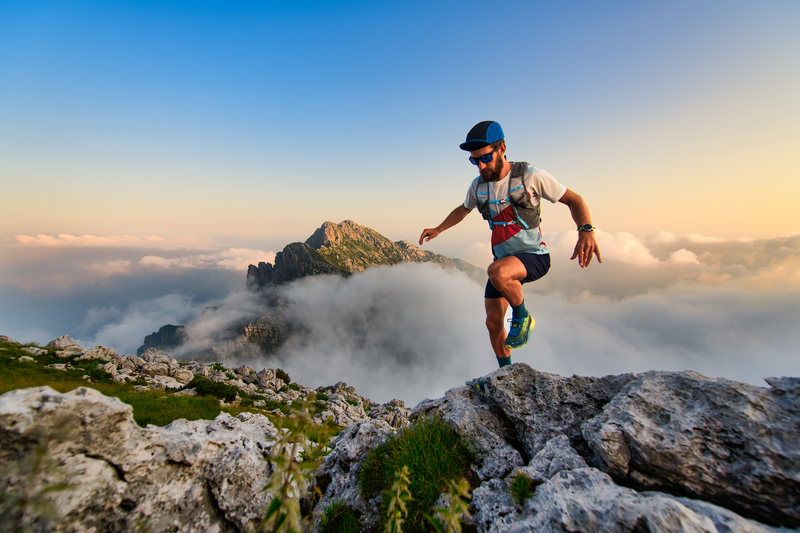
95% of researchers rate our articles as excellent or good
Learn more about the work of our research integrity team to safeguard the quality of each article we publish.
Find out more
REVIEW article
Front. Artif. Intell. , 30 January 2025
Sec. Medicine and Public Health
Volume 8 - 2025 | https://doi.org/10.3389/frai.2025.1518049
ChatGPT represents a transformative technology in healthcare, with demonstrated impacts across clinical practice, medical education, and research. Studies show significant efficiency gains, including 70% reduction in administrative time for discharge summaries and achievement of medical professional-level performance on standardized tests (60% accuracy on USMLE, 78.2% on PubMedQA). ChatGPT offers personalized learning platforms, automated scoring, and instant access to vast medical knowledge in medical education, addressing resource limitations and enhancing training efficiency. It streamlines clinical workflows by supporting triage processes, generating discharge summaries, and alleviating administrative burdens, allowing healthcare professionals to focus more on patient care. Additionally, ChatGPT facilitates remote monitoring and chronic disease management, providing personalized advice, medication reminders, and emotional support, thus bridging gaps between clinical visits. Its ability to process and synthesize vast amounts of data accelerates research workflows, aiding in literature reviews, hypothesis generation, and clinical trial designs. This paper aims to gather and analyze published studies involving ChatGPT, focusing on exploring its advantages and disadvantages within the healthcare context. To aid in understanding and progress, our analysis is organized into six key areas: (1) Information and Education, (2) Triage and Symptom Assessment, (3) Remote Monitoring and Support, (4) Mental Healthcare Assistance, (5) Research and Decision Support, and (6) Language Translation. Realizing ChatGPT’s full potential in healthcare requires addressing key limitations, such as its lack of clinical experience, inability to process visual data, and absence of emotional intelligence. Ethical, privacy, and regulatory challenges further complicate its integration. Future improvements should focus on enhancing accuracy, developing multimodal AI models, improving empathy through sentiment analysis, and safeguarding against artificial hallucination. While not a replacement for healthcare professionals, ChatGPT can serve as a powerful assistant, augmenting their expertise to improve efficiency, accessibility, and quality of care. This collaboration ensures responsible adoption of AI in transforming healthcare delivery. While ChatGPT demonstrates significant potential in healthcare transformation, systematic evaluation of its implementation across different healthcare settings reveals varying levels of evidence quality–from robust randomized trials in medical education to preliminary observational studies in clinical practice. This heterogeneity in evidence quality necessitates a structured approach to future research and implementation.
In the modern era, artificial intelligence is increasingly becoming an integral part of our daily lives, extending its influence beyond the healthcare sector (Jiang et al., 2017; Patel et al., 2009; Yu et al., 2018; Davenport and Kalakota, 2019; Rajpurkar et al., 2022; Dave et al., 2023; Sharma and Sharma, 2023; Sedaghat, 2023; Tustumi et al., 2023). Within the realm of artificial intelligence, a subset known as Large Language Models (LLMs) harnesses the power of deep learning and extensive data to comprehend, summarize, generate, and predict new content. These models rely on artificial neural networks trained on vast repositories of text data gathered from books, documents, websites, and other sources. Through rigorous training, LLMs acquire the ability to generate coherent and contextually relevant text by discerning patterns and correlations in their training data. This proficiency in language understanding and generation significantly benefits applications such as machine translation and text generation. Notably, several prominent companies, including OpenAI, Google, and Microsoft, have introduced chatbots powered by LLMs, as indicated in Table 1.
One noteworthy LLM in this landscape is ChatGPT, a product of OpenAI’s language model development efforts. OpenAI introduced the Generative Transformer pre-training (GPT) LLM model in 2018, featuring a variant of the Transformer architecture trained on a staggering 40GB of text data, incorporating 1.5 billion parameters (Floridi and Chiriatti, 2020). Subsequently, in 2020, GPT-3 emerged, trained on a colossal 570GB of text data, boasting 175 billion parameters, resulting in the creation of the GPT-3 End User Conversation Model (Sharma and Sharma, 2023).
ChatGPT has demonstrated its capabilities in diverse applications, including achieving success in all three print-ahead sections of the USMLE test (Vaswani et al., 2017; Kung et al., 2023). GPT-3.5, encompassing Codex and InstructGPT, achieved human-level performance in challenging evaluations such as USMLE (60.2%), MedMCQA (57.5%), and PubMedQA (78.2%) (Gilson et al., 2023). The integration of ChatGPT into healthcare systems represents a complex interplay between technological capability and systemic readiness. Recent systematic reviews indicate that while individual applications show promise, healthcare systems face significant challenges in standardizing and scaling AI implementation. These challenges span technical infrastructure, workflow integration, staff training, and policy frameworks - requiring careful consideration of both direct benefits and systemic impacts. However, the extent to which ChatGPT excels in complex real-world scenarios, particularly within domains as intricate as medicine, remains an open question. Moreover, ethical concerns surface when considering the use of chatbots for scientific paper writing.
In the early months of 2023, ChatGPT witnessed significant advancements, with the introduction of ChatGPT4. Presently, ChatGPT is finding practical application in everyday healthcare settings, proving beneficial in providing information, answering queries, and offering support to both healthcare professionals and patients (Liévin et al., 2022; Sallam, 2023; Ahn, 2023; Curtis and ChatGPT, 2023; D'Amico et al., 2023; Donato et al., 2023). This research paper aim to systematically review the deployment of ChatGPT across various domains, drawing from a comprehensive search and selecting pertinent publications from reputable sources like PubMed and Google Scholar. The aim is to discern the strengths and weaknesses of ChatGPT, enabling a deeper understanding of its capabilities and guiding further advancements in this field. To achieve this goal, the paper is structured around six distinct themes: (1) Information and Education, (2) Triage and Symptom Assessment, (3) Remote Monitoring and Support, (4) Mental Healthcare Support, (5) Research and Decision Support, and (6) Language Translation. These thematic areas will be thoroughly explored in subsequent sections of this paper.
