- 1Department of Meteorology, University of Reading, Reading, United Kingdom
- 2School of Earth and Space Sciences, University of Science and Technology of China, Hefei, China
- 3CAS Center for Excellence in Comparative Planetology, University of Science and Technology of China, Hefei, China
We updated annual mean reconstructions of near-Earth interplanetary conditions and (signed) open solar flux FS for the past 186 years. Furthermore, we added observations for solar cycle 24 to refine regressions and improved allowance for orthogardenhose and folded (a.k.a., switchback) heliospheric flux from studies using strahl electrons. We also improved the allowance made for the annual mean gardenhose angle of the interplanetary magnetic field. We used both multiple regression with interplanetary magnetic field B and solar wind speed VSW and linear regression with the function
1 Introduction
The potential to quantitatively reconstruct the interplanetary conditions of the previous 100 years from geomagnetic observations was foreseen by Russell (1975) and first attempted by Feynman and Crooker (1978) and Gringauz (1981). These early attempts used the aa geomagnetic index, initially constructed for 1868–1968 by Mayaud (1972) from observations made by geomagnetic observatories in southern England and in Australia. The aa index is the arithmetic mean of the northern and southern hemisphere subindices, aaN and aaS, both of which are constructed from the range (between minimum and maximum) of the irregular variation (i.e., after elimination of the regular daily variation), observed in 3-h intervals in either of the two horizontal components (northward or eastward, whichever gives the larger value). This range is then quantized, using quasi-logarithmic band limits that are specific to the observatory, into k indices, which are converted to ak values using a standard scale. This procedure was first introduced by Bartels et al. (1939) and has been discussed in reviews by Lockwood et al. (2018a) and Lockwood et al. (2019a). In the northern hemisphere, the aa stations used are Greenwich (IAGA code name GRW), Abinger (ABN), and Hartland (HAD), which yield the k-index data sequences kGRW, kABN, and kHAD, respectively, and intercalibrated values are combined into aaN. In the southern hemisphere, the sites are Melbourne (MEL), Toolangi (TOO), and Canberra (CNB), which yield the data sequences kMEL, kTOO, and kCNB and correspondingly formed the southern hemisphere aa index, aaS. After the work of Mayaud, the aa index has subsequently been continued to the present day.
The study by Feynman and Crooker (1978) used the derived correlation of aa with
From the data available from Lockwood et al. (1999), it was evident that there had been a significant rise in the average values of FS over the 20th century. The rise in OSF was reported to be a doubling, a factor that has been contested (e.g., Mursula et al., 2004; Svalgaard and Cliver, 2005). Next, various corrections have been required and implemented along with improvements to the method. The original reconstructions of Lockwood et al. (1999) found that the optimum rise in 11-year running, boxcar averages
Lockwood (2001), Lockwood (2003), and Lockwood et al. (2009b) showed that the OSF subsequently had reached a peak and was falling again and, as discussed later in the present study, both geomagnetic and direct in situ spacecraft observations show that
Because this 20th-century grand maximum peaked in the interval of modern spacecraft data, we here refer to it as the “modern grand solar maximum,” hereafter “MGSM.” There is great interest in this grand maximum because of its potential to tell us about solar evolution, long-term variations in space weather, and the solar magnetic cycle (see studies in the collection edited by Banerjee et al., 2018).
2 Modern grand solar maximum in monitoring data sequences
The MGSM can be seen in several datasets that extend back far enough in time and that continue to the present day. Figure 1 shows two examples, sunspot numbers and the homogeneous aa index, aaH. The top panel shows the newly recalibrated international sunspot number, RISN (Clette and Lefèvre, 2016), and the revised sunspot group number, RG (Vaquero et al., 2016). The latter is scaled by a factor f, the ratio of the means over the interval 1978–2018.
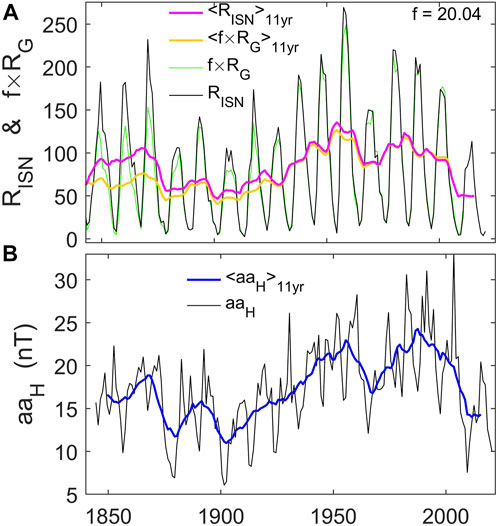
FIGURE 1. Annual means and 11-year running means of (A) sunspot numbers and (B) the homogeneous aa index (aaH). For the sunspot numbers, the black and green lines are the (newly revised) international sunspot number (RISN) and the group sunspot number (RG, here plotted scaled by a factor f, which is the ratio of mean values of RISN and RG over the years 1978–2018). The thick mauve and orange lines indicate the corresponding 11-year running means (
The aaH index is based on the same observations as Mayaud’s classic aa index, but the long-term drifts in the magnetic latitudes of the stations are allowed for by correcting for the distances between the stations and an average auroral oval location. This is done using the IGRF model and (for before 1900) the gufm1 model of the intrinsic geomagnetic field. This is a considerable advance on the original aa index for which these distances were used but assumed constant for each station (Lockwood et al., 2018a). In addition, improved intercalibrations (that allow for the time of year) between data from different stations were used, along with a model to remove the time-of-year/time-of-day effects at a given station location (Lockwood et al., 2018b). These changes result in aaH, unlike aa, having two hemispheric subindices (aaHN and aaHS for the northern and southern hemispheres, respectively) with almost identical long-term variations, despite them being entirely independent in their derivation. In other words, the much better agreement of aaHN and aaHS (compared with that for aaN and aaS of the original aa index) was achieved from the improvements to the derivation algorithm and not by any intercalibration to bring them closer together. The aaH index data series runs from 1868 to the present day and here has been extended back to 1844 using the k-index data from the Helsinki and St. Petersburg magnetometers, using the procedure described by Lockwood et al. (2013a) and Lockwood et al. (2014b). The history of the development of this extension is reviewed in Section 4 of Lockwood et al. (2014b).
In both panels of Figure 1, the thinner lines are annual means, and the thicker lines are 11-point boxcar (running) means of the annual data. After about 1925, the behaviors of RG and RISN are very similar. Sunspot numbers have minima that return to close to zero in every cycle, although there is some cycle-to-cycle variation in the minima as well as in the maxima. The 11-year running means show two clear peaks, the first being the larger of the two because of the exceptionally large amplitude of solar cycle 19 (June 1954 to October 1964). The 11-year averages of cycle 24 (December 2008 to December 2018) are almost exactly the same as they had been in cycle 14 (January 1902 to July 1913) with
Figure 2 shows this grand maximum in two other long solar–terrestrial observation sequences in the same format as Figure 1, both of which commence in the early 1930s. The upper panel is the ionospheric F2 peak critical frequency, foF2, from the intercalibrated Slough and Chilton ionosondes. Near-noon values are shown, being the means of values taken at 11, 12, and 13 UT. The double peak is similar to that in sunspot numbers, and the variation is consistent with that in RISN, given the nonlinear regression of annual means of these foF2 data and RISN, as demonstrated by Lockwood et al. (2016b). Note that the fractional change in foF2 is smaller than that for RISN because the annual means of foF2 fall to minima of approximately 5 MHz, whereas RISN falls to minima near zero. The bottom panel is for the planetary ap geomagnetic range index. This is quite similar to the plot for aaH given in Figure 1A. However, there are some important differences. In particular, the first of the two peaks of the MGSM is the larger for ⟨ap⟩11, whereas for
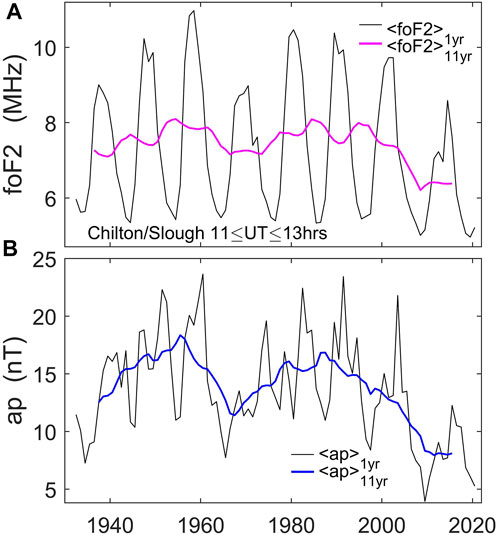
FIGURE 2. Annual means and 11-year running means of (A) the ionospheric F2-layer critical frequency, foF2, seen at Slough/Chilton between 11 and 13 UT and (B) the geomagnetic range index ap.
3 Calibration and checking of geomagnetic data
It is self-evident that the reconstruction of past solar and interplanetary conditions from historic geomagnetic data critically depends on the stability of the calibration of those data. There have been a number of instances of calibration skips and drifts in data from a given station that have emerged from comparisons with data from different stations. For example, in developing the IHV index (Svalgaard et al., 2004; Svalgaard and Cliver, 2007) using data from many stations, Leif Svalgaard in 2004 noted in a letter to the data curators that there appeared to be an error in the early hourly mean data from the Eskdalemuir station, something also noted by Martini and Mursula (2006). From careful comparisons with data from nearby stations, this error was corrected by Macmillan and Clarke (2011). Another example was poor calibration of the horizontal force variometer at Helsinki for a 6-year interval noted by Svalgaard (2014), which was causing the horizontal H component to be too small; Lockwood et al. (2014a) also found that correcting for this using data on the vertical Z component indeed brought the data in line with the observations from other stations, particularly at the nearby St. Petersburg station.
Such corrections have been very valuable and important. However, there is a potential and important pitfall here of which we need to remain aware or we will make erroneous corrections that would be a serious retrograde step. The key point is that if the data sequences from two stations diverge, there are a number of possibilities: first, one of the data sequences is in error (in which case, which one?); second, they could both be in error; third, they could both be correct, and it is the expectation that they should be the same that is in error. The first and second possibilities are self-evident, but the third is more subtle. We know that the geomagnetic activity response depends on the geomagnetic latitude of the station. The secular changes in Earth’s intrinsic magnetic field mean that data from different stations will drift relative to one another (see Lockwood et al., 2018a), and we must be careful not to falsely ascribe these changes to measurement error. The reason is that making a correction based on the assumption that the difference is because of an instrumental error effect means that the variation will be in error when we apply a correction for the secular drift of the station. Furthermore, the sensitivities of the geomagnetic observations to variations in the IMF, B, and solar wind speed, VSW, depend on the station location. Therefore, correcting data from two stations to make them agree, on the false assumption that they should agree, will cause errors in both the derived B and VSW. Another potential pitfall of making corrections is the wrong assumption that the geomagnetic activity data from different stations are linearly proportional because, in reality, local site conditions may mean this is not the case.
As expected, if we have one station that disagrees with several others in similar locations on a given measurement, it is an indication that it has a calibration problem, and this is best dealt with by using the median of the distribution of all the available values (with it being less prone to distortion by poorly calibrated stations than the mean value). The problem is that as we go back in time, we have fewer operating stations, and so the uncertainties associated with using the ensemble of all available stations inevitably increase. Furthermore, the geographic distribution of those stations changes, which introduces an inhomogeneity into the data series. For this reason, the second approach is to use only a small number of stations but try to make the data from them as homogeneous as possible (including allowing for the secular drift in geomagnetic latitude and site differences). Hence, there are two philosophies to generating a reliable geomagnetic data sequence to extend modern spacecraft data on the interplanetary medium back in time: (A) take the mean, or preferably the median, of the distribution of all the stations available at a given time and (B) use a limited number of stations but do all one can to ensure the data series is homogeneous in the sense that data from the past is consistent with the data from the space age (the latter being the data that are compared with interplanetary observations). Approach A has the advantage of using all the available data but the disadvantage that the number of stations decreases as we go back in time, thereby increasing the uncertainties. This also means that the data for early years is not from the same network of station locations as that during the space age. When the number of stations is high, such an index is genuinely global, but as one goes back in time, it becomes increasingly local, applicable only to where the few early stations were sited and not global in nature. Hence, it is not true that using all the data available is necessarily the best approach. Approach B could, in theory, achieve uncertainties that are close to being constant throughout the data series and at all times using data obtained from stations reasonably close to the stations giving the data during the space age. Philosophy B uses model values of the intrinsic magnetic field to allow for the (changing) magnetic latitude of stations and so turn the local measurements into a global estimate. However, it is not deploying all the data available and so is more prone to errors introduced by station calibration errors.
The IDV (interdiurnal variability) geomagnetic index (Svalgaard and Cliver, 2005) and IHV (interhour variability) geomagnetic index (Svalgaard et al., 2004; Svalgaard and Cliver, 2007) are examples of the application of philosophy A, whereas the aaH (Lockwood et al., 2018a,b) and IDV(1d) (Lockwood et al., 2013a,b, 2014a) indices are examples of the application of philosophy B.
Given that geomagnetic responses vary with location, we should not attempt to make all the geomagnetic data agree, because that is based on the incorrect assumption that they should all agree. However, when we use geomagnetic data to reconstruct interplanetary conditions, the results should not depend on where on the surface of the Earth the data come from; hence comparing the interplanetary reconstructions obtained using different geomagnetic data sequences is the most valid test.
3.1 Comparison of the homogeneous aa index and the corresponding k-index data composite from the Potsdam, Seddin, and Niemegk sites
Many of the criticisms of the doubling of the OSF derived by Lockwood et al. (1999) were based on concerns on the calibration of the aa index, some of which were valid, whereas others were not. These mainly centered on apparent calibration skips between the data from different stations in the deployed sequences. However, this was a misunderstanding of the methodology that Mayaud (1972) had adopted when generating the aa composite. Mayaud did not make a year-by-year correction to allow for the effect of the secular drift of the geomagnetic locations of the stations and then “daisy-chain” the data sequences (i.e., link them using intercalibration between the end of the prior sequence and the start of the next one). Rather, he used an average sensitivity for each station and computed for the mid-point of the data sequence from that station. Hence, Mayaud effectively allowed for secular change in the station latitude, but only in discrete steps at the joins of the data sequences between stations. These steps have been wrongly interpreted as “daisy-chaining” calibration errors. This factor has been properly allowed for in the “homogeneous aa” (aaH) dataset (Lockwood et al., 2018a,b), for which site sensitivity at a given date is corrected for on an annual basis using a spline of the IGRF and gufm1 geomagnetic field models from historic and paleomagnetic observations. The data sequences can then be daisy-chained and the calibrations checked at the joins between the station data sequences. Doing this resulted in the data sequences for the northern and southern hemisphere subindices being much more similar for aaH than they are for aa, even though at no stage was a comparison of the two used in the derivation of either (Lockwood et al., 2018a,b).
