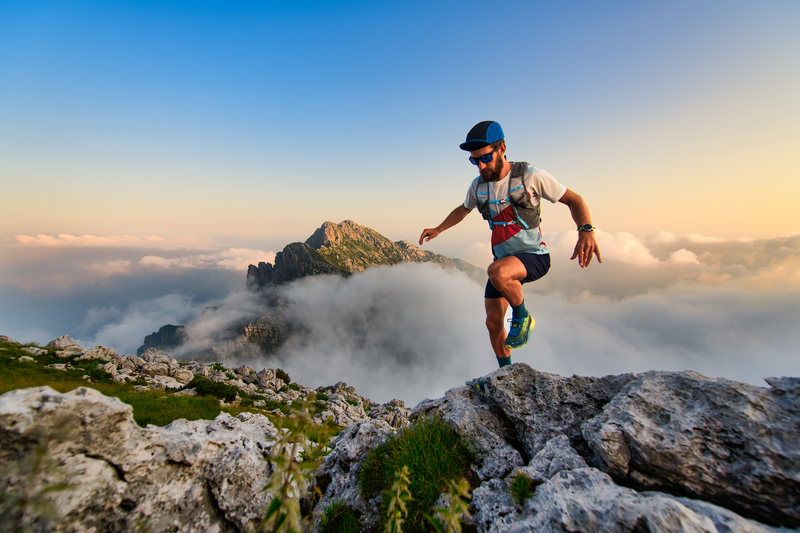
94% of researchers rate our articles as excellent or good
Learn more about the work of our research integrity team to safeguard the quality of each article we publish.
Find out more
ORIGINAL RESEARCH article
Front. Artif. Intell.
Sec. AI for Human Learning and Behavior Change
Volume 8 - 2025 | doi: 10.3389/frai.2025.1449176
The final, formatted version of the article will be published soon.
You have multiple emails registered with Frontiers:
Please enter your email address:
If you already have an account, please login
You don't have a Frontiers account ? You can register here
Students' mental health has received increased attention in recent years: reports of worsened mental health among higher education students call for new ways to support them in their college years. Educational demands are among the concerns that students report, such as the stress of academic performance, the stress related to examinations and the pressure to succeed. One aspect often present in higher education is group work. Group work can be truly beneficial for learning, but it often causes additional stress to students. The present research contributes to the design of a peer assessment tool to support students during group work. In this tool, each student is asked to rate their teammates on several aspects of group work, and a virtual agent delivers support statements in response to such ratings. For the support statements to be appropriate, the virtual agent should adapt them to the recipient and the group work situation they are experiencing. We investigate the adaptation of emotional support statements to the student's personality trait of Conscientiousness and the score assigned to a teammate on one aspect of teamwork, Productivity. The resulting algorithm is then combined with related work on Emotional Stability, and a final algorithm considering both dimensions is created.
Keywords: Emotional support, groups, Personalization, Collaborative Learning, Personality
Received: 14 Jun 2024; Accepted: 06 Mar 2025.
Copyright: © 2025 Saccardi and Masthoff. This is an open-access article distributed under the terms of the Creative Commons Attribution License (CC BY). The use, distribution or reproduction in other forums is permitted, provided the original author(s) or licensor are credited and that the original publication in this journal is cited, in accordance with accepted academic practice. No use, distribution or reproduction is permitted which does not comply with these terms.
* Correspondence:
Isabella Saccardi, Utrecht University, Utrecht, Netherlands
Judith Masthoff, Utrecht University, Utrecht, Netherlands
Disclaimer: All claims expressed in this article are solely those of the authors and do not necessarily represent those of their affiliated organizations, or those of the publisher, the editors and the reviewers. Any product that may be evaluated in this article or claim that may be made by its manufacturer is not guaranteed or endorsed by the publisher.
Research integrity at Frontiers
Learn more about the work of our research integrity team to safeguard the quality of each article we publish.