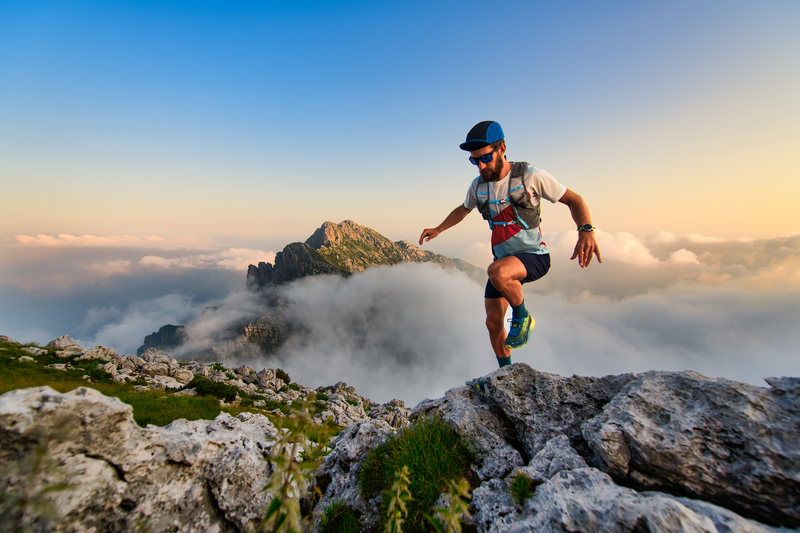
95% of researchers rate our articles as excellent or good
Learn more about the work of our research integrity team to safeguard the quality of each article we publish.
Find out more
ORIGINAL RESEARCH article
Front. Artif. Intell.
Sec. Machine Learning and Artificial Intelligence
Volume 8 - 2025 | doi: 10.3389/frai.2025.1545946
The final, formatted version of the article will be published soon.
You have multiple emails registered with Frontiers:
Please enter your email address:
If you already have an account, please login
You don't have a Frontiers account ? You can register here
This research proposes a novel approach to cryptographic key generation using biometric data from face and finger vein modalities, enhanced by deep learning techniques. By using pretrained models FaceNet and VGG19 for feature extraction and employing a Siamese Neural Network (SNN), the study demonstrates the integration of multimodal biometrics with fuzzy extractors to create secure and reproducible cryptographic keys. Feature fusion techniques, combined with preprocessing and thresholding, ensure robust feature extraction and conversion to binary formats for key generation. The model demonstrates impressive accuracy with a vector converter, achieving a sigma similarity of 93% and sigma difference of 64.0%. Evaluation metrics, including False Acceptance Rate (FAR) and False Rejection Rate (FRR), indicate significant improvements, achieving FRR < 3.4% and FAR < 1%, outperforming previous works. Additionally, the adoption of Goppa code-based cryptographic systems ensures post-quantum security. This work not only enhances biometric cryptography's accuracy and resilience but also paves the way for future exploration of quantum-resistant and scalable systems.
Keywords: deep learning, SNN, Multimodal biometrics, Post-quantum security, Cryptography key
Received: 16 Dec 2024; Accepted: 31 Mar 2025.
Copyright: © 2025 Yirga, YIRGA and Addisu. This is an open-access article distributed under the terms of the Creative Commons Attribution License (CC BY). The use, distribution or reproduction in other forums is permitted, provided the original author(s) or licensor are credited and that the original publication in this journal is cited, in accordance with accepted academic practice. No use, distribution or reproduction is permitted which does not comply with these terms.
* Correspondence:
Tsehayu Yirga, Mekdela Amba University, Dessie, Ethiopia
Disclaimer: All claims expressed in this article are solely those of the authors and do not necessarily represent those of their affiliated organizations, or those of the publisher, the editors and the reviewers. Any product that may be evaluated in this article or claim that may be made by its manufacturer is not guaranteed or endorsed by the publisher.
Research integrity at Frontiers
Learn more about the work of our research integrity team to safeguard the quality of each article we publish.