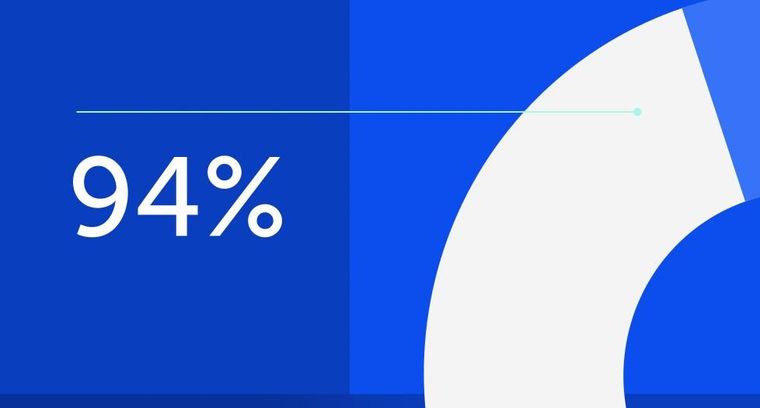
94% of researchers rate our articles as excellent or good
Learn more about the work of our research integrity team to safeguard the quality of each article we publish.
Find out more
PERSPECTIVE article
Front. Astron. Space Sci., 16 August 2022
Sec. Space Physics
Volume 9 - 2022 | https://doi.org/10.3389/fspas.2022.934216
This article is part of the Research TopicGeneration-to-Generation Communications in Space PhysicsView all 27 articles
Modeling the magnetosphere from satellite data has some analogy to the construction of a person’s ‘identikit’ from patchy information, collected by different people at different times under different lighting/viewing conditions. This article is a brief overview of the author’s half-century-long efforts in building Earth’s magnetosphere models, with some feats, setbacks, and lessons learned.
In the spring of 1968, being then a fourth-year physics student, I considered changing my major from quantum mechanics to something more earthly. One day, while hanging around our department I came across a poster for a habilitation thesis defense by a certain Mikhail Pudovkin. The title, “Morphology of Polar Storms”, stirred associations with blizzards, howling winds, icebergs, and Klondike stories by Jack London. As I learned at the event, the dissertation subject was not atmospheric, but geomagnetic storms. It was just there that I first heard the magic terms ‘magnetosphere’, ‘auroral precipitation’, ‘pitch angle’, etc. All that stuff sounded exciting, and Pudovkin himself turned out to be quite a pleasant person, which finally put an end to my vacillations. He agreed to supervise my work and suggested to explore the role of plasma isotropization in the substorm triggering. First calculations with a pancake distribution function gave a wrong polarity of the tail current; to understand the paradox I resorted to tracing particle orbits, then dug into the plasma–field equilibrium issues. However naive those early efforts seem now, they helped me get initial experience and resulted in a publication with my mentor (Pudovkin and Tsyganenko, 1973).
For my PhD work, Pudovkin suggested to calculate magnetospheric configurations using the then existing information of the distant field and magnetopause size/shape. It was just then that he uttered his fateful motto which shaped my future as a geophysicist: “Everybody is drawing the magnetosphere, but nobody knows where the field lines go”. While paying due tribute to the ground-based experiment, he was well aware of the growing role of in-situ observations. As the space age just started, lack of data had to be offset by theoretical estimates; nevertheless the situation gradually improved. By mid-1970s, our laboratory grew in size and became an internationally recognized team. Even though most of its staff could not travel beyond the Iron Curtain, we were often visited by scientists from all over the world. In the fall of 1968, Syun-Ichi Akasofu gave a talk at our seminar on conjugate aurora observations under the Northern and Southern ovals. In the following years, the lab was visited by Alex Dessler, Keith Cole, Alv Egeland, Takesi Nagata, Leif Svalgaard, and other distinguished scientists. Such informal contacts helped exchange ideas and, sometimes, get the needed data. In mid-1970s, being impressed by a paper of Mead and Fairfield (Mead and Fairfield, 1975), I sent them a request for IMP magnetometer data and, to my delight, shortly received a pair of hefty IBM card decks. The favorable reply was a pleasant surprise and, much more important, a good lesson in what was coined later in the AGU’s slogan “unselfish cooperation in science”.
