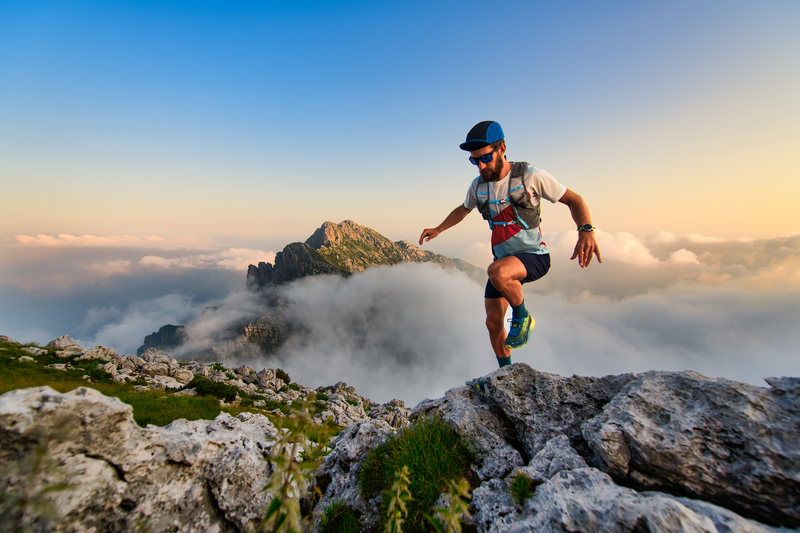
95% of researchers rate our articles as excellent or good
Learn more about the work of our research integrity team to safeguard the quality of each article we publish.
Find out more
ORIGINAL RESEARCH article
Front. Artif. Intell.
Sec. Medicine and Public Health
Volume 8 - 2025 | doi: 10.3389/frai.2025.1560523
The final, formatted version of the article will be published soon.
You have multiple emails registered with Frontiers:
Please enter your email address:
If you already have an account, please login
You don't have a Frontiers account ? You can register here
Lung ultrasound (LUS) has become an essential imaging modality for assessing various pulmonary conditions, including the presence of B-line artifacts. These artifacts are commonly associated with conditions such as increased extravascular lung water, decompensated heart failure, dialysis-related chronic kidney disease, interstitial lung disease, and COVID-19 pneumonia.Accurate detection of the B-line in LUS images is essential for effective diagnosis and treatment.However, interpreting LUS is often subject to observer variability, requiring significant expertise and posing challenges in resource-limited settings with few trained professionals. To address these limitations, deep learning models have been developed for automated B-line detection and localization. However, existing models do not precisely capture the artifacts' shapes and often fail to reveal their intricate textures and patterns. In addition, none has been integrated into mobile LUS screening tools for use in resource-constrained environments. This study introduces YOLOv5-PBB and YOLOv8-PBB, two modified models based on YOLOv5 and YOLOv8, respectively, designed for precise and interpretable B-line localization using polygonal bounding boxes (PBBs). YOLOv5-PBB was enhanced by modifying the detection head, loss function, nonmaximum suppression, and data loader to enable PBB localization. Meanwhile, YOLOv8-PBB was customized to convert segmentation masks into polygonal representations, displaying only boundaries while removing the masks. Additionally, we incorporated an image preprocessing technique into the models to enhance LUS image quality. Moreover, we integrated the smaller, faster inference model into a mobile LUS screening tool, making it accessible for resource-limited settings. The models were trained on a diverse dataset from a publicly available repository and Ugandan health facilities. Experimental results showed that YOLOv8-PBB achieved the highest precision (0.947), recall (0.926), and mean average precision (0.957). YOLOv5-PBB, while slightly lower in performance (precision: 0.931, recall: 0.918, mAP: 0.936), had advantages in model size (14 MB vs. 21 MB) and average inference time (33.1 ms vs. 47.7 ms), making it more suitable for real-time applications in low-resource settings.
Keywords: deep learning, Lung ultrasound, B-line artifact, localization, YOLO
Received: 14 Jan 2025; Accepted: 28 Mar 2025.
Copyright: © 2025 Okila, Katumba, Nakatumba-Nabende, Mwikirize, Murindanyi, Serugunda, Bugeza, Oriekot, Bossa and Nabawanuka. This is an open-access article distributed under the terms of the Creative Commons Attribution License (CC BY). The use, distribution or reproduction in other forums is permitted, provided the original author(s) or licensor are credited and that the original publication in this journal is cited, in accordance with accepted academic practice. No use, distribution or reproduction is permitted which does not comply with these terms.
* Correspondence:
Andrew Katumba, Makerere University, Kampala, Uganda
Eva Nabawanuka, Mulago Hospital, Kampala, Uganda
Disclaimer: All claims expressed in this article are solely those of the authors and do not necessarily represent those of their affiliated organizations, or those of the publisher, the editors and the reviewers. Any product that may be evaluated in this article or claim that may be made by its manufacturer is not guaranteed or endorsed by the publisher.
Research integrity at Frontiers
Learn more about the work of our research integrity team to safeguard the quality of each article we publish.