differentiate between efficient and inefficient low-value care. We can hence directly compare the performance of cost-sharing and rebate plans in reducing inefficient care. Eliciting individuals' risk and loss aversion allows us to link individuals' preferences to their decisions.
In the main experiment, individuals (n = 96) received an initial endowment and faced 52 decision periods. In each period, individuals observed whether they were healthy, suffering from a mild illness or a severe illness. In every period that they turned ill, individuals decided whether or not to receive treatment. Leaving a mild (severe) disease untreated resulted in a penalty that was lower than (identical to) the treatment costs. While treating either illness was associated with identical cost, the presentation of these costs varied according to the design of health insurance plans. In the cost-sharing condition, subjects paid the treatment cost out of their endowment until the deductible was reached, corresponding to out of pocket expenses in the field. In the rebate condition, the treatment costs were deducted from a bonus promised to be paid at the end of the 52 periods and equally sized in monetary terms as the deductible. After the main experiment, we elicited loss aversion and risk preferences each by means of an incentivized price list.
We find lower health care utilization rates under the rebate frame in comparison to the cost-sharing frame. The difference is particularly striking for the inefficient low-value care. Whereas, subjects chose to request care in 88% of the cases of a mild disease under cost-sharing, this rate dropped by 46 percentage points under rebates. Hence, rebates seem particularly effective in decreasing exactly those expenses that an incentive scheme is intended to reduce. We also find a significantly lower rate of health care utilization under rebates than deductibles for the high-value treatment. Yet, the difference in absolute terms is smaller than for low-value care with 8 percentage points (100% under cost-sharing vs. 92% under rebates). We find no evidence that loss aversion influences health care utilization decisions. In contrast, our results are consistent with other behavioral explanations such as relative thinking and the transparency of dynamic incentives varying between the cost-sharing and the rebate design. Intuitively, the rebate design induces individuals to use a separate mental account to evaluate the cost of treatment. Whereas, treatment costs are small relative to an individual's entire endowment, they are large relative to the remaining bonus. Hence, the cost of treatment is overweighted under a rebate design. In addition, individuals might have more difficulties to understand the dynamic incentives under rebates than cost-sharing. It is easier to understand that it can be desirable to exhaust the deductible as this makes any future treatment free of charge. It is less straightforward to see that, by the same reasoning, it can be desirable to exhaust the rebate as this means that any future treatment has no opportunity cost. Overall, our findings are markedly different from the common finding in the field that rebates lead to more health care utilization. Our results indicate that channels different from framing drive this observation. In addition, our results raise doubts that the reduced health care utilization under cost-sharing that is observed in the field is a sign that only inefficient low-value care is reduced.
The remainder of this article is as follows. The next section offers competing hypotheses on the effect of a change in incentive design. Section 3 introduces the experimental design. Section 4 presents the results. The results are discussed in Section 5 before we conclude in Section 6.
2. Hypotheses
Consider a decision-maker (DM) who faces T periods in which she could be either healthy or sick. She experiences a mild sickness with probability πl and a severe sickness with probability πh, with 0 < πl+πh < 1.9 Treatment is associated with medical expenses of c. Foregoing treatment is associated with health cost vl<c in case of a mild sickness and with health cost vh = c in case of a severe sickness. The health state is determined independently for each period.
The DM is insured. In the cost-sharing (CS) design, the DM has an endowment of E+D, but has to pay any medical expense c out of pocket until the deductible D is reached. After the deductible D is reached, the insurance covers all medical expenses. In the rebate/bonus (R) design, the DM starts with an endowment of E, but insurance covers all medical expenses of the DM unconditionally. However, a bonus is promised to be paid at the end of the T accounting periods. The maximum bonus is D and any medical expense is deducted from the bonus until it is exhausted. If cumulative medical expenses exceed D, then no bonus is paid.
2.1. Standard preferences
The DM anticipates her own future treatment choices and includes both their consequences on present incentives and the consequences of present decisions on future decisions. This means that she compares the value v of treating in a given period to the “effective price” ce∈[0, c] of treating in a given period (Keeler et al., 1977). This effective price falls below the “spot price” c because the potential of future sickness means that there is a positive probability that the deductible is reached/the bonus is exhausted irrespective of today's treatment decision.10 Denote by δ = c−ce the deviation from the “spot price” c of treatment due to dynamic incentives. Then it is worthwhile to seek treatment if and only if
The inequality always holds in case of a severe sickness (vh = c). It holds for a mild case of sickness if and only if δ is sufficiently large.
Given that the cost-sharing regime and the rebate regime only differ in whether the amount D is framed either as being part of the endowment or as a segregated bonus, a DM whose decisions are unaffected by framing shows the same behavior in both regimes.
Hypothesis 1. There is no difference in treatment behavior between the cost-sharing and the rebate design.
Several behavioral influences may result in the decision-process being distorted from this optimal decision rule. We distinguish three behavioral channels that could be affected by our change in the design.
2.2. Status-quo loss aversion
The DM could be loss-averse and overweight any deterioration in comparison to the status quo with a loss parameter λ>1 (Johnson et al., 1993). The cost of an untreated disease v is directly deducted from the endowment, and thus constitutes a deterioration of the status quo. In contrast, the dynamic incentives δ do not influence the status quo but are a function of expectations about future behavior and payoffs. It is only the effect of the “spot price” c that depends on the framing. In the CS design, it is directly deducted from the endowment, and thus paying for treatment constitutes a deterioration in comparison to the status quo. In the R design, it is deducted from a (possible) future bonus and thus constitutes a foregone gain instead of a loss relative to the status quo. A loss-averse DM thus seeks treatment in the CS design if and only if
while the loss-averse DM seeks treatment in the R design if and only if
For severe cases (vh = c), loss aversion has no effect in the CS design and increases incentives for treatment in the R design. For mild cases (vl<c), loss aversion decreases incentives for treatment in the CS design and increases incentives for treatment in the R design.
Hypothesis 2. There is more treatment of mild cases in the rebate design compared to the cost-sharing design.
2.3. Mental accounting and relative thinking
Mental accounting refers to the psychological tendency to assign expenses to different accounts that are not perfectly fungible.11 The cost of an untreated disease v and of treatment ce (c) may be evaluated differently depending on the account that they are assigned to. Specifically, the separation of the bonus in the R design may induce a system of mental accounts, that leads people to overweight reductions in the bonus relative to reductions in their overall endowment.12 Confronted with a disease in the cost-sharing design, a loss of v out of the remaining endowment of , where denotes the remaining deductible (endowment net of the deductible), needs to be compared to a loss of c out of the same endowment. Hence, the two losses are attributed to the same mental account and thus weighted the same. In contrast, paying the cost of treatment out of the remaining bonus may feel worse than paying v out of the remaining endowment Ē as the bonus reduction is a larger loss in relative terms. This leads to an overweighting of the cost of treatment in the rebate design.