As time went on, it became clear that new data called for a new mathematical framework: realistic and flexible, on the one hand, and computationally simple/fast, on the other. A host of problems emerged; one of the hurdles was to properly represent the magnetotail field. First attempts to find closed solutions failed, while the numerical approach was unacceptable as computationally expensive. Eventually a simple idea emerged: instead of deriving the field from the current, start from the outset with a simplest field from a diffuse wire. Its integration along the tail with variable intensity factors yielded the long-sought solution, embodied in the TU82 model (Tsyganenko and Usmanov, 1982) and published with my then PhD student and now lifetime friend Arcadi Usmanov. In the next T87 model (Tsyganenko, 1987), the validity region was extended through the Moon’s orbit owing to newly added distant IMP data. Providentially, one of reviewers of that paper was David Stern of GSFC, who happened to play an outstanding role in my career a few years later.
In terms of mistakes and lessons, an instructive episode is worth mentioning. In 1981, I got interested in revisiting the magnetotail equilibrium: the idea was to numerically trace proton orbits and use the obtained scattering matrix to derive steady-state pitch-angle distributions. They turned out isotropic, except for strongly non-adiabatic regimes. The latter result lingered in the back of my mind as counterintuitive, but I ventured to publish the work anyway (Tsyganenko, 1982). Three years later, a paper appeared (Wright, 1985) under almost the same title, with an elegant proof that the stationary scattering must always provide strictly isotropic distributions. Somewhat embarrassed, I went back to my tracing code and found the culprit of the paradox in using single precision instead of double. On a positive side, however, that work had far-reaching ramifications: the sharp boundary between the particle motion regimes was realized as a powerful means to probe the magnetospheric configuration and resulted in a joint paper (Sergeev and Tsyganenko, 1982) with my lifelong colleague Victor Sergeev, followed by whole series of his seminal works on the subject (e.g., (Sergeev et al., 1983), (Sergeev et al., 1993), (Sergeev et al., 2015) and refs. therein).
The next step forward was made in the end of 1980s, which also deserves a brief recounting. The TU82/T87 models shared a common shortfall: the straight tail current with rectilinear inner edge resulted in artificial “pockets” of depressed field near the dawn/dusk magnetopause. In reality, the innermost tail current gradually curves and smoothly joins the ring current. That suggested to explore axisymmetric vector potentials with azimuthal component Aϕ satisfying Poisson’s equation in cylindrical coordinates {ρ, ϕ, z}. A particular solution had already been derived for a Jovian disk model (Connerney and Ness, 1981) as a Fourier-Bessel convolution of an ad hoc current profile. However, models of that kind required lengthy numerical integrations and, hence, were out of the question. An effective workaround was eventually discovered, based on required profile of the magnetic field depression Bz (ρ, a), peaking at origin and falling off to zero at infinity with a spatial scale a. The corresponding 3D magnetic field was obtained by deriving a convolution kernel function and then integrating that kernel with the adopted Bz (ρ, a). To my delight, the integrations yielded an incredibly simple analytic vector potential Aϕ(ρ, a). Moreover, its successive derivatives ∂iAϕ/∂ai provided a whole family of independent potentials with progressively steeper radial fall-off. Their simplicity was amazing, and I still do not know any easier way to derive them, other than to scramble through the direct and inverse integral transformations. The T89 model (Tsyganenko, 1989a) based on those results is still the fastest and widely used in studies where the computation speed is critical, e.g., tracing high-energy solar protons. No wonder that the T89 citation count (now 1,370) was reported among the highest (Abt, 2000); looking back through many years, that work brought me the most satisfaction ever. In this regard, I cannot but gratefully remember another person whose advice stuck in my mind since my freshman/sophomore years. That was late Vladimir Buslaev, then our mathematical physics professor who taught us students to savor the beauty of equations, not to fear of getting lost in long calculations, not to give up too early, and to carefully check/recheck everything with no stones left unturned. Here I would like to add my own more specific advice: in all calculations, try to invent and apply various tests based on fundamental laws. In particular, an unfailing bug-hunting weapon is to make sure that the model ∇ ⋅B is zero.
