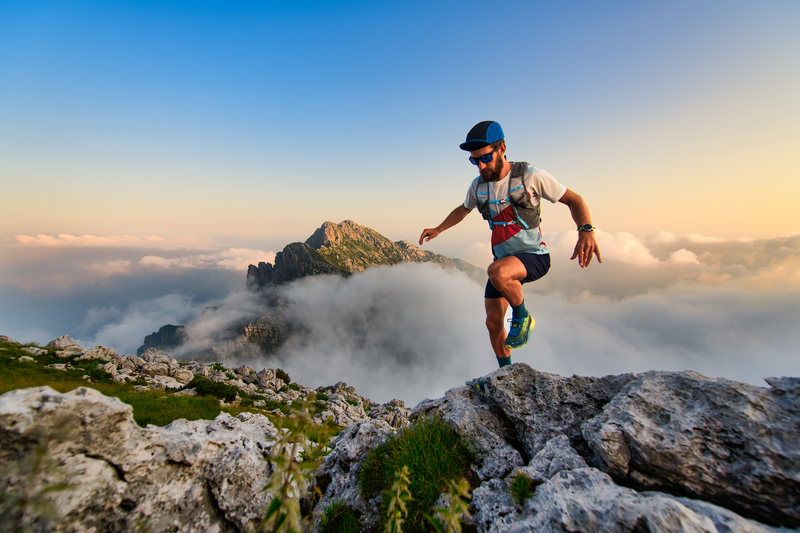
95% of researchers rate our articles as excellent or good
Learn more about the work of our research integrity team to safeguard the quality of each article we publish.
Find out more
ORIGINAL RESEARCH article
Front. Artif. Intell.
Sec. Pattern Recognition
Volume 8 - 2025 | doi: 10.3389/frai.2025.1529814
This article is part of the Research Topic AI-Enabled Breakthroughs in Computational Imaging and Computer Vision View all articles
The final, formatted version of the article will be published soon.
You have multiple emails registered with Frontiers:
Please enter your email address:
If you already have an account, please login
You don't have a Frontiers account ? You can register here
Wireless Capsule Endoscopy (WCE) enables non-invasive imaging of the digestive tract but generates vast video data, posing challenges for real-time and accurate abnormality detection. This paper presents a novel approach that uses a transformer-based model, specifically optimized for WCE video analysis, to improve both the precision and speed of object detection. The proposed method integrates the Real-Time Detection Transformer (RT-DETR) to address a unique challenge posed by WCE data, specifically the uncontrolled illumination variations, highly textured backgrounds, and the requirement for high-speed processing to handle vast amounts of video frames efficiently. The RT-DETR model is designed to capture contextual information between video frames effectively, leading to a more accurate detection of gastrointestinal abnormalities.The RT-DETR models achieved better detection performance on Kvasir-Capsule while operating at real-time speeds. RT-DETR-X demonstrated highest precision while RT-DETR-M succeeded in maintaining a practical balance of accuracy and processing speed. RT-DETR-S ran at 270 FPS for real-time analysis making it the most suitable model for WCE video applications. The experimental outcomes validate that RT-DETR can operate effectively for clinical applications through results which show better accuracy as well as computational efficiency than earlier frameworks.
Keywords: Capsule Endoscopy, object detection, real-time processing, Transformer models, Video Analysis, Wireless communication, medical imaging, deep learning
Received: 17 Nov 2024; Accepted: 03 Apr 2025.
Copyright: © 2025 Habe, Haataja and Toivanen. This is an open-access article distributed under the terms of the Creative Commons Attribution License (CC BY). The use, distribution or reproduction in other forums is permitted, provided the original author(s) or licensor are credited and that the original publication in this journal is cited, in accordance with accepted academic practice. No use, distribution or reproduction is permitted which does not comply with these terms.
* Correspondence:
Tsedeke Temesgen Habe, University of Eastern Finland, Kuopio, Finland
Disclaimer: All claims expressed in this article are solely those of the authors and do not necessarily represent those of their affiliated organizations, or those of the publisher, the editors and the reviewers. Any product that may be evaluated in this article or claim that may be made by its manufacturer is not guaranteed or endorsed by the publisher.
Research integrity at Frontiers
Learn more about the work of our research integrity team to safeguard the quality of each article we publish.