A relative-thinking DM seeks treatment in the CS design if and only if
while the relative-thinking DM seeks treatment in the R design if and only if
Relative thinking does not distort the decision under CS. In contrast, as long as , it distorts the decision in favor of abstaining from treatment in the R design. Note that this holds for both mild and severe cases of the disease.
Hypothesis 3. There is more treatment of both mild and severe cases in the cost-sharing design compared to the rebate design.
2.4. Transparency of dynamic incentives
The relative ease to understand the dynamic incentives could differ between the rebate and the cost-sharing design. Whether and to what extent people understand dynamic incentives has been subject of much discussion and investigation.13 Yet, even if people are not able to perfectly calculate and identify the dynamic incentive structure in the cost-sharing design, they might have a rough understanding that it is desirable to reach the deductible as early as possible, as this means that any future treatment is free of charge. While this is also true in the rebate design, the “charge” in this design refers to the opportunity cost of a reduced bonus instead of a direct payment. Understanding the dynamic incentive structure in the rebate design requires the counterintuitive insight that it is actually beneficial to exhaust the bonus as quickly as possible because this frees oneself of the opportunity cost of future treatment. The change in design may thus impact the transparency of dynamic incentives. Denote by σf∈[0, 1], f∈{CS, R} a parameter measuring the transparency of the dynamic incentives in the two designs. A salient-thinking DM seeks treatment in a design f if and only if
If σR < σCS ≤ 1, then the incentives for treatment are stronger in the cost-sharing design. Unless σR = 0, treatment rates do not differ with the design for severe cases, while there is more treatment of mild cases in the CS design.
Hypothesis 4. There is more treatment of mild cases in the cost-sharing design compared to the rebate design.
2.5. Full model
A DM may be subject to all of these biases to some degree. A full model would thus find a DM treat if and only if
Where r∈[0, 1] denotes the strength of relative thinking. We assume this parameter to be unaffected by the insurance design. However, the following parameters depend on the design.
measures the weight of the spot price c.
measures the sizes of the mental accounts in which the respective losses fall. Finally, σf measures the transparency of dynamic incentives under design f∈{CS, R} with 0 ≤ σR < σCS ≤ 1.
Table 1 provides an overview of the different behavioral hypotheses and their underlying assumptions.
It is important to recognize that the full model of Equation (7) has enough degrees of freedom that any behavior could be rationalized with it. Our strategy is thus not to test whether observed behavior is consistent with the full model: it will be. Instead, we seek to apply Ockham's razor and find the strongest simplification of the full model that is still able to rationalize our data. For example, if we find no difference between rebates and cost-sharing, the simplest model rejects hypotheses 2–4 in favor of hypothesis 1.14
3. Experimental design
We conducted a laboratory experiment that consisted of two parts. In the first part, subjects faced a choice experiment on health care utilization with dynamic incentives. In the second part, we elicited risk preferences and loss aversion.
3.1. Decision situation
The design of Part 1 closely resembles the one in Harrison et al. (2023), which is inspired by a dynamic model of Aron-Dine et al. (2012) and also similar to Einav et al. (2015) in the Medicare D prescription drug context. The metrics used by Aron-Dine et al. (2012) reflect the ones of their empirical exercise and are thus based on insurance data from the USA. Our experimental parametrization slightly deviates from theirs. In the course of laying out the experimental design in the following, we will argue for which parameters it differs and why we did so, respectively.
Subjects faced 52 iterations (periods), resembling 52 weeks of the year, of the following situation. In each period, one of three events occurred: being in good health (“healthy”), sickness A (“severe sickness”), or sickness B (“mild sickness”).15 The events were drawn from the following distribution known to the participants: “healthy” could occur with probability 0.6, while each sickness could occur with probability 0.2. While in Aron-Dine et al. (2015) the latter was only 0.1, we decided to double it in order to investigate spending behavior of the sicker part of the population that is particularly cost-intensive and hence the primary target group to reduce health care costs from a policy perspective. In the event of “healthy,” the subject did not have to make any decision and moved on to the following period. In the event of sickness, the subject had to choose whether to seek treatment or leave the sickness untreated. Treatment was associated with medical expenses of 50 ECU (experimental currency units), while remaining untreated led to a reduction in the subject's endowment of 50 ECU in case of sickness A and of 30 ECU in case of sickness B. Here we also slightly deviate from Aron-Dine et al. (2012) as the penalty for not treating a mild sickness is lower. In combination; the higher sickness probability and lower penalty for not treating a mild sickness exemplify a setting in which an individual faces strong dynamic incentives to inefficiently use low-value care as the expenses for high-value care alone are likely to exceed the deductible. This particularly reflects the situation of an individual for which the recognition of dynamic incentives has strong implications both for behavior and for its efficiency. This group is of particular interest because of its large potential for efficiency gains from incentivization. For example, Brot-Goldberg et al. (2017) find that 25% of total spending reductions from cost-sharing can be ascribed to consumers who are insured with a deductible and are predictably sick. Furthermore, we deliberately set the same costs for treating and for not treating the severe disease for three reasons. First, it constitutes the most conservative parametrization of what can be considered as high-value care. This allows us to focus on efficiency losses through the consumption of low-value care. Second, this parametrization allows us to compare our results to Harrison et al. (2023). Third, the treatment behavior with respect to the severe disease allows us to discern whether subjects do not realize dynamic incentives at all or whether they only underestimate their size. A failure to treat the mild disease can result both from complete neglect and from an underestimation of the dynamic incentives. In contrast, a failure to treat the severe disease can only result from a complete neglect of dynamic incentives. Given that spot incentives leave the DM indifferent between treating and not treating a severe disease, any recognition of dynamic incentives must tip the balance in favor of treatment.
The treatment decision in a period had no effect on the probability of sickness in the following periods, and subjects were informed of that. The order of health events was drawn prior to the experiment and identical across conditions and subjects. This procedure reduces noise by ruling out that “seasonality effects” due to temporally concentrated health events differ across conditions. It also rules out that comorbidities affect treatment decisions and thus generate different treatment paths across participants. In addition, it allows comparing our results directly to those of Harrison et al. (2023).
Subjects started with an initial endowment and were told that they had insurance coverage. The presentation of the insurance contract and the size of the initial endowment differed across conditions. We implemented two conditions that varied the design of the health insurance plan: a cost-sharing (CS) design and a rebate (R) design. For an overview of the experimental conditions (see Table 2).
In the CS design, subjects started with an initial endowment of 3200 ECU. The insurance contract was described as offering no coverage of medical expenses below a deductible of 600 ECU, and offering full coverage of medical expenses beyond the deductible. That is, if a subject chose to treat a sickness, its endowment was reduced by 50 ECU in case that prior cumulative medical expenses had not yet reached 600 ECU. In case that the deductible had been reached in a prior period, the subject could treat a sickness without any reduction in the endowment.