Another important task was to consistently combine the internal field sources with magnetopause currents, such that the total field be confined (shielded) within the boundary. In particular, a conceptual hurdle persisted with the magnetotail, where the shielding currents also had to at least partially contribute to the plasma sheet closure currents. Still in early theoretical speculations, the tail currents were believed to close at high latitudes via theta-shaped dual vortex loops. However, ‘wire’ models based on that notion (Olson and Pfitzer, 1974) could not accurately confine the field inside the magnetopause. An alternative solution was just to extend the equatorial current beyond the magnetosphere and simulate the magnetic effect of closure currents by adding ad hoc curl-free fields. In spite of lack of explicitly defined magnetopause, such models (TU82, T87, T89) still provided fairly realistic configurations, owing to their flexibility and reliance on large amounts of spacecraft data. The magnetopause appeared in those models as a de facto comet-shaped surface separating the field lines with and without topological connection to Earth.
At the end of 1980s, the advent of perestroika brought major upheavals, both on the large societal scale and in my personal life and scientific activities. In the spring of 1989, a joint US–USSR Space Science workshop was held in Moscow, and it was there that my first personal meeting with David Stern took place. Owing to his efforts, I was offered an NRC fellow position at GSFC, which started in February 1992 and opened a new 15-years long page in my personal life and magnetospheric modeling studies.
By that time, enough data of direct magnetopause crossings had been collected, which allowed to create first empirical models of the boundary ((Sibeck et al., 1991), (Roelof and Sibeck, 1993)) and explicitly introduce the magnetopause in the data-based models. The main task was to find a shielding method, not limited to specific boundary shapes. Previous models used simple surfaces that allowed to expand the shielding potential into series of eigenfunctions. Only a few such surfaces satisfied that criterion, in particular, a paraboloid ((Alexeev and Shabansky, 1972), (Stern, 1985)) or an ellipsoid (Tsyganenko, 1989b); a composite model was also developed (Voigt, 1981) combining a cylinder with a hemisphere. All such models shared a common deficiency: lack of flexibility. A breakthrough idea was conceived by Schulz and McNab (Schulz and McNab, 1987): instead of seeking an exact solution of Neumann’s problem, they suggested to derive the shielding field by minimizing the residual flux across the ‘source-surface’ boundary. An advantage of that method was that, instead of a limited number of analytic boundaries, it allowed to represent the magnetopause by any suitable surface and tap the immense variety of curl-free shielding fields. In the data-based modeling, that method was first implemented in the T96 model ((Tsyganenko, 1995), (Tsyganenko et al., 1996)) and serves since then as the unfailing workhorse.
Another major complication stemmed from that the geodipole axis is tilted by
Incidentally, the observed tilt-related effects raise an interesting physics question. Still in early studies (Russell and Brody, 1967), it was found that the deformed tail current assumes a trough-like shape, elevated at midnight but depressed at flanks or vice versa. Much later (Arridge et al., 2008), a similar effect was detected in Saturn’s magnetosphere; the most interesting feature was the bowl-shaped deformation of the equatorial current, shifted away from the magnetic equator in the same direction at all longitudes. At first sight, that might appear as a direct “blowing-off” by the solar wind; however, since the solar wind cannot penetrate inside the magnetosphere, some other factors should be at work. A simple explanation was proposed in a study made with my student Varya Andreeva (Tsyganenko and Andreeva, 2014), based on calculating the inverse mirror ratio ɛ = Bmin/B in a vacuum model with fully shielded tilted dipole. Even though the model did not include any internal currents, the obtained ɛ distributions were found to be strikingly similar to the observed bowl-shaped current. In the limit of a “pole-on” magnetosphere with 90° tilt angle, the ɛ pattern turned into an axisymmetric surface resembling the simulated current [e.g. (Eggington et al., 2020)]. Therefore, the bowl-shaped deformation is just a combined effect of the North-South asymmetry induced by the dipole tilt and the day-night asymmetry due to the solar wind flow.
