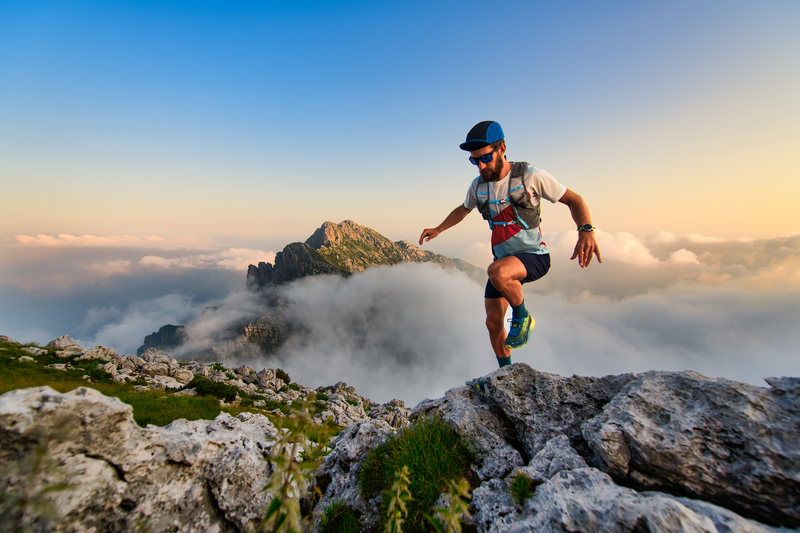
94% of researchers rate our articles as excellent or good
Learn more about the work of our research integrity team to safeguard the quality of each article we publish.
Find out more
ORIGINAL RESEARCH article
Front. Artif. Intell.
Sec. Pattern Recognition
Volume 8 - 2025 | doi: 10.3389/frai.2025.1565889
The final, formatted version of the article will be published soon.
You have multiple emails registered with Frontiers:
Please enter your email address:
If you already have an account, please login
You don't have a Frontiers account ? You can register here
Mine ventilation and dust control systems are crucial for ensuring occupational safety and health during underground mining operations. Traditional long-pressure short-suction systems face challenges such as inefficient airflow organization, formation of vortex dead zones, high energy consumption, and inadequate adaptability to dynamic conditions in mining faces. This study addresses these limitations by proposing an optimized long-pressure short-suction ventilation and dust control system leveraging the Coandă effect. Through numerical simulations, experimental validation, and machine learning techniques, the study develops a comprehensive system to enhance dust control performance. The Coandă effect was employed to optimize the structural design of ventilation ducts, ensuring airflow attachment to tunnel surfaces, reducing dust dispersion, and achieving high-efficiency airflow with lower power consumption. The key parameters optimized include the spacing between the air supply and exhaust ducts, the pressure-to-suction ratio, and the height of the ventilation duct. The optimal pressure-to-suction ratio was found to be 2:3, which minimizes dust concentration at both the mining machine and downstream locations. Numerical simulations and experimental results demonstrated that the optimized system achieved dust concentration reductions of up to 84.12% in high initial dust conditions (800 mg/m³). These findings provide a solid foundation for intelligent and energy-efficient ventilation and dust control in mining operations, ensuring both safety and energy savings.
Keywords: Mine ventilation, Coandă effect, Dust control, CNN-LSTM, Pressure-to-suction ratio
Received: 23 Jan 2025; Accepted: 06 Mar 2025.
Copyright: © 2025 Wang, Zhao, Li and Li. This is an open-access article distributed under the terms of the Creative Commons Attribution License (CC BY). The use, distribution or reproduction in other forums is permitted, provided the original author(s) or licensor are credited and that the original publication in this journal is cited, in accordance with accepted academic practice. No use, distribution or reproduction is permitted which does not comply with these terms.
* Correspondence:
Xinguo Wang, Shangwan Coal Mine, Ulanmulun Town, Ejin Horo Banner, Ordos, China
Disclaimer: All claims expressed in this article are solely those of the authors and do not necessarily represent those of their affiliated organizations, or those of the publisher, the editors and the reviewers. Any product that may be evaluated in this article or claim that may be made by its manufacturer is not guaranteed or endorsed by the publisher.
Research integrity at Frontiers
Learn more about the work of our research integrity team to safeguard the quality of each article we publish.