In the R design, subjects started with an initial endowment of 2600 ECU. The insurance contract was described as offering full coverage of any medical expenses. However, subjects were promised a bonus payment of 600 ECU minus any incurred medical expenses at the end of the 52 periods. The bonus could not fall below zero: if cumulative medical expenses reached 600 ECU, no bonus was paid. That is, if subjects chose to treat a sickness, their bonus was reduced by 50 ECU in case that prior cumulative medical expenses had not yet reached 600 ECU. In case that the bonus was depleted in a prior period, the subject could treat a sickness without any reduction in the bonus.
In both conditions, leaving a sickness untreated resulted in reductions in the endowment of 50 ECU (severe illness) and 30 ECU (mild illness), regardless of the size of the remaining deductible/bonus. After each period, subjects received information about their remaining endowment and the remaining size of the deductible/bonus. The basic difference between the two conditions is thus entirely a difference in framing: 600 ECU were either considered as part of the endowment in CS or called a bonus in R.
To further investigate the source of framing effects, we later compare our data to one cost-sharing condition in Harrison et al. (2023). Here subjects were also insured with a deductible of 600 ECU and faced the same order of treatment decisions over 52 periods. However, instead of an initial endowment, they received an income of 50 ECU in each of the 52 periods.
In Part 2 of the experiment, risk preferences were elicited following Holt and Laury (2002). Subjects made ten binary choices between lotteries in a gain design. In Part 3, we measured subjects' degree of loss aversion following Karle et al. (2015) and Karle et al. (2022), see Section table 1 in the Appendix.16 They employ two price lists, one in the gain domain and one in the mixed domain. Individuals are assumed to have an exponential utility function as proposed by Tversky and Kahneman (1992). Individual k's utility is
Where z denotes the monetary payoff, λk>1 indicates loss aversion, and βk∈(0, 1) the degree of risk aversion (for gains). βk is determined by treating the switching point in the price list for gains as the point of indifference. λk can similarly be inferred from the switching point in the price list for losses based on βk. Based on their degree of loss aversion, we divide individuals into four categories as suggested by Karle et al. (2015), see Table 3.
3.2. Procedure
The experiments were conducted at the Essen Laboratory for Experimental Economics (elfe) in Essen in 2018.17 Sessions lasted about 90 min. Overall, 96 students participated in the experiment, see Table 4 for the sample characteristics. Participants were recruited by the recruiting system ORSEE by Greiner (2015). They were randomly assigned to their cubicles in the laboratory upon arrival. Before each part of the experiment, they received detailed instructions and were given ample time to read and to ask questions. Any questions were answered in private by the same experimenter across all sessions. To ensure participants' understanding of the decision task in each part, they had to answer a set of control questions, and the experiment did not start until everyone had answered the latter correctly. At the end of the experiment, every subject was paid out their payoff from part 1 of the experiment. The earnings were determined by the remaining endowment plus the remaining bonus (if applicable) from the health care utilization decisions. Additionally, each participant was randomly paid out for one decision of either Part 2 or Part 3. The average payoff was 18.90 EUR. Finally, subjects were asked to answer a short questionnaire with questions on demographics and questions related to their behavior in the previous decisions.
4. Results
We report our results based on individual subject decisions. Hence, we consider the average treatment rate of a subject as an independent observation. Comparing differences across conditions, we use the Mann-Whitney-U test if not stated differently. We call results (marginally) significant if p < 0.05 (p < 0.1).
4.1. Aggregate level: Rebates lead to more efficient utilization than cost-sharing
In the aggregate, we find that rebates lead to more efficient utilization of health care than cost-sharing. In particular, we find that subjects always sought treatment for the severe sickness under cost-sharing. The rate of treating the severe sickness is significantly lower (92%) for subjects under rebates than deductibles (p < 0.01). We find even more pronounced differences between the two incentive schemes for the mild sickness (p ≤ 0.001). Whereas subjects sought the inefficient low value care for the mild sickness in 88% of the cases under cost-sharing, a treatment was only requested in 42% of the cases under rebates. Figure 1 illustrates the treatment rates by sickness. As treating the severe sickness is neutral in terms of efficiency whereas treating the mild sickness is inefficient, rebates lead more efficient utilization than cost-sharing.
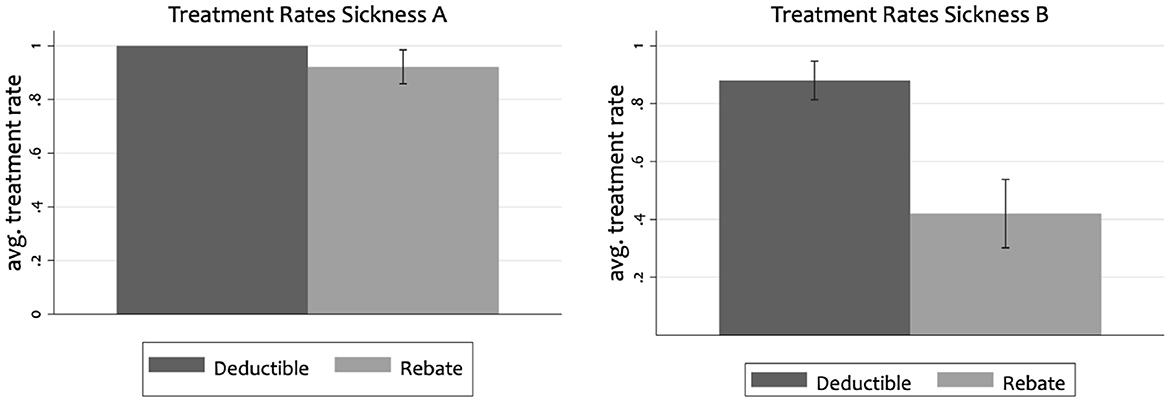
Figure 1. Average treatment rates with confidence intervals for severe sickness (A) and mild sickness (B).
These findings are consistent with subjects constructing separate mental accounts and a reduced transparency of the dynamic incentives in the rebate design (Hypotheses 3 and 4). The cost of treating a disease is higher when compared relative to the rebate amount as when it is compared to the initial endowment. Furthermore, it is more challenging under rebates than cost-sharing to see that performing a treatment liberates oneself from future treatment cost. In contrast, our findings are neither consistent with behavior that is neutral to the design of incentives (Hypothesis 1) nor with a framing effect based on loss aversion (Hypothesis 2). If treatment decisions were driven by loss aversion, we would expect a higher treatment rate for the mild disease under rebates than under cost-sharing.
4.2. Distribution of behavior: Heterogeneity in individuals' utilizations
The average treatment rates across subjects mask a substantial shift in the distribution of individual behavior.