A long, composite sequence of corresponding k-index data is available from the combination of three nearby magnetometer stations, namely, Potsdam, Seddin, and Niemegk in Germany. We here refer to the latitude-corrected k-index data composite from these three stations as kNGK (generated using the correction from the IGRF and gufm1 models in the same way as in the production of aaHN and aaHS), and the mean annual values are compared with the aaH data sequence in the top panels of Figure 3. The scatter plot on the right is between kNGK and aaH for the last 51 years (1970–2020, inclusive), and the data points have been fitted with a linear regression line (mauve) and a second-order polynomial fit (blue). These are then used to scale the kNGK data, which are then compared with aaH (the black line) in the time series shown to the left. The linear fit (mauve line) gives minima in kNGK around 1902 and 1912, which are deeper than the corresponding minima in aaH. If we look at the deep minimum in 2009, we see the same behavior. The correlation coefficients are r = 0.978 and r = 0.983 for the linear and quadratic fits, respectively. We applied the Meng-Z test for the significance between two correlations (allowing for the intercorrelation of the parameters) (Meng et al., 1992); the resulting p-value for the null hypothesis that there is no significant difference between the two correlations was 0.04. Thus, the difference in correlation is significant at the 2σ level. We also computed the Akaike information criterion (AIC) (Akaike, 1974), which were 24.70 and 14.45 for the linear and quadratic fits, which gives an information ratio of 168 (meaning the quadratic fit is 168 times more likely to be correct than the linear fit). We also fitted with a third-order polynomial: the correlation coefficient was r = 0.983 (identical to the quadratic fit), and the AIC was 16.26; this makes the third-order fit less likely than the quadratic fit by an information ratio factor of 2.5, which means the third-order fit suffers from overfitting. From these tests, we used the quadratic fit, and this made a large improvement to the agreement of the early sunspot-minimum values. Hence, if we assumed that the Potsdam composite was correct and that the relationship was linear, we might wish to (erroneously) correct these minima in the aaH data. However, the scatter plot for the past 50 years suggests that the variation is not linear, and when we use a second-order polynomial fit (the blue lines), we find excellent agreement between aaH and kNGK at all times. Hence, aaH is fully consistent with the latitude-corrected Niemegk composite k-index data, as long as we do not impose a (false) expectation of a linear dependence.
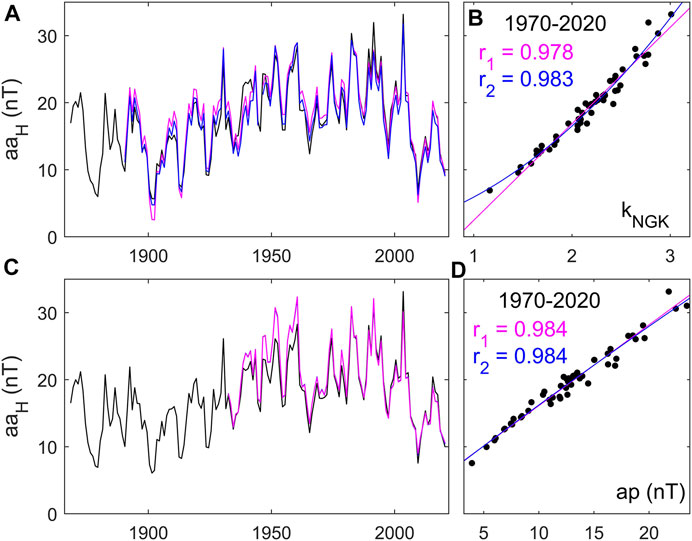
FIGURE 3. (Top) annual means of the aaH index compared with scaled annual means of the k value composite kNGK from the Potsdam, Seddin, and Niemegk magnetometers. The scatter plot in part (B) shows linear (in mauve) and second-order polynomial (in blue) fits to the modern data (1970–2020), which give correlations of 0.978 and 0.984. These fits are used to scale the kNGK data in the full time series shown in part (A), using the same colors as in (B), and these scaled kNGK variations are compared with the aaH data in black. (Bottom) The same for the aaH index and the ap index with the variations shown in (C) and the scatter plot for 1970–2020 shown in (D). In this case, there is almost no difference between the linear and second-order polynomial fits.
3.2 Comparison of the homogeneous aa, interhour variability, and ap indices
The bottom panel of Figure 3 makes a similar comparison of the aaH index with the ap index. The ap (and hence kp) index is currently made from data from 11 stations in the northern hemisphere and two in the southern. It has been continuously available since 1 January 1932, although the number and distribution of stations employed have significantly varied since then: there were initially 10 stations all in the northern hemisphere, the first southern hemisphere station being added in 1958. The bottom right plot of Figure 3 is the scatter plot for 1970–2020 and shows that the linear fit for these data is essentially as good as the quadratic fit. Hence, there is no justification for anything beyond a linear fit. However, when we look at the time series of these fits, we see that although agreement is very good after 1960, before then, aaH is consistently lower than ap. Because it is based on more stations, it might be concluded that it is aaH that is in error. However, if we consider how ap is constructed, the upper panels of Figure 3 present a major problem for this argument. The ap index is constructed using k-index data from a number of mid-latitude stations. The k indices are first converted into standardized values, ks, to account for the time of year and UT response characteristics of the observing site and to, as far as possible, normalize them to the values seen simultaneously by Niemegk, kNGK, which was selected as the reference station. The standardization from k to ks is achieved using conversion tables for each observatory that were defined for the original stations by Bartels (1949, 1957). These give a multiplication factor ks/k that depends on the station location, UT, time of year, and k value. The presently used conversion tables are for three seasons, and many were generated using selected data from 1943 to 1948 only. The three seasons are as follows: (1) the months around winter solstice (January, February, November, and December), (2) the months around the equinoxes (March, April, September, and October), and (3) the months around summer solstice (May, June, July, and August). If this conversion were done adequately for our purposes, we would therefore expect all the ks values (and hence ap) to show the same level of agreement over time as presented in the top panel for kNGK. That this is not the case shows the difference is arising from the method of compilation of ap, and it must be remembered that this is not homogeneous: the number and geographical distribution of the stations used have significantly changed (Lockwood et al., 2019a), and no allowance for secular change in the geomagnetic latitude of stations is made in the lookup tables of the factor ks/k. Further evidence that the long-term change in aaH is correct (and hence that it is not in ap) is provided in Figures 13 and 14 of Lockwood et al. (2017), which show that the long time series of k indices observed at Eskdalemuir, Potsdam/Seddin/Neimegk, and Sondankylä were also the same (to a very high degree of accuracy) as aaH, once allowance was made for the geomagnetic latitudes of the stations and how they varied because of the secular change in the intrinsic geomagnetic field (using the same procedure as was used to make the corresponding allowance for the aaH stations). Hence, although it is constructed from more stations, ap is not suitable for the task of reconstructing past interplanetary conditions and makes the point that a homogeneous nature of geomagnetic data sequences is very important in the context of these reconstructions.
There are other reasons to be confident about the accuracy of aaH, summarized in the top panels in Figure 4. The variations in the annual means of the northern and southern hemisphere subindices of aaH (aaHN and aaHS, respectively) are indicated by red and blue lines in Part A, and a scatter plot is given in Part B. The most significant difference arises at the lowest values, and Part A shows that these are because either the earliest aaHN data at sunspot minimum (from Greenwich) are slightly too low or the earliest aaHS data at sunspot minimum (from Melbourne) are slightly too high. Given that the sunspot maximum values agree well at all times, this indicates a nonlinearity in the response of one (or both) of the stations. Apart from some suggestion from the IHV index that suggests that the Greenwich data are correct, we have no clear evidence as to which station is the cause (or indeed if it is both), and in using aaH, we average the two. As stated above, the agreement is very good: the correlation coefficient is r = 0.983, even with the slight divergence of the earliest sunspot minimum values. As discussed by Lockwood et al. (2006), Lockwood and McWilliams (2021), and Sivadas and Sibeck (2022), correlation can be a limited metric for assessing the similarity of two data series, and in this work, we routinely looked at some other metrics of agreement. These are the number of samples, nr; the effective number of independent samples allowing for the persistence of the two data series (Thiébaux and Zwiers, 1984), ne; the p-value of the null hypothesis that there is no correlation, allowing for the persistence of the two data series, pr; the root-mean-square fit residual as a percentage of the mean value, Δrms; the p-value for the null hypothesis that the residuals are not normally distributed, derived from a chi-squared test, pnorm; and the p-value that the fit is not fully homoskedastic (i.e., that the fit residuals have some variation with the fitted value), from the heteroskedastic test of White (1980), phet. For the correlation between aaHN and aaHS, nr = 153, ne = 24.4, pr < 10–20, Δrms = 6.58%, pnorm = 0.002, and phet = 0.181. Hence, the correlation in this case is very highly significant, the fit residuals are close to normally distributed, the probability of a breakdown of homoskedacity is low, and the normalized r.m.s fit residual is small. For all further correlations quoted in this study, these metrics are given in Supplementary Table S1 of the supplementary material. In addition, quantile–quantile plots were generated for every correlation and visually inspected to check that they were close to linear in form (and so the distributions of the two parameters were similar); fit residuals were plotted as a function of fitted value to give a visual check on homoskedacity.
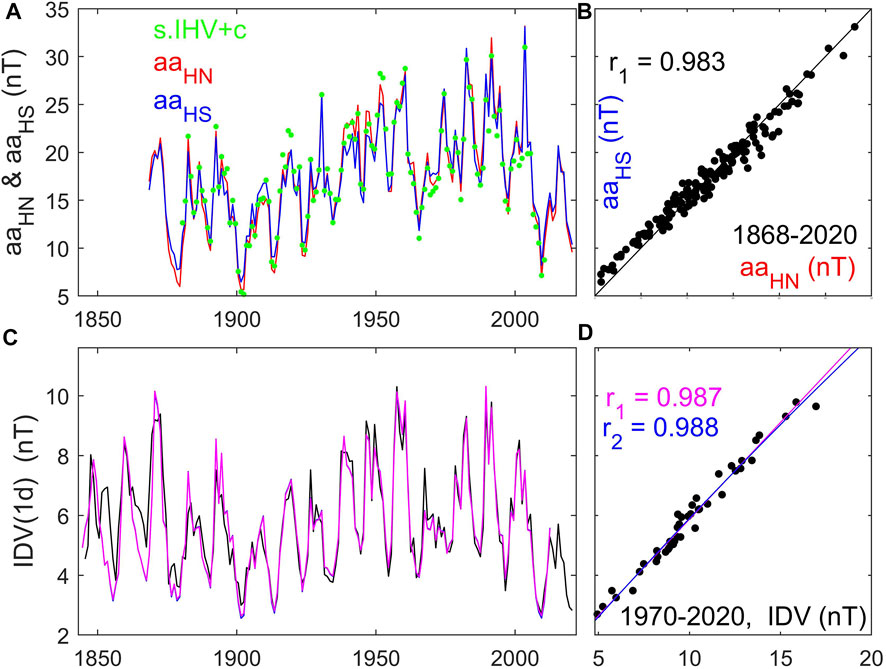
FIGURE 4. (Top panels) Comparison of annual means of the northern and southern hemisphere subindices of aaH (aaHN and aaHS, shown by the red and blue lines, respectively): (A) as a time series and (B) as a scatter plot (correlation coefficient 0.983 for the full 1868–2020 data series). The green dots in (A) are linearly scaled annual means of IHV. (Bottom panels) Comparison of the IDV(1d) and IDV indices in the same format as the upper panel. (C) annual means of IDV(1d) compared with scaled annual means of IDV. The scatter plot in (D) shows linear (in mauve) and second-order polynomial (in blue) fits to the modern data (1970–2020), which give correlation coefficients of 0.987 and 0.988. These fits are used to scale the IDV(1d) data in the full time series shown in (C) using the same colors as in (D) and are compared with IDV in black.
The good correlation between aaHN and aaHS is important because although they are generated using the same procedure, the data contributing to aaHN plays no part in the derivation of aaHS, nor vice versa. Hence, aaHN and aaHS are entirely independent and yet agree with each other very closely. The green dots in Figure 4A are linearly scaled annual means of the IHV index derived by Svalgaard et al. (2004) and Svalgaard and Cliver (2007) using hourly means from many stations. These points indicate that the IHV index is very similar to both aaHN and aaHS. IHV for a given station is defined as the sum of the absolute values of the difference between hourly means for a specified geomagnetic component from 1 h to the next over the 7-h interval around local midnight. An average of the mean and median of all available stations is then taken, and so it is an example of an index generated by philosophy A. Hence, IHV is quite different in its construction from aaH, which is derived from 3-hourly k-values using philosophy B, and yet the two closely agree. This becomes highly significant when we consider that Lockwood et al. (2014b) have shown that IHV and aaH have almost identical dependencies on interplanetary parameters, a fact that is confirmed in the present study using data sequences that contain an extra solar cycle. This being the case, we expect IHV and aaH to show very similar variations, and Figure 4 confirms that they do.
3.3 Testing the interdiurnal variability indices
The IDV index used here was derived by Svalgaard and Cliver (2010), inspired by the u-index of Bartels (1932). The u-index was defined as the weighted means of data, from a variety of stations, on the absolute value of the difference between the mean values of the horizontal (H) or vertical (Z) component (whichever yields the larger value) for the day in question and for the preceding day. The main difference between u and IDV is that to further suppress contamination by changes in the regular diurnal variation (most, but not all, of which is removed from u by taking daily means), IDV only uses the hourly means (or spot values if hourly means are unavailable) for the UT when the station in question is closest to local midnight. Bartels’ work on the u-index was criticized at the time for failing to register the recurrent geomagnetic storms, and as a result, he himself developed the range indices as an alternative (Bartels et al., 1939). However, as pointed out by Svalgaard and Cliver (2005), this feature is a positive advantage of the u and IDV indices as it means that it does not strongly respond, if at all, to solar wind speed variations. Thus, IDV offers a way of directly determining the IMF, which can be readily applied to a great deal of recorded historic data. One potential inhomogeneity in the data series is that most of the older observatory yearbooks contain spot values taken once every hour rather than the hourly means available in later years. Svalgaard and Cliver (2010) analyzed the IDV data sequence and (unlike for IHV, as discussed above) did not find discontinuities associated with the change from spot to hourly mean data, but one should remain aware of the difference. Because it uses all available data, IDV employs philosophy A in its construction.
