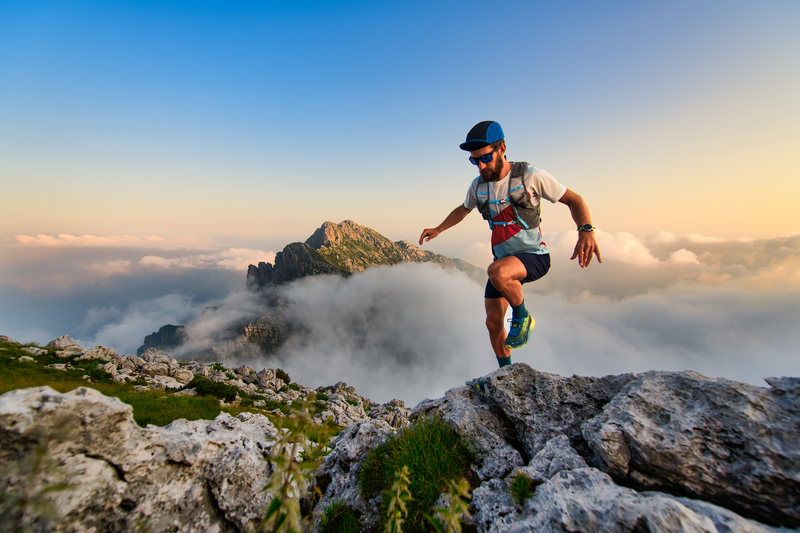
94% of researchers rate our articles as excellent or good
Learn more about the work of our research integrity team to safeguard the quality of each article we publish.
Find out more
ORIGINAL RESEARCH article
Front. Astron. Space Sci.
Sec. Space Physics
Volume 12 - 2025 | doi: 10.3389/fspas.2025.1533126
This article is part of the Research Topic Dynamic Exospheres of Terrestrial Bodies Through The Solar System View all 7 articles
The final, formatted version of the article will be published soon.
You have multiple emails registered with Frontiers:
Please enter your email address:
If you already have an account, please login
You don't have a Frontiers account ? You can register here
The charge exchange interaction between exospheric hydrogen (H) atoms and energetic ions in the terrestrial ring current is a crucial mechanism for dissipating global magnetospheric energy, especially during the recovery phase of geomagnetic storms. Historically, ring current modeling has considered H density distributions temporally static and spherically symmetric owing to the lack of event-specific exospheric models. However, observations of the far-ultraviolet (FUV) emission from exospheric H atoms acquired by NASA's TWINS Lyman-Alpha Detectors (LADs) unveiled not only spatial asymmetries but also significant temporal variability of this neutral population, particularly during storm time. In this work, we investigate the influence of realistic exospheric H density distributions on the ring current decay during the strong storm on June 1st, 2013. To do so, we first estimate time-dependent, three-dimensional (3-D) H density distributions using FUV radiance data acquired by TWINS/LADs with a robust tomographic approach. Then, we use these neutral distributions as inputs for the Comprehensive Inner Magnetosphere-Ionosphere (CIMI) model and simulate the ion ring current behavior as a response to the exospheric dynamics. We compared the resulting ion fluxes with those produced when a static and spherically symmetric H model [Rairden et al., 1986] is used. We found that the TWINS-based global hydrogen density beyond 3 $R_E$ geocentric distances is, on average, $\sim$35$\%$ larger than that of Rairden's model during quiet time and increases up to $\sim$50$\%$ during the geomagnetic storm. Consequently, the ring current ion flux during the recovery phase decays faster when the TWINS-based model is used. Our comparison study shows that using a realistic H-density model produces ~60% lower ion fluxes (H+ and O+ with energy range 0.1-60 keV) than those yielded by Rairden's model, especially during the recovery phase and at L-shells <4RE. Also, when the TWINS-based model is used, the total ring current energy during the recovery phase is ~30% lower than the energy calculated with the static model.
Keywords: Exosphere, Ring current, Tomography, magnetosphere, ion-neutral coupling
Received: 23 Nov 2024; Accepted: 03 Mar 2025.
Copyright: © 2025 Cucho-Padin, Ferradas, Fok, Waldrop, Zoennchen and Kang. This is an open-access article distributed under the terms of the Creative Commons Attribution License (CC BY). The use, distribution or reproduction in other forums is permitted, provided the original author(s) or licensor are credited and that the original publication in this journal is cited, in accordance with accepted academic practice. No use, distribution or reproduction is permitted which does not comply with these terms.
* Correspondence:
Gonzalo Cucho-Padin, NASA Goddard Space Flight Center, Greenbelt, United States
Disclaimer: All claims expressed in this article are solely those of the authors and do not necessarily represent those of their affiliated organizations, or those of the publisher, the editors and the reviewers. Any product that may be evaluated in this article or claim that may be made by its manufacturer is not guaranteed or endorsed by the publisher.
Research integrity at Frontiers
Learn more about the work of our research integrity team to safeguard the quality of each article we publish.