As Figure 2 illustrates, the design of health insurance plans has only small effects on the distribution of behavior for the severe sickness, i.e., the efficiency-neutral treatment. This suggests that our subjects recognized dynamic incentives to treat, but weighted them differently in the CS and R conditions. This lower weight on dynamic incentives induced a stark behavioral change in the treatment of mild diseases. While we find a uni-modal distribution under cost-sharing with a unique mode at the 100% treatment rate, treatment in the rebate condition is characterized by a bimodal distribution with a second mode at the 0% treatment rate. This suggests substantial individual variation in the effect of the design of the health insurance plan. While one group seems hardly affected by the design of health insurance plans, continuing to (almost) always seek treatment for a mild disease, a second group reduces the consumption of inefficient low-value treatment to (almost) zero. The efficiency gains from designing incentives as rebates are thus concentrated in a subgroup of our subject pool.
4.3. Loss aversion and treatment choice: No impact
In contrast to existing field evidence, our loss-aversion task allows us to provide direct evidence on the impact of loss aversion on behavior. We construct our measure using the lottery decisions as brought forward by Karle et al. (2015) and Karle et al. (2022). The loss-aversion measure classifies individuals from gain-seeking or neutral (=1) to strongly loss-averse (=4). We employ an OLS regression to study the impact of loss aversion on the treatment rate, i.e., the share of periods of sickness in which the subjects requested treatment. We control for the experimental condition, gender, age, income, and whether the participants were insured via their parents or self-insured.18 Furthermore, we control for whether an individual is familiar with the concept of expected values.
Table 5 shows the results. The two specifications on the left show the impact on treating the severe sickness. Model (1) only controls for the experimental condition whereas Model (2) also accounts for subject characteristics. The results for the mild sickness are presented in Model (3) and Model (4). We do not find any evidence that loss aversion shapes treatment decisions. All four models indicate that treatment decisions for both sicknesses were independent of our loss-aversion measure.19 Yet, the analysis substantiates that treatment behavior is responsive to the design of health insurance plans. These design differences lead to a significantly higher efficiency in health care utilization under rebates.
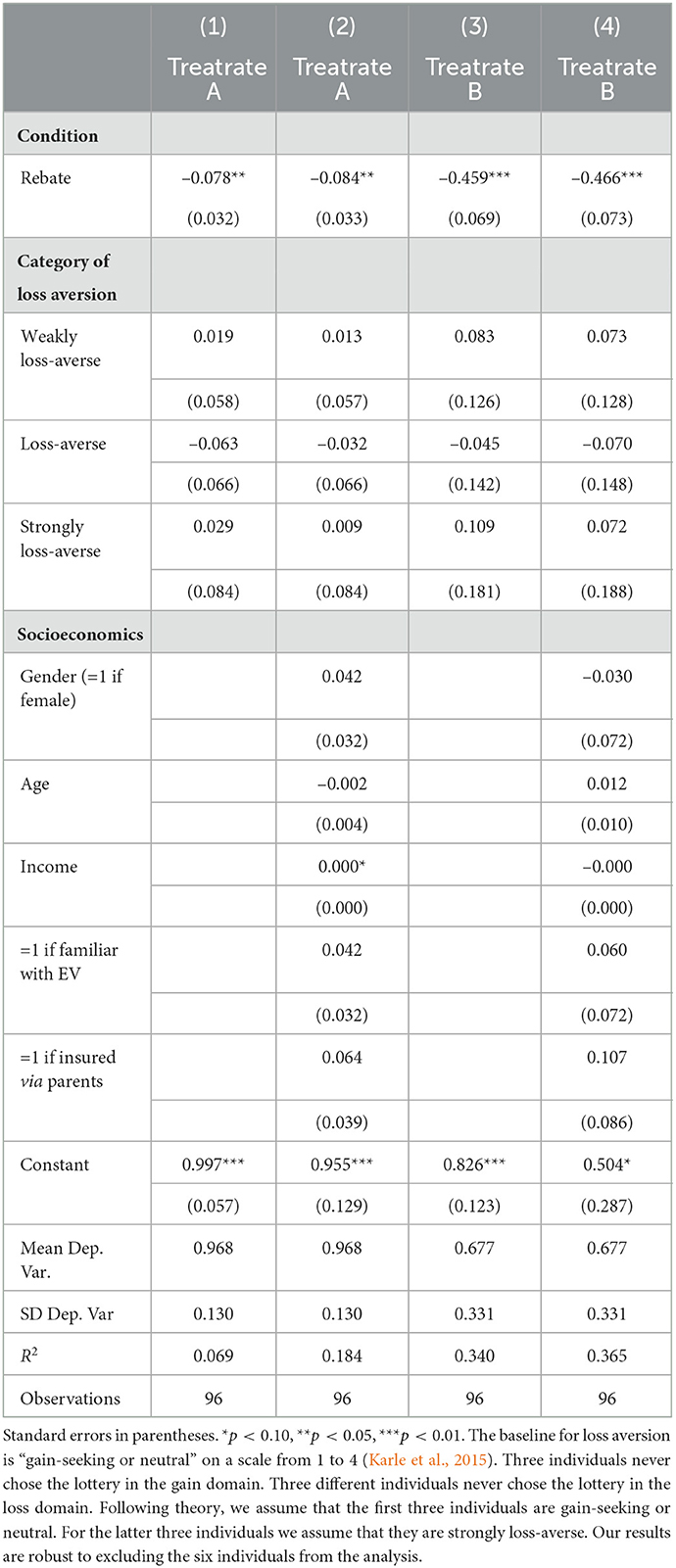
Table 5. OLS regression: Impact of loss aversion on treatment rates (treatrate) for severe sickness (A) and mild sickness (B).
4.4. Differentiating between relative thinking and transparency of dynamic effects
Our findings are consistent with mental accounting/relative thinking (Hypothesis 3) as well as a reduced transparency of the dynamic incentives (Hypothesis 4). To differentiate between the two, we compare our results to Harrison et al. (2023). The authors employ a similar experimental design on cost-sharing. Instead of a fixed initial endowment, subjects received an income stream of 50 ECU in each period. With 52 periods, this is equivalent to a fixed endowment of 2600 ECU, which is below but still comparable to the fixed endowment of 3200 ECU in our cost-sharing treatment. Most importantly, (a) the dynamic incentives δ are identical to both of our treatments, and (b) as in our cost-sharing treatment, relative thinking should not bias decision-making as both the cost and benefit of treatment is associated with the same account: Af(c) = Af(v). Hence, if our results are entirely driven by relative thinking (Hypothesis 3), then we should not see a difference in treatment rates between our cost-sharing treatment and the cost-sharing treatment in Harrison et al. (2023). A difference in treatment rates between the two cost-sharing setups could be caused by a change in the transparency of the dynamic incentives, however. The change from a fixed initial endowment for the entire experiment to a stream of periodic income possibly focuses attention on the spot incentives in every period and thereby reduces the transparency σ of the dynamic incentives δ.20 The comparison of our results to the evidence reported by Harrison et al. (2023) thus embody a variation in design without relative thinking as a possible confound.
