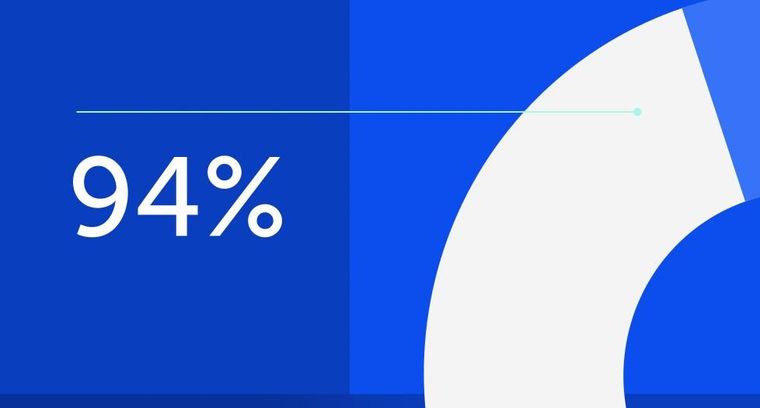
94% of researchers rate our articles as excellent or good
Learn more about the work of our research integrity team to safeguard the quality of each article we publish.
Find out more
ORIGINAL RESEARCH article
Front. Astron. Space Sci., 04 April 2025
Sec. Space Physics
Volume 12 - 2025 | https://doi.org/10.3389/fspas.2025.1383946
This article is part of the Research TopicVariability in the Solar Wind and its Impact on the Coupled Magnetosphere-Ionosphere-Thermosphere SystemView all 11 articles
Introduction: Future crewed missions to Mars will encounter substantially elevated radiation levels compared to low Earth orbit operations. To address this challenge, we present the Space-Dependent Energetic cosmic ray Modulation using MAgnetic spectrometer (SDEMMA) model, a novel framework for modeling galactic cosmic ray (GCR) dynamics in deep-space environments.
Methods: The model employs stochastic differential equations with outer boundary conditions derived from contemporary local interstellar spectrum models. Time-dependent diffusion and drift coefficients were optimized through Markov Chain Monte Carlo parameter fitting against 2006-2019 observational data from the space-borne magnetic spectrometers of AMS-02 and PAMELA.
Results: SDEMMA extends GCR spectral calculations to radial positions beyond 1.0 AU, explicitly resolving radial gradients under diverse heliospheric conditions. The framework provides spatiotemporally resolved GCR spectra for charge numbers Z=1–28 at rigidities >0.2 GV, covering the inner heliosphere between Earth and Mars and currently the 2006-2019 epoch.
Discussion: Implementation demonstrates the model's operational utility: dose equivalent rates behind 30 g/cm2 polyethylene shielding during a flux minimum range from 14-17 cSv/yr, with variance attributable to quality factor selection.
While radiation exposure for astronauts in a low Earth orbit or even during a journey to the Moon can now be considered less challenging, this problem is still not well understood in the next natural step for a journey to the Mars. Due to the absence of the geomagnetic field shielding and a longer trip which last for years, the radiation level is much higher. Few in situ measurements have been performed. The Radiation Assessment Detector (RAD) on the Mars Science Laboratory (MSL), also known as the Curiosity rover, made the first-ever measurement during the transit from Earth to Mars in the 2011 Mars mission time window (Zeitlin et al., 2013). The total measured dose equivalent rate is
Such GCRs induced radiation dose in the transit orbit represents the most important radiation exposure during the human exploration of Mars (Guo et al., 2024), since GCRs are a continuous radiation source that is hard to shield against. On the other hand, the SEP events can be shielded in a better shelter part of a spacecraft for a few hours. And once on the planet, Mars’ atmosphere provides some protection, and a sub-surface shelter gives even more protection. Therefore, this important problem deserves further study. Without expensive in situ measurements, our goal is to develop a model-based calculation for the cumulative radiation dose experienced by astronauts on the journey to Mars (HelMod, 2024; Opher et al., 2023). This calculation scheme is aimed to (a) cover various possible Mars mission time windows under different solar modulation conditions, (b) provide full radial dependence, and (c) be applied to realistic target astronaut phantoms with flexible shielding. The goal involves researches in several broad field, and we plan to achieve it in a step by step manner. In the present paper we will mainly address the more “academic” GCR related issue (a and b), and keep the discussion of the space dosimetry (c) only at an illustrative level. The more realistic “engineering” dosimetric values will be provided in a forthcoming publication.