ChatGPT has demonstrated exceptional capabilities in medical education and information delivery, achieving pass rates of 60.2% on USMLE examinations and consistently outperforming baseline metrics in medical knowledge assessment. Studies show particular strength in clinical reasoning tasks, with accuracy rates of 84% in lower-order thinking questions (Bhayana et al., 2023). This can significantly contribute to simplifying the understanding of intricate diseases (Jin and Dobry, 2023; Hamed et al., 2023; Hoch et al., 2023; Sharma et al., 2023). Khan et al. have taken steps towards developing a data-driven GPT chat model, conducting preliminary experiments that show promise for future applications in enhancing medical education and clinical management (Khan et al., 2023). Dr. David A. Asch, MD, engaged in an interview with ChatGPT to explore its role in healthcare, shedding light on critical considerations before its integration into everyday healthcare practices. These considerations encompass data privacy, security, regulatory compliance, data quality and quantity, integration with existing systems, human oversight, and ethical concerns (Asch, 2023).
Several publications have highlighted ChatGPT’s success in assisting with examinations (Vaswani et al., 2017; Kung et al., 2023; Gilson et al., 2023; Ragel et al., 2006; Fijačko et al., 2023; Choi et al., 2023; Hoch et al., 2023; Hopkins et al., 2023; Bhayana et al., 2023; Oh et al., 2023), underscoring its substantial knowledge base. However, it’s vital to recognize that passing an exam does not equate to becoming a fully competent medical professional. For instance, Hopkin et al. utilized ChatGPT in the context of the neurosurgical written board test, revealing that the model encountered difficulties when responding to image- or diagram-based questions, such as identifying specific anatomical structures. This limitation arises from ChatGPT’s inability to process visual information (Hopkins et al., 2023).
Similarly, Bhayana et al. applied ChatGPT to the Radiology board exam, subjecting it to a comprehensive assessment involving 150 multiple-choice questions designed to align with the standards of the Canadian Royal College and American Board of Radiology exams. These questions were categorized based on the cognitive skills required and encompassed physics and clinical aspects. ChatGPT achieved an overall accuracy rate of 69%, excelling in lower-order thinking questions (84%) compared to higher-order thinking questions (60%). However, the model required assistance in accurately addressing questions related to image description, computation, classification, and conceptual application. Conversely, it demonstrated strong performance in higher-order clinical management questions. In terms of specific topics, ChatGPT faced more challenges with physics questions than clinical ones. While the model achieved perfect accuracy in routine tasks, enhancements are essential to address the complexities posed by certain question types. In sum, these findings showcase ChatGPT’s effectiveness in responding to radiology-related questions, particularly in lower-order thinking and clinical management, while pinpointing areas necessitating further refinement (Bhayana et al., 2023).
ChatGPT comprehensively explains medical conditions, treatments, and preventive measures, aiding patient and professional education. Studies highlight its potential in medical board exams, though limitations arise in visual information processing. For example, Bhayana et al. noted ChatGPT’s struggles with radiology board questions requiring conceptual applications. Enhancing its training datasets could address these gaps. Moreover, ChatGPT could support automated scoring and content creation, advancing medical education.
ChatGPT can play a pivotal role in patient triage by posing questions regarding the patient’s symptoms and conducting an assessment based on their responses. If immediate treatment is warranted, timely notification to the doctor ensures continuity of care. This approach not only eases the workload on medical personnel but also helps reduce potential conflicts between healthcare providers and patients, thereby minimizing the likelihood of future legal issues.
Building upon the groundwork laid by Raita et al., Bhattaram et al. have harnessed machine learning techniques to enhance patient triage, achieving both accuracy and efficiency. However, it’s essential to acknowledge that the reliability of ChatGPT hinges on the training and knowledge embedded in the model, as illuminated by limitations acknowledged by OpenAI (Bhattaram et al., 2023; Raita et al., 2019).
Xue et al. have lauded ChatGPT as a notable advancement in AI technology. Nonetheless, its impact on clinical medicine remains somewhat constrained, given that clinical practice often depends on data analytics, clinical research, guidelines, and the performance of specialized AI models (Xue et al., 2023).
In the field of rheumatology, Nune et al. have explored ChatGPT’s utility across various domains, including patient education on disease-modifying drugs, medical imaging report generation, outpatient consultation note-keeping, medical education and training, and clinical audit and research. However, it’s essential to recognize that while ChatGPT demonstrates capabilities, occasional errors or inaccuracies may still arise. Therefore, consulting with a healthcare professional and providing feedback is pivotal to facilitate ongoing development and bolster AI’s capabilities in the future (Nune et al., 2023). While effective triage systems form the foundation of efficient healthcare delivery, the growing need for continuous patient care beyond clinical settings has highlighted the importance of remote monitoring solutions. The integration of ChatGPT into remote monitoring systems represents a natural progression from initial assessment to ongoing care management, particularly relevant in addressing healthcare access disparities and managing chronic conditions.
ChatGPT facilitates patient triage by analyzing symptom data and prioritizing care needs. Studies, such as those by Bhattaram et al., demonstrate its efficiency in streamlining workflows and reducing legal conflicts (Bhattaram et al., 2023; Raita et al., 2019). However, the model’s reliance on training data limits its adaptability to nuanced clinical scenarios. Future iterations must incorporate real-time data to enhance reliability.
Due to staffing challenges, particularly in remote regions, telemedicine has witnessed substantial growth in specific countries. In this context, ChatGPT emerges as a valuable tool for monitoring patient symptoms. Through interactive questioning, it facilitates the creation of symptom lists and provides guidance from healthcare professionals, thereby aiding in the care of patients with chronic conditions, medication adherence, and postoperative recovery.
Biswas et al. conducted an examination of ChatGPT’s application in public health, particularly within community health settings. Its utility spans multiple facets, including:
• Offering information on public health concerns
• Addressing queries regarding health promotion and disease prevention strategies
• Explaining the roles of community health workers and health educators
• Discussing social and environmental factors influencing community health
• Providing information about community health programs and services
However, it’s essential to acknowledge the limitations associated with ChatGPT in this context, encompassing:
• Limited accuracy
• Potential biases and constraints stemming from the underlying data
• A lack of contextual understanding
• Limited engagement capabilities
• The absence of direct interaction with healthcare professionals (Biswas, 2023)
Lin et al. delved into the transformative potential of AI in primary care practices, specifically focusing on AI’s role in chronic disease monitoring. Their findings indicated that AI-driven, fully automated, text-based health coaching could lead to successful weight loss compared to in-person lifestyle interventions (Lin et al., 2019).
Among the critical data elements for monitoring patient care, discharge summaries play a pivotal role (Royal College of Physicians, 2021; Earnshaw et al., 2020). Patel et al. explored the use of ChatGPT in generating discharge summaries, noting its effectiveness. However, they also identified instances where detailed information or inaccuracies might arise. These concerns can be addressed by having the attending physician review and approve the discharge summary, signifying their supervision. Moreover, utilizing ChatGPT for generating discharge summaries can significantly alleviate the workload associated with this task (Patel and Lam, 2023).