Figure 3 complements Figure 1 with the treatment rates in the Cost-Sharing with Periodic Income (CS-PI) condition. Similar to our cost-sharing condition, Harrison et al. (2023) find a treatment rate for the severe sickness of almost 100%. Moreover, Harrison et al. (2023) find a treatment rate of 71% for the mild sickness B while we find it to be 88% in our cost-sharing design. The differences between CS and CS-PI are not significant, while those between CS-PI and R are significant (p < 0.05 for sickness A and p < 0.001 for sickness B). Without significant differences between CS and CS-PI, we cannot shed further light on the relative contribution of the two possible channels.
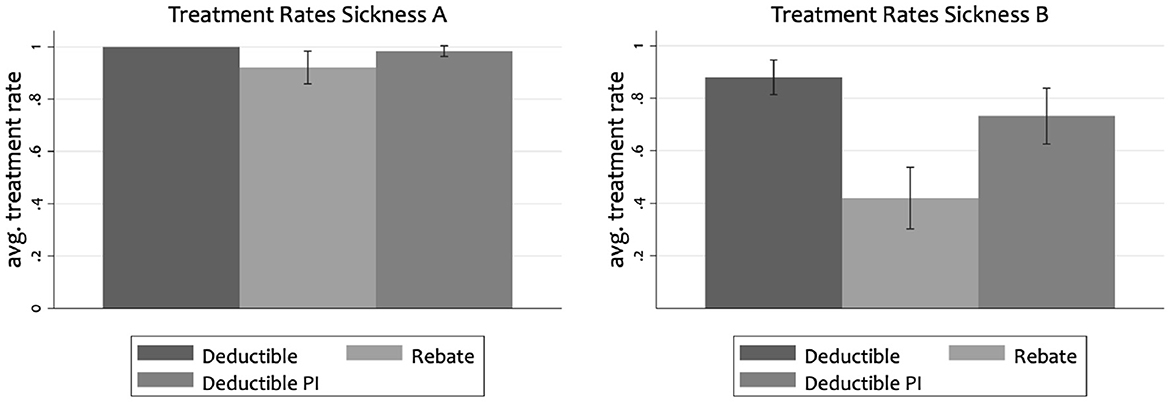
Figure 3. Average treatment rates with conficdence intervals for severe sickness (A) and mild sickness (B). Deductible PI shows results from the cost-sharing condition with periodic income of Harrison et al. (2023).
4.5. Robustness checks
Our findings so far are based on the categorical measure of loss aversion based on Karle et al. (2015). Instead of a categorical measure, we can directly use the degree of loss aversion λ estimated from our choice task. In Section table 2 in the Appendix, we present results using this alternative measure. Again, we find no significant effect of loss aversion on treatment choices.
Another possible confound could be risk preferences apart from loss aversion driving decisions to seek treatment. If subjects' risk preferences differ across conditions, our results may be attributable to differences in risk aversion. In Section table 3 Appendix, we present results in which we control for risk aversion. We find that individuals with severe sickness seek treatment significantly more often than less risk-averse subjects. We find no impact of risk aversion on the likelihood to request treatment for the mild sickness. Importantly, our result of a significant effect of insurance design on the treatment rate remains robust when controlling for risk aversion.
A third concern may arise as observations reflect shares of treatment decisions. To account for these fractional observations, we further employ a fractional probit regression. The results again show no evidence that loss aversion would drive the results (Section table 2 in the Appendix).
5. Discussion
5.1. Interpreting the results with respect to our initial hypotheses
We find lower health care utilization rates under the rebate frame in comparison to the cost-sharing frame. The difference is particularly strong (88 vs. 42%) for inefficient low-value medical expenses, which are exactly the expenses an incentive scheme seeks to reduce. We find no evidence that our measure of loss aversion influences health care utilization decisions. Both of these findings speak against Hypotheses 1 and 2 that suggest either no difference or a larger health care utilization under the rebate frame because people exhibit loss aversion with respect to the status quo. By dropping loss aversion with respect to the status quo, we can thus simplify the general model (Equation 7), by setting λ = 1, without losing explanatory power. In contrast, our evidence favors Hypotheses 3 and 4 that suggest a lower health care utilization under the rebate frame. The two treatments of our experiment on their own cannot distinguish between the two forces—mental accounting/relative thinking and shrouded dynamic incentives—that may lead to the lower health care utilization under the rebate frame. The comparison to results by Harrison et al. (2023) in the previous section unfortunately did not yield further insights that could illuminate the relative importance of the remaining two channels either.
However, the following reasoning explains why we need at least relative thinking to explain our results. It is only possible to rationalize the small but significant decrease in treatment rates for the severe sickness, that we observe in the rebate frame, through relative thinking. It cannot be optimal to abstain from treating a severe case for any value of σR>0 if r = 0. Even if σR = 0, the decision-maker is at most indifferent between treating and not treating the severe sickness. However, if r>0 and σR sufficiently small, a decision-maker strictly prefers to abstain from treating the severe sickness. Hence, a combination of mental accounting and salient thinking best rationalizes our results.
5.2. External validity of our results
A typical concern with results based on experimental data is their external validity. Two issues may be of particular concern in our study. First, our subject pool consists of university students and, hence, includes subjects with an on average higher educational attainment than the general population. Second, while the parameters set within our experimental design allow for a controlled setting to investigate the framing effect of cost-sharing and rebate contracts on health care utilization (and this effect alone), the external validity of our results could be limited due to the particular parameter choices that we made. Although we maintain that our results raise doubts that framing effects are a potential driver of the differences between health care utilization under cost-sharing and rebate plans observed in the field, the strength of the effects that we observe are certainly dependent on the parameters settings within our design. In the following we briefly explain why we set our parameters this way and to which extent they might affect our results.21
The first limitation with regard to external validity is our subject pool consisting of university students. We find that a clear majority of student participants realize the dynamic incentives to consume low-value care when facing a cost-sharing design. With an average treatment rate of 92%, we can confidently say that our subjects understand the dynamic incentives to consume care even if its spot price is high compared to its value. This contrasts with evidence from the field that finds people to respond to the spot prices of care even if they have an extremely low future price (Aron-Dine et al., 2015; Einav et al., 2015; Brot-Goldberg et al., 2017). The stronger recognition of dynamic incentives in our experiment may be a reflection of a better education of our subject pool as compared to the general population. Thus, it is then even more surprising that a majority from this selective subject pool fails to make the individually optimal decision as soon as we switch to a rebate frame. If many of our subjects fail to understand the dynamic incentives under a rebate design, we expect such failure to be even more pronounced in the general population. In conclusion, our experimental results are likely to be a conservative estimate of the effect of a design change in the general population.