GCR spectra are influenced by solar activity, with the solar wind and interplanetary magnetic field playing crucial roles in their modulation. This variability with time is continuously captured by numerous experiments conducted on or around the Earth. Here, we use data in time series from space-borne magnetic spectrometers, specifically the PAMELA and the AMS-02 (Adriani et al., 2013; Martucci et al., 2018; Marcelli et al., 2020; Aguilar et al., 2018; 2021; 2022). Furthermore, the encountered GCR spectra of the astronauts also vary at different radial locations. This variability cannot be covered by most previous measurements, including the PAMELA and the AMS-02. Our approach is to explicitly expand the calculation previously limited to the 1.0 AU slice (O’Neill et al., 2015; Slaba and Whitman, 2019; Boschini et al., 2016) to other radial locations in the inner solar system. With at least the solar modulation effect, the calculated GCR spectra dataset forms a GCR model, which we have named the Space-Dependent Energetic cosmic ray Modulation using MAgnetic spectrometer (SDEMMA) model. Except that its 1.0 AU spectra has been integrated with the ICRP123 fluence-to-dose conversion coefficient (ICRP123 et al., 2013) for the unshielded astronaut dose rate (Chen et al., 2023), this new GCR model is comparable to the Badhwar-O’Neill series of models (O’Neill et al., 2015; Slaba and Whitman, 2019), the HelMod model (Boschini et al., 2016), etc. It can be used for general purposes beyond space dosimetry, and will be long-term supported.
As an example for its dosimetric application, we use this model to calculate the astronaut radiation dose rate between the Earth’s and the Mars’ orbit. While the SDEMMA GCR model provides the number of the incident particles for each species at each kinetic energy, the dose calculation needs another factor, which is the expected dose equivalent caused by a single incident particle (the fluence-to-dose-equivalent conversion coefficient). We have used several sets of fluence-to-dose-equivalent conversion coefficient, including the ones calculated by ourselves using the particle physics toolkit GEANT4 (Agostinelli et al., 2003; Allison et al., 2006; Allison et al., 2016). The dose equivalent rates are obtained in time series upon integration with the two factors.
The paper is organized as follows. In Section 2 we review the GCR spectra calculation method, including the stochastic differential equation (SDE) approach and the local interstellar spectra as the outer boundary conditions. We pay special attention to the heliospheric environment modeling, as well as the data from the PAMELA and the AMS-02 experiments and the fitting procedure to determine the diffusion and drift coefficients. In Section 3 we present a detailed discussion of the GCR flux, which depends on rigidities, GCR species, time, and radial positions. These two sections actually define the SDEMMA model. Then we introduce the fluence-to-dose-equivalent conversion coefficient and calculate the dose equivalent rate in Section 4, using three shielding settings: the unshielded case for uncertainty demonstration, the MSL/RAD shielding case for validation, and the optimized shielding thickness for the reference values. Finally, we summarize in Section 5.
The spectra of GCRs are related to their phase space density through
Here,
As an application of the Feynman-Kac formula treatment of the stochastic diffusion process, the Parker’s transport Equation 1 can be reformulated into an equivalent set of 3D SDEs for the GCR phase space coordinates (Zhang, 1999)
with
Here, three spatial dimensions
The local interstellar spectra for protons from Corti et al. (2019), for iron from Boschini et al. (2021), and for all other elements from Boschini et al. (2020) are used as the outer boundary conditions (Song et al., 2021). They are implemented at 120 AU where the heliopause locates. The spectra of the all the
Our modeling of the heliospheric environment is identical to that of Song et al. (2021). Here, we provide a brief review of the four specific models in the Radial-Tangential-Normal coordinates: the solar wind velocity
Figure 1. The time series of the four observational parameters (A), and the five fitted parameters through the MCMC process and the corresponding
The solar wind velocity up to the termination shock is given by Potgieter et al. (2014)
where
Outside the termination shock of 90 AU, the solar wind transitions from supersonic to subsonic speeds. Here, the velocity is assumed to decrease to
The used 3D HMF is the Parker’s spiral with a polar region enhancement (Jokipii and Kota, 1989).
Here,
In contrast to the two aforementioned models which are determined through observation and have become quite distinctive, there are numerous options in the literature for diffusion and drift coefficients. Here, we use a set of parameterized coefficients as provided by Potgieter (2013). Compared with diffusion coefficients that are more theoretically motivated (e.g., Qin and Zhang, 2014), our parametrized coefficients usually have the advantage of reproducing observational spectra with greater precision, but at the cost of complexity in making forecast.
The drift velocity is given by Potgieter (2013)
with
Here,
The diffusion tensor is given by its components (Potgieter, 2013).
