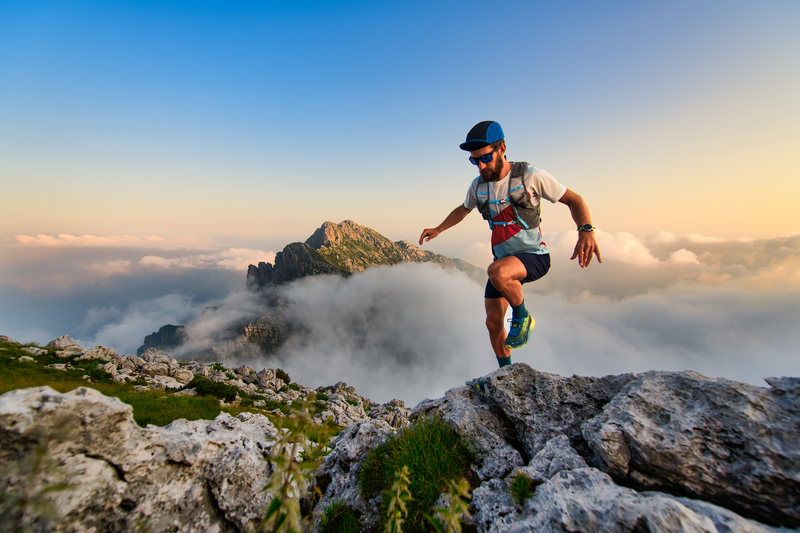
95% of researchers rate our articles as excellent or good
Learn more about the work of our research integrity team to safeguard the quality of each article we publish.
Find out more
PERSPECTIVE article
Front. Bacteriol. , 05 March 2024
Sec. Bacterial Genetics and AI-enhanced Microbial Engineering
Volume 3 - 2024 | https://doi.org/10.3389/fbrio.2024.1334712
Genomic analysis is part of the daily routine for many microbiology researchers. These analyses frequently unveil genes that encode proteins with uncertain functions, and for many bacterial species, these unknown genes constitute a significant proportion of their genomic coding sequences. Because these genes do not have defined functions, they are often overlooked in analyses. Experimentally determining the function of a gene can be challenging; however, ongoing advancements in bioinformatics tools, especially in protein structural analysis, are making it progressively easier to assign functions to hypothetical sequences. Leveraging various complementary tools and automated pipelines for annotating hypothetical sequences could ultimately enhance our comprehension of microbial functions and provide direction for new laboratory experiments.
High-throughput sequencing has made its mark in the biological sciences, and it is now possible to sequence complete genomic DNA samples at low cost. Genomic analyses are currently routine in bacteriology, and can be used, among other things, to identify new organisms (Richter and Rosselló-Móra, 2009) and virulence genes (Li et al., 2018) and to track the spread of antibiotic resistance determinants (Orlek et al., 2023). This explosion in sequences has required the creation of databases to archive, classify, and make genomic data accessible. Some databases—such as the well-known RefSeq (O’Leary et al., 2016), which includes non-redundant, well-annotated sequences—are generalists. Others are specialized and contain sequences from model organisms or organisms that have been extensively studied, such as Pseudomonas (Winsor et al., 2016) or Mycobacterium (Kapopoulou et al., 2011). Finally, some databases, such as EggNOG (Huerta-Cepas et al., 2019) or STRING (Szklarczyk et al., 2015), are geared toward the functional categorization of genes. These latter databases are particularly useful for determining the role of proteins and possibly their interactions in a network. Obviously, functional databases require more precise gene sequence information than generalist databases.
Generally, functional characterization of a gene involves modifying the gene by molecular biology techniques and then observing differences between the mutant strain (with the modified gene) and the parental strain. Although increasingly effective strategies—such as CRISPR-Cas9 (Doudna and Charpentier, 2014)—are available, the characterization of a gene can still be arduous and time-consuming. For example, the integration of the exogenous genetic material into cells, required for genetic manipulation, can be difficult in little-studied organisms for which few or no protocols are available. In some cases, the effect caused by gene alteration may be subtle and difficult to observe. Finally, detecting differences when modifying an essential gene is often impossible because these changes can be fatal for the cell.
