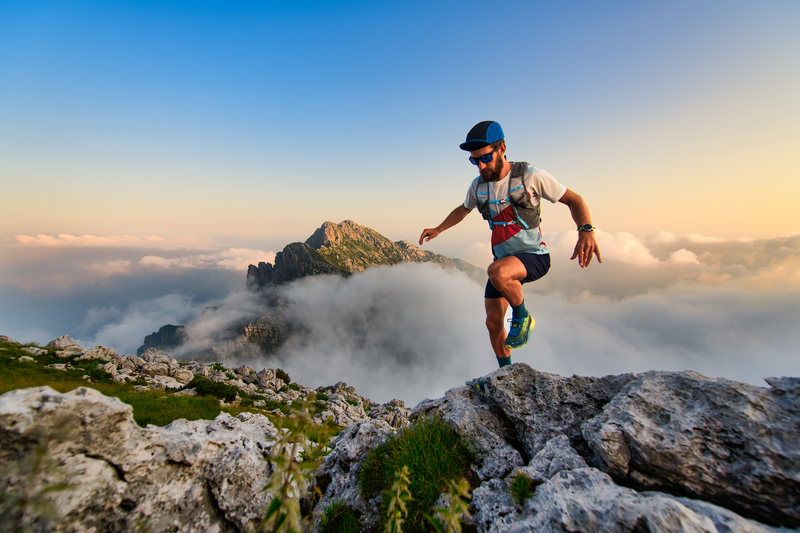
95% of researchers rate our articles as excellent or good
Learn more about the work of our research integrity team to safeguard the quality of each article we publish.
Find out more
ORIGINAL RESEARCH article
Front. Artif. Intell.
Sec. Medicine and Public Health
Volume 8 - 2025 | doi: 10.3389/frai.2025.1543603
The final, formatted version of the article will be published soon.
You have multiple emails registered with Frontiers:
Please enter your email address:
If you already have an account, please login
You don't have a Frontiers account ? You can register here
Introduction The emergence of Artificial Intelligence (AI) Large Language Models (LLMs), capable of producing text that closely resembles human-written content, brings about both opportunities and risks. While these developments offer considerable potential for improving communication, e.g. health-related crisis communication, they also present substantial dangers by enabling the creation of convincing fake news and disinformation. The widespread dissemination of AI-generated disinformation adds complexity to the existing challenges of the ongoing infodemic, with significant implications for public health and the stability of democratic institutions. Rationale Prompt engineering, a technique involving the creation of specific queries given to LLMs, has emerged as a strategy to guide LLMs in generating desired outputs. Recent research has shown that the output of LLMs depends on emotional framing within prompts, suggesting that incorporating emotional cues into prompts could influence their response behavior. In this study, we investigated whether the politeness or impoliteness of prompts influences the frequency of disinformation generation by various LLMs. Results We generated and evaluated a corpus of 19,800 social media posts on public health topics to assess the disinformation generation capabilities of OpenAI’s LLMs, including davinci-002, davinci-003, gpt-3.5-turbo and gpt-4. Our findings revealed that all LLMs successfully produced disinformation (davinci-002, 67%; davinci-003, 86%; gpt-3.5-turbo, 77%; and gpt-4, 99%). Introducing polite language to prompt requests yielded significantly higher success rates for disinformation (davinci-002, 79%; davinci-003, 90%; gpt-3.5-turbo, 94%; and gpt-4, 100%). Impolite prompting led to a strong reduction in disinformation production across all models (davinci-002, 59%; davinci-003, 44%; gpt-3.5-turbo, 28%) and a small reduction for gpt-4 (94%). Conclusion Our study reveals that all tested LLMs successfully produce disinformation. Notably, emotional prompting exerted a significant influence on disinformation production rates, with models displaying higher success rates when prompted with polite language when compared with neutral or impolite requests. Our investigation highlights that LLMs can be exploited to produce disinformation, and underlines the critical need for ethics-by-design approaches in the development of AI technologies. We argue that identifying ways to mitigate the exploitation of LLMs through emotional prompting is crucial to prevent their misuse for purposes detrimental to public health and society.
Keywords: AI, LLM, disinformation, misinformation, infodemic, emotional prompting, OpenAI, Ethics
Received: 11 Dec 2024; Accepted: 17 Mar 2025.
Copyright: © 2025 Vinay, Spitale, Biller-Andorno and Germani. This is an open-access article distributed under the terms of the Creative Commons Attribution License (CC BY). The use, distribution or reproduction in other forums is permitted, provided the original author(s) or licensor are credited and that the original publication in this journal is cited, in accordance with accepted academic practice. No use, distribution or reproduction is permitted which does not comply with these terms.
* Correspondence:
Federico Germani, Institute of Biomedical Ethics and History of Medicine, Faculty of Medicine, University of Zurich, Zurich, Switzerland
Disclaimer: All claims expressed in this article are solely those of the authors and do not necessarily represent those of their affiliated organizations, or those of the publisher, the editors and the reviewers. Any product that may be evaluated in this article or claim that may be made by its manufacturer is not guaranteed or endorsed by the publisher.
Research integrity at Frontiers
Learn more about the work of our research integrity team to safeguard the quality of each article we publish.