Within the realm of medical records, alongside the discharge summary, another vital component is the treatment record. This encompasses various aspects, such as patient history, physical examinations, treatment specifics, and even surgical records. Notably, there is a growing inclination towards embracing the use of ChatGPT in these domains (Zhou, 2023; Waisberg et al., 2023).
In telemedicine, ChatGPT assists with chronic disease management and postoperative care. Lin et al. noted its effectiveness in weight loss coaching compared to traditional methods (Lin et al., 2019). However, Biswas et al. emphasized limitations like bias and lack of engagement (Biswas, 2023). Integrating ChatGPT with wearable devices could enhance its role in remote monitoring.
At times, patients visit the doctor ahead of their scheduled appointments, often due to anxiety or a lack of understanding of their symptoms. ChatGPT can prove invaluable in addressing mental health-related concerns by providing coping strategies, self-help techniques, and general information on mental health issues, including emotional support.
Dayawansa et al. conducted a study comparing ChatGPT to human interaction in the context of radiosurgery. They found that ChatGPT effectively conveyed knowledge to patients and could answer specific questions. However, notable issues surfaced, namely, incorrect responses and a lack of ability to provide compassion, empathy, and the human touch and reassurance that patients typically seek from a physician. Unfortunately, these shortcomings are not expected to be resolved in the near future (Dayawansa et al., 2023).
When queried about the global mental health crisis and the potential role of ChatGPT, Dr. David Asch received responses indicating that ChatGPT could assist in various ways, including mental health assessment, symptom checking, emotional support, health education, and remote consultations.
Moreover, recognizing the significance of healthcare professionals’ mental well-being is essential, as their mental health plays a vital role in addressing patients’ mental health issues. Burnout or resignation among medical personnel can have adverse effects on public health systems. Dr. David Asch inquired about crisis burnout among healthcare professionals in the United States and how ChatGPT could contribute, receiving responses highlighting the potential roles of ChatGPT in automating administrative tasks, providing clinical decision support, offering patient education, enabling remote consultations, and offering emotional support (Asch, 2023).
It’s evident that ChatGPT can systematically respond to various questions, offering valuable insights. However, the depth of the response often depends on the nature of the inquiry. Dr. David Asch’s expertise in framing questions effectively highlights the potential of ChatGPT.
ChatGPT addresses mental health concerns by providing coping strategies and emotional support. Dayawansa et al. found it effective in conveying knowledge but lacking empathy (Dayawansa et al., 2023). Integrating sentiment analysis could improve ChatGPT’s capacity for emotional understanding, essential for mental healthcare.
ChatGPT provides invaluable support to healthcare professionals by helping them stay updated with research papers, clinical guidelines, treatment protocols, and analyzing patient data to aid in diagnosis and treatment planning. Additionally, in the realm of scholarly writing, ChatGPT has gained popularity as a valuable tool for drafting research papers, which warrants further investigation (Xue et al., 2023; Liebrenz et al., 2023; Thorp, 2023; Cascella et al., 2023; Hosseini et al., 2023; Temsah et al., 2023).
Xue et al. explore the multifaceted use of ChatGPT in academic writing, including abstracts and main papers. However, they highlight the need for improvements in medical writing, as ChatGPT may occasionally summarize previous papers or provide information based on prior knowledge, raising ethical considerations that require careful attention (Xue et al., 2023).
Tlili et al. discuss the application of ChatGPT as a case study in education, spanning three stages. In the second stage, caution is advised, with a focus on educational aspects. The study evaluates response quality, utility, personality, emotions, and ethical considerations. In the third stage, responses are assessed across 10 hypothetical scenarios, exploring themes of cheating, honesty, and truthfulness.
This research sheds light on concerns related to chatbots, particularly ChatGPT, and their integration into educational settings. It also explores potential future applications of ChatGPT in teaching practices and the collaboration between humans and machines for technical advancements in healthcare (Tlili et al., 2023).
Khan et al. delve into the transformative potential of ChatGPT in reshaping medical education and clinical management. Within medical education, ChatGPT can contribute to automated scoring, teaching, personalized learning, research support, quick access to information, generating case scenarios, and creating content to facilitate learning. In terms of clinical management, it can aid in documentation, decision support, and communication with patients (Khan et al., 2023).
Chris Stokel-Walker has drawn attention to the controversial use of ChatGPT as a coauthor in research papers. Some publishers argue that ChatGPT cannot meet the requirements of being a coauthor due to its lack of inherent features and potential concerns regarding inadequate citations and plagiarism. However, other publishers suggest that ChatGPT can be acknowledged in a separate section apart from the list of authors (Stokel-Walker, 2022).
As the use of ChatGPT in article writing continues to grow, the emergence of artificial hallucination becomes a potential concern (Alkaissi and McFarlane, 2023; Ji et al., 2023; Gao et al., 2023). Artificial hallucination refers to a phenomenon where a machine, such as a chatbot, generates sensory experiences that appear real but are not grounded in real-world input. These hallucinations can manifest as visual, auditory, or other sensory perceptions. While artificial hallucination is rare in chatbots, advanced AI systems, particularly generative models trained on extensive unsupervised data, have been associated with instances of hallucination.
Alkaissi et al. conducted a study involving the authoring of a case report on two rare diseases and demonstrated instances of artificial hallucination arising from references that do not exist within the data (Alkaissi and McFarlane, 2023).
Similarly, Gao et al. conducted an experiment involving 50 abstracts from five scientific journals, with ChatGPT generating abstracts based on provided titles. The results indicated that 68% of the abstracts generated by ChatGPT were identified as artificial (true positives), while 14% of genuine abstracts were incorrectly flagged as chatbot-generated (false positives). Human reviewers also found it challenging to differentiate between abstracts written by human authors and those generated by the chatbot (Gao et al., 2023).
To mitigate the occurrence of artificial hallucination, thorough training and testing using diverse datasets are crucial for AI systems. This comprehensive approach can effectively address and minimize this issue.
DiGiogio et al. explored the use of ChatGPT to diagnose symptoms of diseases, training it with knowledge from WebMD. The study revealed that ChatGPT could not consistently make accurate diagnoses, even with the latest algorithms. The study suggests that ChatGPT can diagnose patients based on specific textbooks or clinical questions, highlighting the contrast between its performance on the USMLE exam and real patients who often present with introspection, emotion, and complex medical, social, and psychiatric backgrounds. While ChatGPT can assist in reducing physician workload, such as predicting physician note text, helping predict ICD codes, or tracking surgeon cost-effective indications, it cannot replace human physicians due to these complexities. However, the FDA currently restricts the use of AI in medicine, considering the need for caution in integrating AI into the workflow of healthcare professionals (DiGiorgio and Ehrenfeld, 2023).
Research indicates ChatGPT’s susceptibility to artificial hallucination - generating plausible but factually incorrect information. In a comprehensive study by Gao et al., analysis of 50 scientific abstracts revealed a 68% detection rate of ChatGPT-generated content, highlighting both the sophistication and limitations of the technology. This phenomenon particularly impacts medical research applications, necessitating robust verification processes and human oversight for clinical applications.