Sequence similarity searches using bioinformatics tools such as BLAST (Altschul et al., 1990) or DIAMOND (Buchfink et al., 2015) enable the function of genes to be inferred from their evolutionary proximity to other known genes. This principle of inference underlies all automatic annotation tools, such as Prokka (Seemann, 2014), Bakta (Schwengers et al., 2021), and RAST (Aziz et al., 2008). Homology is also useful for assigning functions to genes in organisms that are difficult to manipulate genetically. However, to assign a function to a gene with these tools, at least one evolutionarily close sequence must already have been characterized and be in the database used.
Following a homology search, it is possible that no homologous sequence is found. This gene is considered an ORFan (Fischer and Eisenberg, 1999), and may be either a chance open reading frame (ORF) that codes for nothing, or a real gene identified for the first time. However, homology searches more commonly identify sequences with no known function. These gene sequences are generally considered to code for hypothetical proteins.
By October 2023, the RefSeq database included approximately 5,000,000 protein sequences from Escherichia coli, one of the most studied organisms. The genome of the reference strain E. coli O157:H7 str. Sakai (RefSeq GCF_000008865.2) contains 5155 protein-coding genes. However, more available genomic sequences obviously means that more genes are listed, because each strain—having a life of its own—may have acquired genes horizontally from other bacteria. By the same date, just over 35,000 genomic assemblies were available for E. coli. In addition to the large number of sequences available, E. coli is known to have an open pan-genome, meaning that it has great facility in acquiring genes from other bacteria in its environment (Rasko et al., 2008).
Of the 5,000,000 protein sequences, approximately 500,000 (10%) correspond to hypothetical proteins. These hypothetical protein sequences vary in length (Figure 1), some being far too long to believe they are from coincidental open reading frames. Several sequences even exceed 1000 amino acids (AAs) long, whereas an average bacterial gene, in general, is around 1000 bp (~333 AAs) long. The longest sequence (RefSeq WP_301221190.1) is 7556 AAs. BLASTP analysis against the nr/nt database identified that the sequence of this protein is also present in different species of Staphylococcus, in addition to E. coli.
Interestingly, a re-annotation of the hypothetical protein sequences with a local installation of the eggNOG-mapper tool (Cantalapiedra et al., 2021) revealed a categorization for 145,225 sequences, i.e., almost 30% of the hypothetical sequences (Figure 2). Unfortunately, the category with the most sequences was “S, function unknown,” indicating that the sequences have several homologs in the EggNOG database, but their functions are also unknown. Despite this, many sequences are thought to be involved in cell membrane biogenesis and cell metabolism. Even if it is impossible to assign a clear function to the sequences in the “S” category, a description is often offered by the tool, which can help to determine a potential role for these proteins. For example, of the 44,441 sequences in the “S” category, 5625 (~12.6%) have the term “phage” in the description, suggesting a viral origin.
Figure 2 The 10 functional categories containing the most E. coli hypothetical protein sequences and annotated by eggNOG-mapper.
Many bioinformatics analyses require the investigation of a multitude of bacterial genes (e.g., mutation screening, differential gene expression). Typically, these analyses generate a list of genes of interest. Obviously, the reflex is to look only at known genes, especially those that might have a link with the reason for the analysis, and assume the hypothetical protein-coding genes are non-existent or negligible from a biological point of view. However, if the analysis has identified these genes, they may be of interest.
