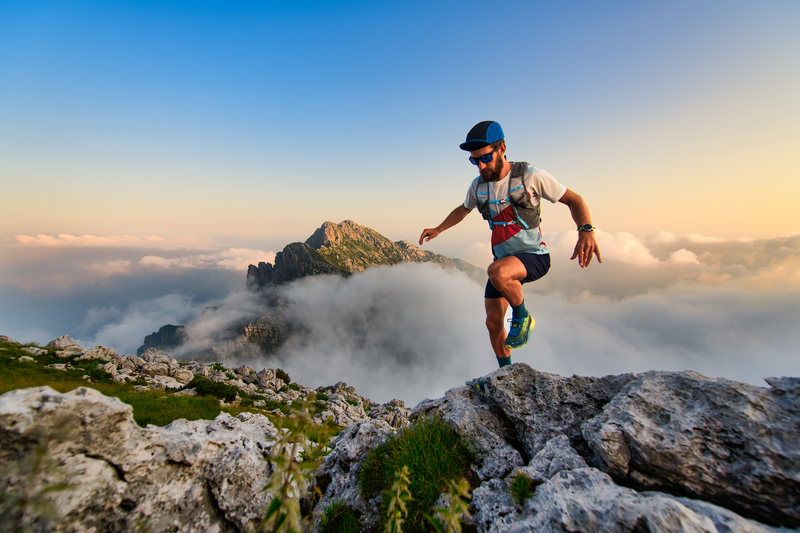
95% of researchers rate our articles as excellent or good
Learn more about the work of our research integrity team to safeguard the quality of each article we publish.
Find out more
PERSPECTIVE article
Front. Artif. Intell.
Sec. Medicine and Public Health
Volume 8 - 2025 | doi: 10.3389/frai.2025.1577529
The final, formatted version of the article will be published soon.
You have multiple emails registered with Frontiers:
Please enter your email address:
If you already have an account, please login
You don't have a Frontiers account ? You can register here
Artificial Intelligence (AI) in healthcare holds transformative potential but faces critical challenges in ethical accountability and systemic inequities. Biases in AI models, such as lower diagnosis rates for Black women or gender stereotyping in Large Language Models, highlight the urgent need to address historical and structural inequalities in data and development processes. Disparities in clinical trials and datasets, often skewed toward high-income, English-speaking regions, amplify these issues. Moreover, the underrepresentation of marginalized groups among AI developers and researchers exacerbates these challenges. To ensure equitable AI, diverse data collection, federated data-sharing frameworks, and bias-correction techniques are essential. Structural initiatives, such as fairness audits, transparent AI model development processes, and early registration of clinical AI models, alongside inclusive global collaborations like TRAIN-Europe and CHAI, can drive responsible AI adoption. Prioritizing diversity in datasets and among developers and researchers, as well as implementing transparent governance will foster AI systems that uphold ethical principles and deliver equitable healthcare outcomes globally.
Keywords: artificial intelligence, healthcare, Bias, diversity, Equity
Received: 16 Feb 2025; Accepted: 01 Apr 2025.
Copyright: © 2025 Hilling, Townsend, Gommers and van Genderen. This is an open-access article distributed under the terms of the Creative Commons Attribution License (CC BY). The use, distribution or reproduction in other forums is permitted, provided the original author(s) or licensor are credited and that the original publication in this journal is cited, in accordance with accepted academic practice. No use, distribution or reproduction is permitted which does not comply with these terms.
* Correspondence:
Denise E. Hilling, Erasmus Medical Center, Rotterdam, Netherlands
Disclaimer: All claims expressed in this article are solely those of the authors and do not necessarily represent those of their affiliated organizations, or those of the publisher, the editors and the reviewers. Any product that may be evaluated in this article or claim that may be made by its manufacturer is not guaranteed or endorsed by the publisher.
Research integrity at Frontiers
Learn more about the work of our research integrity team to safeguard the quality of each article we publish.