The IDV(1d) index was compiled by Lockwood et al. (2013a) using IDV values but applying philosophy B rather than philosophy A. A number of station combinations were possible and investigated, and the decision of which data to use was made using the level of agreement with other stations and avoiding spot values in case they could introduce a systematic difference, albeit small. Lockwood et al. (2013a) knew that the variation of IDV(1d) should be very similar to that of IDV after about 1960, when there were sufficient stations to make philosophy A valid. However, for earlier data, and before 1872 in particular, the homogeneous construction of IDV(1d) should give better values than IDV. The composite IDV(1d) used interdiurnal variation data from Helsinki for 1845–1890 (inclusive) and 1893–1896 and from Eskdalemuir from 1911 to the present. Stations were selected that showed close agreement to the polynomial fit to all data used to allow for the varying station geomagnetic latitudes and which had close agreement with other stations, once that correction had been implemented. The gaps were filled using data from the Potsdam (1891–1892 and 1897–1907) and the nearby Seddin observatories (1908–1910), and intercalibration was achieved using short spline intervals of the Potsdam–Seddin sequence. A key point in the compilation of the index was allowance for the variation of geomagnetic latitude of each station. A survey of the dependencies on interplanetary conditions was made using data from many stations during the space age, and the derived latitudinal dependence was then used to correct the data sequences using the magnetic latitude of the stations derived from the IGRF and gufm1 models of the geomagnetic field. Lockwood et al. (2013a) tested the IDV(1d) composite against data from many other stations, again corrected for their geomagnetic latitude variation (note that this included Niemegk, NGK), and found extremely good agreement with all stations, except for some intervals of the data from Greenwich. Closer inspection of the Greenwich data showed increasing measurement errors up to the introduction of new instrumentation in 1919. As aforementioned, Svalgaard (2014) noted the poor calibration of the “horizontal force” variometer at Helsinki for a 6-year interval, and Lockwood et al. (2014a) found that correcting for this indeed brought the data in line with other local observations.
The lower panels of Figure 4 compare the IDV and IDV(1d) indices. The variations in Part C of Figure 4 show that the agreement is almost perfect after 1959. In this interval, IDV is always compiled from at least 42 stations, whereas IDV(1d) is compiled from just one. This shows that philosophy B can work just as well as A, with the caveat that care must be taken to ensure that the station used is error-free. Going back in time to 1900, some differences between IDV and IDV(1d) are seen in some years, but they are very minor and do not form a consistent trend. Over the years 1903–1958, the number of stations available to compile IDV rose from 10 to 25. Before 1900, there are more significant differences between the two, and in these years, IDV(1d) has the advantage of being homogeneous is its construction with later years and has also been checked against stations close to the Helsinki site used, such as using data from St. Petersburg. On the other hand, IDV at these times is generated using considerably fewer stations, the number being just 1 until 1880. Before 1872, IDV uses Bartels’ u-index data, which Bartels himself was not confident of, describing it as “more for illustration than for actual use.” Hence, IDV is a very different index before 1900 than for the space age when it can be compared with interplanetary data. Holappa and Mursula (2015) criticized the IDV(1d) index because it uses data from the Eskdalemuir observatory, which, despite the work of Macmillan and Clarke (2011), they argue to be inaccurately calibrated in its hourly means (but not in the k-index range data). This is of limited relevance to the IDV and IHV indices for which Eskdalemuir and its similarly operated station, Lerwick, were just two of a basket of stations used in the compilation under philosophy A, but it is important for IDV(1d) that uses Eskdalemuir data with philosophy B. The main evidence that the Eskdalemuir as in error was differences compared with the corresponding data from the Potsdam/Seddin/Niemegk composites, hereafter referred to as IDV(NGK). Holappa and Mursula (2015) attributed the differences to Eskdalemuir because of changes to the instrumentation deployed there whereas the instrumentation was not changed as radically for IDV(NGK), although of course the sites used were. Holappa and Mursula (2015) also tested the data from the Lerwick station that used similar series of instrumentation to Eskdalemuir and found larger errors; this had been noted by Lockwood et al. (2013a), which is why they rejected Lerwick as a potential dataset for compiling the IDV(1d) composite. However, Holappa and Mursula (2015) used the explicit expectation that the ratio of the values from different stations should be constant, which neglects the fact that the magnetic latitudes of both stations have changed differently. Figure 4C shows that for the years that IDV(1d) is based on Eskdalemuir data (after 1911), it agrees very well with IDV, which at these times is based on at least 12 stations. In fact, the difference between the Eskdalemuir and the Potsdam/Seddin/Niemegk data is the greatest in solar cycles 17 and 18 when IDV(1d) agrees very well with IDV, and the latter is based on more than 20 stations. Thus, the argument of Holappa and Mursula (2015) that the Eskdalemuir data are in error, even after correction by Macmillan and Clarke (2011), because they differ from the data from Potsdam/Seddin/Niemegk, is very likely to be based on the false expectation that they should be the same.
To confirm this, the top panels of Figure 5 test the IDV(1d) index, which contains allowance for the secular drift in the geomagnetic latitudes of the stations used, against the NGK composite, after the latter has been similarly corrected (Lockwood et al., 2013a). The format of the comparison is the same as that used for the k-index range tested in Figure 3. To a small extent, the difference noted by Holappa and Mursula (2015) is indeed observed, with slightly higher values seen in IDV(1d) at the peaks of cycles 16–19 (c. 1920–1960) than in the corrected Potsdam composite. Holappa and Mursula (2015) attributed this difference to errors in the Eskdalemuir data, whereas it had been noted in relation to several other stations by Lockwood et al. (2013a), who therefore attributed it to a difference in the Potsdam data, which is why they used IDV (ESK) to compile IDV(1d) after 1911 and not IDV(NGK), the latter using data from Seddin until 1931 and from Niemegk thereafter. The bottom panels of Figure 5 present an example of just how similar the Eskdalemuir data are to the results from other stations, once allowance is made for the secular changes in geomagnetic latitude. Figures 5C,D make a comparison of IDV(1d) with the results from the Tucson magnetometer (with allowance for the magnetic latitude changes of that site). This discussion highlights the points made in the Introduction section, that when two stations disagree, one has to investigate which is in error but also bear in mind that they could both be in error and/or that one’s expectation that they should be the same is at fault. On the latter point, we note that Holappa and Mursula (2015) recommended the same correction for IDV(1d) and IHV, which cannot be correct because we know these indices have different dependencies on interplanetary parameters and that those parameters have different long-term variations. Hence, we urge extreme caution in correcting stations by intercomparison because an incorrect correction is a damaging and retrograde step. Thus, Lockwood et al. (2013a) adopted the philosophy of avoiding stations that gave concern rather than correcting them and then using them. However, as discussed above, an exception to this philosophy had to be made and a correction to the early Helsinki data (for solar cycle 11) implemented as there was no adequate alternative data to deploy Lockwood et al. (2014b).
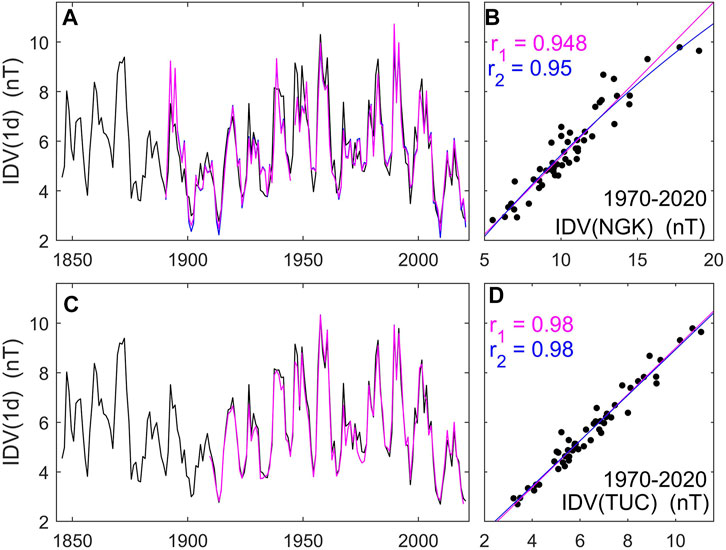
FIGURE 5. (Top) annual means of the IDV(1d) index compared with annual means of the latitude-corrected IDV value the Potsdam, Seddin, and Niemegk magnetometers, IDV(NGK). The scatter plot in (B) shows linear (in mauve) and second-order polynomial (in blue) fits to the modern data (1970–2020), with correlation coefficients of 0.948 and 0.950. These fits are used to scale the IDV(NGK) data in the full time series shown in (A) using the same colors as in (B), and these scaled variations are compared with the IDV(1d) variation in black. (Bottom: parts (C,D)) The same for the IDV(1d) index and the corresponding IDV value from the Tucson data, IDV(TUC), (for both linear and quadratic fits r = 0.980). Note that for both IDV(NGK) and IDV(TUC), the correction for the (varying) station geomagnetic latitude has been made.
4 Procedure for reconstructing near-Earth interplanetary conditions
Recent studies have confirmed that there is no such thing as a “universal coupling function,” a combination of interplanetary parameters that accurately predicts all terrestrial responses to interplanetary variations. We know this because even different geomagnetic activity indices are found to respond differently to a given set of interplanetary conditions, let alone other space weather activity indicators (Lockwood and McWilliams, 2021; Lockwood, 2022).
Table 1 presents the results of a multiple regression analysis of the four indices used in the present study, aaH, IHV, IDV(1d), and IDV. We used the MATLAB two-dimensional polynomial fitting routine “fit2dPolySVD” and evaluated the polynomial using “eval2dPoly” (Whitehead, 2021). We use polynomials of order 2 and, based on previous studies, fit using the near-Earth IMF B and solar wind speed VSW. Table 1 presents the six coefficients (a to f) that yield the predicted index, Ip1, given by
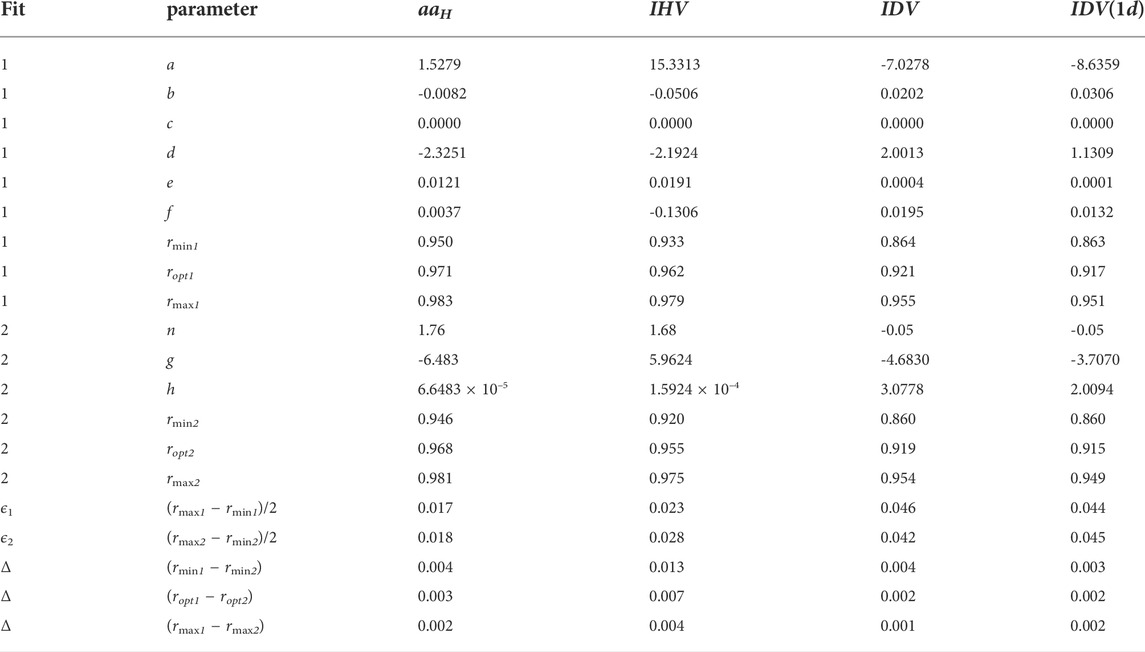
TABLE 1. Fits of near-Earth IMF B and solar wind speed VSW to the four geomagnetic indices used in this study. Fit 1 is a multiple regression using second-order polynomials, and the fitted index is given by
We then evaluate the fit by computing the correlation coefficient between the sequence of Ip1 values from the annual means of B and VSW from the Omni interplanetary data (King and Papitashvili, 2005) and the annual means of the observed index Iobs. The optimum correlation coefficient from this procedure is ropt1, and the 2σ uncertainty range in that value is between rmin1 and rmax1.
The left-hand side of Equation 1 has just two free variables, B and VSW, and so in theory, we could solve for both using just two geomagnetic indices. However, the combination of terms in Equation 1 means the solution is complex and almost certainly would require a numerical iteration technique. Table 1 demonstrates that we obtain almost as high correlations with the much simpler formulation:
which was used by Lockwood et al. (2014b) and is much more readily solved. As for Equation 1, we evaluate the minimum, optimum, and maximum correlation coefficients between Ip2 and Iobs, which were rmin2, ropt2, and rmax2, respectively. All these correlation coefficients are presented in Table 1 for each of the four Iobs indices used, along with the 2σ errors of the optimum correlations in both cases (ϵ1 and ϵ2) and the differences between the correlations for the two fit procedures Δmin = (rmin2 − rmin1), Δopt = (ropt2 − ropt1), and Δmax = (rmax2 − rmax1). Table 1 shows that all the correlations are exceptionally high, with the lowest optimum value being 0.915 and the highest being 0.971, but that the Δ values are positive, showing that using Equation 1 always generates better fits than using Equation 2, as we would expect, because it has six free fit parameters, as opposed to the three in Equation 2. However, these Δ values are very small and considerably smaller than the 2σ uncertainties, ϵ1 and ϵ2. We also applied the Meng-Z test for the significance between two correlations (allowing for the intercorrelation of the parameters) (Meng et al., 1992) and p-values for the null hypothesis that there is no significant difference between the two correlations were always above 0.85. Furthermore, we used fit residual analysis to determine the AIC of each of the fits, and again, in no case did the fit using Equation 1 give a significantly lower value than the fit using Equation 2. These tests justify using the much simpler fit procedure with fewer free fit parameters (Equation. 2).