The principal goal of the empirical modeling is to reconstruct the observed or expected state of a system using the entire body of experimental information. Because of the complexity of Sun-Earth interactions, the main problem is to optimally relate the model parameters to the state of the magnetosphere and its external drivers. Due to lack of the solar wind data, early models were binned into consecutive intervals of the Kp index. After mid-1990s, a more or less continuous monitoring of the solar wind began, which allowed to parameterize the models using both interplanetary and ground-based input. The original approach was to represent the field as a sum of terms (modules) associated with basic current systems, relate their coefficients to external drivers and/or ground indices and fit the model to the entire database. It was shortly realized that the magnetospheric inertia is important, such that not only the driving intensity, but also its previous history was a factor. A simple analogy with the Dst index prediction (Burton et al., 1975) prompted to represent the individual module magnitudes as a result of continuous competition between the external pumping and internal dissipation. That concept was implemented in our joint work with Misha Sitnov (Tsyganenko and Sitnov, 2005) and resulted in a popular storm-time TS05 model.
My following collaboration with Misha resulted in two important developments, of which the first one was to remove the assumption of axial symmetry in the T89 tail disk current and, instead of seeking simple analytical solutions, represent the convolution integrals by discrete series over a set of radial wave numbers and azimuthal harmonics. Because of lack of axial symmetry, the problem could no longer be solved easily by using a vector potential. Instead, we had to first define two separate scalar potentials northward and southward from the current sheet, and then convert them into a single vector potential using a method discovered by luck in a paper by Stern (Stern, 1987). In its final formulation (Tsyganenko and Sitnov, 2007) the new TS07 model represented the field by double Fourier-Bessel series with free coefficients. This opened the way to construct new-generation models with (theoretically) unlimited resolution in the radial distance and longitude angle.
The second upgrade area (Sitnov et al., 2008) dealt with the model parameterization and resulted in a complete revision of the previous approach. In essence, the new method reincarnated the original data binning used in early models, but raised it to a sophisticated level rooted in the formal systems theory. Namely, the system’s evolution was modeled by following its trajectory in the state space and selecting data from a limited volume around the moment of interest. As a result, the magnetosphere dynamics was reconstructed by creating a sequence of ‘instantaneous’ submodels, each fitted to relatively small data subsets, mined from the whole database by means of the ‘nearest-neighbor’ (NN) technique. In tandem with the high-resolution TS07 framework, the NN data mining (DM) revealed many important features previously beyond the reach of empirical models. That marked a genuine leap forward (if not a revolution) in the empirical modeling of the magnetosphere, which already produced a number of interesting results (e.g. (Sitnov et al., 2020), (Tsyganenko et al., 2021a), and references therein).
In the summer of 2007, after 15 years at Goddard I moved back to my alma mater to accept a teaching position and continue my modeling studies. In that year, five Themis satellites were launched, adding to the flurry of data the Cluster tetrahedron has already been contributing since its launch in 2001. The fast buildup of magnetospheric data archives opened new prospects for the modeling and called for even more powerful methods. To that end, an idea emerged to construct a high-resolution model from finite elements covering not only the equatorial plane as in the TS07, but extending into the entire 3D space. Another innovation was to make the building blocks local, which allowed to easily shrink the model’s field of view to a limited area of interest. A feasible solution was eventually found, based on representing the magnetic field as a sum of toroidal and poloidal parts and expanding their generating functions into sums of radial/cylindrical basis functions (RBF/CBF), each centered around a 3D system of nodes. As a realization of that idea, a new model (Tsyganenko and Andreeva, 2016) was constructed, followed by several local studies focused on isolated regions around dayside cusps (Tsyganenko and Andreeva, 2018) or low-latitude magnetosphere (Tsyganenko and Andreeva, 2020). A potentially promising approach (Tsyganenko and Andreeva, 2017), conceived in parallel with the purely RBF/CBF-based models, was to combine the latter with old modular frameworks within hybrid architectures, in which the modular part is fitted to data first, and then the RBF/CBF part is determined, playing thus a role of higher-order correction term.
The page limits do not allow for a more detailed coverage of the subject. An important topic with many interesting aspects that I had to completely leave outside this memoir, is the spacecraft data and methods of their ingestion into the empirical models. A more or less detailed coverage of that subject can be found in a recent review (Tsyganenko et al., 2021b).
In summary (and keeping in mind primary goals of this Perspective issue), I would like to briefly formulate my view of the future prospects of the data-based modeling. Unlike in meteorology, the basic curse of space weather forecasting is lack and irregularity of data from very limited number of satellites probing the magnetosphere at a given moment. As already convincingly demonstrated ((Sitnov et al., 2020), (Stephens et al., 2020)), that shortage can be effectively solved using the DM and deep-learning methods, based on tapping huge resources of archived data. First-principle simulations on the other hand, while rapidly improving in their realism, often provide a quantitatively biased view of what is actually going on in the magnetosphere. A highly promising solution would be to synergistically combine the two approaches, using the DM-based empirical models as a source of virtual assimilation data.
