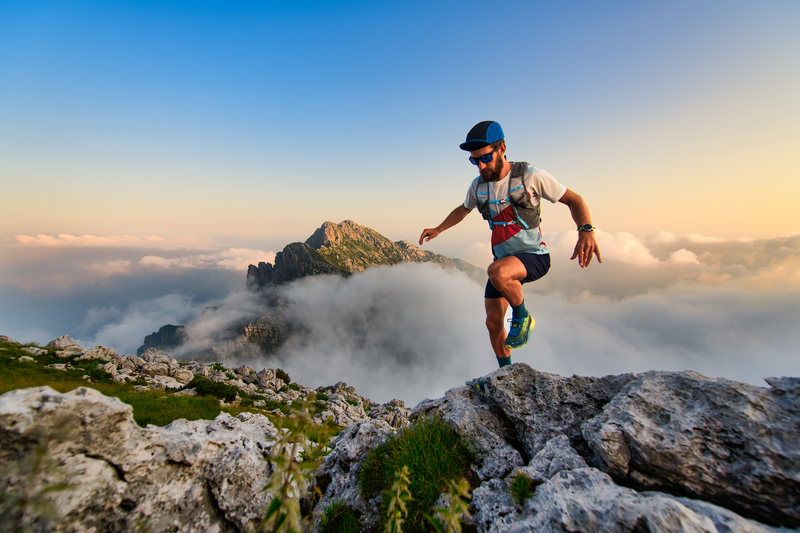
94% of researchers rate our articles as excellent or good
Learn more about the work of our research integrity team to safeguard the quality of each article we publish.
Find out more
REVIEW article
Front. Artif. Intell. , 07 January 2025
Sec. Medicine and Public Health
Volume 7 - 2024 | https://doi.org/10.3389/frai.2024.1487207
The rapid advancement of artificial intelligence (AI) has introduced transformative opportunities in oncology, enhancing the precision and efficiency of tumor diagnosis and treatment. This review examines recent advancements in AI applications across tumor imaging diagnostics, pathological analysis, and treatment optimization, with a particular focus on breast cancer, lung cancer, and liver cancer. By synthesizing findings from peer-reviewed studies published over the past decade, this paper analyzes the role of AI in enhancing diagnostic accuracy, streamlining therapeutic decision-making, and personalizing treatment strategies. Additionally, this paper addresses challenges related to AI integration into clinical workflows and regulatory compliance. As AI continues to evolve, its applications in oncology promise further improvements in patient outcomes, though additional research is needed to address its limitations and ensure ethical and effective deployment.
The term “artificial intelligence (AI) “was coined at the Dartmouth Summer Symposium in 1956 to describe the former ideas about “thinking machine.” AI refers to a machine’s capacity for autonomous learning, identifying patterns and associations from extensive sample data to make efficient decisions in unfamiliar scenarios (Bhinder et al., 2021). Specifically, machine learning (ML) is a subfield of AI, while deep learning (DL) is a subset of MI. In many fields, such as speech recognition, image classification and text understanding, DL breaks through traditional algorithm and gradually evolves into a novel paradigm that starts from training with representative materials to outputting deliverables via end-to-end modeling. In DL, each layer independently adjusts toward the overall goal, and all layers work synergistically to enhance task accuracy (Yu et al., 2017). With its advancement, AI shows the potential to completely change the medical industry in such domains as follows: assisting doctors in diagnosis to minimize misdiagnosis and missed diagnosis; improving diagnostic efficiency to relax the imbalance between supply and demand of medical resources; providing early warning of disease risk and health consultation services; supporting drug research and development while promoting pharmaceutical productivity; and bettering surgical robots and operational accuracy (Liu and Yu, 2022). Figure 1 illustrates the application pathway of AI in cancer diagnosis and treatment.
The aim of this review is to discuss the latest applications and advancements of artificial intelligence (AI) in tumor diagnosis and treatment, with an emphasis on its transformative impact on traditional diagnostic and therapeutic approaches and future potential. By synthesizing existing research findings, this review examines how AI plays a pivotal role in tumor imaging diagnostics, pathological analysis, and treatment optimization. To ensure a comprehensive and rigorous review, the literature selection was based on the following criteria: (1) only peer-reviewed academic articles and research reports published within the last 10 years were included (2); selected literature must involve applications of AI in tumor diagnosis, treatment, imaging analysis, or pathology. (3) Due to the broad scope and cancer types involved, this review primarily focuses on literature concerning breast, lung, and liver cancers, with emphasis on AI systems that have undergone regulatory approval and entered clinical practice, as well as emerging technologies with significant potential still under investigation. Table 1 presents the currently available AI software used in tumor diagnosis and treatment, along with their current developmental status.
Pathological diagnosis, commonly used in clinical practice, reveals etiology based on natural sciences and serves as the “gold standard” for disease identification. Machine-based oncological pathology is the discovery and classification of tumor-related features in pathological photos to determine its nature, stage and prognosis, aiming to improved objectivity and accuracy in diagnosis. In recent years, artificial intelligence, particularly deep learning (DL), has demonstrated significant potential in foci identification, prognosis prediction, microenvironment characterization, metastasis detection, and other pathological analyses for tumor diagnosis. Pathological AI has been applied in multiple types of tumors, such as breast cancer (Zhu et al., 2019), gastric cancer, nasopharyngeal carcinoma (Qi et al., 2021) and colorectal cancer, mainly for determination of benign or malignant nature (George and Sankaran, 2020), disease staging, staining analysis and early screening.
This digital pathology platform has been used for conferences, virtual seminars, presentations and educational purpose etc. AI enhances the utility of whole slide imaging (WSI), as AI tools assist in training next-generation pathologists by providing on-demand, standardized, and interactive digital slides that can be shared among multiple users anytime and anywhere (Zarella et al., 2019). WSI systems featuring automation, high speed, and high resolution have demonstrated a significant impact on medical quality assurance (QA), particularly when supported by AI, which readily provides digital slides to pathologists via laboratory information systems or intranets for various QA tasks, such as remote consultation, measuring inter-and intra-observer differences, competency testing, and slide archiving (Bera et al., 2019). Saillard et al. (2020) developed two DL algorithms based on WSI to predict the survival rate of patients with hepatocellular carcinoma underwent surgical excision, with both models superior to the traditional method based on comprehensive scores of all survival-related baseline variables, and they further verified prognostic value of the models in The Cancer Genome Atlas (TCGA) dataset.