Some research groups have demonstrated the value of investigating genes coding for hypothetical proteins. For example, Rahman et al. identified genes involved in the adaptation of Bacillus paralicheniformis and other genes of potential biotechnological interest among the genes coding for hypothetical proteins (Rahman et al., 2022). In 2020, Araújo et al. characterized genes coding for hypothetical proteins that could be involved in the pathogenesis of the bacterium Corynebacterium pseudotuberculosis (Araújo et al., 2020). A 2017 study also demonstrated that characterizing the coding sequences for hypothetical proteins in Mycobacterium tuberculosis, one of the deadliest bacteria in humans, was of interest in providing new therapeutic targets (Raj et al., 2017). Similar discoveries have been made in eukaryotic organisms. For example, Silva et al. identified a hypothetical protein in Penicillium rubens as having an important role in glucose/galactose metabolism (Silva et al., 2020). Finally, in a recent study, the Q6S8D9_SARS protein of the virus SARS-CoV was determined to potentially alter the host antiviral inflammatory cytokine and interferon production pathways (Rahman et al., 2023), demonstrating that hypothetical viral proteins may also be of interest to investigate.
When a gene is no longer needed by a bacterium, it tends to accumulate mutations due to reduced conservation pressure and drift into a pseudogene that is quickly eliminated (Kuo and Ochman, 2010). Therefore, if genes coding for hypothetical proteins are maintained, it is reasonable to believe that they have a function necessary for the proper functioning or survival of the cell. There is some evidence to support the importance of a gene coding for a hypothetical protein: a gene is too long to be an adventitious reading frame, homologous sequences are found in several organisms, and a transcript is present.
One of the challenges with hypothetical protein-coding genes is assigning functions to them efficiently and with a good degree of certainty. As previously demonstrated by the E. coli example, it may be possible to assign putative functions to several proteins encoded by these genes using various bioinformatics tools. Not all tools use the same databases, algorithms, and criteria to find homologous sequences. Using different, complementary tools can, therefore, lead to better functional annotation. This rationale for using complementary tools was addressed in 2019 by Ijaq et al., who proposed a nine-point classification to help assign function to hypothetical proteins (Ijaq et al., 2019). In addition to sequence homology annotation against different databases, the authors also proposed the use of other tools to infer protein–protein relationships, cellular localization, and protein structures.
The increasing availability of genomic and metagenomic sequences, coupled with advancing computing power, allows for the implementation of large-scale strategies to investigate protein distribution, such as clustering proteins into homologous groups. A recent endeavor clustered 415,971,742 genes predicted from 1749 metagenomes and 28,941 bacterial and archaeal genomes into 2,940,257 high-quality clusters (Vanni et al., 2022), with 43% of clusters identified as unknowns. This information can be important because hypothetical protein families that are conserved in multiple genomes are likely to be functional. Inferring the taxonomy of the microorganisms carrying these unknown families, and considering the environment where they were found, can provide valuable insights. Unknown families typically exhibit narrower taxonomic and ecological distributions compared with known families, indicating their potential significance for niche adaptation (Coelho et al., 2022; Vanni et al., 2022). Intriguingly, another recent study revealed that many unknown families of proteins are conserved in archaeal groups, suggesting their importance in the emergence and diversification of these groups (Méheust et al., 2022).
Protein functions and structures are closely linked, which is why two proteins with similar structures can also have similar functions, even if no sequence homology is detected (Sousounis et al., 2012). Tools for determining 3D protein structures from primary sequences have taken an incredible leap forward in recent years with the integration of deep learning into their algorithms. For instance, the AlphaFold2 tool (Jumper et al., 2021), developed by DeepMind, has enabled the prediction of the structure of over 200 million sequences in the UniProt database; these results are accessible through a database called AlphaFold DB (Varadi et al., 2022). Many of these structures are for hypothetical proteins and may eventually be used to infer the functions of these proteins. An automated pipeline, 3DFI, exploits these structure prediction tools to infer the functionality of hypothetical proteins (Julian et al., 2021). In 2022, a tool called I-TASSER-MTD was published and can predict, from the primary sequence of a protein, its 3D structure, function, ligand, and more (Zhou et al., 2022). Other bioinformatics resources, such as CATH (Knudsen and Wiuf, 2010), enable searches based on protein structures rather than sequences. Realistically, the growing number of protein structures will enable these tools to be increasingly used and integrated into analysis pipelines.