Here
Finally we would like to make comment on the validity of the spectra calculation at locations different from the 1.0 AU. Since all of the above models hold for the entire spatial heliosphere region, for given observational and fitted parameter in time series, the calculation of GCR spectra is equally accurate at other locations within the heliosphere, especially at the range of
As mentioned, the Alpha Magnetic Spectrometer (AMS) 02 detector is one of the most effective tools for measuring GCR spectra. It was launched in May 2011 and installed on the International Space Station at an altitude of about 400 km. The detector can measure all the GCR elements with atomic numbers ranging from 1 to 28, with a strong capability for discriminating between elements and controlling errors. The PAMELA detector is another space-borne magnetic spectrometer before the AMS-02, which was launched in June 2006 into an orbit at an altitude of
We determine the time-series of the aforementioned five parameters by fitting them to the time-dependent spectra measured by AMS-02 and PAMELA in the proton and helium channels (Adriani et al., 2013; Martucci et al., 2018; Marcelli et al., 2020; Aguilar et al., 2018; 2021; 2022). In Figure 1 we have plotted the four observables (
The GCR spectra data are provided in the form of time series only for the proton and helium channels. Therefore, the fitting of diffusion and drift coefficients is performed only for these two species. However, Song et al. (2021) was able to fit both the proton channel and the helium channel with the same parameter set. This indicates the universality of the diffusion and drift coefficients for different GCR species (Tomassetti et al., 2018; Corti et al., 2019; Wang et al., 2019; Ngobeni et al., 2020; Fiandrini et al., 2021). In fact, the diffusion and drift coefficients are statistical measures of the small and large scale magnetic field irregularities. For GCRs with the same rigidity, the local curvature radius of the trajectory of an incident charged particle is determined by the ratio of the rigidity to the magnetic field strength, regardless of the particle’s type or mass. It implies the same trajectory for particles with the same rigidity, explaining the universality of the drift coefficient for all GCR species. On the other hand, the diffusion coefficient is determined by the interaction between particles and waves/turbulence. According to quasi linear theory, the particle with the same gyro-radius resonates with the same waves, thus the resonant condition determines that particles with the same rigidity have the same diffusion coefficient. So here we use the best fits of the combined proton and helium data as the universal coefficients, and apply them to calculate the other 26 elements.
The GCR flux is calculated on a four-dimensional grid, which is summarized in Table 1. Figure 2 shows sample calculations for three dimensions at 1.0 AU, namely during solar minimum and maximum as two representative dates, in each panel for five representative GCR species and the whole rigidity range.
Table 1. The list of variables for our current SDEMMA spectra. The major part of the calculation consists of
Figure 2. The SDE calculated spectra for proton (black), helium (red), oxygen (orange), silicon (green), and iron (blue) at 1.0 AU, at the solar minimum (A) and maximum (B) respectively. The PAMELA and AMS-02 measured proton and helium GCR spectra for the corresponding periods are also shown, except for the PAMELA helium data which is not for one Bartels rotation. Due to the finite sampling number in the SDE method (3,000 pseudoparticles per energy bin), the spectra show some fluctuation on the low rigidity side (see also discussion in Section 3.4).
The calculated range of rigidity is always from 0.2 to 100 GV for all elements. As a result of the MCMC fitting process, the calculated spectra accurately reproduce the measured proton and helium spectra in the rigidity region where PAMELA and AMS-02 have conducted direct measurements (Song et al., 2021; Chen et al., 2023), as a validation of the model. However, due to the finite thickness of the apparatus, low-energy/rigidity GCRs are blocked in the detector and cannot be detected, causing the measured rigidity to terminate at 1 GV for a proton and 1.65 GV for helium for the AMS-02. Beneath these minimal rigidities, one must work with the calculated spectra. As seen in Figure 2, the flux asymptotically vanishes as the rigidity decreases on the low-rigidity side (Moraal and Potgieter, 1982). The GCR spectra also decrease on the high rigidity side, following a power law with a well-known spectral index of approximately
Although heavy ions are less abundant, their dose contribution can be enhanced by powers of their nuclear charge
The AMS-02 measurements for heavy elements extend to silicon and iron channels, but they are now in the form of averages over many years, and results with even shorter period have not been published yet. On the other hand, the ACE/CRIS experiment provides time-dependent measurement for heavy elements, but the detector type is calorimeter rather than magnetic spectrometer. Since we define our model using only the time-dependent data from space-borne magnetic spectrometers, the ACE/CRIS data for heavy elements are used only for cross check, but not for fitting. We respect the aforementioned universality of the diffusion and drift coefficients in calculations of other heavy elements for theoretical consistency. In Chen et al. (2023) we have compared our calculated spectra to the measurements averaged over many years for the elements with available data, and found good agreement.
Solar activity is known for its stochastic fluctuations in addition to its well-known 11-year cycle, which consists of a solar maximum and a solar minimum in each cycle. Many issues related to the time dependence have already been addressed in the previous discussion of Section 2.2. One can also refer to Song et al. (2021) for more information about the GCR spectra prior to June 2017, and a comparison in the form of dose rate time series in Chen et al. (2023) for the 1.0 AU slice result.