The procedure used is a variant of that used by Lockwood et al. (2014b), but with an additional solar cycle of data now available (except in the case of IHV, which has not been updated). That extra cycle (solar cycle number 24) is much weaker than the others previously available in the space age and so extends the range of values covered by the fits, thereby reducing the degree of extrapolation involved in the reconstruction of the lower values derived for around the start of the 20th century. Figure 6 shows the variations of
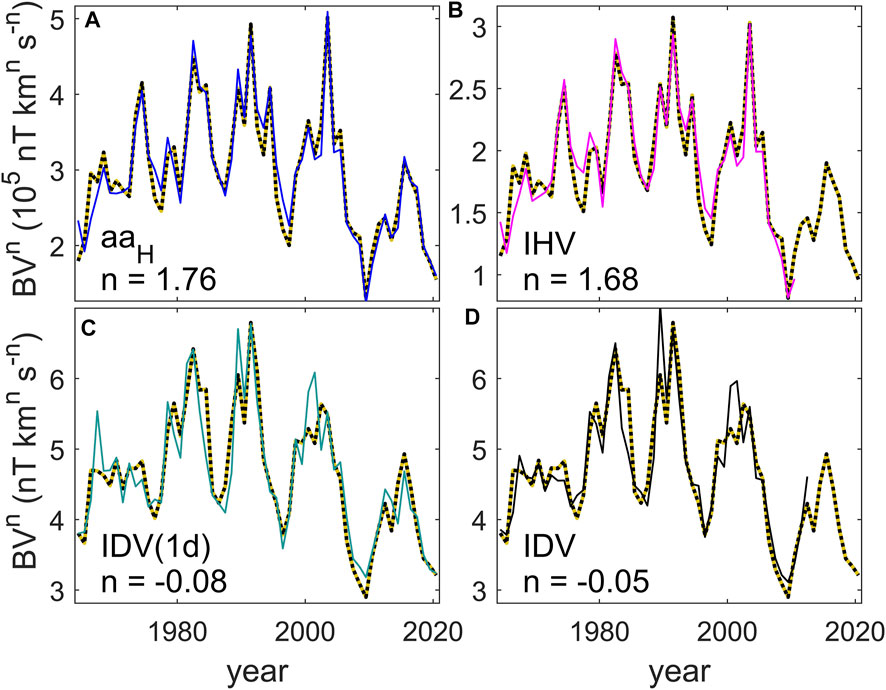
FIGURE 6. Variations of annual means of
The reconstruction procedure is the most sophisticated yet used as it is the only one (as far as we are aware) that employs a Monte Carlo technique, based on the observed fit uncertainties, to generate an ensemble of 1000 reconstructions of
Figure 7 studies how well the exponent n is defined in each case. The top panel, A, shows the correlation coefficients r2 between the index Iobs and
where N is the actual number of data pairs, and a1 is the autocorrelation at lag 1 (Wilks, 1995). The results for S as a function of n are shown in the middle panel (B) of Figure 7.
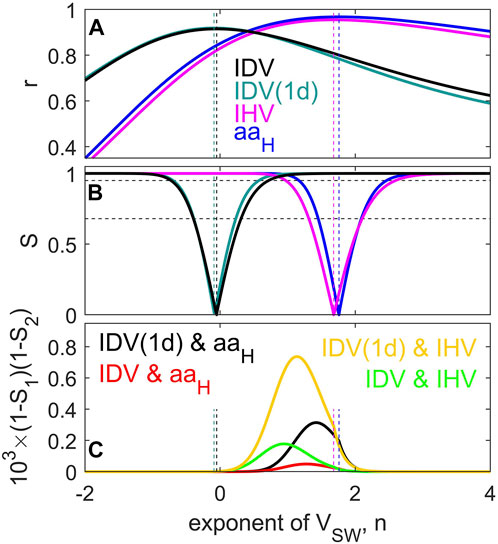
FIGURE 7. (A) correlograms showing the correlation coefficient r2 between the observed geomagnetic index Iobs and
If two indices have identical exponents n, there is no independent information in the two, and B and VSW cannot be separated—the errors in their derivation become infinite. If the n values are similar such that the difference approaches zero, the errors in B and VSW are very large and tend to infinity. For the four indices used here, there are six permutations for combining them in pairs. For two index pairs, specifically IDV and IDV(1d) and IHV and aaH, the peak correlations are at almost identical n, which means they cannot be used to separate the variations of B and VSW. This leaves four usable permutations. The bottom panel (C) of Figure 7 checks that the values of n for these indices are significantly different. The probability of index Iobs1 having a general value of n is (1 − S1), and the probability that a second index Iobs2 has the same value is (1 − S2); hence, the probability that both have that value of n is (1 − S1) (1 − S2), which is plotted as a function of n in the bottom panel. It can be seen that the most clearly distinct combination is IDV and aaH (in red) for which the peak probability of them not being distinct is 0.004% for n near 1. The least-distinct pairing is IHV and IDV(1d) (in orange) for which the peak probability is larger (0.08%) but still very small. For IHV and IDV (in green), it is 0.07%, and for IDV(1d) and aaH (in black), it is 0.06%. The uncertainties in B and VSW from a given index pairing are found to increase with the peak value of (1 − S1)×(1 − S2) and are extremely large, and indeed often infinite, for the two unused pairings, which give (1 − S1)×(1 − S2) that peaks very close to unity around the peaks of the correlations.
5 Reconstructions of near-Earth interplanetary conditions
Figure 8A shows the reconstructions of the near-Earth IMF, B. The four colored lines are the median values of the ensembles of 1 million fits for each of the four index pairings. The blue dots give the annual means of the observations by spacecraft in near-Earth interplanetary space from the Omni dataset, B′. Figure 8B presents the superposed scatter plots of B as a function of B′ for 1964–2020, inclusive, and Table 2 gives the correlation coefficients. The four best-fit least-squares linear regression fits are shown in Figure 8B and are indistinguishable. For all years after 1880, the four median reconstructions are almost identical and lying very close to the center of the gray area, which is the ±2σ uncertainty band of the ensemble of all four million values. This is the ultimate test of the geomagnetic data used to compile all four reconstructions because the interplanetary parameters do not depend on where the geomagnetic data were measured, nor on the instrumentation used, nor on the calibration of the instrumentation. Hence, if there are differences in the geomagnetic data that are used, they should be there and should not be corrected on the (false) assumption that the geomagnetic data should all agree with each other.
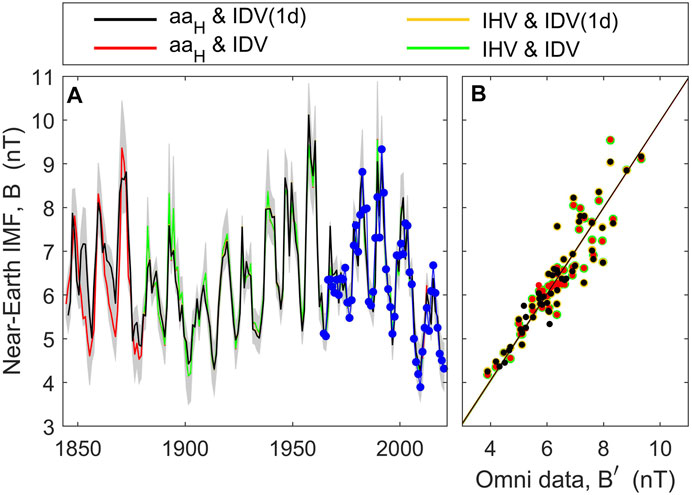
FIGURE 8. Reconstructions of the near-Earth heliospheric field; (B) reconstructions from individual index pairs are plotted as a time series in panel (A) and as a scatter plot against the means of observed values from the Omni dataset, B′, in (B). In both panels, the colors for each index pairing are as used in the bottom panel of Figure 7. The values shown are the medians for the ensemble of one million members. Because differences are generally smaller than the linewidths in panel (A), it is important to note that they are plotted in the above order, i.e., red, green, orange, and then black. Hence, for example, the fact that the orange line cannot be seen is because it is everywhere under the black line. In the scatter plot shown in part (B), the points plotted first are slightly larger, so that they can be seen. The best-fit linear regressions are also shown in (B) using the same colors. In (A), the annual means of Omni observations are shown as blue dots, and the gray area is bounded by the 2σ points of the distribution of all four million ensemble members from the four index pairings.

TABLE 2. Correlation coefficients rm of the optimum fit (the median of the ensemble, denoted by the prime) with the corresponding observed near-Earth interplanetary parameters B and VSW and the OSF FS for each of the four pairings of indices.
Before 1880, the situation is different. In this interval, the only data available are the aaH index (extended back to before 1868 using data from Helsinki, St. Petersburg, and, where possible, Greenwich), IDV(1d) and IDV (Lockwood et al., 2014a). As discussed above, IDV(1d) at this time is based on the Helsinki data (after correction for erroneous hourly means of the horizontal component during cycle 11), whereas IDV uses Bartels’ u-index, which he regarded as illustrative only for most of the interval. The aaH–IDV(1d) pairing gives the black line and the aaH–IDV pairing the red line, and we can see that before 1880, the two give quite similar variations but do diverge somewhat. The procedure at this time generates ensembles of 2 million members: the median of the total ensemble is between the red and black lines, in which the ±2σ uncertainty band is somewhat broader than that for later years.
Figure 9 shows the corresponding four median reconstructions of the near-Earth solar wind speed, VSW. Again, the annual mean from the Omni dataset of spacecraft observations
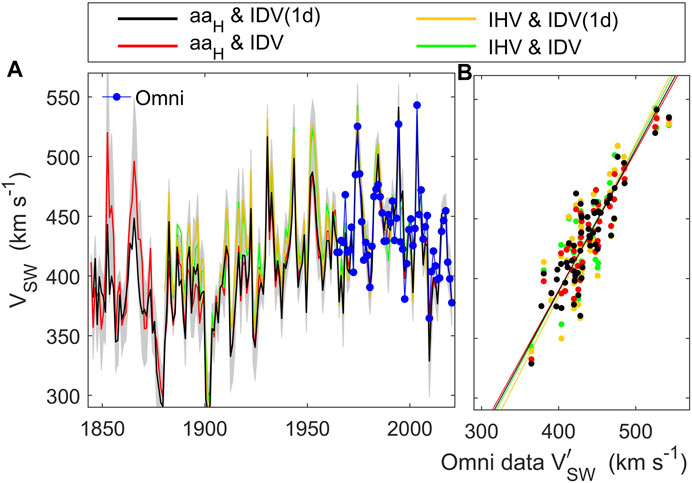
FIGURE 9. The same as Figure 8 for the near-Earth solar wind speed, VSW.
6 Reconstruction of open solar flux
We here use a development of the procedure of Lockwood et al. (2014b) to compute the (signed) OSF FS. We use the Parker spiral theory, and we need to evaluate its accuracy and make any correction required.
Figure 10 shows the results of a survey of daily mean values of the interplanetary data and compares the observed IMF “gardenhose” angle with those predicted by the Parker spiral theory. Polar histograms of the observed (gray filled, θobs) and predicted (mauve outlined, θp) distributions of the IMF gardenhose angle are presented for daily means of the near-continuous interplanetary observations from 1995 to 2020, where
and BY and BX are the Y and X IMF components in the GSE frame, respectively. The plots are for 12 ranges of observed solar wind speed, VSW, each containing one 12th of the total number of samples in the dataset. The predictions use the daily mean VSW value and Parker spiral theory
where ω is the angular velocity of the solar corona with respect to the fixed stars, and r is heliocentric distance. A corresponding plot for hourly means was presented by Lockwood et al. (2019), who studied the causes of the largest deviations from Parker spiral orientations, namely, orthogardenhose flux. We predict the modulus of the radial field
where β is the angle between the IMF vector
where
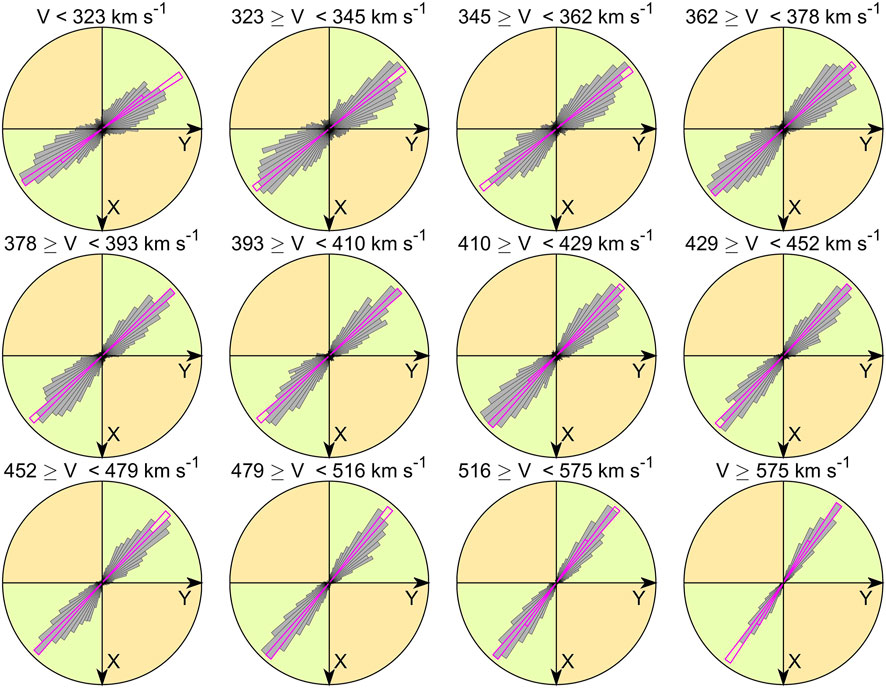
FIGURE 10. Polar histograms of interplanetary magnetic field (IMF) gardenhose angle distributions, both observed (gray filled,θobs) and predicted (mauve outlined, θp), where θ = tan−1 (−BY/BX) for daily means of the near-continuous interplanetary observations from 1995 to 2020. The plots are for 12 ranges of observed solar wind speed, VSW, each containing 639 samples (one 12th of the total total number of samples in the dataset, which is 7668). The predictions use the daily mean VSW value and Parker spiral theory. The corresponding plot for hourly means was presented by Lockwood et al. (2019).
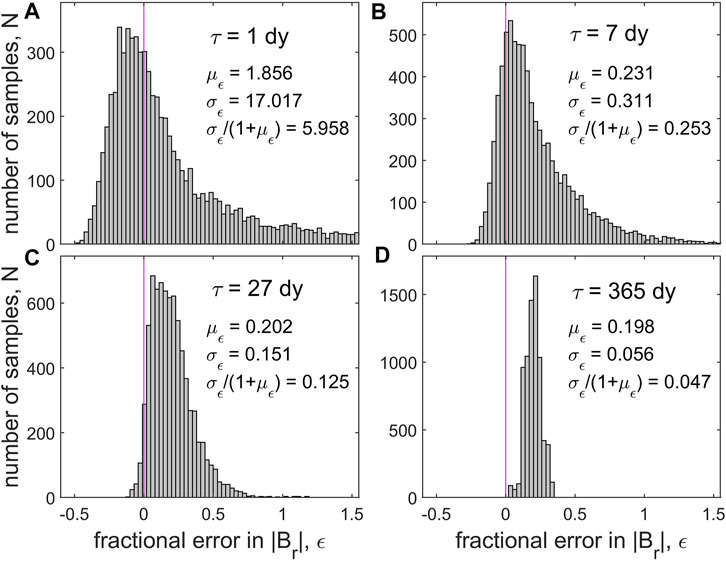
FIGURE 11. Histograms of the fractional error in the absolute value of the radial IMF generated from the Parker spiral theory using the observed solar wind speed VSW and IMF magnitude B from the same dataset used in Figure 10. The fractional error ϵ is defined as (Bcosθp/|[Br]obs|) − 1, where |[Br]obs| is the observed radial component of the IMF, and θp = tan−1 (r.ω/VSW) is the predicted garden house angle, where ω is the angular velocity of the solar corona with respect to the fixed stars, and r is heliocentric distance. Plots are for boxcar running means of interplanetary data over timescales τ of (A) 1 day, (B) 7 days, (C) 27 days, and (D) 1 year. The vertical mauve lines indicate perfect prediction of the observations (ϵ = 0), and the mean, μϵ, and the standard deviation, σϵ, of the distribution of the ϵ values is given in each panel, along with the 1σ error in using the procedure described in the text to compute Br.