Although bioinformatics tools to effectively predict protein functions are becoming more available, these analyses can be computationally demanding. Specialized computing and human resources may be required to successfully perform analyses, especially on tens or even hundreds of hypothetical protein sequences. However, computer hardware is also becoming more efficient, including graphics cards with great computing power through GPUs; these are widely used in protein structure prediction algorithms.
In conclusion, coding sequences for hypothetical bacterial proteins are common in databases, such as RefSeq. However, just because proteins are hypothetical does not mean they are not interesting and without biological function. The use of several complementary tools can significantly aid the functional annotation of protein sequences. The development of bioinformatics tools and tools related to protein structures, combined with the improvement of computer equipment, make it possible that new functions will be assigned to proteins currently considered hypothetical. These analyses, by providing functional evidence, will facilitate the experimental confirmation of these proposed functions.
The original contributions presented in the study are included in the article/supplementary material. Further inquiries can be directed to the corresponding author.
AV: Conceptualization, Data curation, Formal analysis, Funding acquisition, Investigation, Methodology, Project administration, Resources, Software, Validation, Writing – original draft.
The author(s) declare financial support was received for the research, authorship, and/or publication of this article. This research was funded by Natural Sciences and Engineering Research Council of Canada (grant No. RGPIN-2022-03321).
The author thanks Sabrina A. Attéré (Université Laval) for her comments on the manuscript.
The author declares that the research was conducted in the absence of any commercial or financial relationships that could be construed as a potential conflict of interest.
The author(s) declared that they were an editorial board member of Frontiers, at the time of submission. This had no impact on the peer review process and the final decision.
All claims expressed in this article are solely those of the authors and do not necessarily represent those of their affiliated organizations, or those of the publisher, the editors and the reviewers. Any product that may be evaluated in this article, or claim that may be made by its manufacturer, is not guaranteed or endorsed by the publisher.
Altschul S. F., Gish W., Miller W., Myers E. W., Lipman D. J. (1990). Basic local alignment search tool. J. Mol. Biol. 215, 403–410. doi: 10.1016/S0022-2836(05)80360-2
Araújo C. L., Blanco I., Souza L., Tiwari S., Pereira L. C., Ghosh P., et al. (2020). In silico functional prediction of hypothetical proteins from the core genome of Corynebacterium pseudotuberculosis biovar ovis. PeerJ 8, e9643. doi: 10.7717/peerj.9643
Aziz R. K., Bartels D., Best A. A., DeJongh M., Disz T., Edwards R. A., et al. (2008). The RAST server: Rapid annotations using subsystems technology. BMC Genomics 9, 75. doi: 10.1186/1471-2164-9-75
Buchfink B., Xie C., Huson D. H. (2015). Fast and sensitive protein alignment using DIAMOND. Nat. Methods 12, 59–60. doi: 10.1038/nmeth.3176
Cantalapiedra C. P., Hernández-Plaza A., Letunic I., Bork P., Huerta-Cepas J. (2021). eggNOG-mapper v2: Functional annotation, orthology assignments, and domain prediction at the metagenomic scale. Mol. Biol. Evol. 38, 5825–5829. doi: 10.1093/molbev/msab293