As an important indicator for prediction of the efficacy and outcome of neoadjuvant chemotherapy, TIL is regarded by a growing number of professionals as an important biomarker for many types of cancer (Le et al., 2020; Zhang et al., 2023). However, its application in prognosis is largely limited, since TIL requires much labor for its standardized quantification relying on pathologists and it may additionally involve immunohistochemical staining that seriously lacks of unified measurement method. The advance of digital pathology and artificial intelligence inspires increasing interests to the development of automatic tools for counting and analysis of TILs in pathological sections. The “DeepTIL” created by Xu Hongming team aims to effectively and efficiently evaluate the TILs distribution in whole slide images post-H&E staining and to automatically conduct analysis for assistance in the prognosis of patients with colorectal cancer (Xu et al., 2022). Saltz et al. (2018) employed neural network that incorporated pathologist’s feedback to automatically detect the spatial organization of TILs in tissue section images of cancer genome maps, and they found that this characterization could predict the prognosis of 13 different cancer subtypes. Image analysis based on DL could detect TILs in testicular germ cell tumors rather objectively and makes TIL a prognostic marker of disease recurrence (Linder et al., 2019). Ao et al. (2022) reported an automated high-throughput microfluidic platform for simultaneously tracking the dynamics of T-cell infiltration and the cytotoxicity in 3D tumor cultures with adjustable matrix. This platform could evaluate the efficacy of each treatment with the help of a TIL analyzer based on clinical data-driven DL method. PathExplore IOP accelerates the characterization of immune phenotypes by quantifying tumor-infiltrating lymphocytes (TILs) and their spatial distribution utilizing routine hematoxylin and eosin (H&E) samples. This innovative product enables researchers to evaluate the spatial arrangements of TILs within the tumor core and periphery, thereby providing insights into the immune-inflamed, desert, or excluded characteristics of the sample (Path, 2024).
Medical imaging provides most of the medical data, but image recognition in routine diagnoses mainly depends on manual analysis. This traditional recognition method is limited by subjective judgments of radiologists; hence misdiagnosis may occur. AI support in image recognition and disease diagnosis may play an important role in lesion discovery and significantly improve diagnostic efficiency: independent and smart extraction and mining of image information for better image recognition; automatic and intelligent interpretation of the images based on DL and simulating human neural network for better analysis. Here are some examples: S-Detect™, a new ultrasound system supplied by Samsung (a South Korea company), significantly improved diagnostic performance of inexperienced radiologists for malignant breast tumors using DL algorithm for lesion classification (Lee et al., 2019); a team of researchers from Harvard Medical School and Beth Israel Deaconess Medical Center tested GPT-4’s performance in diagnosis of challenging medical records, and they found that it provided correct differentiation in 64% of the cases and even best diagnosis in 39% of all (Kanjee et al., 2023).
[18F] fluorodeoxyglucose ([18F]FDG) positron emission tomography/computed tomography (PET/CT) has become a primary imaging modality in oncology, utilized for disease diagnosis, staging, re-staging, and monitoring therapeutic outcomes (Hu et al., 2021). A research team has developed an automated system for rapid assessment of [18F] FDG PET/CT image quality. The system’s image quality assessment results show high concordance with the ratings of experienced nuclear medicine physicians, with kappa coefficients indicating strong agreement across different anatomical regions. Additionally, the system-generated objective quality metrics (e.g., SUVmax, SUVmean) meet clinical accuracy standards (Qi et al., 2023). AI-enhanced PET radiomics can predict patient response to neoadjuvant chemotherapy (NAC) (Hathi et al., 2020). Moreover, AI tools applied to PET radiomics enhance the detection and characterization of lymph node and distant metastases, improving staging accuracy for breast cancer patients (Li et al., 2021).
MRI radiomics is one of the most widely used techniques, with T2-weighted imaging (T2WI), diffusion-weighted imaging (DWI), and dynamic contrast-enhanced MRI (DCE-MRI) as the commonly used sequences. Radiogenomics establishes models by extracting a large number of quantitative data from segmented images and correlating image features with pathophysiology and gene expression. With further development and progress of AI, radiogenomics is rapidly becoming a powerful new tool in precision medicine, and it may turn into a critical part in clinical treatment planning and prognosis judgment. Fan et al. (2017) pioneered in using 3D volumetric image features to classify molecular subtypes of breast cancer, and then discussed the relationship between DCE-MRI-based radiomic features and molecular typing of breast cancer; By extracting and analyzing the features in morphology, texture, statistics, dynamics and bilateral difference in DCE-MRI images, they succeeded in differentiation of breast cancers among four different molecular subtypes (luminal A, luminal B, HER2 and basal-like) (Fan et al., 2017). Another team reported that a DCE-MRI-based comprehensive analysis of peritumoral and intratumoral radiological features of patients with invasive HER2+ breast cancer, helps in the identification of inherent molecular tumor subtypes and in the insight to the immune response within the environment around lesions, as well as in the prediction of responses to targeted therapy (Braman et al., 2019).
The segmentation of medical images is essential in representation and visualization of relevant structures in the images. Bhanumurthy and Anne (2014) developed an automatic technique that uses AI to accurately detect cerebral MRI signals and segment the images for neoplastic, atrophic and other abnormal tissues. Since the first image recognition system based on convolution neural network (CNN) was proposed in 1995, breast-tumor segmentation using DL has been employed by many medical imaging applications (Conte et al., 2020). Rahimpour et al. (2023) developed a deep CNN using T1-weighted post-contrast and subtraction magnetic resonance imaging (MRI) techniques for automatic three-dimensional (3D) segmentation of breast tumors; their use of visual ensemble selection provided clinically useful segmentation in 77% of the cases, demonstrating the possibility in reducing radiologists’ manual 3D segmentation labor and in greatly promoting the quantitative research of non-invasive biomarkers from breast MRI. George and Sankaran (2020) introduced a decision-making scheme based on patch class probability (NucDeep + SVM + PD) for image-level classification. They evaluated the proposed framework on the publicly accessible BreaKHis dataset through five random trials, achieving a mean image-level recognition rate of 96.66% ± 0.77%, a specificity of 100%, and a sensitivity of 96.21%. Their proposed NucDeep + FF + SVM model was found to be superior to several existing methods at the time and demonstrated comparable state-of-the-art performance even with low training complexity (George and Sankaran, 2020). Qi et al. (2021) reported their work on nasopharyngeal carcinoma: detection by CNN on computed tomography (CT) scans followed by segmentation from MRI images using multimodal MRI fusion network.
With the background of a large number of data available from high-throughput sequencing, rapidly developing AI technology has provided oncologists with deeper insights into tumors, ushering in a new era of clinical oncology focused on precision treatment and personalized medicine.
