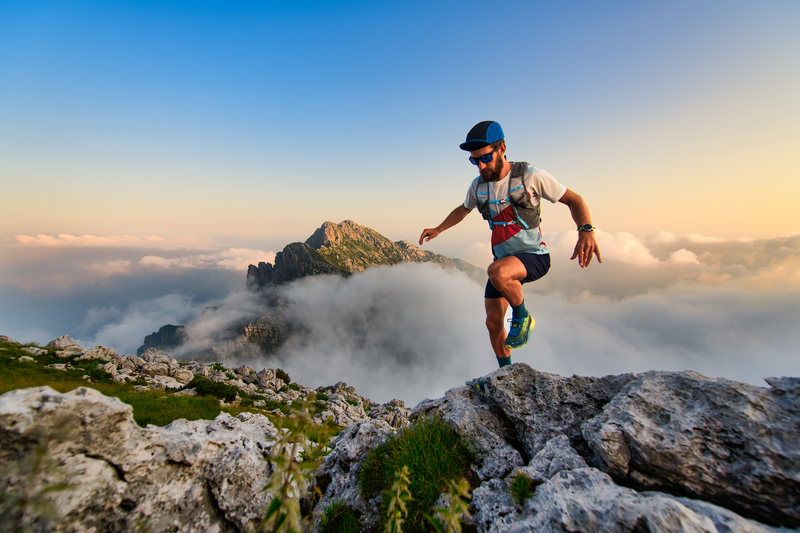
95% of researchers rate our articles as excellent or good
Learn more about the work of our research integrity team to safeguard the quality of each article we publish.
Find out more
ORIGINAL RESEARCH article
Front. Artif. Intell.
Sec. Machine Learning and Artificial Intelligence
Volume 8 - 2025 | doi: 10.3389/frai.2025.1522730
This article is part of the Research Topic Artificial Intelligence in Visual Inspection View all articles
The final, formatted version of the article will be published soon.
You have multiple emails registered with Frontiers:
Please enter your email address:
If you already have an account, please login
You don't have a Frontiers account ? You can register here
In modern medical diagnostics, the problem of accurately extracting structures with low contrast, a problem with existing segmentation methods, has been analyzed. To address this problem, the paper proposes a new approach that combines contour detection methods with a U-net deep learning model to enhance segmentation accuracy in chest X-ray images, focusing on lung, heart, and clavicle segmentation, which is essential for cardiothoracic disease diagnosis and reducing segmentation artifacts caused by overlapping structures. A method is developed to segment anatomical structures such as lungs, heart, and clavicles in chest X-ray images by integrating U-net with Sobel and Scharr filters for edge detection. The applied methods include stepwise processing, where contour detection improves the quality of the original data, and the U-net model performs accurate segmentation. The experimental results obtained showed a significant improvement in accuracy when using the Sobel filter compared to the baseline U-net model. For lung segmentation, an accuracy of 99.26 %, a Dice coefficient of 98.88 %, and a Jaccard index of 97.54 % were achieved, which exceeds the performance of previous methods. Similar results were obtained for heart segmentation – accuracy 99.47 %, Jaccard 94.14 %, and clavicles – accuracy 99.79 %, Jaccard 89.57 %. Thus, a method that integrates U-net with contour detection methods has been proposed, which provides a significant improvement in the quality of segmentation of the studied anatomical structures. In particular, the Sobel filter proved the most effective in improving segmentation in complex anatomical regions.
Keywords: Semantic segmentation, medical imaging, edge detection, deep learning, U-net model
Received: 04 Nov 2024; Accepted: 31 Mar 2025.
Copyright: © 2025 Mochurad. This is an open-access article distributed under the terms of the Creative Commons Attribution License (CC BY). The use, distribution or reproduction in other forums is permitted, provided the original author(s) or licensor are credited and that the original publication in this journal is cited, in accordance with accepted academic practice. No use, distribution or reproduction is permitted which does not comply with these terms.
* Correspondence:
Lesia Mochurad, Lviv Polytechnic National University, Lviv, 79013, Ukraine
Disclaimer: All claims expressed in this article are solely those of the authors and do not necessarily represent those of their affiliated organizations, or those of the publisher, the editors and the reviewers. Any product that may be evaluated in this article or claim that may be made by its manufacturer is not guaranteed or endorsed by the publisher.
Research integrity at Frontiers
Learn more about the work of our research integrity team to safeguard the quality of each article we publish.