Furthermore, the parameters set within our parsimonious experimental design might limit external validity. One assumption we make is that individuals face strong dynamic incentives: they have high probability of reaching the deductible. This high probability of reaching the deductible is modeled by a relatively high probability of sickness leading to an expected number of 20.8 sickness cases within 52 periods. We wanted to create a scenario in which there are strong dynamic incentives to consume low-value care thereby driving a considerable wedge between individual incentives for care consumption and the efficiency of care consumption. Hence, a limitation might be that the majority of the population does not face dynamic incentives that are as large as the ones that we implement in our experimental design. While this is true, the recent literature has pointed to dynamic incentives as a potentially major obstacle to the efficient use of care. This is based on the observation that the majority of health expenditure is produced by a minority of the population that requires extensive medical treatment. This group of users exhibits a large potential for efficiency gains given the intensity of its health care consumption. In addition, it is this very group that faces strong dynamic incentives to use care even if it is of low value. The group of the “predictably sick” thus combines both the potential for large efficiency gains from incentivization and the above-average importance of dynamic incentives. Our results are thus most relevant for the subgroup of the population that indeed faces large dynamic incentives. We expect the difference to be less pronounced for subgroups that face lower dynamic incentives. This is due to the simple reason that the reduced transparency of dynamic incentives through a rebate design has no major impact on individuals who do not face such incentives in the first place.
A further assumption we make is the independent occurrence of sicknesses. We chose diseases to occur independently to simplify the decision problem. Otherwise, people would have been required to update the probability of sickness each period. Given that people often fail at this task, the framing effects that we observe might have been partially confounded with the subjects imperfect updating of probabilities. By modeling an independent occurrence of sickness events, we were able to rule out this possible confound. We do not see how this departure from reality could potentially bias our results. Still, it is an interesting avenue for future research to investigate how well individuals are in updating these probabilities and to which extent it affects their treatment choices.
Moreover, we chose to clearly present costs and benefits of treatment. Of course, costs and particularly benefits of health care are not necessarily evident for individuals in the real world. We consider this departure from the field an actual strength of our experimental approach. For, in the field, it is never quite clear whether the consumption of some type of care is indeed efficient or inefficient. And while there is an entire literature on how to best measure the cost and benefits of health care, we still do not know if the individual who actually makes the choice agrees with the expert opinion. In our experiment, it is perfectly clear that the benefits of treatment never outweigh the cost. Hence, the question of treatment efficiency, and, how the change in framing insurance incentives affect treatment efficiency, are perfectly clear as well. In any case, we do not see how the uncertainty of benefits and costs of treatment could influence the framing effects that we observe.
A further limitation arises from the way we modeled costs and benefits of our two sicknesses. In our experiment, we chose benefits such that treatment is, at best efficiency-neutral, i.e., costs=benefits for the severe sickness. This is the result of our focus on the effect of framing on the consumption of inefficient care. Treatment of sickness A particularly served as a check whether people comprehend dynamic incentives at all. Our analysis does not include a treatment where benefits outweigh costs and decreasing such expenses actually lowers welfare. While the extent to which consumption of care, even under rebates, increases with the benefits of care (from 42 to 92%) leaves us confident that subjects would almost always choose to consume care for which the benefits of health care outweigh its costs, we do not have the data to test this conjecture. Still, it is important to bear in mind, that the overall judgment of the welfare effects of the two frames also depends on the effects on the consumption of efficient care.
Finally, our experiment is limited in terms of external validity since the payment under both frames was made at the end of the experiment. In the real world, bonuses and rebates are paid at the end of an accounting period, while co-payments have to be made closer to the actual consumption of care. In principle, this difference in timing and time preferences may explain some of the observed discrepancy in treatment rates between the two designs in the field. However, our study is deliberately focused on investigating another phenomenon that has also been proposed as possible explanation for differences in utilization: the framing effect. By keeping the timing of payments equal across experimental treatments, we can rule out that any difference that we observe could be a result of time preferences. In this way, we can claim that we observe the pure framing effect. Again, this departure from reality turns out to be an actual advantage of the experimental method as it allows us to isolate a particular effect from possible confounds.
6. Conclusion
In this study we use a controlled laboratory experiment to investigate the behavioral effects of incentive design in insurance contracts. Specifically, we seek to illuminate how the efficiency of treatment decisions by an insured individual varies between different health insurance designs. We elicited participants' loss aversion in a separate incentivized game and study whether loss aversion can explain differences in behavior across designs.
Ruling out liquidity constraints and discounting as possible confounds, we find that rebates are both more effective and more efficient in reducing health spending than cost-sharing. This is in strong contrast to field evidence that finds cost-sharing to be more effective in reducing health spending. It suggests that the discrepancy observed in the field is more than a mere framing effect.22 In addition, the difficulty to differentiate between inefficient low-value and (weakly) efficient high-value care in the field makes it hard to infer the efficiency implications of the observed discrepancy in effectiveness. In contrast, our experimental approach allows us to unambiguously discern the effect of a design change on the efficiency of care consumption.
We do not find significant effects of loss aversion on treatment behavior, a hypothesis proposed by Johnson et al. (1993) that is frequently offered as a possible explanation for the observed differences in the field. Our results suggest that loss aversion is not the main driver behind these differences. In contrast to loss aversion, relative thinking and the transparency of dynamic incentives seem relevant behavioral drivers of the framing effects between rebates and cost-sharing. Future research could further investigate the role of time preferences due to the different timing of payments implied by the cost-sharing and rebate design.
Previous empirical studies such as Brot-Goldberg et al. (2017) have shown that deductibles reduce overall quantities of care. While a reduction in low-value care is desirable from a policy perspective, reducing high-value care is not. Our results show that in the absence of liquidity constraints and discounting, health care utilization rates of high-value care are only slightly lower under rebates than under deductibles whereas there is a substantial reduction in low-value care between the rebate and deductible plans. Rebates achieve this discriminate response by shrouding dynamic incentives to consume low-value care. We thus contribute to the central question of how incentives can be designed to reduce low-value health care selectively. Given our parsimonious experimental design, our behavioral results suggest a rebate plan to be a promising means to raise the efficiency of health-care consumption, not only by encouraging desirable behaviors (Homonoff et al., 2020), but also by discouraging undesirable ones.
Data availability statement
The original contributions presented in the study are included in the article/Supplementary material, further inquiries can be directed to the corresponding author.
Ethics statement
Ethical review and approval was not required for the study on human participants in accordance with the local legislation and institutional requirements. The patients/participants provided their written informed consent to participate in this study.
Author contributions
All authors listed have made a substantial, direct, and intellectual contribution to the work and approved it for publication.
Funding
This study was financial supported by the Bundesministerium für Bildung und Forschung (BMBF, German Federal Ministry of Education and Research) (grant number: 01 EH1602A) is gratefully acknowledged.
