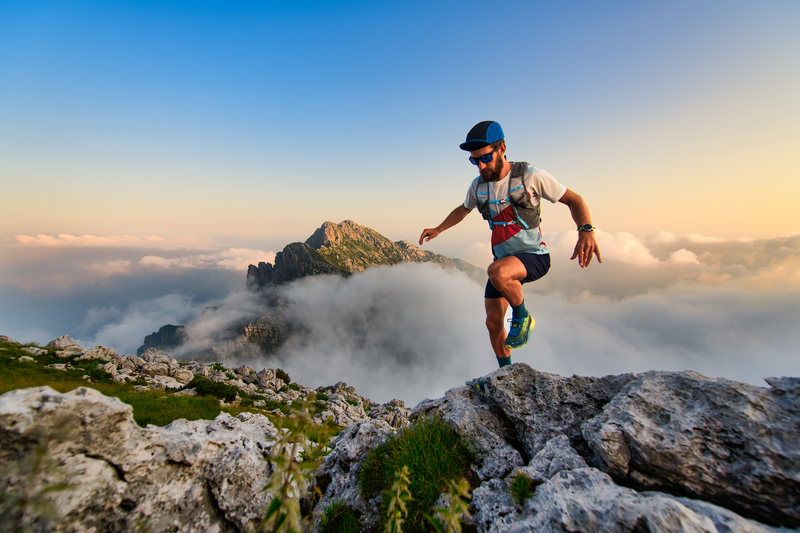
95% of researchers rate our articles as excellent or good
Learn more about the work of our research integrity team to safeguard the quality of each article we publish.
Find out more
ORIGINAL RESEARCH article
Front. Artif. Intell.
Sec. Machine Learning and Artificial Intelligence
Volume 8 - 2025 | doi: 10.3389/frai.2025.1444891
The final, formatted version of the article will be published soon.
You have multiple emails registered with Frontiers:
Please enter your email address:
If you already have an account, please login
You don't have a Frontiers account ? You can register here
Previous work has demonstrated that initialization is very important for both fitting a neural network by gradient descent methods, as well as for Variational inference of Bayesian Neural networks. In this work we investigate how Layer wise Scaled Gaussian Priors perform with Markov chain Monte Carlo trained Bayesian Neural networks. From our experiments on 8 classifications datasets of various complexity, the results indicate that using Layer wise Scaled Gaussian Priors makes the sampling process more efficient as compared to using an Isotropic Gaussian Prior, an Isotropic Cauchy Prior, or an Isotropic Laplace Prior. We also show that the cold posterior effect does not arise when using a either an Isotropic Gaussian or a layer wise Scaled Prior for small feed forward Bayesian Neural Networks. Since Bayesian Neural networks are becoming popular due to their advantages such as uncertainty estimation, and prevention of over-fitting, this work seeks to provide improvements in the efficiency of Bayesian Neural Networks learned using Markov Chain Monte Carlo methods.
Keywords: Bayesian neural network (BNN), MCMC (Markov chain Monte Carlo), deep learning - artificial intelligence, Neural Network, Bayesian inference (BI)
Received: 06 Jun 2024; Accepted: 03 Apr 2025.
Copyright: © 2025 Jawla and Kelleher. This is an open-access article distributed under the terms of the Creative Commons Attribution License (CC BY). The use, distribution or reproduction in other forums is permitted, provided the original author(s) or licensor are credited and that the original publication in this journal is cited, in accordance with accepted academic practice. No use, distribution or reproduction is permitted which does not comply with these terms.
* Correspondence:
Devesh Jawla, Technological University Dublin, Dublin, Ireland
Disclaimer: All claims expressed in this article are solely those of the authors and do not necessarily represent those of their affiliated organizations, or those of the publisher, the editors and the reviewers. Any product that may be evaluated in this article or claim that may be made by its manufacturer is not guaranteed or endorsed by the publisher.
Research integrity at Frontiers
Learn more about the work of our research integrity team to safeguard the quality of each article we publish.