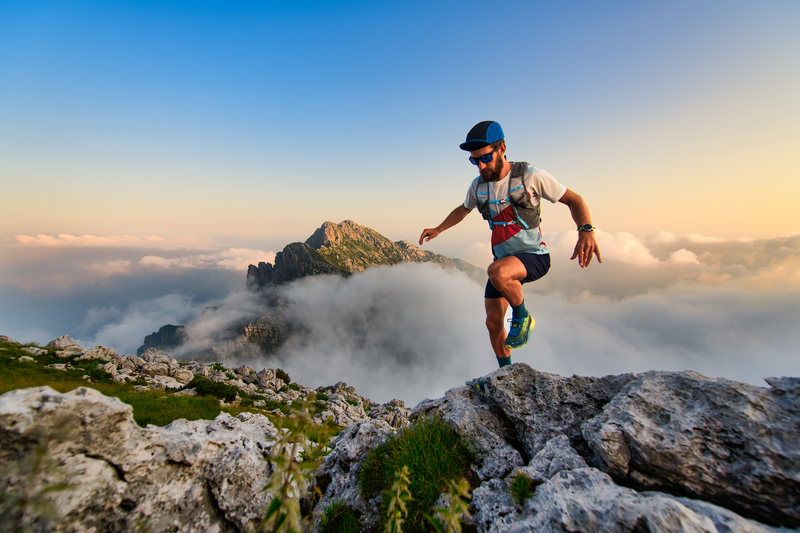
94% of researchers rate our articles as excellent or good
Learn more about the work of our research integrity team to safeguard the quality of each article we publish.
Find out more
ORIGINAL RESEARCH article
Front. Artif. Intell.
Sec. Machine Learning and Artificial Intelligence
Volume 8 - 2025 | doi: 10.3389/frai.2025.1474017
This article is part of the Research Topic Disinformation Countermeasures and Artificial Intelligence View all 7 articles
The final, formatted version of the article will be published soon.
You have multiple emails registered with Frontiers:
Please enter your email address:
If you already have an account, please login
You don't have a Frontiers account ? You can register here
This paper proposes enhancing social communication management with a behavioral economics approach through artificial intelligence instruments. The research aims to explore the influence of social communication on citizens' behavior using large language model services and assess its effectiveness. The paper builds on Daniel Kahneman's dual-process theory, highlighting the intuitive system (System 1) and the rational system (System 2) in decision-making. The author introduces a third system, System 3, representing rooted in identity socially conditioned behavior influenced by societal norms and self-awareness. On this theoretical basis, the paper emphasizes automating communication management through large language model services, freeing up citizens' potential for self-determination and self-organization. By leveraging these services, messages can be crafted to support social transformation while respecting historical, cultural, and political contexts. Based on the preconditions and restrictions described above, we create an agent based on the GPT-4 model from OpenAI to generate messages based on these narratives. The experiment will use an observational study design with virtual persons. To compare the impact of original and modified messages according to the addressee's mentality, we used the Claude 3.5 Sonnet service. We can see that the potential activity of respondents after perceiving the changed message does not change much, and the original message is perceived. Modifying messages by LLM services crafted to support social transformation while respecting historical, cultural, and political contexts cause attitudes to become substantially more negative (2.5 units downward shift in median); the intentions showed a slight positive increase (0.2 units upward change in median).
Keywords: dual-process theory, Management of Social Communications, LLM services, Behavioral Economics, decision-making
Received: 31 Jul 2024; Accepted: 28 Feb 2025.
Copyright: © 2025 Dyachenko, Humenna, Soloviov, Skarga-Bandurova and Nenkov. This is an open-access article distributed under the terms of the Creative Commons Attribution License (CC BY). The use, distribution or reproduction in other forums is permitted, provided the original author(s) or licensor are credited and that the original publication in this journal is cited, in accordance with accepted academic practice. No use, distribution or reproduction is permitted which does not comply with these terms.
* Correspondence:
Yuriy Dyachenko, Kyiv School of Economics, Kyiv, Ukraine
Disclaimer: All claims expressed in this article are solely those of the authors and do not necessarily represent those of their affiliated organizations, or those of the publisher, the editors and the reviewers. Any product that may be evaluated in this article or claim that may be made by its manufacturer is not guaranteed or endorsed by the publisher.
Research integrity at Frontiers
Learn more about the work of our research integrity team to safeguard the quality of each article we publish.