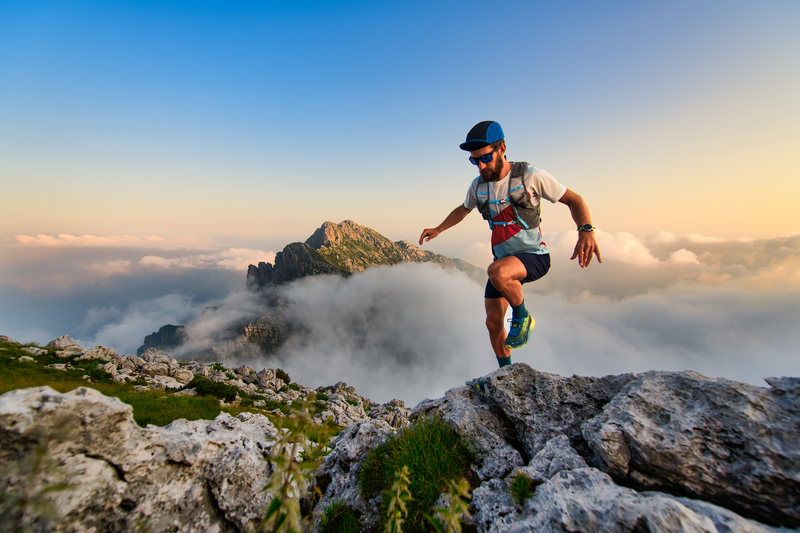
94% of researchers rate our articles as excellent or good
Learn more about the work of our research integrity team to safeguard the quality of each article we publish.
Find out more
ORIGINAL RESEARCH article
Front. Artif. Intell.
Sec. Pattern Recognition
Volume 8 - 2025 | doi: 10.3389/frai.2025.1466643
This article is part of the Research Topic Explainable, Trustworthy, and Responsible AI in Image Processing View all 5 articles
The final, formatted version of the article will be published soon.
You have multiple emails registered with Frontiers:
Please enter your email address:
If you already have an account, please login
You don't have a Frontiers account ? You can register here
The anatomically constrained registration network (AC-RegNet), which yields anatomically plausible results, has emerged as the state-of-the-art registration architecture for chest X-ray (CXR) images. Nevertheless, accurate lung field registration results may be more favored and exciting than the registration results of the entire CXR images and hold promise for dynamic lung field analysis in clinical practice.Objective: Based on the above, a registration model of the dynamic lung field of CXR images based on AC-RegNet and static CXR images is urgently developed to register these dynamic lung fields for clinical quantitative analysis.Methods: This paper proposes a fully automatic three-stage registration pipeline for the dynamic lung field of CXR images. First, the dynamic lung field mask images are generated from a pre-trained standard lung field segmentation model with the dynamic CXR images. Then, a lung field abstraction model is designed to generate the dynamic lung field images based on the dynamic lung field mask images and their corresponding CXR images. Finally, we propose a three-step registration training method to train the AC-RegNet, obtaining the registration network of the dynamic lung field images (AC-RegNet_V3). The proposed AC-RegNet_V3 with the four basic segmentation networks achieve the mean dice similarity coefficient (DSC) of 0.991, 0.993, 0.993, and 0.993, mean Hausdorff distance (HD) of 12.512, 12.813, 12.449, and 13.661, mean average symmetric surface distance (ASSD) of 0.654, 0.550, 0.572, and 0.564, and mean squared distance (MSD) of 559.098, 577.797, 548.189, and 559.652, respectively. Besides, compared to the dynamic CXR images, the mean DSC of these four basic segmentation networks with AC-RegNet has been significantly improved by 7.2%, 7.4%, 7.4%, and 7.4% (p-value<0.0001). Meanwhile, the mean HD has been significantly improved by 8. 994, 8.693, 9.057, and 7.845 (p-value<0.0001). Similarly, the mean ASSD has significantly improved by 4.576, 4.680, 4.658, and 4.658 (p-value<0.0001). Last, the mean MSD has significantly improved by 508.936, 519.776, 517.904, and 520.626 (p-value <0.0001).Our proposed three-stage registration pipeline has demonstrated its effectiveness in dynamic lung field registration. Therefore, it could become a powerful tool for dynamic lung field analysis in clinical practice, such as pulmonary airflow detection and air trapping location.
Keywords: dynamic chest X-ray images, Lung field segmentation, Medical image registration, Anatomical constraints, Convolutional Neural Network, AC-RegNet
Received: 18 Jul 2024; Accepted: 21 Feb 2025.
Copyright: © 2025 Yang, Zheng, Guo, Gao, Guo, Chen, Liu, Wu, Ouyang, Chen and Kang. This is an open-access article distributed under the terms of the Creative Commons Attribution License (CC BY). The use, distribution or reproduction in other forums is permitted, provided the original author(s) or licensor are credited and that the original publication in this journal is cited, in accordance with accepted academic practice. No use, distribution or reproduction is permitted which does not comply with these terms.
* Correspondence:
Yingwei Guo, Schol of Electrical and Information Engineering, Northeast Petroleum University, Daqing, China
Huai Chen, Department of Radiology, The Second Affiliated Hospital of Guangzhou Medical University, Guangzhou, 510260, Guangdong Province, China
Yan Kang, College of Health Science and Environmental Engineering, Shenzhen Technology University, Shenzhen, 518118, Guangdong, China
Disclaimer: All claims expressed in this article are solely those of the authors and do not necessarily represent those of their affiliated organizations, or those of the publisher, the editors and the reviewers. Any product that may be evaluated in this article or claim that may be made by its manufacturer is not guaranteed or endorsed by the publisher.
Research integrity at Frontiers
Learn more about the work of our research integrity team to safeguard the quality of each article we publish.