The original contributions presented in the study are included in the article/Supplementary Material, further inquiries can be directed to the corresponding author.
The author confirms being the sole contributor of this work and has approved it for publication.
The author's studies in the field of magnetospheric modeling have been supported by the Russian Foundation for Basic Research grants 17-05-00415 and 20-05-00218.
The author acknowledges support of RFBR grant 20–05-00218.
The author declares that the research was conducted in the absence of any commercial or financial relationships that could be construed as a potential conflict of interest.
All claims expressed in this article are solely those of the authors and do not necessarily represent those of their affiliated organizations, or those of the publisher, the editors and the reviewers. Any product that may be evaluated in this article, or claim that may be made by its manufacturer, is not guaranteed or endorsed by the publisher.
Abt, H. A. (2000). The most frequently cited astronomical papers published during the past decade. Bull. Am. Astronomical Soc. 32, 937. https://adsabs.harvard.edu/full/2000BAAS…32..937A.
Alexeev, I. I., and Shabansky, V. P. (1972). A model of a magnetic field in the geomagnetosphere. Planet. Space Sci. 20 (1), 117–133. doi:10.1016/0032-0633(72)90146-8
Arridge, C. S., Russell, C. T., Khurana, K. K., Achilleos, N., Cowley, S. W. H., Dougherty, M. K., et al. (2008). Saturn's magnetodisc current sheet. J. Geophys. Res. 113 (8). doi:10.1029/2007ja012540
Burton, R. K., McPherron, R. L., and Russell, C. T. (1975). An empirical relationship between interplanetary conditions and Dst. J. Geophys. Res. 80 (31), 4204–4214. doi:10.1029/JA080i031p04204
Connerney, J. E. P., and Ness, N. F. (1981). AcuaModeling the jovian current sheet and inner magnetosphere. J. Geophys. Res. 86 (A10), 8370–8384. doi:10.1029/JA086iA10p08370
Eggington, J. W. B., Eastwood, J. P., Mejnertsen, L., Desai, R. T., and Chittenden, J. P. (2020). Dipole tilt effect on magnetopause reconnection and the steady-state magnetosphere-ionosphere system: global MHD simulations. J. Geophys. Res. Space Phys. 125, e2019JA027510. doi:10.1029/2019JA027510
Mead, G. D., and Fairfield, D. H. (1975). A quantitative magnetospheric model derived from spacecraft magnetometer data. J. Geophys. Res. 80 (4), 523–534. doi:10.1029/JA080i004p00523
Olson, W. P., and Pfitzer, K. A. (1974). A quantitative model of the magnetospheric magnetic field. J. Geophys. Res. 79 (25), 3739–3748. doi:10.1029/JA079i025p03739
Pudovkin, M. I., and Tsyganenko, N. A. (1973). Particle motion and currents in the neutral sheet of the magnetospheric tail. Planet. Space Sci. 21, 2027–2037. doi:10.1016/0032-0633(73)90131-1
Roelof, E. C., and Sibeck, D. G. (1993). Magnetopause shape as a bivariate function of interplanetary magnetic fieldBzand solar wind dynamic pressure. J. Geophys. Res. 98 (12), 21421450–21450. doi:10.1029/93ja02362
Russell, C. T., and Brody, K. I. (1967). Some remarks on the position and shape of the neutral sheet. J. Geophys. Res. 72 (23), 6104–6106. doi:10.1029/jz072i023p06104
Schulz, M., and McNab, M. C. (1987). Source-surface model of the magnetosphere. Geophys. Res. Lett. 14 (3), 182–185. doi:10.1029/gl014i003p00182
Sergeev, V. A., Chernyaeva, S. A., Apatenkov, S. V., Ganushkina, N. Y., and Dubyagin, S. V. (2015). Energy-latitude dispersion patterns near the isotropy boundaries of energetic protons. Ann. Geophys. 33, 1059–1070. doi:10.5194/angeo-33-1059-2015
Sergeev, V. A., Malkov, M., and Mursula, K. (1993). Testing the isotropic boundary algorithm method to evaluate the magnetic field configuration in the tail. J. Geophys. Res. 98 (5), 7609–7620. doi:10.1029/92JA02587
Sergeev, V. A., Sazhina, E. M., Tsyganenko, N. A., Lundblad, J. A., and Soraas, F. (1983). Pitch angle scattering of energetic protons in the magnetotail current sheet as the dominant source of their isotropic precipitation into the nightside ionosphere. Planet. Space Sci. 31, 1147–1155. doi:10.1016/0032-0633(83)90103-4