Coelho L. P., Alves R., del Río Á.R., Myers P. N., Cantalapiedra C. P., Giner-Lamia J., et al. (2022). Towards the biogeography of prokaryotic genes. Nature 601, 252–256. doi: 10.1038/s41586-021-04233-4
Doudna J. A., Charpentier E. (2014). The new frontier of genome engineering with CRISPR-Cas9. Science 346, 1258096. doi: 10.1126/science.1258096
Fischer D., Eisenberg D. (1999). Finding families for genomic ORFans. Bioinformatics 15, 759–762. doi: 10.1093/bioinformatics/15.9.759
Huerta-Cepas J., Szklarczyk D., Heller D., Hernández-Plaza A., Forslund S. K., Cook H., et al. (2019). eggNOG 5.0: a hierarchical, functionally and phylogenetically annotated orthology resource based on 5090 organisms and 2502 viruses. Nucleic Acids Res. 47, D309–D314. doi: 10.1093/nar/gky1085
Ijaq J., Malik G., Kumar A., Das P. S., Meena N., Bethi N., et al. (2019). A model to predict the function of hypothetical proteins through a nine-point classification scoring schema. BMC Bioinf. 20, 14. doi: 10.1186/s12859-018-2554-y
Julian A. T., Mascarenhas dos Santos A. C., Pombert J.-F. (2021). 3DFI: a pipeline to infer protein function using structural homology. Bioinform. Adv. 1, vbab030. doi: 10.1093/bioadv/vbab030
Jumper J., Evans R., Pritzel A., Green T., Figurnov M., Ronneberger O., et al. (2021). Highly accurate protein structure prediction with AlphaFold. Nature 596, 583–589. doi: 10.1038/s41586-021-03819-2
Kapopoulou A., Lew J. M., Cole S. T. (2011). The MycoBrowser portal: A comprehensive and manually annotated resource for mycobacterial genomes. Tuberculosis 91, 8–13. doi: 10.1016/j.tube.2010.09.006
Kuo C.-H., Ochman H. (2010). The extinction dynamics of bacterial pseudogenes. PloS Genet. 6, e1001050. doi: 10.1371/journal.pgen.1001050
Li J., Tai C., Deng Z., Zhong W., He Y., Ou H.-Y. (2018). VRprofile: gene-cluster-detection-based profiling of virulence and antibiotic resistance traits encoded within genome sequences of pathogenic bacteria. Brief Bioinform. 19, 566–574. doi: 10.1093/bib/bbw141
Méheust R., Castelle C. J., Jaffe A. L., Banfield J. F. (2022). Conserved and lineage-specific hypothetical proteins may have played a central role in the rise and diversification of major archaeal groups. BMC Biol. 20, 154. doi: 10.1186/s12915-022-01348-6
O’Leary N. A., Wright M. W., Brister J. R., Ciufo S., Haddad D., McVeigh R., et al. (2016). Reference sequence (RefSeq) database at NCBI: current status, taxonomic expansion, and functional annotation. Nucleic Acids Res. 44, D733–D745. doi: 10.1093/nar/gkv1189
Orlek A., Anjum M. F., Mather A. E., Stoesser N., Walker A. S. (2023). Factors associated with plasmid antibiotic resistance gene carriage revealed using large-scale multivariable analysis. Sci. Rep. 13, 2500. doi: 10.1038/s41598-023-29530-y
Rahman M. F., Hasan R., Biswas M. S., Shathi J. H., Hossain M. F., Yeasmin A., et al. (2023). A bioinformatics approach to characterize a hypothetical protein Q6S8D9_SARS of SARS-CoV. Genomics Inform 21, e3. doi: 10.5808/gi.22021
Rahman M., Heme U. H., Parvez Md. A.K. (2022). In silico functional annotation of hypothetical proteins from the Bacillus paralicheniformis strain Bac84 reveals proteins with biotechnological potentials and adaptational functions to extreme environments. PloS One 17, e0276085. doi: 10.1371/journal.pone.0276085
Raj U., Sharma A. K., Aier I., Varadwaj P. K. (2017). In silico characterization of hypothetical proteins obtained from Mycobacterium tuberculosis H37Rv. Netw. Model. Anal. Health Inform Bioinforma 6, 5. doi: 10.1007/s13721-017-0147-8
Rasko D. A., Rosovitz M. J., Myers G. S. A., Mongodin E. F., Fricke W. F., Gajer P., et al. (2008). The pangenome structure of Escherichia coli: Comparative genomic analysis of E. coli commensal and pathogenic isolates. J. Bacteriol 190, 6881–6893. doi: 10.1128/JB.00619-08
