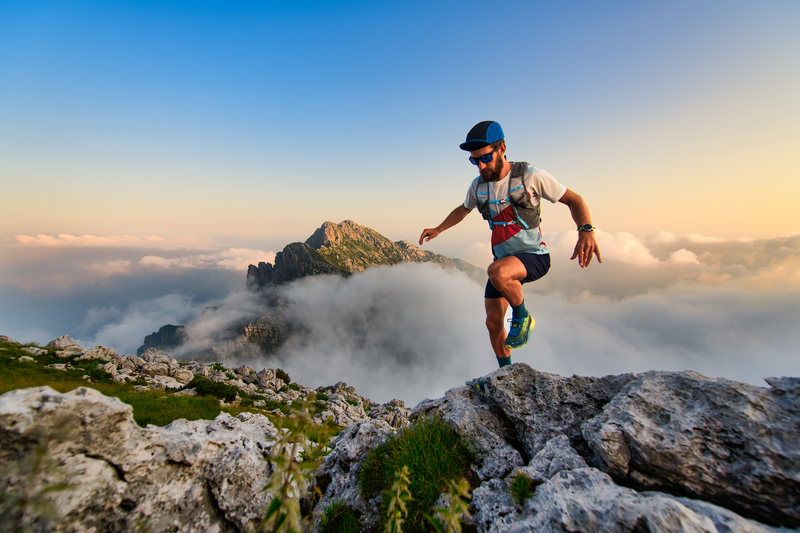
95% of researchers rate our articles as excellent or good
Learn more about the work of our research integrity team to safeguard the quality of each article we publish.
Find out more
EDITORIAL article
Front. Astron. Space Sci. , 05 October 2022
Sec. Astrostatistics
Volume 9 - 2022 | https://doi.org/10.3389/fspas.2022.1045609
This article is part of the Research Topic Asteroid Modeling: Processing and Combining Diverse Datasets View all 6 articles
Editorial on the Research Topic
Asteroid modeling: Processing and combining diverse datasets
The main goal of this collection was to enhance the understanding of individual asteroids and asteroid populations using information from multiple divergent datasets.
For decades now, we have seen a continuous exponential growth of data in asteroid science resulting from targeted observations as well in data originating from surveys dedicated to other astronomical objects but containing serendipitous asteroid measurements. These measurements are diverse from sparse to dense in time, differ in type (occultations vs. photometry) and are often burdened with different systematic and random errors. Combing and processing those distinct-in-nature data thus becomes a conceptual and computational challenge. Furthermore, comparison of results based on various datasets and methods may also prove problematic.
These problems are addressed in this Research Topic in the context of modelling asteroids in four original research papers and one mini-review. The articles in this issue range from applying machine learning methods to more traditional modelling. Machine learning algorithms are exploited in Penttilä et al.; Klimczak et al., whereas more conventional methods are considered by Muinonen et al.; Frenandez-Valenzuela, and the work of Durech et al. utilizes both types of modelling. Various data types are processed from simulated spectroscopic data in Penttilä et al. through the photometric data from large surveys such as the ATLAS (Durech et al.), Gaia mission (Muinonen et al.), and traditional ground-based spectra (Klimczak et al.).
Classification of asteroids into taxonomic classes is considered by Klimczak et al.; Penttilä et al. The work of Pentillä et al. focuses on introducing and testing tools for the future Vera C. Rubin Observatory. The authors train a neural network (NN) with the aim of classifying objects into the classes of the Bus-DeMeo scheme. They report an overall 90% prediction accuracy for classifying the data into 11 categories. The bottom-line conclusion is that NNs provide a promising prospect for characterizing asteroids based on multi-filter survey data. Klimczak et al. explore several algorithms (logistic regression, naïve Bayes, support vector machines, gradient boosting, and multilayer perceptron) with the main goal of identifying the key features that contribute the most towards classification of asteroids into the Bus-DeMeo classes. The article arrives at a recommendation of filters to be used in future surveys to optimize the scientific output toward asteroid characterization. Those papers highlight the importance of machine learning algorithms and future surveys in taxonomical characterization of asteroids.