Precision medicine applies advanced medical techniques and proper biomarkers aiming at precise location of disease foci and optimal selection of treatment targets to tailor therapy regimen as per deep understanding of disease-specific manifestations and variations as well as individuals’ special conditions with the ultimate objective for the best efficacy. Different from personalized medicine, precision medicine features even more comprehensive considerations covering disease, medication and patient, rooting in more accurate analyses of disease characteristics and manifestation at a deeper level and in emphasizing even better application of medical technology during diagnosis and treatment, as well as in the best appropriateness of drug use. It is a consensus among the medical community that “precision cancer medicine” represents the trend (Mendelsohn, 2013). Predictive biomarkers help clinicians identify the patients with potentials to benefit from certain treatments and enable them make corresponding decision. In oncology, when a biomarker is proved to show statistical difference in therapeutic effect between the two patient populations with the biomarker positive versus negative, it is considered being predictive. For example, human epidermal growth factor 2 (HER2) can serve as a molecular marker, since its expression level in breast cancer, gastric cancer and other tumors predicts the efficacy of HER2 targeted drugs (Swain et al., 2023); among non-small cell lung cancers (NSCLCs), epidermal growth factor receptor (EGFR) deletion of exon 19 and its mutations in exon 21 are predictive for the use of EGFR tyrosine kinase inhibitors, such as osimertinib and erlotinib (Chan and Hughes, 2015). AI also presents some advantages for predictive or prognostic imaging biomarkers, since AI-aided analyses of imaging biomarkers are non-invasive, non-destructive, rapid, easily serializable and cheap, and because they only require conventional radiological scans (Verma et al., 2017), moreover they are fully compatible with existing clinical workflows, just like those of AI-aided pathological biomarkers (Bera et al., 2019). Trebeschi et al. (2019) established and validated a machine learning biomarker capable of predicting immunotherapy responders and non-responders, using AI-supported characterization of the primary and metastatic lesions of advanced malignant melanoma and NSCLC post-immunotherapy; in addition, the biomarker could characterize the tumors on the whole 3D volume, avoiding possible such sampling errors in heterogeneous biopsy samples as those reported in the literature (Cyll et al., 2017), and it was able to detect the changes of tumor microenvironment.
As another contributive approach in precision therapy and a new genetic testing method emergent in recent years, gene sequencing can be used to predict individual health by analyzing the whole sequences of genetic molecules with samples of personal blood or saliva, demonstrating positive effect in human health management. The value of gene sequencing lies in identifying one’s susceptible gene to guide cancer prevention and treatment (Chen et al., 2022). ML-assisted methylation sequencing of circulating tumor DNA provided advantages in cancer screening and treatment efficacy assessment (Fiala and Diamandis, 2019). For the first time, a research team applied DL to detect characteristics of multi-omics relevant to survival of patients with hepatocellular carcinoma (HCC); with their DL-aided model built on RNA sequencing, miRNA sequencing and TCGA methylation data together with clinical data from 360 HCC patients, they achieved prediction performance comparable to that derived from the combination of genomics and clinical data (Chaudhary et al., 2018). Cristiano et al. (2019) established an ML model incorporating genome-wide fragment characteristics to evaluate fragmentation pattern of cell-free DNA in the whole genomic length, realizing sensitivities ranging from 57 to 99% + with the specificity at 98% and an overall area under ROC curve (AUC) of 0.94 among seven types of cancer, including bile duct, breast, colorectal, gastric, lung, ovarian, and pancreatic cancers; in 75% of the cases, fragment characteristics could be used to determine tissues of cancer origin from limited number of foci (Cristiano et al., 2019).
Apart from gene sequencing, immunotherapy constitutes another important part of precision medicine. As to oncology, the main immunotherapy strategies include adoptive cellular immunotherapy, immune checkpoint monoclonal antibody therapy, vaccine therapy, non-specific immune stimulation (cytokine therapy). Despite the impressive progress in immunotherapy, obstacles and challenges are still in the way, including limitation in response rate and inability to predict efficacy and side effects (such as autoimmune response or cytokine release syndrome), thus hindering further application of immunotherapy in clinical practice (Zhang and Zhang, 2020). AI partly overcomes these problems and provides precise treatment by means of, for instance, evaluating efficacy of immunotherapy (Ao et al., 2022), predicting patients’ prognosis and treatment response (Xu et al., 2021; Wang et al., 2022), and distinguishing tumor subtypes to guide treatment (Zhu et al., 2023). Here are some particular examples: plasma cell subtyping with the help of an artificial neural network based on ML algorithm could provide an effective prognostic indicator for prostate cancer and be an option for potential responders to immunotherapy (Xie et al., 2022). With an ML algorithm, a classifier based on five hub gene was constructed to predict the stemness subtypes of patients with hepatocellular carcinoma in a more convenient and applicable way; it could not only act as a guide for further study of the mechanism between tumor stem cells and tumor microenvironment, but also as a potential method for selecting patients more responsive to immunotherapy (Chen et al., 2022). Zhu et al. (2023) used ML methods to identify colon cancer subtypes to provide guidance for subtype-specific medication. Another study integrated ML and single-cell trajectory analysis of T cell depletion to establish a T-cell exhaustion score (TES) model for predicting disease prognosis and immunotherapy responsiveness in patients with colon cancer (Shen et al., 2023). DNA methylation profile has been identified as an indicator of tumor immune microenvironment; because of its stability and convenient measurement via fluid biopsy, it becomes a potential hotspot for predicting therapeutic response to immune checkpoint inhibitors (ICIs). A research team used the methylation level of selected sites to construct an ML model for responsiveness prediction, and this model performed well at both pan-cancer and tumor-specific levels (Xu et al., 2021). A team from Pohang University of Science and Technology proposed a ML framework that uses web-based analysis to identify biomarkers of ICI therapy, and therewith they accurately predicted the ICI response to melanoma, gastric and bladder cancers (Kong et al., 2022). For those patients being sensitive to immunotherapy drugs, ELISE (Ensemble Learning for Immunotherapeutic Response Evaluation) was proposed to generate a highly accurate method for predicting individual responses; the trial results showed that ELISE offered higher accuracy in predicting the response of esophageal adenocarcinoma patients to Atezolizumab; in addition, ELISE could be scaled to accurately evaluate the responsiveness of various immunotherapeutic drugs among other cancers, like programmed death-1/programmed death-ligand 1 (PD1/PD-L1) inhibitors against metastatic urothelial cancer, and melanoma antigen family A3 (MAGE-A3) immunotherapy for metastatic melanoma (Jin et al., 2022).
The history of new drug research and development is also a history of technological evolution thereof, from random drug screening during 1930s to 1960s, across high-throughput selection within 1970s to the early years in 21st century, and to current virtual exploration. Bioanalysis enhanced by ML provides inspires in mining characterization and association in biological networks, supplement to effective deal with high-throughput data of heterogeneous and complex molecules (You et al., 2022). Rapidly developing multi-omic technology in the oncological area has become one of the critical factors for AI-aided biological analysis in discovering new anticancer targets (do Valle et al., 2018). These techniques primarily manifest in the analysis of epigenetics, genomics, proteomics, and metabonomics as well as multi-omic integration. For example, do Valle et al. (2021) have developed a web-based biological analysis framework to calculate the proximity between polyphenol targets and disease proteins; their study showing the network relationship between disease proteins and polyphenol targets provides a calculation method for revealing the effects of polyphenols on disease and offers a basis for finding new anticancer targets (do Valle et al., 2021). A supercomputer provided by International Business Machines Corporation (IBM) enables Atomwise, a US drug research and development company, to screen molecules with potential for drug development from a large-scale data storage system; relying on the AI platform “Interrogative Biology” built by itself, this research company makes an in-depth analysis of the defense system of human cells via the in vivo mechanism, and it explores the physiological law of human body with the help of big data and AI technology to find human molecules with therapeutic potential; while controlling costs and saving workload, this mode contributes to cancer therapy as well (Barden and Omuro, 2023). AlphaFold was awarded the 2024 Nobel Prize in Chemistry. AlphaFold AI plays a significant role in drug discovery, particularly in protein structure prediction, target identification, virtual screening, drug design, and understanding mechanisms of drug resistance. First, AlphaFold can efficiently and accurately predict three-dimensional protein structures, which is critical for understanding the functions and interactions of drug targets (Jumper et al., 2021). In addition, by leveraging its structural prediction capabilities, potential drug targets can be identified, especially in research focused on tumors and other complex diseases. The protein structure information provided by AlphaFold can also be applied to virtual screening, aiding in the rapid assessment of compound-target binding affinities. During drug design, its structural data can be utilized for molecular docking and drug optimization, enhancing the efficiency of new drug design (Baek et al., 2021). Finally, by analyzing how mutations affect protein structure, AlphaFold assists in studying mechanisms of drug resistance, offering new insights into managing drug resistance (Zhao et al., 2024). AlphaFold continues to evolve, with AlphaFold 3 (AF3) extending its applications beyond protein structure prediction to encompass high-precision predictions for 59 types of complexes, including the structures and interactions of diverse biomolecules like nucleic acids and ligands. However, AlphaFold 3 still faces limitations in stereochemistry, model hallucination, dynamics, and target-specific accuracy (Abramson et al., 2024).