The mean μϵ and standard deviation σϵ of the distribution of ϵ values are given in each panel along with the 1σ fractional error in using the procedure and then correcting for the offset of the mean value, σϵ/(1 + μϵ). For daily means, that error is by a factor of close to 6, but the distribution narrows as τ is increased, such that for τ = 7 days, the error is 25%; for τ = 27 days, it is 12.5%; and for τ = 1 year, it is just below 5%.
We also have to consider another factor that has been given a variety of names: “excess flux” (Lockwood et al., 2009b,a; Lockwood and Owens, 2009), “inversions” (Owens et al., 2013), “folded flux” (Owens et al., 2017b), and “switchbacks” (e.g., Mozer et al., 2020). The radial heliospheric magnetic field (HMF) can be used to compute the OSF because of two factors. First, we are averaging out any structure in the Carrington longitude by taking means over several solar rotations for annual means at Earth (specifically 13.42 rotations in a leap year and 13.38 in a non-leap year). The second factor is that the Ulysses satellite showed that the radial component of the heliospheric field was approximately independent of heliographic latitude (Balogh et al., 1995; Smith and Balogh, 1995), a result we can understand considering that close to the Sun, the plasma beta is low, i.e., pressure is dominated by the magnetic field. This means solar wind flows close to the Sun are slightly nonradial until the tangential pressure is equalized, which means that the modulus of the radial magnetic field |Br| is equalized, and this is carried out by the radial solar wind flow into the heliosphere (Suess and Smith, 1996; Suess et al., 1996, 1998). The tangential magnetic pressure depends on the radial field of either polarity (toward or away from the Sun), and this is why it is the modulus of the radial field that is constant. At a heliocentric distance r, the unsigned magnetic flux (of either polarity) threading the surface of radius r is 4πr2|Br|. Owens et al. (2008) surveyed radial field measurements throughout the heliosphere and showed that although |Br| remained approximately independent of latitude at all r, the unsigned flux FS increased with r. We here use signed flux (of one polarity), and Maxwell’s equation (
where Δ is the “excess flux” that increases with r (Lockwood and Owens, 2009). This is the magnetic flux that threads the coronal source surface (with either “Toward,” T, or “Away,” A, field polarity in the − r and + r directions, respectively) and loops back toward the Sun in the heliosphere (termed an “inversion,” a “switchback,” or “folded flux”) so that it threads a surface at a given heliocentric distance r more than once. Excess flux also includes orthogardenhose flux tubes that are also bent back toward the Sun but by a smaller angle, such that they are no longer in the gardenhose sectors of IMF orientation. If it does this once, such a heliospheric flux tube containing OSF flux dFS of A polarity at the coronal source surface contributes flux 2. dFS to total A flux threading the sphere of radius r and adds dFS to the total T flux threading the sphere. Likewise, if the flux dFS at the source surface is of T polarity, it contributes 2. dFS to the total T flux threading the sphere of radius r and dFS to the total A flux threading the sphere. In both cases, the excess flux added is Δ = 2. dFS (Lockwood and Owens, 2013). If the flux tube were to do this twice, it would add Δ = 4. dFS, and so on. Note that excess flux is undoubtedly a real physical phenomenon and cannot be dismissed as noise or an artifact of using a modulus—indeed, the use of the modulus is necessary here to avoid artifacts induced by averaging over a region containing two opposite polarities. Note that the theory of why the heliospheric radial field is constant (Suess and Smith, 1996; Suess et al., 1996, 1998) shows that it is the modulus that we should use because both the T and A fields contribute to the tangential field pressure. Note also that excess flux has two causes. The first is orthogardenhose flux, where flux tubes are bent back toward the Sun but by an angle large enough to take it out of the gardenhose IMF orientation sector but not large enough to take it into the gardenhose sector of the opposite T/A polarity. The second is folded flux (a.k.a. switchbacks), where the flux tube is bent back by an angle large enough (approaching 180°) for it to remain in a gardenhose orientation sector (but becomes T flux where it was A or vice versa). Both contribute to excess flux, but only in the orthogardenhose flux case can it be identified from the field orientation. Recent results from the Parker Solar Probe mission showed how common switchbacks are in the inner heliosphere (e.g., Mozer et al., 2020), and so accurately computing the excess flux Δ is of great importance.
However, there is no easy way to compute the total excess flux, Δ. From potential field source surface (PFSS) modeling, we can identify the polarity of the radial field at the source surface and average over the T and A sectors of the source surface. If we could identify where sector boundaries in the source surface map to in the satellite data, we could average the signed Br over the intervals of one source polarity (it is important to note that we need source polarity, not polarity at the spacecraft), which would cancel excess flux because the additional + dFS of A flux of any orthogardenhose or folded flux tube would cancel the −dFS of the corresponding T flux. Smith and Balogh (1995) first identified the latitudinal invariance of the radial field (often referred to as the “Ulysses result”) by assuming that they could define the source sector boundaries from the field polarity at the spacecraft. However, the T/A polarity at the source surface does not always stay the same all the way to the spacecraft, and so, we cannot, in general, identify the source sector boundaries in the satellite data. For example, if there is a short interval of opposite polarity radial field in a sector, one can never know if it is folded flux (and so does not appear in the source surface) or if it reflects real polarity structure on the source surface. Hence, this method does not provide a self-consistent method, and we cannot rely on this method to average out Δ (Lockwood and Owens, 2009). In practice, comparison of OSF values from the (PFSS) modeling of the corona can be made to agree by simply averaging the radial heliospheric field at the spacecraft over a given timescale. Some of these agreements are achieved by averaging out toward and away excess flux, but toward and away structure that maps back to the source surface, and hence is genuine OSF, is also averaged out. It was found that an averaging timescale of approximately 1 day made spacecraft and PFSS estimates agree rather well, but there was no physical reason to choose this averaging timescale, and so, it was an arbitrary correction, and we could never be sure it always applied. In addition, it must be remembered that the PFSS method contains many assumptions.
Other methods, such as using orientation with respect to the Parker spiral theory (Erdős and Balogh, 2012, 2014), can be used to remove the orthogardenhose component of the excess flux but relied on the use of an averaging timescale over an arbitrary timescale to remove the folded flux component and so were not any more satisfactory. Lockwood et al. (2009a) devised a method to use the gradients in solar wind speed with frozen-in flux to estimate the excess flux (the kinematic correction) and showed that it could broadly explain the difference between PFSS and spacecraft values more physically. However, by far, the best method is that devised by Owens et al. (2017b) using strahl electrons to identify folded flux (and orthogardenhose flux) and compute the excess flux. Strahl electrons are generated by the high temperatures of the solar corona. They flow along heliospheric field lines, and because the field-aligned velocity is much higher than field-perpendicular velocities of the field lines, the field lines move only a small distance during the short electron transit times, and the electron trajectories are close to (but not quite exactly) field-aligned. Strahl electrons are sometimes seen moving back toward the Sun, and this is a unique identifier of excess flux (either orthogardenhose or folded flux). Strahl on orthogardenhose HMF is sunward, as is strahl on inverted gardenhose HMF that has been through a switchback and is folded back on itself. Owens et al. (2017b) used strahl data from the Advanced Composition Explorer (ACE) spacecraft to compute the total flux of field lines showing sunward strahl and properly measure the excess flux Δ for the first time. The results showed that the kinematic correction was valuable but slightly and systematically overestimated the excess flux. Frost et al. (2022) have recently extended the survey of Owens et al. (2017b) by using both the ACE and WIND spacecraft, and we here use linear regression their values of the OSF derived using Equation 8 to scale OSF values obtained from the geomagnetic reconstructions of B and VSW using Equation 6. This yields
where s and c are the coefficients from the linear regression of the estimates
It should be noted that the correction for excess flux from strahl electrons by Frost et al. (2022) provides a slightly larger OSF than the kinematic correction, which was used in past reconstructions by Lockwood et al. (2014b). On the other hand, Figure 11 shows that proper allowance for Parker spiral means we need to reduce OSF by a factor of (1 + μϵ) ≈ 1.2. These two opposing effects happen to be of similar magnitude, and so, the OSF values derived here are quite similar to those derived by Lockwood et al. (2014b).
The signed OSF derived this way is presented in Figure 12 and compared with the values of Frost et al. (2022) indicated by the blue dots after 1995 in Part A. Before 1995, no suitable strahl data are available, and the blue dots for 1964–1994 are the kinematically corrected values of Lockwood et al. (2009a) that have been adjusted using the best-fit linear regression of the excess flux estimates with the Frost et al. (2022) values for 1995–2020. This composite of satellite OSF values, corrected for excess flux,
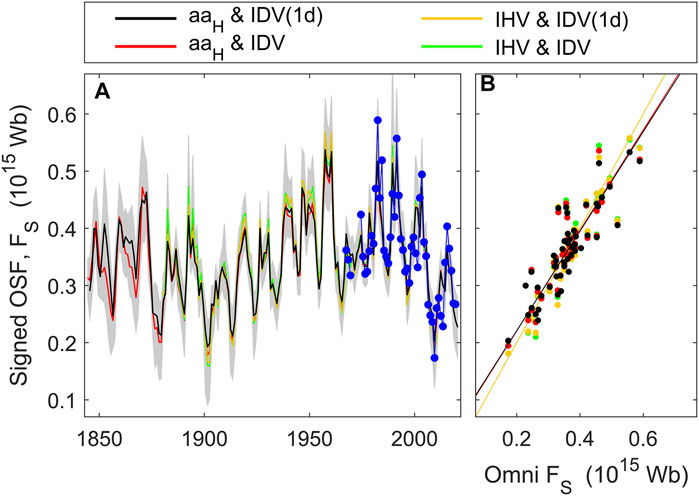
FIGURE 12. The same as Figure 8 for the signed open solar flux (OSF) FS. The blue dots in part (A) and x-axis in part (B) are the values from satellite observations that have been corrected using the excess flux. For 1995–2020, excess flux has been derived using strahl electrons by Frost et al. (2022), but for 1966–1994, no suitable strahl data are available, and the values are kinematically corrected using the method of Lockwood et al. (2009a), with values adjusted using the linear regression with the strahl-corrected values for 1995–2020.
Here, we have used the aaH rather than the corrected version of aa employed by Lockwood et al. (2014b), which has introduced some differences. The main effect of this change has been the reduction of discrepancies between the reconstructions for different geomagnetic index pairings as well as the reduction of the overall 2σ uncertainties. However, the use of aaH has made only minimal difference to the variation of the optimum OSF reconstruction. What has had a much greater effect, and is the main reason why the percentage rise in OSF was somewhat lower than those in the results of Lockwood et al. (2014b), is the evolving improvement to the excess flux calculation. This has resulted in the best estimate of the percentage rise in OSF over the first half of the 20th century changing from 100% in the study by Lockwood et al. (2014b) to the 67% found here. The use of strahl electrons is by far the best method devised (Owens et al., 2017b; Frost et al., 2022), and it generates slightly lower excess flux values than the kinematic correction employed by Lockwood et al. (2014b). This raises all OSF estimates, and a small rise in OSF estimates for early in the 20th century explains the change in the percent rise estimate.
7 Long-term variations in the modern grand maximum
Figures 1 and 2 use running boxcar averages over 11 years to look at the long-term variations. However, because the solar cycles are not exactly 11 years in duration, this gives a residual of approximately 11-year period in the smoothed variations. In addition, the variation in solar cycle durations means that removing them completely using a low-pass filter causes changes to the long-term variation. Lockwood and Frölich (2007) devised a method to give the long-term variations without this ripple nor the need to assume the solar cycle duration. The boxcar running means are taken over a range of interval durations τ between 8 and 14 years. Twice in each solar cycle, these means form “nodes,” where their value is almost the same for all τ. We take the average of the running means at these nodes and interpolate between them using the piecewise cubic hermite interpolating polynomial (PCHIP) interpolation. Figure 13B shows the result for the (revised) international sunspot number, RISN. The nodes are shown as yellow points, and the interpolated variation is black. Note that the green line (the boxcar smoothed variation for τ = 11 years) is always very close to the black line, but its residual 11-year ripple has been ironed out. The plot starts shortly after the emergence from the Maunder minimum. Compared with the relatively quiet latest values
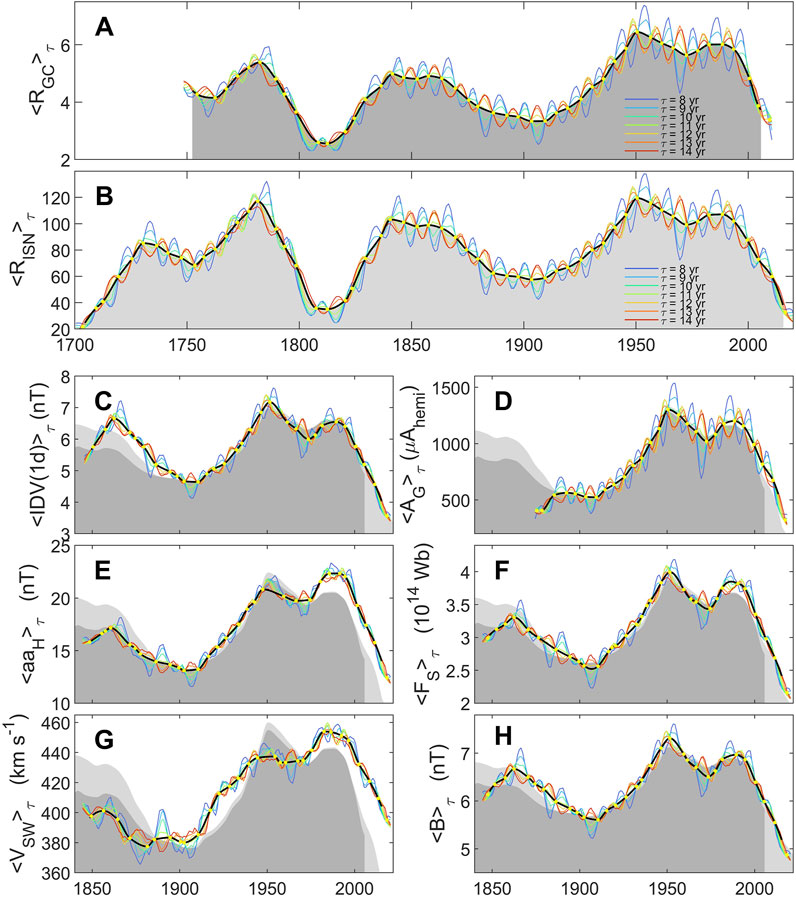
FIGURE 13. Smoothed long-term data series showing the MGSM. These are generated by taking boxcar running means
The ISN data are annual mean values. These are available from a full set of daily values for 1818 onward. Before 1818, there are gaps in the daily series, but monthly means are still generated from 1749 onward and annual means from 1700. Hence, the sequence shown is not homogeneous in the data available. In addition, sunspot observers, their apparatus, and their location differ, and indeed, observers’ acuity may vary over time, for example, improving with new instrumentation or decaying with failing eyesight. For later years in the sequence, the mean of observation distribution can be taken, but going back in time, the sequence often relies on single observers, the results from whom need calibration. However, at times, there is little overlap to allow this calibration (Clette and Lefèvre, 2016; Muñoz-Jaramillo and Vaquero, 2019). These calibrations are daisy-chained, and errors in the regression between successive observers (see Lockwood et al., 2016a) accumulate as one goes back in time. A method to calibrate early data against one common standard was devised by Usoskin et al. (2016) using “active day fractions” (ADFs, the fraction of days on which a given observer detects sunspots), which works well at moderate sunspot numbers but has limitations at both low and high levels (Willamo et al., 2018). Chatzistergos et al. (2017) used both daisy-chaining and ADFs to generate a long sunspot number data series, RGC; they also used group sunspot numbers, which, unlike RISN, means that the more unreliable observations of individual spots are avoided. Figure 13A shows the variation of ⟨RGC⟩, and it has similarities—but also some noticeable differences—to RISN. In particular, ⟨RGC⟩ is increasingly lower than ⟨RISN⟩ before about 1900, which means that for RGC, the MGSM is larger than the previous maxima in amplitude as well as in integrated solar activity level.