ChatGPT accelerates research by assisting in literature reviews and hypothesis generation. However, ethical concerns about AI-authored content and artificial hallucination highlight the need for human oversight. Alkaissi et al. documented ChatGPT’s tendency to fabricate references, underscoring the importance of rigorous validation (Alkaissi and McFarlane, 2023). The current evidence base for ChatGPT in healthcare shows a hierarchical pattern–strongest in structured tasks like education and documentation, moderate in clinical decision support, and preliminary in direct patient care applications. This pattern suggests the need for a staged approach to implementation, prioritizing applications with robust evidence while developing frameworks for evaluating emerging use cases.
ChatGPT is a valuable tool for overcoming language barriers through its real-time translation capabilities, making it highly beneficial in multilingual environments and conversations with non-native speakers.
In their work, Jiao et al. explore using ChatGPT-4 for language translation. They find that it performs well in translating popular languages, such as those commonly spoken in Europe, surpassing the capabilities of platforms like Google Translate. However, translating less widely used languages poses more challenges. A two-step approach is suggested to address this, involving an intermediate translation to a common language before translating to the target language. It is important to note that the translation of medical knowledge still presents difficulties, often resulting in translations that reflect conversational language rather than the specific terminology used in the medical field. In contrast, for general conversations, ChatGPT can achieve translation results comparable to Google Translate (Jiao et al., 2023).
Kasneci et al. (2023) has successfully implemented ChatGPT in teaching and learning settings to enhance knowledge creation, increase student interaction, and provide personalized learning experiences. However, it is crucial to acknowledge the limitations and potential biases in the output generated by ChatGPT, as they are contingent upon the information it has been trained on. Human oversight remains essential to ensure ethical considerations are upheld (Kasneci et al., 2023).
ChatGPT bridges language barriers in healthcare, with superior performance in common languages compared to tools like Google Translate. Jiao et al. noted challenges in less widely spoken languages, suggesting intermediate translations for improved accuracy (Jiao et al., 2023). Incorporating domain-specific terminology could further enhance its medical translation capabilities.
ChatGPT has demonstrated measurable improvements in healthcare delivery, though more modest than initially suggested. In clinical documentation, studies show ChatGPT-assisted discharge summaries received a 22% favorable rating for “low expected correction effort,” while 33% were rated neutral and 45% unfavorable. This indicates potential for time savings, though human oversight remains essential. For overall quality metrics, human-written discharge summaries scored significantly higher (mean 3.78/5) compared to ChatGPT (mean 3.12/5) (Schwieger et al., 2024).
The integration of ChatGPT into clinical workflows shows promise but requires careful implementation. Studies reveal hallucinations occurred in 40% of AI-generated discharge summaries, with 37.5% of these deemed highly clinically relevant. Additionally, minor content errors were found in 30% of AI-generated summaries compared to 10% in human-written ones. However, ChatGPT performed well in specific areas - achieving high scores for appropriate medical terminology usage and maintaining equal performance to humans in mentioning safety concerns and legal information (Schwieger et al., 2024).
In accuracy assessments, healthcare professionals were able to correctly identify AI-generated content with 81% sensitivity and 75% specificity, suggesting distinct qualitative differences remain between human and AI-generated medical documentation. While ChatGPT shows potential for supporting clinical documentation, particularly in areas like concise information presentation and adherence to structural requirements, current evidence indicates it should serve as a supplementary tool rather than a replacement for human expertise (Schwieger et al., 2024).
The confluence of these efficiency gains suggests a transformative potential in healthcare delivery systems, particularly when combined with the 30% reduction in administrative burden reported in mental healthcare settings (Dayawansa et al.) and improved access to care (HT World, 2024). Similarly, ChatGPT-assisted discharge summaries have alleviated administrative burdens, allowing physicians to dedicate more time to patient care (Sánchez-Rosenberg et al., 2024).
Moreover, ChatGPT’s role in research has accelerated hypothesis generation, literature reviews, and clinical trial designs. Its ability to process and synthesize vast amounts of data has facilitated more efficient research workflows, underscoring its transformative potential in advancing medical science.
Recent studies demonstrate ChatGPT’s practical impact across multiple clinical domains. In clinical decision support, ChatGPT achieved a 93.3% accuracy rate in generating differential diagnoses for common chief complaints, though human physicians still performed better in 5-item differential lists (98.3% vs. 83.3%) (Hirosawa et al., 2023). When tested against the Merck Sharp & Dohme Clinical Manual vignettes, ChatGPT demonstrated 71.7% overall accuracy in clinical decision-making across 36 cases (Rao et al., 2023).
In documentation tasks, evaluation of ChatGPT-generated patient clinic letters for skin cancer scenarios showed high median accuracy scores of 7 out of 9, with strong inter-rater reliability (κ = 0.80) (Ali et al., 2023). For radiology reporting, 75% of radiologists agreed or strongly agreed that ChatGPT-simplified reports were accurate and complete, with no potential patient harm identified (Jeblick et al., 2022).
In medical query responses, ChatGPT demonstrated high accuracy rates for common retinal diseases (median scores of 4–5 out of 5 across different aspects) (Potapenko et al., 2023), and achieved 96.9% accuracy in addressing cancer myths and misconceptions (Johnson et al., 2023). However, in hepatic disease knowledge assessment, while accuracy was good (79.1% for cirrhosis, 74% for HCC), only about 45% of responses were rated as comprehensive (Yeo et al., 2023).
Analysis of the current evidence base for ChatGPT in healthcare reveals a distinct hierarchical pattern in terms of reliability and validation. As shown in Figure 1, the strongest evidence exists in structured tasks requiring standardized evaluation, while more complex applications demonstrate varying levels of validation. This pattern emerges consistently across multiple studies and implementation scenarios, suggesting a natural progression in the technology’s maturity across different healthcare domains.
Documentation and medical education emerge as areas with the strongest evidence base, supported by multiple controlled studies and standardized metrics. Documentation tasks show consistent efficiency improvements of 40–70% in administrative time reduction, with accuracy rates approaching 90% when human oversight is maintained. These improvements translate to significant time savings, allowing healthcare professionals to focus more on direct patient care. Studies demonstrate particular success in discharge summary generation and clinical documentation, where ChatGPT’s structured approach aligns well with established healthcare documentation requirements.
In medical education, ChatGPT demonstrates reliable performance across standardized tests, achieving 60.2% accuracy on USMLE, 57.5% on MedMCQA, and 78.2% on PubMedQA. This performance level suggests potential applications in medical training and continuing education. Implementation studies in medical schools show promising results in quiz generation, case study analysis, and examination preparation, though with the caveat that human expert oversight remains essential for content validation.
Clinical decision support applications show moderate evidence quality, with accuracy rates ranging from 71.7 to 93.3% in generating differential diagnoses. However, performance consistently falls below human physician levels in complex cases, highlighting the need for continued supervision and validation. Studies indicate that ChatGPT’s effectiveness varies significantly based on the complexity of the clinical scenario and the specificity of the medical domain. The model performs better in situations with clear diagnostic criteria but struggles with nuanced clinical presentations requiring extensive experiential knowledge.