The application of AI in surgery is represented mainly in surgical decision-making, postoperative prediction and robotic surgery. As to the first aspect, here are some examples: The trial with AI plus multicenter registration data to predict disease-free survival rate among participants with pancreatic cancer demonstrated that AI could provide a valuable decision support system for these patients going to receive surgery (Lee et al., 2021). Neoadjuvant systemic therapy (NAST), though, achieved complete pathological remission in 40–70% of breast cancer patients, the uncertainty of the residual disease still makes it hard to identify patients who are eligible to omit surgery (Heil et al., 2021). This difficulty contributed to the birth of a multimodal ML algorithm (Intelligent Vacuum Assisted Biopsy (VAB)) that integrates four variables: patient, tumor, imaging and biopsy, and this algorithm proved its performance by reliable elimination of cancer if any left from NAST (Pfob and Heil, 2023; Pfob et al., 2022). For early gastric cancer, the biggest challenge in the selection between endoscopic resection and surgical excision is the differentiation of invasion depth as intramucosal or submucosal, but no existing accurate method is available (Sano et al., 1990). To address this problem, an AI classifier was designed and validated, accordingly a diagnostic approach was fabricated involving both AI and endoscopists, and it truly improved the diagnostic efficiency (Goto et al., 2023). In terms of robotic surgery, it brings many advantages for surgeons, like accurate movement, instability resistance, large spatial freedom and potent three-dimensional imaging (Park and Hyung, 2020). For instance, Da Vinci, a robotic surgical system developed by Intuitive Surgical, timely provides doctors with objective and accurate surgical information (Nota et al., 2019). At present, robot-assisted surgery has been used for endometrial cancer (Zanagnolo et al., 2017), thymoma (Shen et al., 2022), gastric cancer (Park and Hyung, 2020), colon cancer (Cuk et al., 2022), prostate cancer, bladder cancer (Falagario et al., 2020) and so on, and it exhibits multiple advantages in both intraoperative and postoperative stages, i.e., less blood loss (Park and Hyung, 2020) and drainage during operation, and less days of pleural drainage, shorter hospital stay and less complications after operation (Shen et al., 2022). Finally, AI is promising in prediction of postoperative recurrence and survival rate. Chung Heewon’s team developed an AI model that used a large training cohort and a number of variables (including preoperative and postoperative nutrition and fat/muscle index) to predict 5-year survival probability of stomach cancer patients at 1 year post-gastrectomy, and they achieved generally accurate prediction (Chung et al., 2023); Lai et al. (2023) collected data from 2,936 patients to build a training set, developed an AI model TRAIN-AI to predict the recurrence of hepatocellular carcinoma after transplantation, and built a network calculator to improve the availability of the model; they planned further update of this AI model by increasing the number of patients (Lai et al., 2023). Provided proper application after strict assessment and external verification, AI may change surgical care by supporting surgical decision-making, identifying and mitigating changeable risk factors, identifying and managing complications, and sharing resource-use decisions (Loftus et al., 2020). However, surgical robots may prolong surgery duration and increase medical costs (Olavarria et al., 2020), requiring attention in further development and improvement.
Before making a radiotherapy plan, doctors may averagely take more than 200 CT images for each patient and spend 3–5 h to identify the target area. AI may lower the workload herein. Specifically speaking, an AI system first defines an imaging scheme proper for a particular confirmed diagnosis, then delineates the target tumor areas on CTs through automatic image recognition, and provides a corresponding radiotherapy plan to submit doctor for manual review; Moreover, AI can monitor the implementation of the plan during actual treatment (Liu and Yu, 2022). The application of AI in radiology has greatly improved the accuracy and safety of every step in cancer radiotherapy, including initial decision-making, treatment planning, and in-process dose adjustment, as well as efficacy evaluation and side effect prediction.
Developed by IBM with the help of top oncologists at Memorial Sloan Kettering Cancer Center (MSKCC), Watson for Oncology (WFO) is an AI-aided medical application that focuses on providing decision support in cancer treatment. It uses AI and cognitive computing technology in analyzing medical literature, patient records and other clinical information to help doctors make personalized cancer treatment plans. A meta-analysis evaluated the consistency of treatment regimens between WFO and multidisciplinary team (MDT) involving seven types (breast, rectal, colon, stomach, lung, ovary and cervical) of cancer and concluded that they were highly comparable (Jie et al., 2021). WFO features in improved work efficiency, reduced workload (Printz, 2017), lowered artificial calculation errors in both radiotherapy regimen and drug selection (Keiffer, 2015). It allows more patients participate in decision-making of their own treatment plan and know more about possible adverse effects, therefore being beneficial to alleviating physician-patient disputes (Fang et al., 2018). Nevertheless, due to variation in clinical guidelines, drug preference and economic factors among different countries, the consistency between WFO and MDT for cancer patients is limited in some aspect, showing requirements for further improvement of the system.
