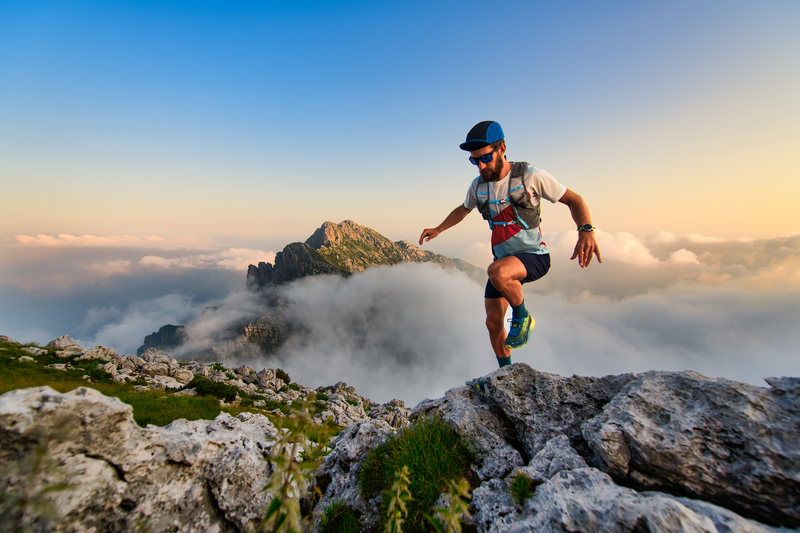
94% of researchers rate our articles as excellent or good
Learn more about the work of our research integrity team to safeguard the quality of each article we publish.
Find out more
ORIGINAL RESEARCH article
Front. Artif. Intell.
Sec. Medicine and Public Health
Volume 8 - 2025 | doi: 10.3389/frai.2025.1388180
This article is part of the Research Topic Outbreak Oracles: How AI's Journey through COVID-19 Shapes Future Epidemic Strategy View all 6 articles
The final, formatted version of the article will be published soon.
You have multiple emails registered with Frontiers:
Please enter your email address:
If you already have an account, please login
You don't have a Frontiers account ? You can register here
The main aim of this study is to employ artificial intelligence and machine learning methods to assess and correct pronunciation disorders in post-traumatic military patients, acknowledging the critical need for effective communication rehabilitation in individuals who have experienced trauma, such as head injuries or war-related incidents. Tasks include reviewing existing research, selecting appropriate machine learning methods, generating relevant training data, and implementing a software architecture tailored to analyze and correct pronunciation defects in this specific population. The analysis of machine learning methods led to the selection of two experimental models: a Convolutional Neural Network (CNN) utilizing mel-spectrograms for image-based sound representation and a Long Short-Term Memory (LSTM) network combined with mel-frequency cepstral coefficients, aiming to explore the effectiveness of sequential data processing in the context of pronunciation disorder classification in post-traumatic military patients. The results of the two models were compared based on the loss and accuracy functions of the training and validation data, error matrices, and such key metrics as precision, recall, and F1-score. Both models showed promising results in classifying dysarthria stages, but the CNN model performed slightly better in predicting all classes than the LSTM.
Keywords: Pronunciation, Unclear Pronunciation, Dysarthria, Classification, CNN, LSTM, Artificial intelligence tools
Received: 19 Feb 2024; Accepted: 24 Feb 2025.
Copyright: © 2025 Melnykova, Pavlyk, Patereha and Skopivskyi. This is an open-access article distributed under the terms of the Creative Commons Attribution License (CC BY). The use, distribution or reproduction in other forums is permitted, provided the original author(s) or licensor are credited and that the original publication in this journal is cited, in accordance with accepted academic practice. No use, distribution or reproduction is permitted which does not comply with these terms.
* Correspondence:
Nataliia Melnykova, Department of Artificial Intelligence Systems, Lviv Polytechnic National University, Lviv, Ukraine
Disclaimer: All claims expressed in this article are solely those of the authors and do not necessarily represent those of their affiliated organizations, or those of the publisher, the editors and the reviewers. Any product that may be evaluated in this article or claim that may be made by its manufacturer is not guaranteed or endorsed by the publisher.
Research integrity at Frontiers
Learn more about the work of our research integrity team to safeguard the quality of each article we publish.