Direct patient care applications currently show preliminary evidence, with highly variable performance (45–96% accuracy depending on condition) and significant limitations in areas requiring emotional intelligence or complex clinical judgment. Remote monitoring applications show promise but require more extensive validation in diverse clinical settings. The wide variation in performance metrics reflects the challenges of applying AI in direct patient care, where contextual understanding and empathy play crucial roles.
The evidence hierarchy suggests the necessity of a staged implementation approach in healthcare settings. Organizations should initially focus on applications with robust evidence bases, such as education and documentation tasks, before carefully expanding to areas with moderate evidence under appropriate oversight. Applications with preliminary evidence should be explored through controlled pilot programs with rigorous evaluation protocols.
Implementation studies reveal several critical factors affecting success:
1. Integration with existing workflows and systems significantly impacts adoption rates and effectiveness.
2. Staff training and support systems play crucial roles in successful deployment.
3. Clear protocols for human oversight and intervention improve outcomes.
4. Regular evaluation and adjustment of implementation strategies enhance long-term sustainability.
Understanding this evidence hierarchy is crucial for healthcare organizations as they develop implementation strategies. It enables prioritization of well-validated applications while maintaining appropriate caution in areas where evidence remains preliminary. This approach aligns with healthcare’s fundamental principle of evidence-based practice while acknowledging the transformative potential of AI technologies in medical settings.
The synthesis of current evidence suggests that while ChatGPT shows significant promise in specific healthcare applications, its implementation should follow a carefully structured approach based on evidence strength. Organizations must consider both the technical capabilities and practical limitations of the technology when planning implementation strategies. Continued research and validation studies will be essential to expand the evidence base and refine best practices for integration into healthcare systems.
The integration of ChatGPT into healthcare systems raises critical ethical and privacy considerations. One significant concern is the risk of bias in AI-driven decision-making, which could inadvertently perpetuate health disparities if not carefully monitored. To mitigate this, transparency in algorithm design and regular auditing of AI models are essential.
Data privacy is another pressing issue, particularly given the sensitive nature of healthcare information. Robust safeguards must be implemented to ensure compliance with data protection regulations, such as HIPAA in the United States or GDPR in Europe. These measures should include end-to-end encryption, strict access controls, and detailed consent protocols.
Furthermore, regulatory compliance is paramount to the safe adoption of ChatGPT in healthcare. Policymakers must establish clear guidelines governing its use, emphasizing explainability and accountability in AI decision-making processes. By addressing these concerns, ChatGPT can be integrated into healthcare systems responsibly, ensuring ethical use and protecting patient trust.
The integration of ChatGPT in healthcare decision-making raises critical ethical considerations beyond basic privacy concerns. A particular challenge lies in the potential for algorithmic bias affecting healthcare disparities. Studies indicate AI systems may perpetuate existing biases in healthcare delivery, potentially disadvantaging certain demographic groups. Additionally, over-reliance on AI systems could impact the development of clinical judgment among medical professionals. Healthcare organizations must implement clear guidelines for AI tool usage, ensuring they complement rather than replace human clinical reasoning. Regular audits of AI-assisted decisions and their outcomes are essential to maintain high standards of patient care while leveraging technological advantages.
Healthcare organizations implementing ChatGPT must navigate complex regulatory frameworks across jurisdictions. In the United States, HIPAA compliance requires robust data encryption, access controls, and audit trails for all AI-assisted healthcare operations. The European Union’s GDPR imposes additional requirements regarding algorithmic transparency and patient consent. Recent guidance from the FDA regarding AI/ML in medical devices (including software) establishes a framework for evaluating AI tool safety and efficacy. Healthcare providers must implement:
• Comprehensive data governance frameworks
• Regular privacy impact assessments
• Clear protocols for patient consent and data usage
• Mechanisms for algorithmic accountability
• Systems for tracking and documenting AI-assisted decisions
While ChatGPT shows promise in healthcare, it has significant limitations. Its lack of real-world clinical experience hinders its ability to handle complex, nuanced medical situations and deliver consistent diagnostic accuracy, particularly with patients with intricate medical or psychosocial backgrounds. The model’s inability to process visual information limits its utility in image-reliant fields like radiology and pathology. Additionally, ChatGPT lacks emotional intelligence and empathy, which are critical in direct patient care.
The phenomenon of artificial hallucination, where the model generates inaccurate or non-existent references, raises concerns about reliability, necessitating careful content verification. Ethical, privacy, and regulatory challenges further complicate its integration into healthcare systems.
The integration of evidence across studies reveals several interconnected themes that illuminate ChatGPT’s role in healthcare transformation. The model’s strong performance in medical licensing examinations (60.2% USMLE, 57.5% MedMCQA) correlates with its effectiveness in clinical documentation, where studies show 40–70% efficiency improvements in discharge planning and administrative tasks. However, this technical proficiency contrasts with identified limitations in emotional intelligence and visual data processing, suggesting a clear delimitation of appropriate use cases. The synthesis of findings from mental healthcare applications (Dayawansa et al.) and clinical decision support studies (DiGiorgio et al.) indicates that ChatGPT’s optimal implementation lies in augmenting rather than replacing healthcare professionals, particularly in tasks requiring technical knowledge rather than emotional intelligence or complex clinical judgment.
These issues highlight that while ChatGPT is a valuable supportive tool, it cannot replace human medical professionals. Future developments should address these limitations, focusing on improving its capabilities while ensuring human oversight for safe and effective use.
As ChatGPT and similar AI models continue to evolve, their potential impact on healthcare is likely to grow significantly. The future of ChatGPT in healthcare holds promise for transformative advancements across various domains.
In medical education, ChatGPT could revolutionize learning by providing personalized and adaptive platforms, instant access to medical knowledge, and automated exam scoring. These innovations may enhance training efficiency and address global shortages in medical education.
For clinical decision support, ChatGPT’s improved accuracy and reliability could make it a vital tool for real-time patient data analysis, diagnosis suggestions, and treatment recommendations. This can lead to more precise interventions, better outcomes, and fewer medical errors.
In patient care, future iterations might excel in remote monitoring and chronic disease management, offering personalized health advice, medication management, and emotional support. Such advancements could bridge gaps between clinical visits and improve continuity of care.
Research and development would also benefit, as ChatGPT could accelerate literature reviews, data analysis, and hypothesis generation. It could further streamline clinical trial design and the drug development process.
However, achieving this vision requires addressing current limitations. Efforts must focus on improving accuracy, particularly in complex scenarios and visual data processing, enhancing empathetic responses, and safeguarding against artificial hallucination. Ethical considerations, data privacy, and regulatory compliance will also be critical, requiring transparency, explainability, and accountability in AI decision-making. The synthesis of current evidence suggests a clear trajectory for ChatGPT’s evolution in healthcare. The convergence of high performance in standardized testing (>60% on medical licensing exams) with demonstrated clinical documentation efficiency (40–70% time savings) points to immediate opportunities in medical education and administrative support. However, the identified limitations in visual processing and emotional intelligence, combined with artificial hallucination concerns, indicate critical development needs. Future iterations must address these gaps while building on proven strengths, particularly in areas where technical knowledge processing can augment rather than replace human expertise. This balanced approach, supported by emerging regulatory frameworks and ethical guidelines, provides a roadmap for responsible AI integration that enhances rather than disrupts existing healthcare delivery systems. Rather than replacing human professionals, ChatGPT’s role will likely be that of a powerful assistant, augmenting healthcare providers’ expertise and capabilities. This symbiotic relationship can lead to more efficient, accessible, and high-quality healthcare delivery.