Starting from June 2006 of the beginning of the PAMELA experiment (Adriani et al., 2013; Martucci et al., 2018; Marcelli et al., 2020), the original fitting up to June 2017 in Song et al. (2021) is further extended to October 2019, in order to match the latest available AMS-02 time-dependent proton and helium data (Aguilar et al., 2018; Aguilar et al., 2021; Aguilar et al., 2022). New data after October 2019 will be implemented as soon as they become available.
Currently the model does not include forecast for future GCR spectra, which is crucial for future spacecraft design and space mission planing. For future solar modulation, all the GCR spectra forecast should be based on the forecast of future heliospheric environment parameters. A new GCR spectra forecast approach has been developed with the machine learning technique in Du et al. (2025), which uses the same previous GCR spectra data but not the Parker’s transport equation. A more traditional approach of forecast based on solving the Parker’s transport equation, more similar to the current forecast of Badhwar-O’Neil model and HelMod model, is also under development.
In Gieseler et al. (2008) the radial gradient was measured to be
In Figure 3 we present several flux ratios
Figure 3. The flux
In Figure 4 we plot the flux ratios
Figure 4. The proton flux
Note that there are various techniques for solving Parker’s transport equation. Beyond the SDE method, there is another class of method, which is the alternating directional implicit (ADI) method (Potgieter and Moraal, 1985). Different from the SDE method which introduces the stochastic motion for a traced pseudoparticle and solves the problem in a Monte Carlo way, the ADI method focus on directly solving the partial differential equation in a deterministic way. In the latter approach a static equilibrium phase space density flow configuration is solved, then information across the whole spatial region is immediately extracted. On the other hand, in the SDE method the calculation of spectrum can only be done spatial point by spatial point, so if a radial gradient is the primary goal the SDE calculation is relatively less effective. But since the sampled pseudoparticle is simulated in real time, as mentioned before we can adopt a dynamical heliospheric environment with the observed
In Table 2 we present a simple comparison of our SDEMMA model with other recent GCR models, including the latest version of the Badhwar-O’Neill models (O’Neill et al., 2015; Slaba and Whitman, 2019) and the HelMod model (Boschini et al., 2016). All versions of NASA’s Badhwar-O’Neill models are based on a one-dimensional (radial) solution to Parker’s transport equation. Only one time-dependent variable, the modulation potential
A dedicated and more comprehensive comparison of GCR models is given in Liu et al. (2024), which includes our model, the BON2020 model, the HelMod model, as well as the CREME model (Tylka et al., 1997; Adams et al., 2012) and DLR model (Matthiä et al., 2013).
Based on the SDEMMA model, we calculate the astronaut dose equivalent rate
By cancelling the factors such as
The fluence-to-dose-equivalent conversion coefficient has the meaning of the expected dose equivalent caused by a single incident particle, if the incident particle is integrated over all contributing area on the normal plane of its “beam” direction. It is calculated by particle physics Monte Carlo code. We have calculated the isotropic fluence-to-dose-equivalent conversion coefficients using the Monte Carlo toolkit GEANT4 (Agostinelli et al., 2003; Allison et al., 2006; Allison et al., 2016) using the ICRU sphere1 as the dose counter, for all the
The first
Figure 5. The unshielded dose equivalent rate
The MSL/RAD shielding during the cruise stage in the transit orbit is too complicated to simulate exactly. In simulation we simplify the shielding to three aluminum layers:
We have performed two round of simple simulations. The first one also using the same isotropic GCR incidence and the ICRU sphere gives a dose equivalent rate of 2.07 mSv/d averaged over the MSL/RAD time window. While the isotropy is the case for the astronaut in deep space, the MSL/RAD detector has a small field of view of
The last
Figure 6 shows our final
Figure 6. The dose equivalent rate
The fluence-to-dose-equivalent conversion coefficient and dose equivalent rate calculation using the detailed human voxel phantom will be provided in a forthcoming publication.
We have presented a model for the modulated galactic cosmic ray spectra called SDEMMA. This model is based on data from the space-borne magnetic spectrometers PAMELA and AMS-02. In this model, we use the 3D stochastic differential equation method (Equations 2, 3) to calculate spectra, incorporating the recently developed local interstellar spectra of galactic cosmic rays for all the
We have also developed a set of fluence-to-dose-equivalent conversion coefficients based on simplified dose counter but several shielding considerations. Combining the SDEMMA GCR model and the dose coefficients, the astronaut radiation dose equivalent rates on the transfer orbit are calculated using Equation 14, for the considered period with an explicit radial dependence.
