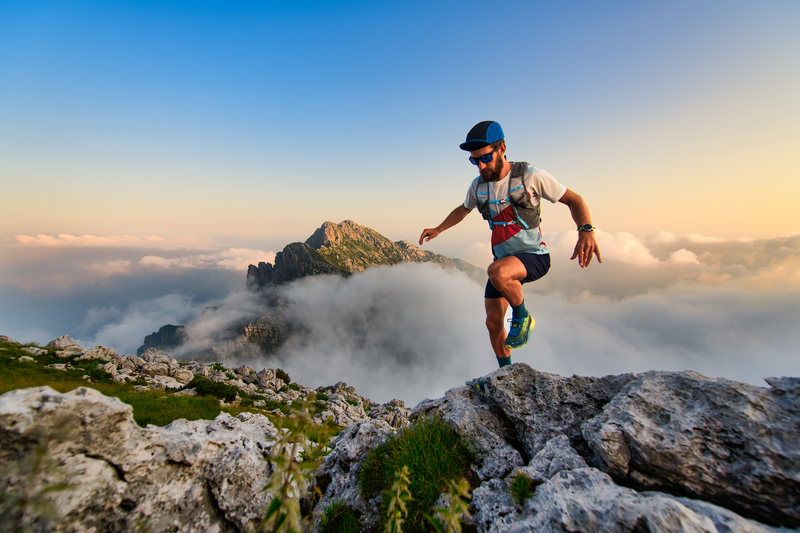
94% of researchers rate our articles as excellent or good
Learn more about the work of our research integrity team to safeguard the quality of each article we publish.
Find out more
ORIGINAL RESEARCH article
Front. Astron. Space Sci.
Sec. Astrostatistics
Volume 12 - 2025 | doi: 10.3389/fspas.2025.1473492
The final, formatted version of the article will be published soon.
You have multiple emails registered with Frontiers:
Please enter your email address:
If you already have an account, please login
You don't have a Frontiers account ? You can register here
Clusters to model the Initial Mass Function requires involvement of binary as well as single star components. Components of masses from Gaia EDR3 were estimated using the massluminosity relationship and contribution of each to the total system is studied. Stochastic models describing the contribution of each component are developed for binary as well as single stars incorporating the escape mass theory of the assumed pair. Binary masses, fitted to suitable bi-variate distributions are simulated using Griddy Gibbs sampler, an MCMC algorithm. Stellar masses of single stars are simulated from suitable uni-variate distribution. The mass spectrum of the binary as well as single components are then considered together to form the initial mass function. The resulting mass function under opacity limited fragmentation scenario are further investigated at different projected distances from the core to radius where signature of mass segregation is found.
Keywords: initial mass function, Izawa Bi-variate Gamma Distribution, Escape Mass, Griddy-Gibbs sampler, Mass segregation
Received: 31 Jul 2024; Accepted: 17 Feb 2025.
Copyright: © 2025 Sinha Adhikary and Das. This is an open-access article distributed under the terms of the Creative Commons Attribution License (CC BY). The use, distribution or reproduction in other forums is permitted, provided the original author(s) or licensor are credited and that the original publication in this journal is cited, in accordance with accepted academic practice. No use, distribution or reproduction is permitted which does not comply with these terms.
* Correspondence:
Abisa Sinha Adhikary, Amity University Kolkata, Kolkata, India
Disclaimer: All claims expressed in this article are solely those of the authors and do not necessarily represent those of their affiliated organizations, or those of the publisher, the editors and the reviewers. Any product that may be evaluated in this article or claim that may be made by its manufacturer is not guaranteed or endorsed by the publisher.
Research integrity at Frontiers
Learn more about the work of our research integrity team to safeguard the quality of each article we publish.