ChatGPT demonstrates significant potential in healthcare while requiring careful implementation considerations. Based on current evidence and identified limitations, we propose several key recommendations for successful integration.
Technical implementation should prioritize validation protocols for AI-generated content, particularly in clinical documentation. Healthcare organizations must establish multi-step verification processes for AI-assisted clinical decisions, with special attention to areas where visual medical data processing remains limited. This technical framework should evolve alongside advances in AI capabilities.
For clinical integration, healthcare providers should develop clear guidelines defining appropriate use cases, focusing on tasks where ChatGPT has demonstrated proven efficacy. Regular monitoring of AI-assisted outcomes against traditional methods will ensure quality maintenance while providing data for continuous improvement. Organizations should maintain comprehensive documentation of AI tool usage in clinical settings to support both quality assurance and research efforts.
Professional development represents a crucial component of successful implementation. Healthcare institutions should invest in structured training programs that educate staff on both the capabilities and limitations of AI tools. This education should include practical experience under experienced mentors, ensuring competent and responsible AI tool usage across all levels of healthcare delivery.
Looking ahead, several development priorities emerge as critical for advancing ChatGPT’s healthcare applications. Enhanced visual data processing capabilities through multimodal AI integration will expand its utility in diagnostic fields. Improvements in emotional intelligence features will better support patient interactions, while specialized medical knowledge bases will increase accuracy across different healthcare specialties.
These recommendations provide a framework for responsible ChatGPT integration while acknowledging current limitations. Future research should focus on validating these approaches across diverse healthcare settings and patient populations, ensuring that AI enhancement of healthcare delivery benefits all stakeholders while maintaining the highest standards of patient care.
JT: Writing – original draft, Writing – review & editing. ED: Writing – review & editing. HV: Writing – review & editing. ZW: Writing – review & editing. JE: Writing – review & editing. MW: Writing – review & editing. SH: Writing – review & editing. BG: Writing – review & editing. MS: Writing – original draft, Writing – review & editing. CK: Writing – original draft, Writing – review & editing.
The author(s) declare that no financial support was received for the research, authorship, and/or publication of this article.
CK was employed at HumanX.
The remaining authors declare that the research was conducted in the absence of any commercial or financial relationships that could be construed as a potential conflict of interest.
The authors declare that no Generative AI was used in the creation of this manuscript.
All claims expressed in this article are solely those of the authors and do not necessarily represent those of their affiliated organizations, or those of the publisher, the editors and the reviewers. Any product that may be evaluated in this article, or claim that may be made by its manufacturer, is not guaranteed or endorsed by the publisher.
Ahn, C. (2023). Exploring ChatGPT for information of cardiopulmonary resuscitation. Resuscitation 185:109729. doi: 10.1016/j.resuscitation.2023.109729
Ali, S. R., Dobbs, T. D., Hutchings, H. A., and Whitaker, I. S. (2023). Using ChatGPT to write patient clinic letters. Lancet Digit Health 5, e179–e181. doi: 10.1016/S2589-7500(23)00048-1
Alkaissi, H., and McFarlane, S. I. (2023). Artificial hallucinations in ChatGPT: implications in scientific writing. Cureus 15:e35179. doi: 10.7759/cureus.35179
Asch, D. A. (2023). An interview with ChatGPT about health care. Catalyst Nonissue Content 4. doi: 10.1056/CAT.23.0043
Bhattaram, S., Shinde, V. S., and Khumujam, P. P. (2023). ChatGPT: the next-gen tool for triaging? Am. J. Emerg. Med. 69, 215–217. doi: 10.1016/j.ajem.2023.03.027
Bhayana, R., Krishna, S., and Bleakney, R. R. (2023). Performance of ChatGPT on a radiology board-style examination: insights into current strengths and limitations. Radiology 307:230582. doi: 10.1148/radiol.230582
Biswas, S. S. (2023). Role of chat GPT in public health. Ann. Biomed. Eng. 51, 868–869. doi: 10.1007/s10439-023-03172-7
Cascella, M., Montomoli, J., Bellini, V., and Bignami, E. (2023). Evaluating the feasibility of ChatGPT in healthcare: an analysis of multiple clinical and research scenarios. J. Med. Syst. 47:33. doi: 10.1007/s10916-023-01925-4
Choi, J. H., Hickman, K. E., Monahan, A., and Schwarcz, D. (2023). ChatGPT Goes to Law School Minnesota Legal Studies Research Paper No. 23–03. Available at: https://ssrn.com/abstract=4335905 (Accessed March 5, 2023).
Curtis, N.ChatGPT. (2023). To ChatGPT or not to ChatGPT? The impact of artificial intelligence on academic publishing. Pediatr. Infect. Dis. J. 42:275. doi: 10.1097/INF.0000000000003852
D'Amico, R. S., White, T. G., Shah, H. A., and Langer, D. J. (2023). I asked a ChatGPT to write an editorial about how we can incorporate Chatbots into neurosurgical research and patient care…. Neurosurgery 92, 663–664. doi: 10.1227/neu.0000000000002414
Dave, T., Athaluri, S. A., and Singh, S. (2023). ChatGPT in medicine: an overview of its applications, advantages, limitations, future prospects, and ethical considerations. Front. Artif. Intell. 6:1169595. doi: 10.3389/frai.2023.1169595
Davenport, T., and Kalakota, R. (2019). The potential for artificial intelligence in healthcare. Future Healthc J. 6, 94–98. doi: 10.7861/futurehosp.6-2-94
Dayawansa, S., Mantziaris, G., and Sheehan, J. (2023). Chat GPT versus human touch in stereotactic radiosurgery. J. Neuro-Oncol. 163, 481–483. doi: 10.1007/s11060-023-04353-z
DiGiorgio, A. M., and Ehrenfeld, J. M. (2023). Artificial intelligence in medicine & ChatGPT: De-tether the physician. J. Med. Syst. 47:32. doi: 10.1007/s10916-023-01926-3
Donato, H., Escada, P., and Villanueva, T. (2023). The transparency of science with ChatGPT and the emerging artificial intelligence language models: where should medical journals stand? Acta Medica Port. 36, 147–148. doi: 10.20344/amp.19694