The SDEMMA dataset for GCR spectra time series can be downloaded as a zip file from https://en.iat.cn/resource.
The OMNI solar wind and HMF data are available from the GSFC website https://omniweb.gsfc.nasa.gov. The Wilcox Solar Observatory HCS data is available from the WSO website http://wso.stanford.edu/. The sun spot number data is available from the Solar Influences Data analysis Center website https://www.sidc.be.
The PAMELA proton and helium spectra time series can be extracted from https://tools.ssdc.asi.it/CosmicRays/chargedCosmicRays.jsp. The AMS-02 proton and helium spectra tables in time series are available via the corresponding supplemental material and data links of Phys. Rev. Lett. 121, 051101 (2018), Phys. Rep. 894, 1 (2021), and Phys. Rev. Lett. 128, 231102 (2022) at the AMS group publication page https://ams02.space/publications.
The HelMod GCR spectra time series are available from https://www.helmod.org/index. php?view=article&id=76:transfer-orbit-fluence&catid=14. The fluence-to-dose-equivalent conversion coefficients for the ICRU sphere can be downloaded as a zip file from https://en.iat.cn/resource.
The ICRP123 fluence-to-dose-equivalent conversion coefficients are available via the “Supplemental Material” link at the ICRP123 publication URL https://www.icrp.org/publication.asp?id=ICRP%20Publication%20123.
XS: Data curation, Formal Analysis, Investigation, Methodology, Resources, Software, Validation, Writing–review and editing. RH: Conceptualization, Funding acquisition, Project administration, Supervision, Visualization, Writing–original draft, Writing–review and editing. SX: Data curation, Writing–review and editing. XC: Data curation, Writing–review and editing. XL: Funding acquisition, Methodology, Software, Writing–review and editing.
The author(s) declare that financial support was received for the research and/or publication of this article. The presented work was supported by the Shandong Institute of Advanced Technology start funding (2020106R01, 2020106R02) and the NSFC grants (U2106201).
We are grateful to the useful discussion with Jingnan Guo, Tong Su, Weiwei Xu and Vladimir Mikhailov. The generative AI of Wordvice AI and DeepSeek-R1 have been used for English improvement.
The authors declare that the research was conducted in the absence of any commercial or financial relationships that could be construed as a potential conflict of interest.
All claims expressed in this article are solely those of the authors and do not necessarily represent those of their affiliated organizations, or those of the publisher, the editors and the reviewers. Any product that may be evaluated in this article, or claim that may be made by its manufacturer, is not guaranteed or endorsed by the publisher.
1The ICRU sphere is a phantom used in radiation protection. It has a diameter of 30 cm, and a hypothetical tissue equivalent material of density 1 g/cm3 and composition of oxygen
Adams, J. H., Barghouty, A. F., Mendenhall, M. H., Reed, R. A., Sierawski, B. D., Warren, K. M., et al. (2012). Creme: the 2011 revision of the cosmic ray effects on micro-electronics code. IEEE Trans. Nucl. Sci. 59, 3141–3147. doi:10.1109/TNS.2012.2218831
Adriani, O., Barbarino, G. C., Bazilevskaya, G. A., Bellotti, R., Boezio, M., Bogomolov, E. A., et al. (2013). Time dependence of the proton flux measured by pamela during the 2006 july-2009 december solar minimum. Astrophys. J. 765, 91. doi:10.1088/0004-637x/765/2/91
Agostinelli, S., Allison, J., Amako, K., Apostolakis, J., Araujo, H., Arce, P., et al. (2003). Geant4—a simulation toolkit. Nucl. Instrum. Methods Phys. Res. Sect. A Accel. Spectrom. Detect. Assoc. Equip. 506, 250–303. doi:10.1016/S0168-9002(03)01368-8
Aguilar, M., Aisa, D., Alpat, B., Alvino, A., Ambrosi, G., Andeen, K., et al. (2015). Precision measurement of the proton flux in primary cosmic rays from rigidity 1 GV to 1.8 TV with the alpha magnetic spectrometer on the international space station. Phys. Rev. Lett. 114, 171103. doi:10.1103/PhysRevLett.114.171103
Aguilar, M., Ali Cavasonza, L., Alpat, B., Ambrosi, G., Arruda, L., Attig, N., et al. (2018). Observation of fine time structures in the cosmic proton and helium fluxes with the alpha magnetic spectrometer on the international space station. Phys. Rev. Lett. 121, 051101. doi:10.1103/PhysRevLett.121.051101