Parts C to H of Figure 13 show the smoothed variation of other indices and reconstructed parameters in the same format as Parts A and B. However, these do no extend back far enough to compare with the peak before the Dalton minima, nor quite to the peak of ⟨RISN⟩ in 1840, but they do show interesting similarities and differences in behavior to both ⟨RISN⟩ and ⟨RGC⟩ (shown linearly regressed to the parameter in question in each panel by the light- and dark-gray areas, respectively).
Figure 13C presents the IDV(1d) index. The plot for IDV is essentially identical (not shown), because the agreement of IDV and IDV(1d) is so close (see the bottom panels of Figure 4). The agreement between the long-term variations in ⟨IDV(1d)⟩ (and hence also of ⟨IDV⟩) and the best linear regressions of ⟨RISN⟩ and ⟨RGC⟩ is extremely close but is less good for the earlier data. This could potentially indicate that these two geomagnetic sequences are in error at this time or the sunspot data are in error, or both. However, we urge caution before drawing the conclusion that there is an error in one or both of these data sequences. Close inspection shows that the fall in group sunspot numbers after 1985 has been slightly greater than that in sunspot numbers, and hence, it may be a false assumption that the two should agree that closely. Nevertheless, the divergence in the earliest data is considerable. Figure 13C illustrates that the first peak of the 20th-century grand maximum is the larger for IDV(1d) (and so for IDV also) as it is for the sunspot numbers. The earliest IDV(1d) data are more similar to RISN than RGC.
Figure 13D presents the corresponding plot for the sunspot group area ⟨AG⟩ composite of data from the Royal Greenwich Observatory (RGO), solar optical observing network, and Kislovodsk, Pulkovo, Debrecen, Kodaikanal, Rome, Catania, and Yunnan observatories, which covers the period between 1874 and 2019 and was compiled and being intercalibrated by Mandal et al. (2020). The group area ⟨AG⟩ shows the 20th-century grand maximum and is very similar in amplitude and form to that in the sunspot numbers. The agreement is very good except for the very earliest AG data, which largely come from RGO.
Figure 13E presents the variation for the aaH index, which shows great agreement with the aaHN and aaHS subindices, with the Potsdam/Seddin/Niemegk k-index composite and the IHV index (see top panels of Figure 4), for which the corresponding plot is therefore almost identical (not shown). However, the aaH data, even with the Helsinki/St. Petersburg extension, do not quite reach back to the peak in sunspot numbers in 1840, but the 1848 values do imply that this peak is not as pronounced for aaH as for RISN and is more like RGC. Hence, where IDV(1d) is more like RISN at this time, aaH is more like RGC, and so using the geomagnetic data to try to discriminate between the two sunspot records gives ambiguous results. However, the most interesting feature is that the second of the 20th-century grand maximum peaks in aaH is larger than the first, opposite to the sunspot data. Looking at the reconstructed values, the reason is clear. The IMF strength ⟨B⟩ (Figure 13H) and the OSF ⟨FS⟩ (Figure 13F) have long-term variations that are very similar to those for the susnpot numbers. In contrast, the solar wind velocity variation ⟨VSW⟩ (Figure 13G) is significantly different from those for the sunspot numbers with a broader maximum and dominant peak in 1985. So the dependence of aaH on
8 Discussion and conclusion
There are several important points to note about these reconstructions. First, the fact that four independent geomagnetic index pairings generate very similar reconstructions of near-Earth IMF strength B, solar wind velocity VSW, and OSF FS (demonstrated by Figures 8, 9, 12, respectively) is highly significant. The fact that none of these parameters depends in any way on how and where the geomagnetic data are measured shows that the indices are generally homogeneous in their calibration and accuracy. Note that the indices can differ from each other, but after 1880, at least, the very close similarities of the reconstructions eliminate the possibility that any such differences are an error; in other words, where the geomagnetic indices differ, they should differ, and correcting them to agree with each other would be a retrograde and erroneous step. It is worth noting that to reach this position, we have had to allow for the effect of the geomagnetic latitudes of the stations and how those latitudes have changed because of secular change in the geomagnetic field. This has been done in full for the aaH and IDV(1d) indices. The IDV index does make allowance for the geomagnetic latitudes of stations but not for the secular change in those geomagnetic latitudes; however, unlike aaH and IDV(1d) (which use philosophy B in their construction, i.e., daisy chaining of data series that have been corrected for the effects of secular change in the intrinsic field), IDV uses a basket of stations (philosophy A) that make it global in nature. This works when there are sufficient stations in a uniform network across the globe so that secular changes at one station are counterbalanced by changes at others. We note that this becomes increasingly less valid as we go back in time, and the number of available magnetometer stations decreases. Nevertheless, IDV and IDV(1d) agree to a remarkable extent back to approximately 1880. Before then, we have more faith in IDV(1d) because the lack of stations is exposing the weakness of philosophy A when station numbers are low. For the IHV index, Svalgaard and Cliver (2007) showed that the values did not depend on latitude, as long as the stations were well away from the auroral oval (below 55° geomagnetic latitude). Hence, no correction for latitude was needed because stations that were well removed from the auroral oval were chosen at all times.
The agreement between the four reconstructions for B, VSW, and FS from the different index pairings shown here means that we can move on from discussions about the accuracy of any of these geomagnetic index data series and exploit the differences between them to infer the behavior and different aspects of the Sun and near-Earth space. The only caveat to this relates to the earliest geomagnetic data. We find very good agreement between the reconstructions after 1880, which leaves a 48-year interval between the advent of the first well-calibrated magnetometer (the work of of Gauss in 1832) and the date when all the indices become unambiguously fit for purpose (i.e., when all the reconstructions based on them agree). For the earliest data, philosophy A (using a basket of stations) is evidently inadequate, and indices using philosophy B (using a well-calibrated and stable station with model allowance for its changing geomagnetic latitude) should be deployed.
Figure 14 presents a comparison of the reconstruction of annual means in OSF presented here with some other recent reconstructions. In Parts A, C, and D, the green and yellow dashed lines indicate the previous reconstruction from geomagnetic data by Lockwood et al. (2014b) (LEA14), the mauve and cyan lines indicate the modeled reconstructions from sunspot numbers by Krivova et al. (2021) (KEA21) and Lockwood and Owens (2014) (L&O14), respectively. The pale-mauve area indicates the reconstruction from the 14C cosmogenic isotope by Usoskin et al. (2021) (UEA21) (which extends up to 1899). Figure 14B presents histograms of the annual values from these reconstructions and emphasizes that the MGSM has given unusually high OSF values. The reconstruction of UEA21, based on 14C data from 1971 to 1899 gives a mode value considerably lower than that observed for the more recent data. Comparison with the reconstructions based on sunspot number (for after 1616) suggests that there were prior grand minima to 1616 that were deeper than the Maunder minimum. The distribution of reconstructed values presented here (for after 1844) has a higher mode value than for the longer data series derived from 14C and sunspot numbers, and for the spacecraft observation period, it is higher still. The distributions for the spacecraft observations, most of which were during the MGSM, contain the highest OSF values in the last 1000 years, and hence, the space age and the MGSM have been an interval of unusually high OSF.
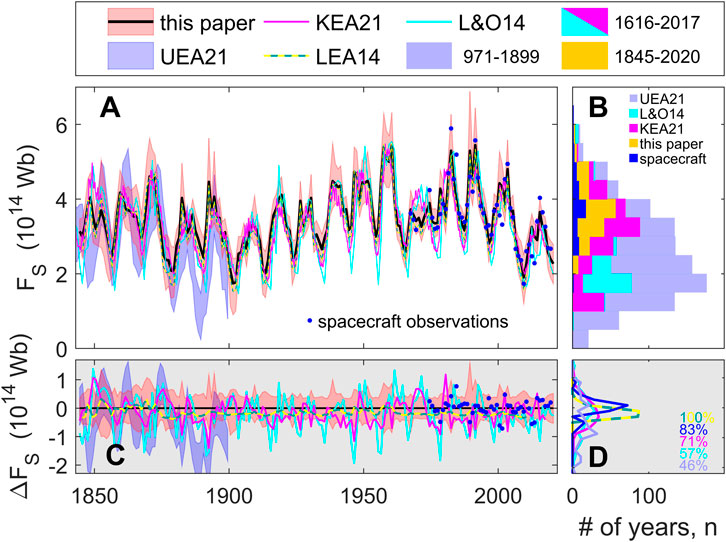
FIGURE 14. Comparison of long-long–term reconstructions of signed OSF, FS. (A) time series. The black line indicates the reconstruction presented in this article, with its ±2σ uncertainty band shaded in pink. The green and yellow dashed lines indicate the previous reconstruction from geomagnetic data by Lockwood et al. (2014b) (LEA14), and the mauve and cyan lines are the modeled reconstructions from sunspot numbers by Krivova et al. (2021) (KEA21) and Lockwood and Owens (2014) (L&O14), respectively. The pale-mauve area is the reconstruction from the 14C cosmogenic isotope by Usoskin et al. (2021) (UEA21) (extends up to 1899). The annual means of the observations in the near-Earth heliosphere are indicated by blue dots. Part (C) shows deviations from the ensemble median series presented in this article, ΔFS, presented using the same color scheme. Part (D) gives the probability distributions of the ΔFS values, the numbers being the percentage of values that are within the ±2σ uncertainty band around zero. Part (B) shows histograms of the complete FS datasets: the pale-mauve histogram is the UEA21 dataset (covers 971–1899); the mauve histogram is the KEA21 series (1616–2017); the cyan histogram the L&O14 series (1616–2017); the orange histogram is the dataset presented in this article (1844–2020); and the blue histogram is the spacecraft observations (1964–2019).
The corrections to the open flux estimates made here (using a better estimate of the excess flux from strahl electron observations and the better allowance for the effects of the Parker spiral gardenhose angle) have important effects. The green and yellow dashed lines in Parts A and C of Figure 14 indicate that the optimum OSF reconstruction presented here has slightly increased OSF values compared with the previous estimates by Lockwood et al. (2014b). There has also been a very slight decrease in the rise since 1900 introduced by using the aaH index, with its allowance for the secular drift in the geomagnetic latitude of the stations. The LEA14 values always lie within the 2σ uncertainties of the present values. Figure 14A shows that all reconstructions agree closely on the solar cycle and long-term variations of OSF. The percentages of simultaneous values that lie within the estimated uncertainties of the reconstructions presented in this study are given in Part D; they are 83% for the spacecraft observations, 71% for the KEA17 reconstruction from sunspot numbers, 57% for the L&O14 reconstructions from sunspot numbers, and 46% for the UEA21 reconstruction from 14C (which ends before the start of the MGSM).
Implementing the improvements detailed in this study, we find the rise in solar activity levels in the 20th-century results in solar cycle means of the OSF FS increasing from 2.46 × 1014Wb in 1906 to 4.10 × 1014Wb in 1949, at the peak of the MGSM. Hence, we find that the rise in open flux was by a factor of 67%. It is interesting to note that the peak OSF of the grand maximum derived here is only slightly lower than those estimated by (Lockwood et al., 1999) and by (Lockwood et al., 2014b). However, the value for cycle 14 (around 1906) found here is larger than those estimated in the aforementioned studies, and this lowers the estimated percentage rise in OSF during the MGSM. This is still considerably larger than the 25% rise estimated by Svalgaard and Cliver (2005) and Svalgaard and Cliver (2010), who actually discussed the near-Earth IMF B rather than the OSF FS but did not acknowledge the differences between these two parameters (Lockwood and Owens, 2011). We here find that the solar cycle means in the IMF B rose from 5.21 in 1901 to 7.63 nT in 1955, indicating a rise of 46%. Lockwood et al. (2006) showed that the low estimate of Svalgaard and Cliver (2005) for the rise in B was because of the adoption of unsafe linear regression procedures.
The variations shown here are otherwise very similar to those derived by Lockwood et al. (2014b), and the variations in the IMF B and solar wind speed VSW are very similar indeed. Hence, the addition of the solar cycle 24 data, where available, has strengthened the correlations used but not changed these results to any great extent. The slight reduction in the amplitude of the MGSM on OSF was caused by the more rigorous computation of the excess flux using strahl observations.
The variations confirm the similarity of the long-term variations of the IMF and OSF and the sunspot numbers that have been noted before and so give further confidence that OSF modeling based on sunspot numbers (Solanki et al., 2000, 2002; Mackay and Lockwood, 2002; Vieira and Solanki, 2010; Owens and Lockwood, 2012; Lockwood and Owens, 2014; Owens et al., 2017a; Krivova et al., 2021) are not missing a key external factor, although some (small) adjustments of coefficients may be needed to account for the adjusted OSF reconstruction presented here.
Mursula et al. (2017) suggested that the occurrence of low-latitude coronal hole, and hence fast solar wind at Earth and the consequent rise in average solar wind speed, is enhanced during the declining phase of a grand maximum. Figure 14E supports this as average flow speeds are indeed found to be enhanced (relative to the average sunspot numbers) as the open flux declines after the grand maximum. However, the same is true in the rising phase, albeit to a smaller extent. A more detailed study of the enhanced solar wind flow speed will be presented in Paper II (Lockwood et al., 2022).