Acknowledgments
We thank Glenn Harrison, Heiko Karle, Martin Karlsson, Mathias Kifmann, Hartmut Kliemt, and Dennis Strümpel for their helpful comments and discussions. The authors are grateful for comments by participants at the TIBER symposium in Tilburg, at the Allocation & Distribution Workshop in Konstanz, the Economics Seminar in Münster, the Behavioral Health Economics Workshop in Innsbruck, and the World Risk and Insurance Economics Congress.
Conflict of interest
The authors declare that the research was conducted in the absence of any commercial or financial relationships that could be construed as a potential conflict of interest.
Publisher's note
All claims expressed in this article are solely those of the authors and do not necessarily represent those of their affiliated organizations, or those of the publisher, the editors and the reviewers. Any product that may be evaluated in this article, or claim that may be made by its manufacturer, is not guaranteed or endorsed by the publisher.
Supplementary material
The Supplementary Material for this article can be found online at: https://www.frontiersin.org/articles/10.3389/frbhe.2023.1043188/full#supplementary-material
Footnotes
1. ^Pauly (1968), Zeckhauser (1970), and Arrow (1971).
2. ^Newhouse and The Insurance Experiment Group (1993), Zweifel and Manning (2000), Finkelstein et al. (2012), Aron-Dine et al. (2013), and Einav and Finkelstein (2018).
3. ^See also Siu et al. (1986) and Zweifel and Manning (2000).
4. ^Aron-Dine et al. (2015), Einav et al. (2015), Brot-Goldberg et al. (2017), and Harrison et al. (2023).
5. ^In contrast, rebates are successfully employed by insurers to promote healthy behaviors such as gym visits (Homonoff et al., 2020).
6. ^Tversky and Kahneman (1981), Sonnemans et al. (1998), and Chang et al. (2019).
7. ^See e.g., Christ et al. (2012), Hossain and List (2012), Fryer et al. (2012), Armantier and Boly (2015), and Dellavigna and Pope (2018).
8. ^Individuals may perceive paying an out-of-pocket cost under a deductible as a loss. In contrast, the reduction of a promised bonus/rebate is perceived as a foregone gain. If people are loss averse, they will consider the out-of-pocket payment a heavier burden compared to a similar-sized bonus reduction.
9. ^Note that in the experiment πl = πh < 1.
10. ^ce = 0 if the deductible is already reached/ the bonus is already exhausted. In contrast, ce = c if the remaining deductible/bonus is large enough, such that it cannot be exhausted before the end of the accounting period. Ellis (1986) shows that the effective price is well approximated by the expected “end-of-year” price ptc, where pt∈[0, 1] denotes the probability in period t with which the DM expects not to reach the deductible (exhaust the bonus) before the end of the accounting period.
11. ^Kahneman and Tversky (1984), Thaler (1990), and Thaler (1999).
12. ^For example, see Problem 7 in Kahneman and Tversky (1984).
13. ^Aron-Dine et al. (2015), Einav et al. (2015), Brot-Goldberg et al. (2017), and Harrison et al. (2023).
14. ^An alternative, but hardly simpler, explanation for such evidence could be that hypotheses 2–4 are true, but happen to exactly counterbalance each other.
15. ^The severe (mild) sickness was neutrally labeled as “sickness A(B)” in the experiment.
16. ^Note that we make use of the theoretical foundation of the loss aversion measure by Karle et al. (2015). Our price lists are taken from Karle et al. (2022). In the CS-PI condition of Harrison et al. (2023), loss aversion was not elicited.
17. ^The sessions for the cost-sharing control condition CS-PI were conducted in 2016 by Harrison et al. (2023).
18. ^We included whether the participants were insured via their parents or self-insured as it indicates whether student participants have experience with making treatment choices given their own health insurance or not. Individuals that are still insured via their parents health plans are often fully insured and hence have no experience with insurance incentives such as deductibles or rebates.
19. ^Section 2.2. shows that loss aversion could have a negative effect in the CS condition and a positive effect in the R condition. Our finding of an insignificant effect could thus be the result of these two effects canceling each other out in the pooled data. However, when running separate regressions for each condition, we do not find a significant effect of loss aversion in either of them.
20. ^The comparison to our rebate design is less straightforward. While the periodic income stream may focus attention on the spot incentives, understanding the dynamic incentives is still more difficult in the rebate design. We can thus not differentiate whether the transparency of dynamic incentives is larger or lower in our rebate design as compared to the cost-sharing of Harrison et al. (2023). A direct comparison is also confounded by the possibility of relative thinking possibly distorting incentives in the rebate design.
21. ^See also the motivation of the design in Section 3.
22. ^Remmerswaal et al. (2019) and Fels (2020) offer alternative explanations related to discounting or liquidity/affordability constraints. The importance of recognizing these constraints when determining the effects of insurance incentives such as cost-sharing is corroborated by empirical evidence such as Nilsson and Paul (2018).
References
Armantier, O., Boly, A. (2015). Framing of incentives and effort provision. Int. Econ. Rev. 56, 917–938. doi: 10.1111/iere.12126
Aron-Dine, A., Einav, L., Finkelstein, A. (2013). The rand health insurance experiment, three decades later. J. Econ. Perspect. 27, 197–222. doi: 10.1257/jep.27.1.197
Aron-Dine, A., Einav, L., Finkelstein, A., Cullen, M. (2012). “Moral hazard in health insurance: How important is forward looking behavior?” in NBER Working Paper, Vol. 17802. Available online at: https://www.nber.org/papers/w17802
Aron-Dine, A., Einav, L., Finkelstein, A., Cullen, M. (2015). Moral hazard in health insurance: do dynamic incentives matter? Rev. Econ. Stat. 97, 725–741. doi: 10.1162/REST_a_00518
Brot-Goldberg, Z., Chandra, A., Handel, B., Kolstad, J. (2017). What does a deductible do? the impact of cost-sharing on health care prices, quantities, and spending dynamics. Q. J. Econ. 132, 1261–1318. doi: 10.1093/qje/qjx013
Chang, D., Chen, R., Krupka, E. (2019). Rhetoric matters: a social norms explanation for the anomaly of framing. Games Econ. Behav. 116, 158–178. doi: 10.1016/j.geb.2019.04.011
Christ, M., Sedatole, K., Towry, K. (2012). Sticks and carrots: The effect of contract frame on effort in incomplete contracts. Account. Rev. 87, 1913–1938. doi: 10.2308/accr-50219
Dellavigna, S., Pope, D. (2018). What motivates effort? evidence and expert forecasts. Rev. Econ. Stud. 85, 1029–1069. doi: 10.1093/restud/rdx033
Diederich, J., Eckel, C., Epperson, R., Goeschl, T., Grossman, P. (2021). Subsidizing unit donations: matches, rebates, and discounts compared. Exp. Econ. 697, 1–25. doi: 10.1007/s10683-021-09732-9
Einav, L., Finkelstein, A. (2018). Moral hazard in health insurance: what we know and how we know it. J. Eur. Econ. Assoc. 16, 957–982. doi: 10.1093/jeea/jvy017
Einav, L., Finkelstein, A., Schrimpf, P. (2015). The response of drug expenditure to nonlinear contract design: evidence from medicare part d. Q. J. Econ. 130, 841–899. doi: 10.1093/qje/qjv005
Ellis, R. (1986). Rational behavior in the presence of coverage ceilings and deductibles. Rand. J. Econ. 17, 158–175. doi: 10.2307/2555381
Fels, M. (2020). Incentivizing efficient utilization without reducing access: the case against cost-sharing in insurance. Health Econ. 29, 827–840. doi: 10.1002/hec.4023
Finkelstein, A., Taubman, S., Wright, B., Bernstein, M., Gruber, J., Newhouse, J., et al. (2012). The oregon health insurance experiment: Evidence from the first year. Q. J. Econ. 127, 1057–1106. doi: 10.1093/qje/qjs020
Fryer, R., Levitt, S., List, J., Sadoff, S. (2012). “Enhancing the efficacy of teacher incentives through loss aversion: a field experiment.” in NBER Working Paper, Vol. 18237. Available online at: https://www.nber.org/papers/w18237
Greiner, B. (2015). Subject pool recruitment procedures: organizing experiments with orsee. J. Econ. Sci. Assoc. 1, 114–125. doi: 10.1007/s40881-015-0004-4
Harrison, G. W., Kairies-Schwarz, N., Han, J. (2023). Deductibles and health care utilization: An experiment on the role of forward-looking behavior. CEAR Working Paper, 2023–01.