As a new type of radiotherapy, intensity-modulated radiotherapy (IMRT) provides accurate conformal radiation with beams at different intensity based on differentiation tumor target areas from OARs. Contouring or delineation is the premise and guarantee for efficacy and safety of radiotherapy. Manual delineation, the traditional way, is usually time-consuming and tedious, still with significant interobserver variation. So oncological researchers in the field of breast cancer (Mikalsen et al., 2023), prostate cancer (Urago et al., 2021), cervical cancer (Yao et al., 2022) and the like have been exploring the feasibility of clinical utilization of AI in contouring tumor target and OARs. Mikalsen et al. (2023) prospectively included 30 patients who received radiotherapy for breast cancer to evaluate the geometric consistency between automatic segmentation and manual contour. Their verification showed that the profile of OARs generated by the DL segmentation model (DLS) could be used in clinical practice, but most of the clinical target volume (CTV) according to the DLS required correction. Urago et al. (2021) compared MIM Maestro (based on atlas) and MIM Contour Protégé AI (based on artificial intelligence) in automatic segmentation of OARs for 21 patients with prostate cancer. Their work showed that the AI-based model rendered higher accuracy in bladder and rectum than three atlas-based ones: Dice similarity coefficient (DSC), Hausdorff distance (HD) and mean distance to agreement (MDA) (Urago et al., 2021). Yao et al. (2022) studied the application of both AccuLearning-based small sample size training and the AccuContour automatic delineation in MRI imaging of patients with cervical cancer; compared to manual procedure averagely lasting 30 min, AI technique spent only 1 min, and the latter displayed good performance for multiple structures; the result suggested that the smart approach is clinically feasible to improve the quality and efficiency in segmentation of OARs related to radiotherapy against cervical cancer (Yao et al., 2022). Another team tried AccuContour for automatic delineation of OARs in nasopharyngeal carcinoma subject to radiotherapy, and they found that this AI-aided software basically worked well, and that the results of small volume OARs, although with an accuracy slightly poor than that of larger volume ones, could be used in clinical work after minor rectification by doctors (Wu et al., 2021). All these above verified that AI-assisted radiotherapy greatly improves the work efficiency of doctors and enjoys fairly good accuracy. However, some limitations in AI application are also indicated by these studies as follows: (1) small sample size for training; (2) dataset annotation and delineation criteria with variation dependent on local preferences and institutional standards; (3) challenged interpretability and data dependence of the models because of inherent complexity of basic algorithms; and (4) risks of weakening clinicians’ role and losing some basic knowledge due to over-dependence on automation (Batumalai et al., 2020).
Artificial intelligence has remarkably achieved in medical image analysis and pathological diagnosis. Its deep learning technology enables automatic identification and analysis of patterns from tumor images, and it improves early diagnosis of tumors, contributing to reduced burden of medical system and alleviated shortage of medical resources. In addition, AI stands out for its performance in tumor treatment planning and in provision for doctors with more suitable advice on the ground of accurate analysis of clinical data to formulate individual regimens, and in turn it improves therapy success rate, cancels unnecessary treatment to reduce economic burden of patients, and ultimately it realizes precise medical care. In spite of its great progress in tumor diagnosis and treatment, AI still faces some problems and challenges that may affect its application in practice: (1) sample data imbalance: relatively poor availability of data for some types of tumor resulting in the imbalance of samples used for training AI; (2) data privacy and security issues; (3) poor interpretation: being regarded as “black box” models, complex AI techniques such as deep learning are usually difficult to be explained for their rationale in decision-making. In the field of medicine especially in the diagnosis and treatment of tumors, healthcare professionals and patients prefer to understand the mechanism leading to certain specific diagnosis or treatment recommendations. Therefore, lack of exact interpretation of the models may limit their practical application; (4) challenges in clinical verification; (5) regulatory and ethical issues. To sum up, the application of AI in tumor diagnosis and treatment has brought revolutionary changes in the field of medicine, but still companied by some problems requiring settlement to ensure its effectiveness and reliability in real-world clinical practice.
FS: Writing – original draft. LZ: Writing – review & editing. ZT: Writing – review & editing.
The author(s) declare that financial support was received for the research, authorship, and/or publication of this article. This study was funded by National Natural Science Foundation of China (81602340, 81472183), Tianjin Key Medical Discipline (Specialty) Construction Project (TJYXZDXK-009A, TJYXZDXK-012A), Tianjin Medical University Cancer Hospital “14th Five-Year” Peak Discipline Support Program Project, Shenzhen Chipscreen Biosciences project, and Science and Technology Project of Tianjin Health Commission (Grant no. TJWJ2021MS009).
This work utilized generative AI technology for the creation of table.The specific AI tool used was ChatGPT (version GPT-4), developed by OpenAI.
The authors declare that the research was conducted in the absence of any commercial or financial relationships that could be construed as a potential conflict of interest.
All claims expressed in this article are solely those of the authors and do not necessarily represent those of their affiliated organizations, or those of the publisher, the editors and the reviewers. Any product that may be evaluated in this article, or claim that may be made by its manufacturer, is not guaranteed or endorsed by the publisher.
Abramson, J., Adler, J., Dunger, J., Evans, R., Green, T., Pritzel, A., et al. (2024). Accurate structure prediction of biomolecular interactions with alpha fold 3. Nature 630, 493–500. doi: 10.1038/s41586-024-07487-w
Ao, Z., Cai, H., Wu, Z., Hu, L., Nunez, A., Zhou, Z., et al. (2022). Microfluidics guided by deep learning for cancer immunotherapy screening. Proc. Natl. Acad. Sci. USA 119:e2214569119. doi: 10.1073/pnas.2214569119
Baek, M., DiMaio, F., Anishchenko, I., Dauparas, J., Ovchinnikov, S., Lee, G. R., et al. (2021). Accurate prediction of protein structures and interactions using a three-track neural network. Science 373, 871–876. doi: 10.1126/science.abj8754
Barden, M. M., and Omuro, A. M. (2023). Top advances of the year: neuro-oncology. Cancer 129, 1467–1472. doi: 10.1002/cncr.34711
Batumalai, V., Jameson, M. G., King, O., Walker, R., Slater, C., Dundas, K., et al. (2020). Cautiously optimistic: a survey of radiation oncology professionals’ perceptions of automation in radiotherapy planning. Tech. Innov. Patient Support Radiat. Oncol 16, 58–64. doi: 10.1016/j.tipsro.2020.10.003
Bera, K., Schalper, K. A., Rimm, D. L., Velcheti, V., and Madabhushi, A. (2019). Artificial intelligence in digital pathology — new tools for diagnosis and precision oncology. Nat. Rev. Clin. Oncol. 16, 703–715. doi: 10.1038/s41571-019-0252-y
Bhanumurthy, M. Y., and Anne, K. (2014). “An automated detection and segmentation of tumor in brain MRI using artificial intelligence,” in 2014 IEEE International Conference on Computational Intelligence and Computing Research. Coimbatore: IEEE, 1–6.