The reconstructions of the OSF from geomagnetic activity provide a vital dataset for understanding the long-term evolution of the solar atmosphere and the solar magnetic cycle. In particular, like in situ measurements by satellites in near-Earth orbit or in halo orbits around the L1 Lagrange point, the geomagnetic data are measurements of the ecliptic plane and so at low heliographic latitudes (between approximately −7° and +7°) and usually in the streamer belt. However, the results from the Ulysses mission show that they have implications for the solar atmosphere and inner heliosphere at all solar latitudes. This generalization of localized measurements to a global solar value, such as the OSF, may only be valid to first order, and it is probable that the results from Solar Orbiter and Solar Probe will give refinements that can be implemented. In addition, properly joined-up numerical modeling of the solar atmosphere and inner heliosphere offers great promise of improvements.
That being said, tests have shown that the Ulysses result is certainly valid for computing the OSF to first order (Lockwood et al., 2004; Owens et al., 2008; Lockwood and Owens, 2009), and the reconstructions based on geomagnetic data have already been vital. They extend back long enough to allow us to calibrate the very long data series of cosmogenic abundance data in terms of OSF (Usoskin et al., 2021). However, they also raise an interesting dichotomy. The source surface OSF estimates derived from remote photospheric magnetic field observations and numerical modeling are typically a factor of two lower than estimates derived from the in situ satellite and geomagnetic data (Linker et al., 2017; Wallace et al., 2019). Observations of coronal holes also lead to values that are smaller by a similar factor (Lowder et al., 2014, 2017). OSF has been determined using a number of modeling and observational methods. These range from simple PFSS models (Altschuler and Newkirk, 1969; Schatten et al., 1969; Wang and Sheeley, 1992) to complex magnetohydrodynamic (MHD) coronal models (e.g., Lionello et al., 2009). These methods are based on global magnetograms, maps assembled from full-disk observations of the line-of-sight photospheric field. The methods use a number of (different) assumptions and pre-process the input photospheric data in different ways. This can be crucial, for example, Wang and Sheeley (1995) made PFSS and in situ OSF values consistent by adopting a latitude-dependent saturation level for the magnetograph data. However, this is just one of a large number of potential causes of the discrepancy (Stevens et al., 2012). Recent observations by Parker Solar Probe indicate that the discrepancy is not because of heliocentric distance in the heliosphere: modeled and remotely sensed OSF values are also too small than those derived from in situ observations closer to the Sun (at heliocentric distances down to 0.13AU) (Badman et al., 2021).
In Paper II (Lockwood et al., 2022), we look at how these results from geomagnetic observations can be combined with long data series of solar observations to gain a deeper understanding of the long-term change in the solar corona and heliosphere during the rise and fall of the MGSM. The reconstructed data series presented here and the spacecraft interplanetary data are available in the Supplementary Material attached to this study.
Author contributions
ML carried out the analysis and wrote the article with contributions by all co-authors. MO and LB helped with the software development, and CS and BY generated the ionospheric database used. AF carried out the strahl electron excess flux analysis. All co-authors provided parts of the text and proofreading of the manuscript.
Funding
This work is supported by a number of grants. ML, MO, CS, and LB are supported by consolidated grant number ST/M000885/1 from the UK Science and Technology Facilities Council (STFC), and ML and MO are also funded by the SWIGS Directed Highlight Topic Grant number NE/P016928/1/from the UK Natural Environment Research Council (NERC). AF is funded by an STFC PhD fellowship. The work of YC was carried out while visiting University of Reading and was funded by grants from CAS (Key Research Program of Frontier Sciences QYZDB − SSW − DQC015), NSFC (41822405, 41774181, 41904151, 42004143), the Strategic Priority Program of CAS (XDB41000000), Anhui Provincial Natural Science Foundation (908085MD107), project funded by China Postdoctoral Science Foundation (2019M652194), and the Fundamental Research Funds for the Central Universities (WK2080000122). The work of BY was carried out while visiting University of Reading, supported by the Royal Society with a Newton International Fellowship (grant No. NIF − R1 − 180815) and by the B-type Strategic Priority Program of CAS (grant No. XDB41000000), the National Natural Science Foundation of China (grants 41774158, 41974174, 41831071), the Project of Stable Support for Youth Team in Basic Research Field, CAS (grant No. YSBR − 018), the CNSA pre-research Project on Civil Aerospace Technologies (grant No. D020105), Anhui Provincial Natural Science Foundation (grant No. 1908085QD155), and the Fundamental Research Fund for the Central Universities.
Acknowledgments
The authors are grateful to the funding bodies listed in the funding section and also to the staff of the data centers that store, maintain, and give access to the data: the Space Physics Data Facility, NASA/Goddard Space Flight Center; L’École et Observatoire des Sciences de la Terre (EOST), a joint of the University of Strasbourg and the French National Center for Scientific Research (CNRS) and the International Service of Geomagnetic Indices (ISGI); the UK Solar System Data Centre (UKSSDC); the Sunspot Index and Long-term Solar Observations (SILSO) World Data Center. We are also grateful to Richard Whitehead for making the two-dimensional MATLAB polynomial fitting routines available.
Conflict of interest
The authors declare that the research was conducted in the absence of any commercial or financial relationships that could be construed as a potential conflict of interest.
Publisher’s note
All claims expressed in this article are solely those of the authors and do not necessarily represent those of their affiliated organizations, or those of the publisher, the editors, and the reviewers. Any product that may be evaluated in this article, or claim that may be made by its manufacturer, is not guaranteed or endorsed by the publisher.
Supplementary material
The supplementary material for this article can be found online at: https://www.frontiersin.org/articles/10.3389/fspas.2022.960775/full#supplementary-material
References
Akaike, H. (1974). A new look at the statistical model identification. IEEE Trans. Autom. Contr. 19, 716–723. doi:10.1109/TAC.1974.1100705
Altschuler, M. D., and Newkirk, G. (1969). Magnetic fields and the structure of the solar corona: I: Methods of calculating coronal fields. Sol. Phys. 9, 131–149. doi:10.1007/BF00145734
Badman, S. T., Bale, S. D., Rouillard, A. P., Bowen, T. A., Bonnell, J. W., Goetz, K., et al. (2021). Measurement of the open magnetic flux in the inner heliosphere down to 0.13 AU. Astron. Astrophys. 650, A18. doi:10.1051/0004-6361/202039407
Balogh, A., Southwood, D. J., Forsyth, R. J., Horbury, T. S., Smith, E. J., and Tsurutani, B. T. (1995). The heliospheric magnetic field over the south polar region of the sun. Science 268, 1007–1010. doi:10.1126/science.268.5213.1007
Banerjee, D., Jian, J., Kusano, K., and Solanki, S. K. (2018). “Long-term datasets for the understanding of solar and stellar magnetic cycles,”. No. 340 in IAU symposium proceedings series in Proceedings of the 340th Symposium of the International Astronomical Union, Jaipur, India, February 19-23, 2018. (Cambridge, United Kingdom; New York, NY: Cambridge University Press). OCLC: on1052842365.
Barnard, L., Lockwood, M., Hapgood, M., Owens, M., Davis, C., and Steinhilber, F. (2011). Predicting space climate change. Geophys. Res. Lett. 38, L16103. doi:10.1029/2011GL048489
Bartels, J., Heck, N., and Johnston, H. (1939). The three-hour-range index measuring geomagnetic activity. J. Geophys. Res. 44, 411–454. doi:10.1029/TE044i004p00411
Bartels, J. (1932). Terrestrial magnetic activity and its relations to solar phenomena. J. Geophys. Res. 7, 1–52. doi:10.1029/TE037i001p00001
Bartels, J. (1957). The geomagnetic measures for the time-variations of solar corpuscular radiation, described for use in correlation studies in other geophysical fields. Ann. Intern. Geophys. Year 4, 227–236.
Chatzistergos, T., Usoskin, I., Kovaltsov, G., Krivova, N., and Solanki, S. (2017). New reconstruction of the sunspot group numbers since 1739 using direct calibration and “backbone” methods. Astron. Astrophys. 602, A69. doi:10.1051/0004-6361/201630045
Clette, F., and Lefèvre, L. (2016). The new sunspot number: Assembling all corrections. Sol. Phys. 291, 2629–2651. doi:10.1007/s11207-016-1014-y
Crooker, N., and Gringauz, K. (1993). On the low correlation between long-term averages of solar wind speed and geomagnetic activity after 1976. J. Geophys. Res. 98, 59–62. doi:10.1029/92JA01978
Erdős, G., and Balogh, A. (2014). Magnetic flux density in the heliosphere through several solar cycles. Astrophys. J. 781, 50. doi:10.1088/0004-637X/781/1/50
Erdős, G., and Balogh, A. (2012). Magnetic flux density measured in fast and slow solar wind streams. Astrophys. J. 753, 130. doi:10.1088/0004-637X/753/2/130
Feynman, J., and Crooker, N. (1978). The solar wind at the turn of the century. Nature 275, 626–627. doi:10.1038/275626a0
Finch, I., Lockwood, M., and Rouillard, A. (2008). Effects of solar wind magnetosphere coupling recorded at different geomagnetic latitudes: Separation of directly-driven and storage/release systems. Geophys. Res. Lett. 35, L21105. doi:10.1029/2008GL035399
Frost, A. M., Macneil, A. R., Owens, M. J., and Lockwood, M. (2022). Estimating the open solar flux from in situ measurements. Sol. Phys. 297, 82. doi:10.1007/s11207-022-02004-6
Gringauz, K. (1981). Average characteristics of the solar wind and its variation during the solar cycle. Sol. Wind 4, 84–95. Place: Lindau Publisher: MPI für Aeronomie, Lindau, Germany
Holappa, L., and Mursula, K. (2015). Toward more reliable long-term indices of geomagnetic activity: Correcting a new inhomogeneity problem in early geomagnetic data. J. Geophys. Res. Space Phys. 120, 8288–8297. doi:10.1002/2015JA021752
King, J., and Papitashvili, N. (2005). Solar wind spatial scales in and comparisons of hourly Wind and ACE plasma and magnetic field data. J. Geophys. Res. 110, A02104. doi:10.1029/2004JA010649
Krivova, N., Solanki, S. K., Hofer, B., Wu, C.-J., Usoskin, I. G., and Cameron, R. (2021). Modelling the evolution of the Sun’s open and total magnetic flux. Astron. Astrophys. 650, A70. doi:10.1051/0004-6361/202140504
Linker, J., Caplan, R., Downs, C., Riley, P., Mikic, Z., Lionello, R., et al. (2017). The open flux problem. Astrophys. J. 848, 70. doi:10.3847/1538-4357/aa8a70
Lionello, R., Linker, J. A., and Mikić, Z. (2009). Multispectral emission of the sun during the first whole sun month : Magnetohydrodynamic simulations. Astrophys. J. 690, 902–912. doi:10.1088/0004-637X/690/1/902
Lockwood, M., Barnard, L. A., Nevanlinna, H., Owens, M. J., Harrison, R. G., Rouillard, A. P., et al. (2013a). Reconstruction of geomagnetic activity and near-earth interplanetary conditions over the past 167 yr - Part 1: A new geomagnetic data composite. Ann. Geophys. 31, 1957–1977. doi:10.5194/angeo-31-1957-2013
Lockwood, M., Barnard, L. A., Nevanlinna, H., Owens, M. J., Harrison, R. G., Rouillard, A. P., et al. (2013b). Reconstruction of geomagnetic activity and near-earth interplanetary conditions over the past 167 yr - Part 2: A new reconstruction of the interplanetary magnetic field. Ann. Geophys. 31, 1979–1992. doi:10.5194/angeo-31-1979-2013
Lockwood, M., Chambodut, A., Barnard, L. A., Owens, M. J., Clarke, E., and Mendel, V. (2018a). A homogeneous aa index: 1. Secular variation. J. Space Weather Space Clim. 8, A53. doi:10.1051/swsc/2018038
Lockwood, M., Chambodut, A., Finch, I. D., Barnard, L. A., Owens, M. J., and Haines, C. (2019a). Time-of-day/time-of-year response functions of planetary geomagnetic indices. J. Space Weather Space Clim. 9, A20. doi:10.1051/swsc/2019017
Lockwood, M. (2001). Long-term variations in the magnetic fields of the sun and the heliosphere: Their origin, effects and implications. J. Geophys. Res. 106, 16021–16038.