The articles by Durech et al.; Muinonen et al. focus on photometric data. Durech et al. processed data for about 5,000 asteroids to determine their rotational periods based on roughly 100,000 measurements from the ATLAS survey. The computations were performed in distributed computing project Asteroids@home. The authors combined the lightcurve inversion method with a bootstrapping technique and determined reliable periods for thousands of asteroids. Muinonen et al. present a Bayesian lightcurve inversion method for retrieval of asteroid phase functions. The method is applied to a combined sparse space-based and dense ground-based data resulting in absolute magnitudes and phase-functions for about 500 asteroids. Development of such methods and computing techniques that combine diverse data types is forced by the data explosion, and often leads to more constrained model parameters.
The article by Frenandez-Valenzuela is a mini-review focusing on modelling trans-Neptunian objects and centaurs based on absolute measurements and lightcurve data. Frenandez-Valenzuela investigate the performance of models in obtaining various physical properties including pole orientations, shapes, densities, departure from hydrostatic equilibrium, and presence of rings. The authors point out that this sort of analysis of existing data or those arriving from future surveys will aid the recognition of asteroids that may possess rings or other peculiar features. Dedicated observation campaigns of these objects would help in understanding how common these bodies are and thus understand their formation and survivability.
Owing to the rise of big data multiple advances continue to be made in asteroid science in the data processing part, usage of modern machine learning methods, and development of novel methods allowing for incorporation of diverse data types. These approaches often must be tailored to the specific problems at hand. In particular, asteroid science often relies on serendipitous measurements originating from surveys and space missions with other primary targets, thus requiring more ingenuity and creativity in the data processing part. This is reflected in the current Research Topic.
All authors contributed to the editorial. DO wrote the preliminary version. Co-editors AP and JD provided comments and improved the text of the editorial.
The authors declare that the research was conducted in the absence of any commercial or financial relationships that could be construed as a potential conflict of interest.
All claims expressed in this article are solely those of the authors and do not necessarily represent those of their affiliated organizations, or those of the publisher, the editors and the reviewers. Any product that may be evaluated in this article, or claim that may be made by its manufacturer, is not guaranteed or endorsed by the publisher.
Keywords: asteroid, machine learning, lightcurve, spectra, solar system, photometry
Citation: Oszkiewicz DA, Durech J and Penttilä A (2022) Editorial: Asteroid modeling: Processing and combining diverse datasets. Front. Astron. Space Sci. 9:1045609. doi: 10.3389/fspas.2022.1045609
Received: 15 September 2022; Accepted: 16 September 2022;
Published: 05 October 2022.
Edited and reviewed by:
Didier Fraix-Burnet, UMR5274 Institut de Planétologie et d'Astrophysique de Grenoble (IPAG), FranceCopyright © 2022 Oszkiewicz, Durech and Penttilä. This is an open-access article distributed under the terms of the Creative Commons Attribution License (CC BY). The use, distribution or reproduction in other forums is permitted, provided the original author(s) and the copyright owner(s) are credited and that the original publication in this journal is cited, in accordance with accepted academic practice. No use, distribution or reproduction is permitted which does not comply with these terms.
*Correspondence: Dagmara Anna Oszkiewicz, ZGFnbWFyYS5vc3praWV3aWN6QGFzdHJvLmFtdS5lZHUucGw=
Disclaimer: All claims expressed in this article are solely those of the authors and do not necessarily represent those of their affiliated organizations, or those of the publisher, the editors and the reviewers. Any product that may be evaluated in this article or claim that may be made by its manufacturer is not guaranteed or endorsed by the publisher.
Research integrity at Frontiers
Learn more about the work of our research integrity team to safeguard the quality of each article we publish.