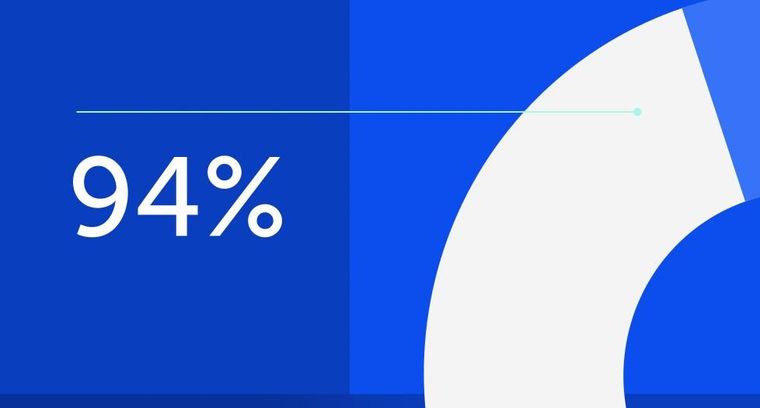
94% of researchers rate our articles as excellent or good
Learn more about the work of our research integrity team to safeguard the quality of each article we publish.
Find out more
ORIGINAL RESEARCH article
Front. Artif. Intell.
Sec. Machine Learning and Artificial Intelligence
Volume 8 - 2025 | doi: 10.3389/frai.2025.1546179
The final, formatted version of the article will be published soon.
Select one of your emails
You have multiple emails registered with Frontiers:
Notify me on publication
Please enter your email address:
If you already have an account, please login
You don't have a Frontiers account ? You can register here
The exponential growth of digital data, particularly in specialized domains like healthcare, necessitates advanced knowledge representation and integration techniques. RDF knowledge graphs offer a powerful solution, yet their creation and maintenance, especially for complex medical ontologies like SNOMED CT, remain challenging. Traditional methods often struggle with the scale, heterogeneity, and semantic complexity of medical data. This paper introduces a methodology leveraging the contextual understanding and reasoning capabilities of LLMs to automate and enhance medical ontology mapping for RDF knowledge graph construction. We conduct a comprehensive comparative analysis of six systems-GPT-4o, Claude 3.5 Sonnet v2, Gemini 1.5 Pro, Llama 3.3 70B, DeepSeek R1 and BERTMap-using a novel evaluation framework that combines quantitative metrics (precision, recall, F1-score) with qualitative assessments of semantic accuracy. Our approach integrates a data preprocessing pipeline with an LLM-powered semantic mapping engine, utilizing BioBERT embeddings and ChromaDB vector database for efficient concept retrieval. Experimental results on a dataset of 108 medical terms demonstrate the superior performance of modern LLMs, particularly GPT-4o, achieving a precision of 93.75% and an F1-score of 96.26%. These findings highlight the potential of LLMs in bridging the gap between structured medical data and semantic knowledge representation, towards more accurate and interoperable medical knowledge graphs.
Keywords: LLM, ontology, knowledge graph, Health data, RDF, SNOMED CT
Received: 16 Dec 2024; Accepted: 07 Apr 2025.
Copyright: © 2025 Mavridis, Tegos, Anastasiou, Papoutsoglou and Meditskos. This is an open-access article distributed under the terms of the Creative Commons Attribution License (CC BY). The use, distribution or reproduction in other forums is permitted, provided the original author(s) or licensor are credited and that the original publication in this journal is cited, in accordance with accepted academic practice. No use, distribution or reproduction is permitted which does not comply with these terms.
* Correspondence: Georgios Meditskos, Aristotle University of Thessaloniki, Thessaloniki, Greece
Disclaimer: All claims expressed in this article are solely those of the authors and do not necessarily represent those of their affiliated organizations, or those of the publisher, the editors and the reviewers. Any product that may be evaluated in this article or claim that may be made by its manufacturer is not guaranteed or endorsed by the publisher.
Supplementary Material
Research integrity at Frontiers
Learn more about the work of our research integrity team to safeguard the quality of each article we publish.