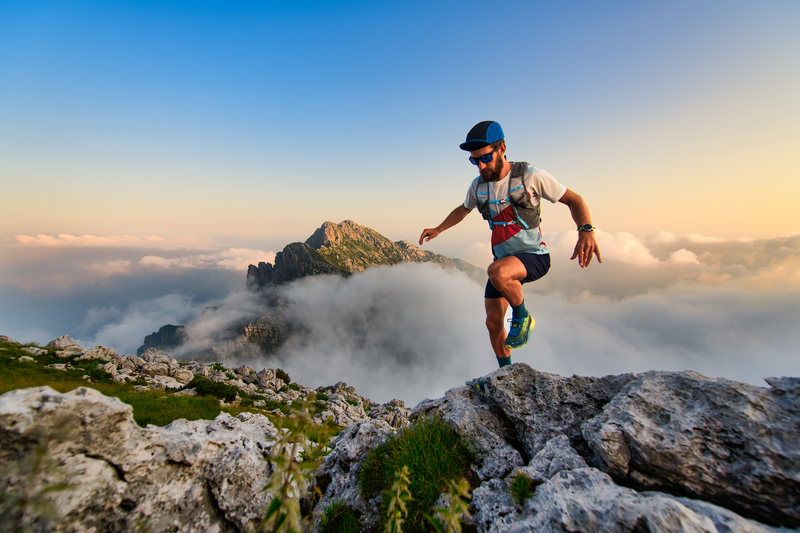
95% of researchers rate our articles as excellent or good
Learn more about the work of our research integrity team to safeguard the quality of each article we publish.
Find out more
METHODS article
Front. Astron. Space Sci.
Sec. Stellar and Solar Physics
Volume 12 - 2025 | doi: 10.3389/fspas.2025.1541901
The final, formatted version of the article will be published soon.
You have multiple emails registered with Frontiers:
Please enter your email address:
If you already have an account, please login
You don't have a Frontiers account ? You can register here
The Extreme Ultraviolet Imaging Spectrometer (EIS) on the Hinode spacecraft has substantially advanced our understanding of the Sun's upper atmosphere. Unfortunately, after being in operation since 2006, the EIS detectors have become noisy, which poses a challenge to data analysis. This paper presents a Conditional Generative Adversarial Network (cGAN) tailored to address the unique noise characteristics inherent in EIS data over the mission. Generative Adversarial Networks are deep learning models that learn to generate realistic data by training a pair of networks in an adversarial process, a mechanism that makes them particularly effective at capturing complex data distributions. Our cGAN model employs a U-Net-based generator and a conditioned discriminator, and it is trained and validated on a synthetic dataset designed to simulate the noise characteristics of EIS observations. The model converges quickly and produces denoised images that closely resemble the ground truth. Application to real EIS observations produces encouraging results, with the model effectively removing noise and largely preserving the spatial and spectral features of the data. When comparing the results of Gaussian fits to the line profiles, however, we find that the model produces only a modest enhancement over the current interpolation method.
Keywords: machine learning, Solar physics, Solar spectra, Image processing - de-noising, Solar atmosphere
Received: 09 Dec 2024; Accepted: 26 Mar 2025.
Copyright: © 2025 Rahman and Warren. This is an open-access article distributed under the terms of the Creative Commons Attribution License (CC BY). The use, distribution or reproduction in other forums is permitted, provided the original author(s) or licensor are credited and that the original publication in this journal is cited, in accordance with accepted academic practice. No use, distribution or reproduction is permitted which does not comply with these terms.
* Correspondence:
Harry P Warren, Naval Research Laboratory, Washington D.C., United States
Disclaimer: All claims expressed in this article are solely those of the authors and do not necessarily represent those of their affiliated organizations, or those of the publisher, the editors and the reviewers. Any product that may be evaluated in this article or claim that may be made by its manufacturer is not guaranteed or endorsed by the publisher.
Research integrity at Frontiers
Learn more about the work of our research integrity team to safeguard the quality of each article we publish.