Lockwood, M., Finch, I. D., Chambodut, A., Barnard, L. A., Owens, M. J., and Clarke, E. (2018b). A homogeneous aa index: 2. Hemispheric asymmetries and the equinoctial variation. J. Space Weather Space Clim. 8, A58. doi:10.1051/swsc/2018044
Lockwood, M., Forsyth, R. B., Balogh, A., and McComas, D. J. (2004). Open solar flux estimates from near-earth measurements of the interplanetary magnetic field: Comparison of the first two perihelion passes of the ulysses spacecraft. Ann. Geophys. 22, 1395–1405. doi:10.5194/angeo-22-1395-2004
Lockwood, M., and Frölich, C. (2007). Recent oppositely directed trends in solar climate forcings and the global mean surface air temperature. Proc. R. Soc. A 463, 2447–2460. doi:10.1098/rspa.2007.1880
Lockwood, M., and McWilliams, K. (2021). On optimum solar wind-magnetosphere coupling functions for transpolar voltage and planetary geomagnetic activity. JGR. Space Phys. 126. doi:10.1029/2021JA029946
Lockwood, M., Nevanlinna, H., Barnard, L., Owens, M. J., Harrison, R. G., Rouillard, A. P., et al. (2014b). Reconstruction of geomagnetic activity and near-Earth interplanetary conditions over the past 167 yr – Part 4: Near-Earth solar wind speed, IMF, and open solar flux. Ann. Geophys. 32, 383–399. doi:10.5194/angeo-32-383-2014
Lockwood, M., Nevanlinna, H., Vokhmyanin, M., Ponyavin, D., Sokolov, S., Barnard, L. A., et al. (2014a). Reconstruction of geomagnetic activity and near-Earth interplanetary conditions over the past 167 yr – Part 3: Improved representation of solar cycle 11. Ann. Geophys. 32, 367–381. doi:10.5194/angeo-32-367-2014
Lockwood, M., Owens, M., Barnard, L., and Usoskin, I. (2016a). Tests of sunspot number sequences: 3. Effects of regression procedures on the calibration of historic sunspot data. Sol. Phys. 291, 2829–2841. doi:10.1007/s11207-015-0829-2
Lockwood, M., and Owens, M. (2014). Centennial variations in sunspot number, open solar flux and streamer belt width: 3. Modeling. J. Geophys. Res. Space Phys. 119, 5193–5209. doi:10.1002/2014JA019973
Lockwood, M., and Owens, M. (2013). Comment on ”What causes the flux excess in the heliospheric magnetic field?” by E.J. Smith. J. Geophys. Res. Space Phys. 118, 1880–1887. doi:10.1002/jgra50223
Lockwood, M., Owens, M. J., Barnard, L. A., Scott, C. J., and Watt, C. E. (2017). Space climate and space weather over the past 400 years: 1. The power input to the magnetosphere. J. Space Weather Space Clim. 7, A25. doi:10.1051/swsc/2017019
Lockwood, M., and Owens, M. J. (2011). Centennial changes in the heliospheric magnetic field and open solar flux: The consensus view from geomagnetic data and cosmogenic isotopes and its implications: Centennial Changes in IMF and Open Flux. J. Geophys. Res. 116, A04109. doi:10.1029/2010JA016220
Lockwood, M., Owens, M. J., and Macneil, A. (2019). On the origin of ortho-gardenhose heliospheric flux. Sol. Phys. 294, 85. doi:10.1007/s11207-019-1478-7
Lockwood, M., Owens, M. J., and Rouillard, A. P. (2009a). Excess open solar magnetic flux from satellite data: 2. A survey of kinematic effects. J. Geophys. Res. 114, A11104. doi:10.1029/2009JA014450
Lockwood, M., and Owens, M. (2009). The accuracy of using the Ulysses result of the spatial invariance of the radial heliospheric field to compute the open solar flux. Astrophys. J. 701, 964–973. doi:10.1088/0004-637X/701/2/964
Lockwood, M., Owens, M. J., Yardley, S. L., Virtenan, I. O. I., Yeates, A. R., and Muñoz-Jaramillo, A. (2022). Application of historic datasets to understanding open solar flux and the 20th-century grand solar maximum. 2. Solar observations. Front. Phys. 10, 976444. doi:10.3389/fphy.2022.976444
Lockwood, M., Rouillard, A. P., Finch, I., and Stamper, R. (2006). Comment on “the IDV index: Its derivation and use in inferring long-term variations of the interplanetary magnetic field strength” by Leif Svalgaard and Edward W. Cliver. J. Geophys. Res. 111, A09109. doi:10.1029/2006JA011640
Lockwood, M., Rouillard, A. P., and Finch, I. (2009b). The rise and fall of open solar flux during the current grand solar maximum. Astrophys. J. 700, 937–944. doi:10.1088/0004-637X/700/2/937
Lockwood, M., Scott, C. J., Owens, M. J., Barnard, L. A., and Willis, D. M. (2016b). Tests of sunspot number sequences: 1. Using ionosonde data. Sol. Phys. 291, 2785–2809. doi:10.1007/s11207-016-0855-8
Lockwood, M. (2022). Solar wind—magnetosphere coupling functions: Pitfalls, limitations, and applications. Space weather. 20, e2021SW002989. doi:10.1029/2021SW002989
Lockwood, M., Stamper, R., and Wild, M. N. (1999). A doubling of the Sun's coronal magnetic field during the past 100 years. Nature 399, 437–439. doi:10.1038/20867
Lockwood, M. (2003). Twenty-three cycles of changing open solar magnetic flux. J. Geophys. Res. 108, 1128. doi:10.1029/2002JA009431
Lowder, C., Qiu, J., and Leamon, R. (2017). Coronal holes and open magnetic flux over cycles 23 and 24. Sol. Phys. 292, 18. doi:10.1007/s11207-016-1041-8
Lowder, C., Qiu, J., Leamon, R., and Liu, Y. (2014). Measurements of EUV coronal holes and open magnetic flux. Astrophys. J. 783, 142. doi:10.1088/0004-637X/783/2/142
Mackay, D. H., and Lockwood, M. (2002). The evolution of the sun’s open magnetic flux: ii. full solar cycle simulations. Sol. Phys. 209, 287–309. doi:10.1023/A:1021230604497
Macmillan, S., and Clarke, E. (2011). Resolving issues concerning Eskdalemuir geomagnetic hourly values. Ann. Geophys. 29, 283–288. doi:10.5194/angeo-29-283-2011
Mandal, S., Krivova, N. A., Solanki, S. K., Sinha, N., and Banerjee, D. (2020). Sunspot area catalog revisited: Daily cross-calibrated areas since 1874. Astron. Astrophys. 640, A78. doi:10.1051/0004-6361/202037547
Martini, D., and Mursula, K. (2006). Correcting the geomagnetic IHV index of the Eskdalemuir observatory. Ann. Geophys. 24, 3411–3419. doi:10.5194/angeo-24-3411-2006
Mayaud, P.-N. (1972). The aa indices: A 100-year series characterizing the magnetic activity. J. Geophys. Res. 77, 6870–6874. doi:10.1029/ja077i034p06870
Meng, X.-l., Rosenthal, R., and Rubin, D. B. (1992). Comparing correlated correlation coefficients. Psychol. Bull. 111, 172–175. doi:10.1037/0033-2909.111.1.172
Mozer, F. S., Agapitov, O. V., Bale, S. D., Bonnell, J. W., Case, T., Chaston, C. C., et al. (2020). Switchbacks in the solar magnetic field: Their evolution, their content, and their effects on the plasma. Astrophys. J. Suppl. Ser. 246, 68. doi:10.3847/1538-4365/ab7196
Muñoz-Jaramillo, A., and Vaquero, J. (2019). Visualization of the challenges and limitations of the long-term sunspot number record. Nat. Astron. 3, 205–211. doi:10.1038/s41550-018-0638-2
Mursula, K., Holappa, L., and Lukianova, R. (2017). Seasonal solar wind speeds for the last 100 years: Unique coronal hole structures during the peak and demise of the grand modern maximum. Geophys. Res. Lett. 44, 30–36. doi:10.1002/2016GL071573
Mursula, K., Martini, D., and Karinen, A. (2004). Did open solar magnetic field increase during the last 100 Years? A reanalysis of geomagnetic activity. Sol. Phys. 224, 85–94. doi:10.1007/s11207-005-4981-y
Owens, M. J., Arge, C. N., Crooker, N. U., Schwadron, N. A., and Horbury, T. S. (2008). Estimating total heliospheric magnetic flux from single-point in situ measurements. J. Geophys. Res. 113, A12103. doi:10.1029/2008JA013677
Owens, M. J., Crooker, N. U., and Lockwood, M. (2013). Solar origin of heliospheric magnetic field inversions: Evidence for coronal loop opening within pseudostreamers. J. Geophys. Res. Space Phys. 118, 1868–1879. doi:10.1002/jgra.50259
Owens, M. J., and Lockwood, M. (2012). Cyclic loss of open solar flux since 1868: The link to heliospheric current sheet tilt and implications for the Maunder minimum. J. Geophys. Res. 117, A04102. doi:10.1029/2011JA017193
Owens, M. J., Lockwood, M., and Riley, P. (2017a). Global solar wind variations over the last four centuries. Sci. Rep. 7, 41548. doi:10.1038/srep41548
Owens, M. J., Lockwood, M., Riley, P., and Linker, J. (2017b). Sunward strahl: A method to unambiguously determine open solar flux from in situ spacecraft measurements using suprathermal electron data. J. Geophys. Res. Space Phys. 122, 10980. doi:10.1002/2017JA024631
Parker, E. N. (1958). Dynamics of the interplanetary gas and magnetic fields. Astrophys. J. 128, 664. doi:10.1086/146579
Russell, C. (1975). On the possibility of deducing interplanetary and solar parameters from geomagnetic records. Sol. Phys. 42, 259–269. doi:10.1007/BF00153301
Sargent, H. H. (1979). “A geomagnetic activity recurrence index,” in Solar-terrestrial influences on weather and climate. Editors B. McCormac, and T. Seliga (Dordrecht: Springer Netherlands), 101–104. doi:10.1007/978-94-009-9428-7_10
Schatten, K. H., Wilcox, J. M., and Ness, N. F. (1969). A model of interplanetary and coronal magnetic fields. Sol. Phys. 6, 442–455. doi:10.1007/BF00146478
Sivadas, N., and Sibeck, D. (2022). Regression bias in using solar wind measurements. Front. Astron. Space Sci. 9, 924976. doi:10.3389/fspas.2022.924976
Smith, E., and Balogh, A. (1995). Ulysses observations of the radial magnetic field. Geophys. Res. Lett. 22, 3317–3320. doi:10.1029/95GL02826
Solanki, S. K., Schüssler, M., and Fligge, M. (2000). Evolution of the Sun’s large-scale magnetic field since the Maunder minimum. Nature 408, 445–447. doi:10.1038/35044027
Solanki, S. K., Schüssler, M., and Fligge, M. (2002). Secular variation of the Sun’s magnetic flux. Astron. Astrophys. 383, 706–712. doi:10.1051/0004-6361:20011790
Stamper, R., Lockwood, M., Wild, M. N., and Clark, T. D. G. (1999). Solar causes of the long-term increase in geomagnetic activity. J. Geophys. Res. 104, 28325–28342. doi:10.1029/1999JA900311
Steinhilber, F., Abreu, J. A., Beer, J., Brunner, I., Christl, M., Fischer, H., et al. (2012). 9,400 years of cosmic radiation and solar activity from ice cores and tree rings. Proc. Natl. Acad. Sci. U. S. A. 109, 5967–5971. doi:10.1073/pnas.1118965109
Stevens, M., Linker, J., Riley, P., and Hughes, W. J. (2012). Underestimates of magnetic flux in coupled MHD model solar wind solutions. J. Atmos. Solar-Terrestrial Phys. 83, 22–31. doi:10.1016/j.jastp.2012.02.005
Suess, S. T., Phillips, J. L., McComas, D. J., Goldstein, B. E., Neugebauer, M., and Nerney, S. (1998). The solar wind inner heliosphere. Space Sci. Rev. 83, 75–86. doi:10.1023/A:1005069328058
Suess, S. T., and Smith, E. J. (1996). Latitudinal dependence of the radial IMF component: Coronal imprint. Geophys. Res. Lett. 23, 3267–3270. doi:10.1029/96GL02908
Suess, S. T., Smith, E. J., Phillips, J., Goldstein, B. E., and Nerney, S. (1996). Latitudinal dependence of the radial imf component - interplanetary imprint. Astron. Astrophys 316, 304–312.
Svalgaard, L., and Cliver, E. W. (2010). Heliospheric magnetic field 1835-2009. J. Geophys. Res. 115, A09111. doi:10.1029/2009JA015069
Svalgaard, L., and Cliver, E. W. (2007). Interhourly variability index of geomagnetic activity and its use in deriving the long-term variation of solar wind speed. J. Geophys. Res. 112, A10111. doi:10.1029/2007JA012437
Svalgaard, L., Cliver, E. W., and Le Sager, P. (2004). IHV: A new long-term geomagnetic index. Adv. Space Res. 34, 436–439. doi:10.1016/j.asr.2003.01.029
Svalgaard, L., and Cliver, E. W. (2005). The IDV index: Its derivation and use in inferring long-term variations of the interplanetary magnetic field strength. J. Geophys. Res. 110, A12103. doi:10.1029/2005JA011203
Svalgaard, L. (2014). Correction of errors in scale values for magnetic elements for Helsinki. Ann. Geophys. 32, 633–641. doi:10.5194/angeo-32-633-2014
Thiébaux, H., and Zwiers, F. (1984). The interpretation and estimation of effective sample size. J. Clim. Appl. Meteor. 23, 800–811. doi:10.1175/1520-0450(1984)023<0800:TIAEOE>2.0.CO;2
Usoskin, I., Arlt, R., Asvestari, E., Hawkins, E., Käpylä, M., Kovaltsov, G. A., et al. (2015). The Maunder minimum (1645–1715) was indeed a grand minimum: A reassessment of multiple datasets. Astron. Astrophys. 581, A95. doi:10.1051/0004-6361/201526652
Usoskin, I. G. (2017). A history of solar activity over millennia. Living Rev. Sol. Phys. 14, 3. doi:10.1007/s41116-017-0006-9
Usoskin, I., Kovaltsov, G., Lockwood, M., Mursula, K., Owens, M., and Solanki, S. (2016). A new calibrated sunspot group series since 1749: Statistics of active day fractions. Sol. Phys. 291, 2685–2708. doi:10.1007/s11207-015-0838-1
Usoskin, I., Solanki, S., Krivova, N., Hofer, B., Kovaltsov, G., Wacker, L., et al. (2021). Solar cyclic activity over the last millennium reconstructed from annual 14 C data. Astron. Astrophys. 649, A141. doi:10.1051/0004-6361/202140711
Vaquero, J. M., Svalgaard, L., Carrasco, V. M. S., Clette, F., Lefèvre, L., Gallego, M. C., et al. (2016). A revised collection of sunspot group numbers. Sol. Phys. 291, 3061–3074. doi:10.1007/s11207-016-0982-2
Vieira, E. A., and Solanki, S. K. (2010). Evolution of the solar magnetic flux on time scales of years to millenia. Astron. Astrophys. 509, A100. doi:10.1051/0004-6361/200913276
Wallace, S., Arge, C. N., Pattichis, M., Hock-Mysliwiec, R. A., and Henney, C. J. (2019). Estimating total open heliospheric magnetic flux. Sol. Phys. 294, 19. doi:10.1007/s11207-019-1402-1
Wang, Y.-M., and Sheeley, N. R. (1992). On potential field models of the solar corona. Astrophys. J. 392, 310. doi:10.1086/171430
Wang, Y.-M., and Sheeley, N. R. (1995). Solar implications of Ulysses interplanetary field measurements. Astrophys. J. 447. doi:10.1086/309578
White, H. (1980). A heteroskedasticity-consistent covariance matrix estimator and a direct test for heteroskedasticity. Econometrica 48, 817–838. doi:10.2307/1912934
Whitehead, R. (2021). 2D polynomial fitting with SVD. MATLAB Central File Exchange (Accessed July 13, 2021).
Wilks, D. S. (1995). Statistical methods in the atmospheric sciences: An introduction in International geophysics series (San Diego: Academic Press), 59.
Keywords: open solar flux, historic reconstructions, geomagnetic activity data, sunspot activity dependence, in situ heliospheric measurements, solar wind speed, near-Earth interplanetary field
Citation: Lockwood M, Owens MJ, Barnard LA, Scott CJ, Frost AM, Yu B and Chi Y (2022) Application of historic datasets to understanding open solar flux and the 20th-century grand solar maximum. 1. Geomagnetic, ionospheric, and sunspot observations. Front. Astron. Space Sci. 9:960775. doi: 10.3389/fspas.2022.960775
Received: 03 June 2022; Accepted: 13 July 2022;
Published: 01 September 2022.
Edited by:
Dipankar Banerjee, Indian Institute of Astrophysics, IndiaReviewed by:
Ilya Usoskin, University of Oulu, FinlandSudip Mandal, Max Planck Institute for Solar System Research, Germany
Copyright © 2022 Lockwood, Owens, Barnard, Scott, Frost, Yu and Chi. This is an open-access article distributed under the terms of the Creative Commons Attribution License (CC BY). The use, distribution or reproduction in other forums is permitted, provided the original author(s) and the copyright owner(s) are credited and that the original publication in this journal is cited, in accordance with accepted academic practice. No use, distribution or reproduction is permitted which does not comply with these terms.
*Correspondence: Mike Lockwood, bS5sb2Nrd29vZEByZWFkaW5nLmFjLnVr