Aguilar, M., Ali Cavasonza, L., Ambrosi, G., Arruda, L., Attig, N., Barao, F., et al. (2021). The alpha magnetic spectrometer (AMS) on the international space station: Part II - results from the first seven years. Phys. Rep. 894, 1–116. doi:10.1016/j.physrep.2020.09.003
Aguilar, M., Ali Cavasonza, L., Ambrosi, G., Arruda, L., Attig, N., Barao, F., et al. (2022). Properties of daily helium fluxes. Phys. Rev. Lett. 128, 231102. doi:10.1103/PhysRevLett.128.231102
Allison, J., Amako, K., Apostolakis, J., Araujo, H., Arce Dubois, P., Asai, M., et al. (2006). Geant4 developments and applications. IEEE Trans. Nucl. Sci. 53, 270–278. doi:10.1109/TNS.2006.869826
Allison, J., Amako, K., Apostolakis, J., Arce, P., Asai, M., Aso, T., et al. (2016). Recent developments in Geant4. Nucl. Instrum. Methods Phys. Res. Sect. A Accel. Spectrom. Detect. Assoc. Equip. 835, 186–225. doi:10.1016/j.nima.2016.06.125
Boschini, M., Della Torre, S., Gervasi, M., La Vacca, G., and Rancoita, P. (2022). The transport of galactic cosmic rays in heliosphere: the HelMod model compared with other commonly employed solar modulation models. Adv. Space Res. 854, 2636–2648. doi:10.1016/j.asr.2022.03.026
Boschini, M. J., Della Torre, S., Gervasi, M., Grandi, D., Jóhannesson, G., La Vacca, G., et al. (2021). The discovery of a low-energy excess in cosmic-ray iron: evidence of the past supernova activity in the local bubble. Astrophys. J. 913, 5. doi:10.3847/1538-4357/abf11c
Boschini, M. J., Della Torre, S., Gervasi, M., La Vacca, G., and Rancoita, P. G. (2016). Propagation of cosmic rays in heliosphere: the HELMOD model. Adv. Space Res. 207, 2859–2879. doi:10.1016/j.asr.2017.04.017
Boschini, M. J., Torre, S. D., Gervasi, M., Grandi, D., Jóhannesson, G., Vacca, G. L., et al. (2020). Inference of the local interstellar spectra of cosmic-ray nuclei Z ≤ 28 with the GalProp–HelMod framework. Astrophys. J. Suppl. 250, 27. doi:10.3847/1538-4365/aba901
Badhwar-O’Neill(2014). Galactic cosmic ray flux model description. NASA Technical Reports-2015-218569
Chen, L., Chen, X., Huo, R., Xu, S., and Xu, W. (2025). Astronaut dose coefficients calculated using GEANT4 and comparison with icrp123. Radiat. Environ. Biophysics XX, XXX.
Chen, X., Xu, S., Song, X., Huo, R., and Luo, X. (2023). Astronaut radiation dose calculation with a new galactic cosmic ray model and the AMS-02 data. Space weather. 21, e2022SW003285. doi:10.1029/2022SW003285
Corti, C., Potgieter, M. S., Bindi, V., Consolandi, C., Light, C., Palermo, M., et al. (2019). Numerical modeling of galactic cosmic-ray proton and helium observed by ams-02 during the solar maximum of solar cycle 24. Astrophysical J. 871, 253. doi:10.3847/1538-4357/aafac4
Cucinotta, F. A., Kim, M.-H. Y., and Chappell, L. J. (2011). Space radiation cancer risk projections and uncertainties - 2010. NASA Tech. Reports-2011-216155.
Du, Y.-L., Song, X., and Luo, X. (2025). Deep learning the forecast of galactic cosmic-ray spectra. Astrophysical J. Lett. 978, L36. doi:10.3847/2041-8213/ada427
Fiandrini, E., Tomassetti, N., Bertucci, B., Donnini, F., Graziani, M., Khiali, B., et al. (2021). Numerical modeling of cosmic rays in the heliosphere: analysis of proton data from ams-02 and pamela. Phys. Rev. D. 104, 023012. doi:10.1103/PhysRevD.104.023012
Gieseler, J., Heber, B., Dunzlaff, P., Müller-Mellin, R., Klassen, A., and Gomez-Herrero, R. (2008). “The radial gradient of galactic cosmic rays: ulysses KET and ACE CRIS Measurements,” in International cosmic ray conference. Vol. 1 of international cosmic ray conference, 571–574.1. Available online at https://ui.adsabs.harvard.edu/abs/2008ICRC
Guo, J., Slaba, T. C., Zeitlin, C., Wimmer-Schweingruber, R. F., Badavi, F. F., Böhm, E., et al. (2017). Dependence of the martian radiation environment on atmospheric depth: modeling and measurement. J. Geophys. Res. Planets 122, 329–341. doi:10.1002/2016JE005206
Guo, J., Wang, B., Whitman, K., Plainaki, C., Zhao, L., Bain, H. M., et al. (2024). Particle radiation environment in the heliosphere: status, limitations, and recommendations. Adv. Space Res. doi:10.1016/j.asr.2024.03.070
Guo, J., Zeitlin, C., Wimmer-Schweingruber, R. F., Hassler, D. M., Posner, A., Heber, B., et al. (2015). Variations of dose rate observed by MSL/RAD in transit to Mars. Astronomy and Astrophysics 577, A58. doi:10.1051/0004-6361/201525680
HelMod (2024). Available online at: https://www.helmod.org/index.php?view=article&id=76:transfer-orbit-fluence&catid=14.