Bhinder, B., Gilvary, C., Madhukar, N. S., and Elemento, O. (2021). Artificial intelligence in Cancer research and precision medicine. Cancer Discov. 11, 900–915. doi: 10.1158/2159-8290.CD-21-0090
Braman, N., Prasanna, P., Whitney, J., Singh, S., Beig, N., Etesami, M., et al. (2019). Association of Peritumoral Radiomics with Tumor Biology and Pathologic Response to preoperative targeted therapy for HER2 (ERBB2)-positive breast Cancer. JAMA Netw. Open 2:e192561. doi: 10.1001/jamanetworkopen.2019.2561
Chan, B. A., and Hughes, B. G. (2015). Targeted therapy for non-small cell lung cancer: current standards and the promise of the future. Transl. Lung Cancer Res. 4, 36–54. doi: 10.3978/j.issn.2218-6751.2014.05.01
Chaudhary, K., Poirion, O. B., Lu, L., and Garmire, L. X. (2018). Deep learning-based multi-omics integration robustly predicts survival in liver Cancer. Clin. Cancer Res. 24, 1248–1259. doi: 10.1158/1078-0432.CCR-17-0853
Chen, D.-L., Cai, J.-H., and Wang, C. C. N. (2022). Identification of key prognostic genes of triple negative breast Cancer by LASSO-based machine learning and bioinformatics analysis. Genes 13:902. doi: 10.3390/genes13050902
Chen, D., Liu, J., Zang, L., Xiao, T., Zhang, X., Li, Z., et al. (2022). Integrated machine learning and Bioinformatic analyses constructed a novel Stemness-related classifier to predict prognosis and immunotherapy responses for hepatocellular carcinoma patients. Int. J. Biol. Sci. 18, 360–373. doi: 10.7150/ijbs.66913
Chung, H., Ko, Y., Lee, I.-S., Hur, H., Huh, J., Han, S.-U., et al. (2023). Prognostic artificial intelligence model to predict 5 year survival at 1 year after gastric cancer surgery based on nutrition and body morphometry. J. Cachexia. Sarcopenia Muscle 14, 847–859. doi: 10.1002/jcsm.13176
Conte, L., Tafuri, B., Portaluri, M., Galiano, A., Maggiulli, E., and De Nunzio, G. (2020). Breast Cancer mass detection in DCE–MRI using deep-learning features followed by discrimination of infiltrative vs. In situ carcinoma through a machine-learning approach. Appl. Sci. 10:6109. doi: 10.3390/app10176109
Cristiano, S., Leal, A., Phallen, J., Fiksel, J., Adleff, V., Bruhm, D. C., et al. (2019). Genome-wide cell-free DNA fragmentation in patients with cancer. Nature 570, 385–389. doi: 10.1038/s41586-019-1272-6
Cuk, P., Kjær, M. D., Mogensen, C. B., Nielsen, M. F., Pedersen, A. K., and Ellebæk, M. B. (2022). Short-term outcomes in robot-assisted compared to laparoscopic colon cancer resections: a systematic review and meta-analysis. Surg. Endosc. 36, 32–46. doi: 10.1007/s00464-021-08782-7
Cyll, K., Ersvær, E., Vlatkovic, L., Pradhan, M., Kildal, W., Avranden Kjær, M., et al. (2017). Tumour heterogeneity poses a significant challenge to cancer biomarker research. Br. J. Cancer 117, 367–375. doi: 10.1038/bjc.2017.171
do Valle, Í. F., Menichetti, G., Simonetti, G., Bruno, S., Zironi, I., Durso, D. F., et al. (2018). Network integration of multi-tumor omics data suggests novel targeting strategies. Nat. Commun. 9:4514. doi: 10.1038/s41467-018-06992-7
do Valle, I. F., Roweth, H. G., Malloy, M. W., Moco, S., Barron, D., Battinelli, E., et al. (2021). Network medicine framework shows that proximity of polyphenol targets and disease proteins predicts therapeutic effects of polyphenols. Nat. Food 2, 143–155. doi: 10.1038/s43016-021-00243-7
Falagario, U., Veccia, A., Weprin, S., Albuquerque, E. V., Nahas, W. C., Carrieri, G., et al. (2020). Robotic-assisted surgery for the treatment of urologic cancers: recent advances. Expert Rev. Med. Devices 17, 579–590. doi: 10.1080/17434440.2020.1762487
Fan, M., Li, H., Wang, S., Zheng, B., Zhang, J., and Li, L. (2017). Radiomic analysis reveals DCE-MRI features for prediction of molecular subtypes of breast cancer. PLoS One 12:e0171683. doi: 10.1371/journal.pone.0171683
Fang, J., Zhu, Z., Wang, H., Hu, F., Liu, Z., Guo, X., et al. (2018). The establishment of a new medical model for tumor treatment combined with Watson for oncology, MDT and patient involvement. JCO 36:e18504. doi: 10.1200/JCO.2018.36.15_suppl.e18504
Fiala, C., and Diamandis, E. P. (2019). New approaches for detecting cancer with circulating cell-free DNA. BMC Med. 17:159. doi: 10.1186/s12916-019-1400-z
George, K., and Sankaran, P. (2020). Computer assisted recognition of breast cancer in biopsy images via fusion of nucleus-guided deep convolutional features. Comput. Methods Prog. Biomed. 194:105531. doi: 10.1016/j.cmpb.2020.105531
Goto, A., Kubota, N., Nishikawa, J., Ogawa, R., Hamabe, K., Hashimoto, S., et al. (2023). Cooperation between artificial intelligence and endoscopists for diagnosing invasion depth of early gastric cancer. Gastric Cancer 26, 116–122. doi: 10.1007/s10120-022-01330-9
Hathi, D. K., Li, W., Seo, Y., Flavell, R. R., Kornak, J., Franc, B. L., et al. (2020). Evaluation of primary breast cancers using dedicated breast PET and whole-body PET. Sci. Rep. 10:21930. doi: 10.1038/s41598-020-78865-3
Heil, J., Pfob, A., and Morrow, M. (2021). De-escalation of breast and axillary surgery in exceptional responders to neoadjuvant systemic treatment. Lancet Oncol. 22, 435–436. doi: 10.1016/S1470-2045(21)00057-7
Hu, P., Zhang, Y., Yu, H., Chen, S., Tan, H., Qi, C., et al. (2021). Total-body 18F-FDG PET/CT scan in oncology patients: how fast could it be? Eur. J. Nucl. Med. Mol. Imaging 48, 2384–2394. doi: 10.1007/s00259-021-05357-5
Jie, Z., Zhiying, Z., and Li, L. (2021). A meta-analysis of Watson for oncology in clinical application. Sci. Rep. 11:5792. doi: 10.1038/s41598-021-84973-5
Jin, W., Yang, Q., Chi, H., Wei, K., Zhang, P., Zhao, G., et al. (2022). Ensemble deep learning enhanced with self-attention for predicting immunotherapeutic responses to cancers. Front. Immunol. 13:1025330. doi: 10.3389/fimmu.2022.1025330
Jumper, J., Evans, R., Pritzel, A., Green, T., Figurnov, M., Ronneberger, O., et al. (2021). Highly accurate protein structure prediction with alpha fold. Nature 596, 583–589. doi: 10.1038/s41586-021-03819-2
Kanjee, Z., Crowe, B., and Rodman, A. (2023). Accuracy of a generative artificial intelligence model in a complex diagnostic challenge. JAMA 330, 78–80. doi: 10.1001/jama.2023.8288
Keiffer, M. R. (2015). Utilization of clinical practice guidelines: barriers and facilitators. Nurs. Clin. N. Am. 50, 327–345. doi: 10.1016/j.cnur.2015.03.007
Kong, J., Ha, D., Lee, J., Kim, I., Park, M., Im, S.-H., et al. (2022). Network-based machine learning approach to predict immunotherapy response in cancer patients. Nat. Commun. 13:3703. doi: 10.1038/s41467-022-31535-6
Lai, Q., De Stefano, C., Emond, J., Bhangui, P., Ikegami, T., Schaefer, B., et al. (2023). Development and validation of an artificial intelligence model for predicting post-transplant hepatocellular cancer recurrence. Cancer Commun. 43, 1381–1385. doi: 10.1002/cac2.12468
Le, H., Gupta, R., Hou, L., Abousamra, S., Fassler, D., Torre-Healy, L., et al. (2020). Utilizing automated breast Cancer detection to identify spatial distributions of tumor-infiltrating lymphocytes in invasive breast Cancer. Am. J. Pathol. 190, 1491–1504. doi: 10.1016/j.ajpath.2020.03.012
Lee, K.-S., Jang, J.-Y., Yu, Y.-D., Heo, J. S., Han, H.-S., Yoon, Y.-S., et al. (2021). Usefulness of artificial intelligence for predicting recurrence following surgery for pancreatic cancer: retrospective cohort study. Int. J. Surg. 93:106050. doi: 10.1016/j.ijsu.2021.106050
Lee, J., Kim, S., Kang, B. J., Kim, S. H., and Park, G. E. (2019). Evaluation of the effect of computer aided diagnosis system on breast ultrasound for inexperienced radiologists in describing and determining breast lesions. Med. Ultrason. 21, 239–245. doi: 10.11152/mu-1889
Li, Z., Kitajima, K., Hirata, K., Togo, R., Takenaka, J., Miyoshi, Y., et al. (2021). Preliminary study of AI-assisted diagnosis using FDG-PET/CT for axillary lymph node metastasis in patients with breast cancer. EJNMMI Res. 11:10. doi: 10.1186/s13550-021-00751-4
Linder, N., Taylor, J. C., Colling, R., Pell, R., Alveyn, E., Joseph, J., et al. (2019). Deep learning for detecting tumour-infiltrating lymphocytes in testicular germ cell tumours. J. Clin. Pathol. 72, 157–164. doi: 10.1136/jclinpath-2018-205328
Liu, D. M., and Yu, H. J. (2022). Smart healthcare: Applications and future of digital intelligence. 1st Edn. Hangzhou: Zhejiang University Press.
