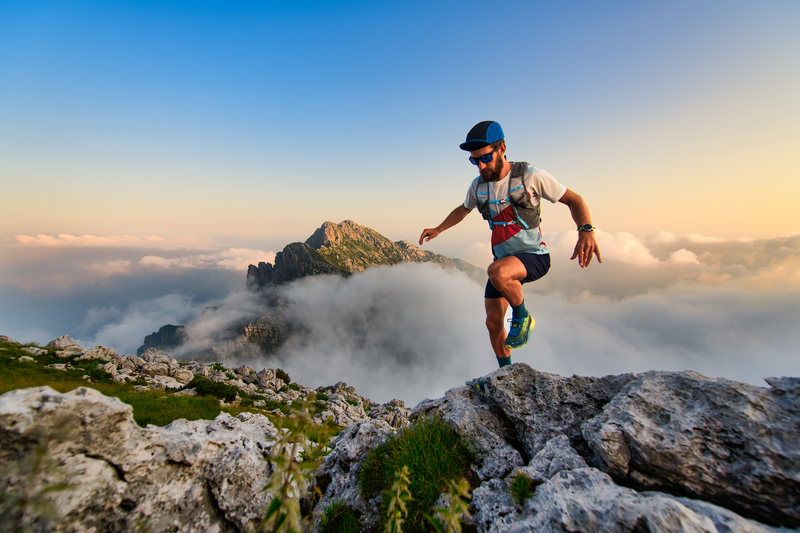
94% of researchers rate our articles as excellent or good
Learn more about the work of our research integrity team to safeguard the quality of each article we publish.
Find out more
BRIEF RESEARCH REPORT article
Front. Artif. Intell.
Sec. Machine Learning and Artificial Intelligence
Volume 8 - 2025 | doi: 10.3389/frai.2025.1542320
This article is part of the Research Topic Exploring the Power of AI and ML in Smart Grids: Advancements, Applications, and Challenges View all 5 articles
The final, formatted version of the article will be published soon.
You have multiple emails registered with Frontiers:
Please enter your email address:
If you already have an account, please login
You don't have a Frontiers account ? You can register here
This research presents an optimized system for predicting energy usage in smart grids by integrating the Temporal Fusion Transformer (TFT) with the Aquila Optimizer (AO). The study addresses the growing need for accurate energy consumption forecasts in smart grids, driven by the increasing adoption of renewable energy and real-time data collection through smart meters. The TFT model leverages self-attention mechanisms to handle complex timeseries data, improving forecasting accuracy across various time horizons. To enhance predictive performance, the Aquila Optimizer, a nature-inspired algorithm, is employed to finetune critical hyperparameters, ensuring optimal model convergence and performance. The proposed AO-TFT model is evaluated against traditional models like LSTM and CNN-BiLSTM, demonstrating superior accuracy, lower RMSE, and faster computation times. The research also analyses the impact of various factors, including building types, weather conditions, and load variations on energy prediction. The proposed AO-TFT model achieved a significantly lower RMSE of 0.48 and MAE of 0.31, demonstrating superior accuracy compared to traditional models.Future work is suggested to explore hybrid optimization techniques and real-time adaptive models for dynamic grid management.
Keywords: Smart Grids, Temporal Fusion Transformer, Aquila optimizer, energy usage prediction, deep learning, Nature-inspired optimization
Received: 09 Dec 2024; Accepted: 10 Feb 2025.
Copyright: © 2025 Badhe, Neve, Yele, Abhang, Dhule and Mali. This is an open-access article distributed under the terms of the Creative Commons Attribution License (CC BY). The use, distribution or reproduction in other forums is permitted, provided the original author(s) or licensor are credited and that the original publication in this journal is cited, in accordance with accepted academic practice. No use, distribution or reproduction is permitted which does not comply with these terms.
* Correspondence:
Namdeo Badhe, Thakur College of Engineering and Technology, Mumbai, India
Disclaimer: All claims expressed in this article are solely those of the authors and do not necessarily represent those of their affiliated organizations, or those of the publisher, the editors and the reviewers. Any product that may be evaluated in this article or claim that may be made by its manufacturer is not guaranteed or endorsed by the publisher.
Research integrity at Frontiers
Learn more about the work of our research integrity team to safeguard the quality of each article we publish.