ICRP103 (2007). 2007 recommendations of the international commission on radiological protection. ICRP publication 103. Ann. ICRP 37.
ICRP123 Dietze, G., Bartlett, D. T., Cool, D. A., Cucinotta, F. A., Jia, X., et al. (2013). ICRP, 123. Assessment of radiation exposure of astronauts in space. ICRP Publication 123. Ann. ICRP 42, 1–339. doi:10.1016/j.icrp.2013.05.004
ICRP60 (1991). 1990 recommendations of the international commission on radiological protection. ICRP publication 60. Ann. ICRP 21.
Jokipii, J. R., and Kota, J. (1989). The polar heliospheric magnetic field. Geophys. Res. Lett. 16, 1–4. doi:10.1029/GL016i001p00001
Langner, U. W., and Potgieter, M. S. (2004). Solar wind termination shock and heliosheath effects on the modulation of protons and antiprotons. J. Geophys. Res. Space Phys. 109. doi:10.1029/2003JA010158
Langner, U. W., Potgieter, M. S., and Webber, W. R. (2003). Modulation of cosmic ray protons in the heliosheath. J. Geophys. Res. Space Phys. 108. doi:10.1029/2003JA009934
Li, H., Wang, C., and Richardson, J. D. (2008). Properties of the termination shock observed by voyager 2. Geophys. Res. Lett. 35. doi:10.1029/2008GL034869
Liu, W., Guo, J., Wang, Y., and Slaba, T. C. (2024). A comprehensive comparison of various galactic cosmic-ray models to the state-of-the-art particle and radiation measurements. Astrophysical J. Suppl. Ser. 271, 18. doi:10.3847/1538-4365/ad18ad
Marcelli, N., Boezio, M., Lenni, A., Menn, W., Munini, R., Aslam, O. P. M., et al. (2020). Time dependence of the flux of helium nuclei in cosmic rays measured by the pamela experiment between 2006 july and 2009 december. Astrophys. J. 893, 145. doi:10.3847/1538-4357/ab80c2
Martucci, M., Munini, R., Boezio, M., Felice, V. D., Adriani, O., Barbarino, G. C., et al. (2018). Proton fluxes measured by the PAMELA experiment from the minimum to the maximum solar activity for solar cycle 24. Astrophys. J. 854, L2. doi:10.3847/2041-8213/aaa9b2
Matthiä, D., Berger, T., Mrigakshi, A. I., and Reitz, G. (2013). A ready-to-use galactic cosmic ray model. Adv. Space Res. 51, 328. doi:10.1016/j.asr.2012.09.022
Moraal, H., and Potgieter, M. S. (1982). Solutions of the spherically-symmetric cosmic-ray transport equation in interplanetary space. Astrophysics Space Sci. 84, 519–533. doi:10.1007/BF00651330
Naito, M., Kodaira, S., Ogawara, R., Tobita, K., Someya, Y., Kusumoto, T., et al. (2020). Investigation of shielding material properties for effective space radiation protection. Life Sci. Space Res. 26, 69–76. doi:10.1016/j.lssr.2020.05.001
Ngobeni, M. D., Aslam, O. P. M., Bisschoff, D., Potgieter, M. S., Ndiitwani, D. C., Boezio, M., et al. (2020). The 3d numerical modeling of the solar modulation of galactic protons and helium nuclei related to observations by pamela between 2006 and 2009. Astrophysics Space Sci. 365, 182. doi:10.1007/s10509-020-03896-1
O’Neill, P., Golge, S., and Slaba, T. C. (2015). Galactic cosmic ray flux model description. NASA Technical Reports-2015-218569
Opher, M., Richardson, J., Zank, G., Florinski, V., Giacalone, J., Sokol, J. M., et al. (2023). Solar wind with hydrogen ion charge exchange and large-scale dynamics (shield) drive science center. Front. Astronomy Space Sci. 10. doi:10.3389/fspas.2023.1143909
Potgieter, M. S. (2013). Solar modulation of cosmic rays. Living Rev. Sol. Phys. 10, 3. doi:10.12942/lrsp-2013-3
Potgieter, M. S., and Moraal, H. (1985). A drift model for the modulation of galactic cosmic rays. Astrophys. J. 294, 425–440. doi:10.1086/163309
Potgieter, M. S., Vos, E. E., Boezio, M., De Simone, N., Di Felice, V., and Formato, V. (2014). Modulation of galactic protons in the heliosphere during the unusual solar minimum of 2006 to 2009. Sol. Phys. 289, 391–406. doi:10.1007/s11207-013-0324-6