Richter M., Rosselló-Móra R. (2009). Shifting the genomic gold standard for the prokaryotic species definition. Proc. Natl. Acad. Sci. U.S.A. 106, 19126–19131. doi: 10.1073/pnas.0906412106
Schwengers O., Jelonek L., Dieckmann M. A., Beyvers S., Blom J., Goesmann A. (2021). Bakta: rapid and standardized annotation of bacterial genomes via alignment-free sequence identification. Microb. Genom 7, 685. doi: 10.1101/2021.09.02.458689
Seemann T. (2014). Prokka: rapid prokaryotic genome annotation. Bioinformatics 30, 2068–2069. doi: 10.1093/bioinformatics/btu153
Silva F. F., Gonçalves D. B., Lopes D. O. (2020). The use of bioinformatics tools to characterize a hypothetical protein from Penicillium rubens. Genet. Mol. Res. 19, GMR18574. doi: 10.4238/gmr18574
Sousounis K., Haney C. E., Cao J., Sunchu B., Tsonis P. A. (2012). Conservation of the three-dimensional structure in non-homologous or unrelated proteins. Hum. Genomics 6, 10. doi: 10.1186/1479-7364-6-10
Szklarczyk D., Franceschini A., Wyder S., Forslund K., Heller D., Huerta-Cepas J., et al. (2015). STRING v10: protein–protein interaction networks, integrated over the tree of life. Nucleic Acids Res. 43, D447–D452. doi: 10.1093/nar/gku1003
Vanni C., Schechter M. S., Acinas S. G., Barberán A., Buttigieg P. L., Casamayor E. O., et al. (2022). Unifying the known and unknown microbial coding sequence space. Elife 11, e67667. doi: 10.7554/eLife.67667
Varadi M., Anyango S., Deshpande M., Nair S., Natassia C., Yordanova G., et al. (2022). AlphaFold Protein Structure Database: massively expanding the structural coverage of protein-sequence space with high-accuracy models. Nucleic Acids Res. 50, D439–D444. doi: 10.1093/nar/gkab1061
Winsor G. L., Griffiths E. J., Lo R., Dhillon B. K., Shay J. A., Brinkman F. S. L. (2016). Enhanced annotations and features for comparing thousands of Pseudomonas genomes in the Pseudomonas genome database. Nucleic Acids Res. 44, D646–D653. doi: 10.1093/nar/gkv1227
Keywords: bacterial genomics, bioinformatics, genome annotation, hypothetical proteins, protein structures
Citation: Vincent AT (2024) Bacterial hypothetical proteins may be of functional interest. Front. Bacteriol. 3:1334712. doi: 10.3389/fbrio.2024.1334712
Received: 07 November 2023; Accepted: 23 February 2024;
Published: 05 March 2024.
Edited by:
Daniel Yero, Autonomous University of Barcelona, SpainReviewed by:
Raphaël Méheust, Commissariat à l’Energie Atomique et aux Energies Alternatives (CEA), FranceCopyright © 2024 Vincent. This is an open-access article distributed under the terms of the Creative Commons Attribution License (CC BY). The use, distribution or reproduction in other forums is permitted, provided the original author(s) and the copyright owner(s) are credited and that the original publication in this journal is cited, in accordance with accepted academic practice. No use, distribution or reproduction is permitted which does not comply with these terms.
*Correspondence: Antony T. Vincent, YW50b255LnZpbmNlbnRAZnNhYS51bGF2YWwuY2E=
Disclaimer: All claims expressed in this article are solely those of the authors and do not necessarily represent those of their affiliated organizations, or those of the publisher, the editors and the reviewers. Any product that may be evaluated in this article or claim that may be made by its manufacturer is not guaranteed or endorsed by the publisher.
Research integrity at Frontiers
Learn more about the work of our research integrity team to safeguard the quality of each article we publish.