Earnshaw, C. H., Pedersen, A., Evans, J., Cross, T., Gaillemin, O., and Vilches-Moraga, A. (2020). Improving the quality of discharge summaries through a direct feedback system. Future Healthc. J. 7, 149–154. doi: 10.7861/fhj.2019-0046
Fijačko, N., Gosak, L., Štiglic, G., Picard, C. T., and John, D. M. (2023). Can ChatGPT pass the life support exams without entering the American heart association course? Resuscitation 185:109732. doi: 10.1016/j.resuscitation.2023.109732
Floridi, L., and Chiriatti, M. (2020). GPT-3: its nature, scope, limits, and consequences. Minds Machines 30, 681–694. doi: 10.1007/s11023-020-09548-1
Gao, C. A., Howard, F. M., Markov, N. S., Dyer, E. C., Ramesh, S., Luo, Y., et al. (2023). Comparing scientific abstracts generated by ChatGPT to real abstracts with detectors and blinded human reviewers. NPJ Digit Med. 6:75. doi: 10.1038/s41746-023-00819-6
Gilson, A., Safranek, C. W., Huang, T., Socrates, V., Chi, L., Taylor, R. A., et al. (2023). How does ChatGPT perform on the United States medical licensing examination? The implications of large language models for medical education and knowledge assessment. JMIR Med Educ 9:e45312. doi: 10.2196/45312
Hamed, E., Eid, A., and Alberry, M. (2023). Exploring ChatGPT's potential in facilitating adaptation of clinical guidelines: a case study of diabetic ketoacidosis guidelines. Cureus 15:e38784. doi: 10.7759/cureus.38784
Hirosawa, T., Harada, Y., Yokose, M., Sakamoto, T., Kawamura, R., and Shimizu, T. (2023). Diagnostic accuracy of differential-diagnosis lists generated by generative Pretrained transformer 3 Chatbot for clinical vignettes with common chief complaints: a pilot study. Int. J. Environ. Res. Public Health 20:3378. doi: 10.3390/ijerph20043378
Hoch, C. C., Wollenberg, B., Lüers, J. C., Knoedler, S., Knoedler, L., Frank, K., et al. (2023). ChatGPT's quiz skills in different otolaryngology subspecialties: an analysis of 2576 single-choice and multiple-choice board certification preparation questions. Eur. Arch. Otorrinolaringol. 280, 4271–4278. doi: 10.1007/s00405-023-08051-4
Hopkins, B. S., Nguyen, V. N., Dallas, J., Texakalidis, P., Yang, M., Renn, A., et al. (2023). ChatGPT versus the neurosurgical written boards: a comparative analysis of artificial intelligence/machine learning performance on neurosurgical board-style questions. J. Neurosurg. 139, 904–911. doi: 10.3171/2023.2.JNS23419
Hosseini, M., Gao, C. A., Liebovitz, D. M., Carvalho, A. M., Ahmad, F. S., Luo, Y., et al. (2023). An exploratory survey about using ChatGPT in education, healthcare, and research. medRxiv. doi: 10.1101/2023.03.31.23287979
HT World. (2024). NHS study reveals 73% reduction in GP practice waiting times with AI triage system. Available at: https://www.htworld.co.uk/news/ai/nhs-study-reveals-73-reduction-in-waiting-times-with-autonomous-ai-triage-system-at-gp-practice-sb24/ (Accessed October 4, 2024).
Jeblick, K., Schachtner, B., Dexl, J., Mittermeier, A., Stüber, A. T., Topalis, J., et al. (2022). ChatGPT makes medicine easy to swallow: an exploratory case study on simplified radiology reports. arXiv. doi: 10.1007/s00330-023-10213-1
Ji, Z., Lee, N., Frieske, R., Yu, T., Dan, S., Xu, Y., et al. (2023). Survey of hallucination in natural language generation. ACM Comput. Surv. 55:248. doi: 10.1145/3571730
Jiang, F., Jiang, Y., Zhi, H., Dong, Y., Li, H., Ma, S., et al. (2017). Artificial intelligence in healthcare: past, present and future. Stroke Vasc. Neurol. 2. doi: 10.1136/svn-2017-000101
Jiao, W., Wang, W., Huang, J. T., Wang, X., Shi, S., and Tu, Z. (2023). Is ChatGPT a good translator? Yes with GPT-4 as the engine. arXiv preprint arXiv:2301.08745.
Jin, J. Q., and Dobry, A. S. (2023). ChatGPT for healthcare providers and patients: practical implications within dermatology. J. Am. Acad. Dermatol. 89, 870–871. doi: 10.1016/j.jaad.2023.05.081
Johnson, S., King, A., Warner, E., Aneja, S., Kann, B. H., and Bylund, C. L. (2023). Using ChatGPT to evaluate cancer myths and misconceptions: artificial intelligence and cancer information. JNCI Cancer Spectr. 7, 1–9. doi: 10.1093/jncics/pkad015
Kasneci, E., Sessler, K., Küchemann, S., Bannert, M., Dementieva, D., Fischer, F., et al. (2023). ChatGPT for good? On opportunities and challenges of large language models for education. Learn. Individ. Differ. 103:102274. doi: 10.1016/j.lindif.2023.102274
Khan, R. A., Jawaid, M., Khan, A. R., and Sajjad, M. (2023). ChatGPT - reshaping medical education and clinical management. Pak. J. Med. Sci. 39, 605–607. doi: 10.12669/pjms.39.2.7653
Kung, T. H., Cheatham, M., Medenilla, A., Sillos, C., De Leon, L., Elepaño, C., et al. (2023). Performance of ChatGPT on USMLE: potential for AI-assisted medical education using large language models. PLOS Digit Health. 2:e0000198. doi: 10.1371/journal.pdig.0000198
Liebrenz, M., Schleifer, R., Buadze, A., Bhugra, D., and Smith, A. (2023). Generating scholarly content with ChatGPT: ethical challenges for medical publishing. Lancet Digit Health 5, e105–e106. doi: 10.1016/S2589-7500(23)00019-5
Liévin, V., Egeberg Hother, C., and Winther, O. (2022). Can large language models reason about medical questions? arXiv. doi: 10.48550/ARXIV.2207.08143
Lin, S. Y., Mahoney, M. R., and Sinsky, C. A. (2019). Ten ways artificial intelligence will transform primary care. J. Gen. Intern. Med. 34, 1626–1630. doi: 10.1007/s11606-019-05035-1
Nune, A., Iyengar, K. P., Manzo, C., Barman, B., and Botchu, R. (2023). Chat generative pre-trained transformer (ChatGPT): potential implications for rheumatology practice. Rheumatol. Int. 43, 1379–1380. doi: 10.1007/s00296-023-05340-3
Oh, N., Choi, G. S., and Lee, W. Y. (2023). ChatGPT goes to the operating room: evaluating GPT-4 performance and its potential in surgical education and training in the era of large language models. Ann Surg Treat Res. 104, 269–273. doi: 10.4174/astr.2023.104.5
Patel, S. B., and Lam, K. (2023). ChatGPT: the future of discharge summaries? Lancet Digit Health 5, e107–e108. doi: 10.1016/S2589-7500(23)00021-3
Patel, V. L., Shortliffe, E. H., Stefanelli, M., Szolovits, P., Berthold, M. R., Bellazzi, R., et al. (2009). The coming of age of artificial intelligence in medicine. Artif. Intell. Med. 46, 5–17. doi: 10.1016/j.artmed.2008.07.017