Qin, G., and Shen, Z.-N. (2017). Modulation of galactic cosmic rays in the inner heliosphere, comparing with pamela measurements. Astrophysical J. 846, 56. doi:10.3847/1538-4357/aa83ad
Qin, G., and Zhang, L.-H. (2014). The modification of the nonlinear guiding center theory. Astrophysical J. 787, 12. doi:10.1088/0004-637X/787/1/12
Raath, J. L., Potgieter, M. S., Strauss, R. D., and Kopp, A. (2016). The effects of magnetic field modifications on the solar modulation of cosmic rays with a SDE-based model. Adv. Space Res. 57, 1965–1977. doi:10.1016/j.asr.2016.01.017
Semkova, J., Koleva, R., Benghin, V., Dachev, T., Matviichuk, Y., Tomov, B., et al. (2018). Charged particles radiation measurements with liulin-mo dosimeter of frend instrument aboard exomars trace gas orbiter during the transit and in high elliptic mars orbit. Icarus 303, 53–66. doi:10.1016/j.icarus.2017.12.034
Slaba, T. C., and Whitman, K. (2019). The badhwar - O’neill 2020 model. NASA Technical Reports-2019-220419
Song, X., Luo, X., Potgieter, M. S., Liu, X., and Geng, Z. (2021). A numerical study of the solar modulation of galactic protons and helium from 2006 to 2017. Astrophys. J. Suppl. 257, 48. doi:10.3847/1538-4365/ac281c
Tomassetti, N., Barão, F., Bertucci, B., Fiandrini, E., Figueiredo, J., Lousada, J., et al. (2018). Testing diffusion of cosmic rays in the heliosphere with proton and helium data from ams. Phys. Rev. Lett. 121, 251104. doi:10.1103/PhysRevLett.121.251104
Tylka, A., Adams, J., Boberg, P., Brownstein, B., Dietrich, W., Flueckiger, E., et al. (1997). Creme96: a revision of the cosmic ray effects on micro-electronics code. IEEE Trans. Nucl. Sci. 44, 2150–2160. doi:10.1109/23.659030
Wang, B.-B., Bi, X.-J., Fang, K., Lin, S.-J., and Yin, P.-F. (2019). Time-dependent solar modulation of cosmic rays from solar minimum to solar maximum. Phys. Rev. D. 100, 063006. doi:10.1103/PhysRevD.100.063006
Zeitlin, C., Hassler, D. M., Cucinotta, F. A., Ehresmann, B., Wimmer-Schweingruber, R. F., Brinza, D. E., et al. (2013). Measurements of energetic particle radiation in transit to mars on the mars science laboratory. Science 340, 1080–1084. doi:10.1126/science.1235989
Keywords: galactic cosmic ray, solar modulation, stochastic differential equation, spatial dependence, fluence-to-dose conversion coefficient, dose equivalent rate
Citation: Song X, Huo R, Xu S, Chen X and Luo X (2025) The SDEMMA model for galactic cosmic ray and its dosimetric application. Front. Astron. Space Sci. 12:1383946. doi: 10.3389/fspas.2025.1383946
Received: 08 February 2024; Accepted: 11 March 2025;
Published: 04 April 2025.
Edited by:
Yulia Bogdanova, Rutherford Appleton Laboratory, United KingdomReviewed by:
Mike Hapgood, Rutherford Appleton Laboratory, United KingdomCopyright © 2025 Song, Huo, Xu, Chen and Luo. This is an open-access article distributed under the terms of the Creative Commons Attribution License (CC BY). The use, distribution or reproduction in other forums is permitted, provided the original author(s) and the copyright owner(s) are credited and that the original publication in this journal is cited, in accordance with accepted academic practice. No use, distribution or reproduction is permitted which does not comply with these terms.
*Correspondence: Ran Huo, aHVvckBpYXQuY24=
Disclaimer: All claims expressed in this article are solely those of the authors and do not necessarily represent those of their affiliated organizations, or those of the publisher, the editors and the reviewers. Any product that may be evaluated in this article or claim that may be made by its manufacturer is not guaranteed or endorsed by the publisher.
Research integrity at Frontiers
Learn more about the work of our research integrity team to safeguard the quality of each article we publish.