Hayen, A., Klein, T., Salm, M. (2021). Does the framing of patient cost-sharing incentives matter? the effects of deductibles vs. no-claim refunds. J. Health Econ. 80, 102520. doi: 10.1016/j.jhealeco.2021.102520
Holt, C. A., Laury, S. K. (2002). Risk aversion and incentive effects. Am. Econ. Rev. 92, 1644–1655. doi: 10.1257/000282802762024700
Homonoff, T., Willage, B., Willén, A. (2020). Rebates as incentives: the effects of a gym membership reimbursement program. J. Health Econ. 70, 102285. doi: 10.1016/j.jhealeco.2019.102285
Hossain, T., List, J. (2012). The behavioralist visits the factory: increasing productivity using simple framing manipulations. Manag. Sci. 58, 2151–2167. doi: 10.1287/mnsc.1120.1544
Johnson, E., Hershey, J., Meszaros, J., Kunreuther, H. (1993). Framing, probability distortions, and insurance decisions. J. Risk Uncertain. 7, 35–51. doi: 10.1007/BF01065313
Kahneman, D., Tversky, A. (1984). Choices, values, and frames. Am. Psychol. 39, 341–350. doi: 10.1037/0003-066X.39.4.341
Karle, H., Engelmann, D., Peitz, M. (2022). Student performance and loss aversion. Scandinavian J. Econ, 124, 420–456.
Karle, H., Kirchsteiger, G., Peitz, M. (2015). Loss aversion and consumption choice: theory and experimental evidence. Am. Econ. J. Microecon. 7, 101–120. doi: 10.1257/mic.20130104
Keeler, E., Newhouse, J., Phelps, C. (1977). Deductibles and the demand for medical care services: the theory of a consumer facing a variable price schedule under uncertainty. Econometrica 45, 641–656. doi: 10.2307/1911679
Newhouse J. The Insurance Experiment Group (1993). Free for All: Lessons from the Health Insurance Experiment. Cambridge, MA: Harvard University Press.
Nilsson, A., Paul, A. (2018). Patient cost-sharing, socioeconomic status, and children's health care utilization. J. Health Econ. 59, 109–124. doi: 10.1016/j.jhealeco.2018.03.006
Remmerswaal, M., Boone, J., Bijlsma, M., Douven, R. (2019). Cost-sharing design matters: a comparison of the rebate and deductible healthcare. J. Public Econ. 170, 83–97. doi: 10.1016/j.jpubeco.2019.01.008
Siu, A., Sonnenberg, F., Manning, W., Goldberg, G., Bloomfield, E., Newhouse, J., et al. (1986). Inappropriate use of hospitals in a randomized trial of health insurance plans. N. Engl. J. Med. 315, 1259–1266. doi: 10.1056/NEJM198611133152005
Sonnemans, J., Schram, A., Offerman, T. (1998). Public good provision and public bad prevention: the effect of framing. J. Econ. Behav. Organ. 34, 143–161. doi: 10.1016/S0167-2681(97)00042-5
Thaler, R. H. (1990). Anomalies: saving, fungibility, and mental accounts. J. Econ. Perspect. 4, 193–205. doi: 10.1257/jep.4.1.193
Thaler, R. H. (1999). Mental accounting matters. J. Behav. Decis. Mak. 12, 183–206. doi: 10.1002/(SICI)1099-0771(199909)12:3andlt;183::AID-BDM318andgt;3.0.CO;2-F
Tversky, A., Kahneman, D. (1981). The framing of decisions and the psychology of choice. Science 211, 453–458. doi: 10.1126/science.7455683
Tversky, A., Kahneman, D. (1992). Advances in prospect theory: cumulative representation of uncertainty. J. Risk Uncertain. 5, 297–323. doi: 10.1007/BF00122574
Zeckhauser, R. (1970). Medical insurance: a case study of the tradeoff between risk spreading and appropriate incentives. J. Econ. Theory 2, 10–26. doi: 10.1016/0022-0531(70)90010-4
Zweifel, P. (1987). Bonus systems in health insurance: A microeconomic analysis. Health Policy 7, 273–288. doi: 10.1016/0168-8510(87)90037-6
Keywords: framing, cost-sharing, rebates, loss aversion, relative thinking
Citation: Kairies-Schwarz N, Rieger-Fels M and Waibel C (2023) Cost-sharing or rebate: The impact of health insurance design on reducing inefficient care. Front. Behav. Econ. 2:1043188. doi: 10.3389/frbhe.2023.1043188
Received: 13 September 2022; Accepted: 18 January 2023;
Published: 22 February 2023.
Edited by:
Arthur E. Attema, Erasmus University Rotterdam, NetherlandsReviewed by:
Alexander Koch, Aarhus University, DenmarkFranziska Then, University of Duisburg-Essen, Germany
Copyright © 2023 Kairies-Schwarz, Rieger-Fels and Waibel. This is an open-access article distributed under the terms of the Creative Commons Attribution License (CC BY). The use, distribution or reproduction in other forums is permitted, provided the original author(s) and the copyright owner(s) are credited and that the original publication in this journal is cited, in accordance with accepted academic practice. No use, distribution or reproduction is permitted which does not comply with these terms.
*Correspondence: Nadja Kairies-Schwarz, bmFkamEua2Fpcmllcy1zY2h3YXJ6QHVuaS1kdWVzc2VsZG9yZi5kZQ==