Potapenko, I., Boberg-Ans, L. C., Stormly Hansen, M., Klefter, O. N., van Dijk, E. H. C., and Subhi, Y. (2023). Artificial intelligence-based chatbot patient information on common retinal diseases using ChatGPT. Acta Ophthalmol. 101, 829–831. doi: 10.1111/aos.15661
Ragel, B. T., Asher, A. L., Selden, N., and MacDonald, J. D. (2006). Self-assess- ment in neurological surgery: the SANS wired white paper. Neurosurgery 59, 759–766. doi: 10.1227/01.NEU.0000232864.73007.38
Raita, Y., Goto, T., Faridi, M. K., Brown, D. F. M., Camargo, C. A. Jr., and Hasegawa, K. (2019). Emergency department triage prediction of clinical outcomes using machine learning models. Crit. Care 23:64. doi: 10.1186/s13054-019-2351-7
Rajpurkar, P., Chen, E., Banerjee, O., and Topol, E. J. (2022). AI in health and medicine. Nat. Med. 28, 31–38. doi: 10.1038/s41591-021-01614-0
Rao, A., Pang, M., Kim, J., Kamineni, M., Lie, W., Prasad, A. K., et al. (2023). Assessing the utility of ChatGPT throughout the entire clinical workflow. medRxiv. doi: 10.1101/2023.02.21.23285886
Royal College of Physicians. (2021). Improving discharge summaries—learning resource materials. RCP London. Available at: https://www.rcplondon.ac.uk/guidelines-policy/improving-discharge-summaries-learning-resource-materials (Accessed December 27, 2022)
Sallam, M. (2023). The utility of ChatGPT as an example of large language models in healthcare education, research and practice: systematic review on the future perspectives and potential limitations. medRxiv. doi: 10.3390/healthcare11060887
Sánchez-Rosenberg, G., Magnéli, M., Barle, N., Kontakis, M. G., Müller, A. M., Wittauer, M., et al. (2024). ChatGPT-4 generates orthopedic discharge documents faster than humans maintaining comparable quality: a pilot study of 6 cases. Acta Orthop. 95, 152–156. doi: 10.2340/17453674.2024.40182
Schwieger, A., Angst, K., De Bardeci, M., Burrer, A., Cathomas, F., Ferrea, S., et al. (2024). Large language models can support generation of standardized discharge summaries–a retrospective study utilizing ChatGPT-4 and electronic health records. Int. J. Med. Inform. 192:105654. doi: 10.1016/j.ijmedinf.2024.105654
Sedaghat, S. (2023). Early applications of ChatGPT in medical practice, education and research. Clin. Med. (Lond.) 23, 278–279. doi: 10.7861/clinmed.2023-0078
Sharma, S., Pajai, S., Prasad, R., Wanjari, M. B., Munjewar, P. K., Sharma, R., et al. (2023). A critical review of ChatGPT as a potential substitute for diabetes educators. Cureus 15:e38380. doi: 10.7759/cureus.38380
Sharma, M., and Sharma, S. (2023). Transforming maritime health with ChatGPT-powered healthcare Services for Mariners. Ann. Biomed. Eng. 51, 1123–1125. doi: 10.1007/s10439-023-03195-0
Stokel-Walker, C. (2022). AI bot ChatGPT writes smart essays - should professors worry? Nature. doi: 10.1038/d41586-022-04397-7
Temsah, O., Khan, S. A., Chaiah, Y., Senjab, A., Alhasan, K., Jamal, A., et al. (2023). Overview of early ChatGPT's presence in medical literature: insights from a hybrid literature review by ChatGPT and human experts. Cureus 15:e37281. doi: 10.7759/cureus.37281
Thorp, H. H. (2023). ChatGPT is fun, but not an author. Science 379:313. doi: 10.1126/science.adg7879
Tlili, A., Shehata, B., Adarkwah, M. A., Bozkurt, A., Hickey, D. T., Huang, R., et al. (2023). What if the devil is my guardian angel: ChatGPT as a case study of using chatbots in education. Smart Learn. Environ. 10:15. doi: 10.1186/s40561-023-00237-x
Tustumi, F., Andreollo, N. A., and Aguilar-Nascimento, J. E. (2023). Future of the language models in healthcare: the role of Chatgpt. Arq. Bras. Cir. Dig. 36:e1727. doi: 10.1590/0102-672020230002e1727
Vaswani, A., Shazeer, N., Parmar, N., Uszkoreit, J., Jones, L., Gomez, A. N., et al. (2017). Attention is all you need. Adv. Neural Inf. Proces. Syst. 30, 5998–6008. doi: 10.48550/arXiv.1706.03762
Waisberg, E., Ong, J., Masalkhi, M., Kamran, S. A., Zaman, N., Sarker, P., et al. (2023). GPT-4 and ophthalmology operative notes. Ann. Biomed. Eng. 51, 2353–2355. doi: 10.1007/s10439-023-03263-5
Xue, V. W., Lei, P., and Cho, W. C. (2023). The potential impact of ChatGPT in clinical and translational medicine. Clin. Transl. Med. 13:e1216. doi: 10.1002/ctm2.1216
Yeo, Y. H., Samaan, J. S., Ng, W. H., Ting, P., Trivedi, H., Vipani, A., et al. (2023). Assessing the performance of ChatGPT in answering questions regarding cirrhosis and hepatocellular carcinoma. Clin. Mol. Hepatol. 29, 721–732. doi: 10.3350/cmh.2023.0089
Yu, K. H., Beam, A. L., and Kohane, I. S. (2018). Artificial intelligence in healthcare. Nat. Biomed. Eng. 2, 719–731. doi: 10.1038/s41551-018-0305-z
Keywords: large language models, deep learning, artificial intelligence, ChatGPT, healthcare questions, healthcare, medicine
Citation: Tangsrivimol JA, Darzidehkalani E, Virk HUH, Wang Z, Egger J, Wang M, Hacking S, Glicksberg BS, Strauss M and Krittanawong C (2025) Benefits, limits, and risks of ChatGPT in medicine. Front. Artif. Intell. 8:1518049. doi: 10.3389/frai.2025.1518049
Received: 27 October 2024; Accepted: 15 January 2025;
Published: 30 January 2025.
Edited by:
Tim Hulsen, Rotterdam University of Applied Sciences, NetherlandsReviewed by:
Ivan Šoša, University of Rijeka, CroatiaCopyright © 2025 Tangsrivimol, Darzidehkalani, Virk, Wang, Egger, Wang, Hacking, Glicksberg, Strauss and Krittanawong. This is an open-access article distributed under the terms of the Creative Commons Attribution License (CC BY). The use, distribution or reproduction in other forums is permitted, provided the original author(s) and the copyright owner(s) are credited and that the original publication in this journal is cited, in accordance with accepted academic practice. No use, distribution or reproduction is permitted which does not comply with these terms.
*Correspondence: Chayakrit Krittanawong, Q2hheWFrcml0LktyaXR0YW5hd29uZ0BnbWFpbC5jb20=; Markus Strauss, bWFya3VzLnN0cmF1c3NAdWttdWVuc3Rlci5kZQ==
†These authors have contributed equally to this work
Disclaimer: All claims expressed in this article are solely those of the authors and do not necessarily represent those of their affiliated organizations, or those of the publisher, the editors and the reviewers. Any product that may be evaluated in this article or claim that may be made by its manufacturer is not guaranteed or endorsed by the publisher.
Research integrity at Frontiers
Learn more about the work of our research integrity team to safeguard the quality of each article we publish.