Loftus, T. J., Tighe, P. J., Filiberto, A. C., Efron, P. A., Brakenridge, S. C., Mohr, A. M., et al. (2020). Artificial intelligence and surgical decision-making. JAMA Surg. 155, 148–158. doi: 10.1001/jamasurg.2019.4917
Mendelsohn, J. (2013). Personalizing oncology: perspectives and prospects. J. Clin. Oncol. 31, 1904–1911. doi: 10.1200/JCO.2012.45.3605
Mikalsen, S. G., Skjøtskift, T., Flote, V. G., Hämäläinen, N. P., Heydari, M., and Rydén-Eilertsen, K. (2023). Extensive clinical testing of deep learning segmentation models for thorax and breast cancer radiotherapy planning. Acta Oncol. 62, 1184–1193. doi: 10.1080/0284186X.2023.2270152
Nota, C. L. M. A., Smits, F. J., Woo, Y., Borel Rinkes, I. H. M., Molenaar, I. Q., Hagendoorn, J., et al. (2019). Robotic developments in Cancer surgery. Surg. Oncol. Clin. N. Am. 28, 89–100. doi: 10.1016/j.soc.2018.07.003
Olavarria, O. A., Bernardi, K., Shah, S. K., Wilson, T. D., Wei, S., Pedroza, C., et al. (2020). Robotic versus laparoscopic ventral hernia repair: multicenter, blinded randomized controlled trial. BMJ 370:m2457. doi: 10.1136/bmj.m2457
Park, S. H., and Hyung, W. J. (2020). Current perspectives on the safety and efficacy of robot-assisted surgery for gastric cancer. Expert Rev. Gastroenterol. Hepatol. 14, 1181–1186. doi: 10.1080/17474124.2020.1815531
Path, A. I.. (2024). Path explore: an AI-powered pathology panel to unlock untapped insights from the tumor microenvironment. Available at: https://www.pathai.com/resources/pathai-announces-pathexplore-an-ai-powered-pathology-panel-to-unlock-untapped-insights-from-the-tumor-microenvironment/ (accessed November10, 2024).
Pfob, A., and Heil, J. (2023). Artificial intelligence to de-escalate loco-regional breast cancer treatment. Breast 68, 201–204. doi: 10.1016/j.breast.2023.02.009
Pfob, A., Sidey-Gibbons, C., Rauch, G., Thomas, B., Schaefgen, B., Kuemmel, S., et al. (2022). Intelligent vacuum-assisted biopsy to identify breast Cancer patients with pathologic complete response (yp T0 and ypN0) after Neoadjuvant systemic treatment for omission of breast and axillary surgery. J. Clin. Oncol. 40, 1903–1915. doi: 10.1200/JCO.21.02439
Printz, C. (2017). Artificial intelligence platform for oncology could assist in treatment decisions. Cancer 123:905. doi: 10.1002/cncr.30655
Qi, Y., Li, J., Chen, H., Guo, Y., Yin, Y., Gong, G., et al. (2021). Computer-aided diagnosis and regional segmentation of nasopharyngeal carcinoma based on multi-modality medical images. Int. J. Comput. Assist. Radiol. Surg. 16, 871–882. doi: 10.1007/s11548-021-02351-y
Qi, C., Wang, S., Yu, H., Zhang, Y., Hu, P., Tan, H., et al. (2023). An artificial intelligence-driven image quality assessment system for whole-body [18F] FDG PET/CT. Eur. J. Nucl. Med. Mol. Imaging 50, 1318–1328. doi: 10.1007/s00259-022-06078-z
Rahimpour, M., Saint Martin, M. J., Frouin, F., Akl, P., Orlhac, F., Koole, M., et al. (2023). Visual ensemble selection of deep convolutional neural networks for 3D segmentation of breast tumors on dynamic contrast enhanced MRI. Eur. Radiol. 33, 959–969. doi: 10.1007/s00330-022-09113-7
Saillard, C., Schmauch, B., Laifa, O., Moarii, M., Toldo, S., Zaslavskiy, M., et al. (2020). Predicting survival after hepatocellular carcinoma resection using deep learning on histological slides. Hepatology 72, 2000–2013. doi: 10.1002/hep.31207
Saltz, J., Gupta, R., Hou, L., Kurc, T., Singh, P., Nguyen, V., et al. (2018). Spatial organization and molecular correlation of tumor-infiltrating lymphocytes using deep learning on pathology images. Cell Rep. 23, 181–193. doi: 10.1016/j.celrep.2018.03.086
Sano, T., Okuyama, Y., Kobori, O., Shimizu, T., and Morioka, Y. (1990). Early gastric caner: Endoscopie diagnosis of depth of invasion. Digest Dis. Sci. 35, 1340–1344. doi: 10.1007/BF01536738
Shen, C., Li, J., Li, J., and Che, G. (2022). Robot-assisted thoracic surgery versus video-assisted thoracic surgery for treatment of patients with thymoma: a systematic review and meta-analysis. Thorac. Cancer 13, 151–161. doi: 10.1111/1759-7714.14234
Shen, X., Zuo, X., Liang, L., Wang, L., and Luo, B. (2023). Integrating machine learning and single-cell trajectories to analyze T-cell exhaustion to predict prognosis and immunotherapy in colon cancer patients. Front. Immunol. 14:1162843. doi: 10.3389/fimmu.2023.1162843
Swain, S. M., Shastry, M., and Hamilton, E. (2023). Targeting HER2-positive breast cancer: advances and future directions. Nat. Rev. Drug Discov. 22, 101–126. doi: 10.1038/s41573-022-00579-0
Trebeschi, S., Drago, S. G., Birkbak, N. J., Kurilova, I., Cǎlin, A. M., Delli Pizzi, A., et al. (2019). Predicting response to cancer immunotherapy using noninvasive radiomic biomarkers. Ann. Oncol. 30, 998–1004. doi: 10.1093/annonc/mdz108
Urago, Y., Okamoto, H., Kaneda, T., Murakami, N., Kashihara, T., Takemori, M., et al. (2021). Evaluation of auto-segmentation accuracy of cloud-based artificial intelligence and atlas-based models. Radiat. Oncol. 16:175. doi: 10.1186/s13014-021-01896-1
Verma, V., Simone, C. B., Krishnan, S., Lin, S. H., Yang, J., and Hahn, S. M. (2017). The rise of radiomics and implications for oncologic management. JNCI J. Natl. Cancer Inst. 109:djx055. doi: 10.1093/jnci/djx055