Sergeev, V. A., and Tsyganenko, N. A. (1982). Energetic particle losses and trapping boundaries as deduced from calculations with a realistic magnetic field model. Planet. Space Sci. 30 (10), 999–1006. doi:10.1016/0032-0633(82)90149-0
Sibeck, D. G., Lopez, R. E., and Roelof, E. C. (1991). Solar wind control of the magnetopause shape, location, and motion. J. Geophys. Res. 96 (4), 5489. doi:10.1029/90ja02464
Sitnov, M. I., Stephens, G. K., Tsyganenko, N. A., Korth, H., Roelof, E. C., Brandt, P. C., et al. (2020). Reconstruction of extreme geomagnetic storms: breaking the data paucity curse. Space weather. 18, e2020SW002561. doi:10.1029/2020SW002561
Sitnov, M. I., Tsyganenko, N. A., Ukhorskiy, A. Y., and Brandt, P. C. (2008). Dynamical data-based modeling of the stormtime geomagnetic field with enhanced spatial resolution. J. Geophys. Res. 113, A07218. doi:10.1029/2007JA013003
Stephens, G. K., Bingham, S. T., Sitnov, M. I., Gkioulidou, M., Merkin, V. G., Korth, H., et al. (2020). Storm time plasma pressure inferred from multimission measurements and its validation using Van Allen Probes particle data. Space weather. 18, e2020SW002583. doi:10.1029/2020SW002583
Stern, D. P. (1985). Parabolic harmonics in magnetospheric modeling: the main dipole and the ring current. J. Geophys. Res. 90 (11), 863. doi:10.1029/ja090ia11p10851
Stern, D. P. (1987). Tail modeling in a stretched magnetosphere: 1. methods and transformations. J. Geophys. Res. 92 (5), 4437. doi:10.1029/JA092iA05p04437
Tsyganenko, N. A. (1989a). A magnetospheric magnetic field model with a warped tail current sheet. Planet. Space Sci. 37 (1), 5–20. doi:10.1016/0032-0633(89)90066-4
Tsyganenko, N. A. (1989b). A solution of the chapman-ferraro problem for an ellipsoidal magnetopause. Planet. Space Sci. 37 (9), 1037–1046. doi:10.1016/0032-0633(89)90076-7
Tsyganenko, N. A., and Andreeva, V. A. (2017). A hybrid approach to empirical magnetosphere modeling. J. Geophys. Res. Space Phys. 122, 8198–8213. doi:10.1002/2017JA024359
Tsyganenko, N. A., and Andreeva, V. A. (2016). An empirical RBF model of the magnetosphere parameterized by interplanetary and ground-based drivers. J. Geophys. Res. Space Phys. 121 (10), 10786–10802. doi:10.1002/2016JA023217
Tsyganenko, N. A., and Andreeva, V. A. (2018). Empirical modeling of dayside magnetic structures associated with polar cusps. J. Geophys. Res. Space Phys. 123, 9078–9092. doi:10.1029/2018JA025881
Tsyganenko, N. A., Andreeva, V. A., Kubyshkina, M. V., Sitnov, M. I., and Stephens, G. K. (2021). “Data-based modeling of the Earth’s magnetic field,” in Space Physics and aeronomy collection volume 2: Magnetospheres in the solar system, geophysical monograph 259Romain maggiolo, nicolas andre, hiroshi hasegawa, and daniel T. Welling. American geophysical union. First Edition (Hoboken: Wiley & Sons, Inc.). doi:10.1002/9781119815624.ch39
Tsyganenko, N. A., and Andreeva, V. A. (2020). Magnetospheric “penetration” of IMF viewed through the lens of an empirical RBF modeling. JGR. Space Phys. 125, e2019JA027439. doi:10.1029/2019JA027439
Tsyganenko, N. A., and Andreeva, V. A. (2014). On the ‘bowl-shaped’ deformation of planetary equatorial current sheets. Geophys. Res. Lett. 41 (4), 1079–1084. doi:10.1002/2014gl059295
Tsyganenko, N. A., Andreeva, V. A., Sitnov, M. I., Stephens, G. K., Gjerloev, J. W., Chu, X., et al. (2021). Reconstructing substorms via historical data mining: Is it really feasible? JGR. Space Phys. 126, e2021JA029604. doi:10.1029/2021JA029604
Tsyganenko, N. A. (1996). “Effects of the solar wind conditions on the global magnetospheric configuration as deduced from data-based models,” in International conference on substorms, proceedings of the 3rd international conference. Editors E. J. Rolfe,, and B. Kaldeich (Paris: Publication ESA SP-389, European Space Agency), 181–185.
