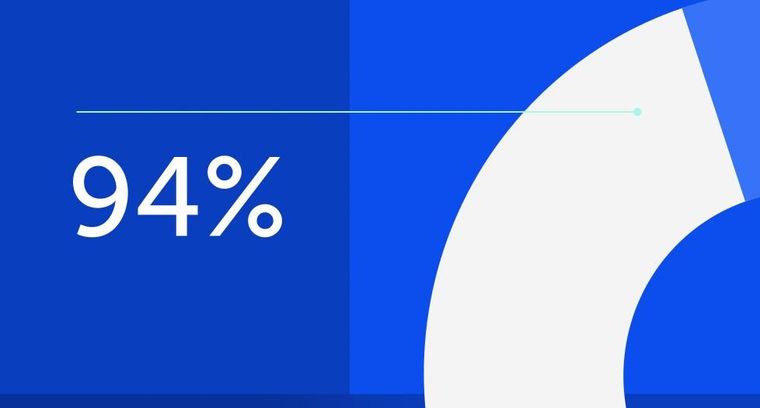
94% of researchers rate our articles as excellent or good
Learn more about the work of our research integrity team to safeguard the quality of each article we publish.
Find out more
ORIGINAL RESEARCH article
Front. Artif. Intell.
Sec. Machine Learning and Artificial Intelligence
Volume 8 - 2025 | doi: 10.3389/frai.2025.1507922
The final, formatted version of the article will be published soon.
Select one of your emails
You have multiple emails registered with Frontiers:
Notify me on publication
Please enter your email address:
If you already have an account, please login
You don't have a Frontiers account ? You can register here
Autism Spectrum Disorder (ASD) is a multifaceted neurodevelopmental condition that challenges early diagnosis due to its diverse manifestations across different developmental stages. Timely and accurate detection is essential to enable interventions that significantly enhance developmental outcomes. This study introduces a robust and interpretable machine learning framework to diagnose ASD using questionnaire data. The proposed framework leverages a stacked ensemble model, combining Random Forest (RF), Extra Tree (ET), and CatBoost (CB) as base classifiers, with an Artificial Neural Network (ANN) serving as the meta-classifier. The methodology addresses class imbalance using Safe-Level SMOTE, dimensionality reduction via Principal Component Analysis (PCA), and feature selection using Mutual Information and Pearson correlation. Evaluation on publicly available datasets representing toddlers, children, adolescents, adults, and a merged dataset (Combining children, adolescents, and adults dataset)demonstrates high diagnostic accuracy, achieving 99.86%, 99.68%, 98.17%, 99.89%, and 96.96%, respectively. Comparative analysis with standard machine learning models underscores the superior performance of the proposed framework. SHapley Additive exPlanations (SHAP) were used to interpret feature importance, while Monte Carlo Dropout (MCD) quantified uncertainty in predictions. This framework provides a scalable, interpretable, and reliable solution for ASD screening across diverse populations and developmental stages.
Keywords: Autism Spectrum Disorder, Ensemble model, uncertainty analysis, Explainable AI, Monte Carlo dropout, SHAP Scalable, Interpretable Ensemble Model for Early Autism Diagnosis
Received: 08 Oct 2024; Accepted: 21 Apr 2025.
Copyright: © 2025 Mumenin, Rahman, Yousuf, Noori and Uddin. This is an open-access article distributed under the terms of the Creative Commons Attribution License (CC BY). The use, distribution or reproduction in other forums is permitted, provided the original author(s) or licensor are credited and that the original publication in this journal is cited, in accordance with accepted academic practice. No use, distribution or reproduction is permitted which does not comply with these terms.
* Correspondence: Farzan M. Noori, University of Oslo, Oslo, Norway
Disclaimer: All claims expressed in this article are solely those of the authors and do not necessarily represent those of their affiliated organizations, or those of the publisher, the editors and the reviewers. Any product that may be evaluated in this article or claim that may be made by its manufacturer is not guaranteed or endorsed by the publisher.
Supplementary Material
Research integrity at Frontiers
Learn more about the work of our research integrity team to safeguard the quality of each article we publish.