Wang, J., He, X., Bai, Y., Du, G., and Cai, M. (2022). Identification and validation of novel biomarkers affecting bladder cancer immunotherapy via machine learning and its association with M2 macrophages. Front. Immunol. 13:1051063. doi: 10.3389/fimmu.2022.1051063
Wu, Z., Pang, Y., Ming, Z., Liu, K., Wang, D., and Yan, J. (2021). Application of artificial intelligence technology in automatic delineation of organs at risk in nasopharyngeal carcinoma radiotherapy. Practical Oncol 35:13741. doi: 10.11904/j.issn.1002-3070.2021.02.007
Xie, X., Dou, C.-X., Luo, M.-R., Zhang, K., Liu, Y., Zhou, J.-W., et al. (2022). Plasma cell subtypes analyzed using artificial intelligence algorithm for predicting biochemical recurrence, immune escape potential, and immunotherapy response of prostate cancer. Front. Immunol. 13:946209. doi: 10.3389/fimmu.2022.946209
Xu, H., Cha, Y. J., Clemenceau, J. R., Choi, J., Lee, S. H., Kang, J., et al. (2022). Spatial analysis of tumor-infiltrating lymphocytes in histological sections using deep learning techniques predicts survival in colorectal carcinoma. J. Pathol. Clin. Res. 8, 327–339. doi: 10.1002/cjp2.273
Xu, B., Lu, M., Yan, L., Ge, M., Ren, Y., Wang, R., et al. (2021). A Pan-Cancer analysis of predictive methylation signatures of response to cancer immunotherapy. Front. Immunol. 12:796647. doi: 10.3389/fimmu.2021.796647
Yao, K., Liu, J., Wang, M., Wang, Q., Wu, H., Jiang, F., et al. (2022). Research on application of automatic contouring based on MRI image in cervical cancer. Med. Health Equip. 43, 50–62. doi: 10.19745/j.1003-8868.2022011
You, Y., Lai, X., Pan, Y., Zheng, H., Vera, J., Liu, S., et al. (2022). Artificial intelligence in cancer target identification and drug discovery. Signal Transduct. Target. Ther. 7:156. doi: 10.1038/s41392-022-00994-0
Yu, G., Wei, P., Chen, Y., and Zhu, M. (2017). Artificial intelligence in pathological diagnosis and assessment of human solid tumors: application and considerations. Acad. J. Second Mil. Med. Univ. 38, 134–140. doi: 10.16781/j.0258-879x.2017.11.134
Zanagnolo, V., Garbi, A., Achilarre, M. T., and Minig, L. (2017). Robot-assisted surgery in gynecologic cancers. J. Minim. Invasive Gynecol. 24, 379–396. doi: 10.1016/j.jmig.2017.01.006
Zarella, M. D., Bowman, D., Aeffner, F., Farahani, N., Xthona, A., Absar, S. F., et al. (2019). A practical guide to whole slide imaging: a white paper from the digital pathology association. Arch. Pathol. Lab Med. 143, 222–234. doi: 10.5858/arpa.2018-0343-RA
Zhang, Y., and Zhang, Z. (2020). The history and advances in cancer immunotherapy: understanding the characteristics of tumor-infiltrating immune cells and their therapeutic implications. Cell. Mol. Immunol. 17, 807–821. doi: 10.1038/s41423-020-0488-6
Zhang, N., Zhang, H., Liu, Z., Dai, Z., Wu, W., Zhou, R., et al. (2023). An artificial intelligence network-guided signature for predicting outcome and immunotherapy response in lung adenocarcinoma patients based on 26 machine learning algorithms. Cell Prolif. 56:e13409. doi: 10.1111/cpr.13409
Zhao, X., Yang, V. B., Menta, A. K., Blum, J., Wahida, A., and Subbiah, V. (2024). Alpha Fold’s predictive revolution in precision oncology. AI Prec. Oncol. 1, 160–167. doi: 10.1089/aipo.2024.0010
Zhu, Z., Harowicz, M., Zhang, J., Saha, A., Grimm, L. J., Hwang, E. S., et al. (2019). Deep learning analysis of breast MRIs for prediction of occult invasive disease in ductal carcinoma in situ. Comput. Biol. Med. 115:103498. doi: 10.1016/j.compbiomed.2019.103498
Keywords: artificial intelligence, tumor, pathological diagnosis, image recognition, precision medicine, therapy
Citation: Sun F, Zhang L and Tong Z (2025) Application progress of artificial intelligence in tumor diagnosis and treatment. Front. Artif. Intell. 7:1487207. doi: 10.3389/frai.2024.1487207
Received: 28 August 2024; Accepted: 19 December 2024;
Published: 07 January 2025.
Edited by:
Tim Hulsen, Rotterdam University of Applied Sciences, NetherlandsReviewed by:
Sunyoung Jang, The Pennsylvania State University, United StatesCopyright © 2025 Sun, Zhang and Tong. This is an open-access article distributed under the terms of the Creative Commons Attribution License (CC BY). The use, distribution or reproduction in other forums is permitted, provided the original author(s) and the copyright owner(s) are credited and that the original publication in this journal is cited, in accordance with accepted academic practice. No use, distribution or reproduction is permitted which does not comply with these terms.
*Correspondence: Zhongsheng Tong, dG9uZ3pob25nc2hlbmdfb25AMTYzLmNvbQ==
Disclaimer: All claims expressed in this article are solely those of the authors and do not necessarily represent those of their affiliated organizations, or those of the publisher, the editors and the reviewers. Any product that may be evaluated in this article or claim that may be made by its manufacturer is not guaranteed or endorsed by the publisher.
Research integrity at Frontiers
Learn more about the work of our research integrity team to safeguard the quality of each article we publish.