Tsyganenko, N. A. (1987). Global quantitative models of the geomagnetic field in the cislunar magnetosphere for different disturbance levels. Planet. Space Sci. 35 (11), 1347–1358. doi:10.1016/0032-0633(87)90046-8
Tsyganenko, N. A. (1998). Modeling of twisted/warped magnetospheric configurations using the general deformation method. J. Geophys. Res. 103 (10), 23551–23563. doi:10.1029/98JA02292
Tsyganenko, N. A. (1995). Modeling the Earth’s magnetospheric magnetic field confined within a realistic magnetopause. J. Geophys. Res. 100 (4), 5599. doi:10.1029/94JA03193
Tsyganenko, N. A. (1982). Pitch-angle scattering of energetic particles in the current sheet of the magnetospheric tail and stationary distribution functions. Planet. Space Sci. 30 (5), 433–437. doi:10.1016/0032-0633(82)90052-6
Tsyganenko, N. A., and Sitnov, M. I. (2007). Magnetospheric configurations from a high-resolution data-based magnetic field model. J. Geophys. Res. 112, A06225. doi:10.1029/2007JA012260
Tsyganenko, N. A., and Sitnov, M. I. (2005). Modeling the dynamics of the inner magnetosphere during strong geomagnetic storms. J. Geophys. Res. 110, A03208. doi:10.1029/2004JA010798
Tsyganenko, N. A., and Usmanov, A. V. (1982). Determination of the magnetospheric current system parameters and development of experimental geomagnetic field models based on data from IMP and HEOS satellites. Planet. Space Sci. 30 (10), 985–998. doi:10.1016/0032-0633(82)90148-9
Voigt, G.-H. (1981). A mathematical magnetospheric field model with independent physical parameters. Planet. Space Sci. 29 (1), 1–20. doi:10.1016/0032-0633(81)90134-3
Keywords: magnetosphere, modeling, satellite data, magnetopause, geomagnetic storms
Citation: Tsyganenko NA (2022) A lifetime with models, or toils and thrills of number crunching. Front. Astron. Space Sci. 9:934216. doi: 10.3389/fspas.2022.934216
Received: 02 May 2022; Accepted: 05 July 2022;
Published: 16 August 2022.
Edited by:
Joseph E. Borovsky, Space Science Institute, United StatesReviewed by:
Michael Schulz, Lockheed Martin Solar and Astrophysics Laboratory (LMSAL), United StatesCopyright © 2022 Tsyganenko . This is an open-access article distributed under the terms of the Creative Commons Attribution License (CC BY). The use, distribution or reproduction in other forums is permitted, provided the original author(s) and the copyright owner(s) are credited and that the original publication in this journal is cited, in accordance with accepted academic practice. No use, distribution or reproduction is permitted which does not comply with these terms.
*Correspondence: Nikolai A. Tsyganenko , bmlrb2xhaS50c3lnYW5lbmtvQGdtYWlsLmNvbQ==
Disclaimer: All claims expressed in this article are solely those of the authors and do not necessarily represent those of their affiliated organizations, or those of the publisher, the editors and the reviewers. Any product that may be evaluated in this article or claim that may be made by its manufacturer is not guaranteed or endorsed by the publisher.
Research integrity at Frontiers
Learn more about the work of our research integrity team to safeguard the quality of each article we publish.