- 1Formerly at Laboratory of Studies and Research in Management of Organizations and Territories (ERMOT), Faculty of Legal, Economic, and Social Sciences of Fez, Sidi Mohamed Ben Abdellah University, Fez, Morocco
- 2Laboratory of Research in Economy and Management of Organisations (LAREMO), National School of Business and Management of Beni Mellal, Sultan Moulay Slimane University, Beni Mellal, Morocco
Introduction: The adoption of artificial intelligence (AI) in the workplace is changing the way organizations function, and profoundly affecting employees. These organizational changes raise crucial questions about the employee’s future and well-being. Our study aims to explore the intersection between artificial intelligence and employee well-being through a bibliometric review and a contextual analysis.
Methodology: Carried out in May 2024, our study is divided into two phases. The first phase, dedicated to bibliometric review, was conducted using the PRISMA method, and explored the Scopus and Web of Science databases for the period from 2015 to 2024. A total of 92 articles were selected for quantitative analysis using VOSviewer software. The second phase is based on an in-depth systematic analysis of 25 articles selected from those previously identified. These articles were selected on the basis of their relevance to the research question, and were subjected to in-depth thematic analysis using NVivo software.
Results: The bibliometric analysis results reveal a significant increase in publications starting from the year 2020, highlighting advancements in research, primarily in the United States and China. The co-occurrence analysis identifies four main clusters: ethics, work autonomy, employee stress, and mental health, thus illustrating the dynamics created by artificial intelligence in the professional environment. Furthermore, the systematic analysis has brought to light theoretical gaps and under-explored areas, such as the need to conduct empirical studies in non-Western cultural contexts and among diverse target groups, including older adults, individuals of different sexes, people with low education levels, and participants from various sectors, including primary and secondary industries, small manufacturing businesses, call centers, as well as public and private healthcare sectors.
Conclusion: Existing literature emphasize the importance for organizations to implement supportive strategies aimed at mitigating the potential adverse effects of AI on employee well-being, while also leveraging its benefits to enhance workplace autonomy and satisfaction and promote AI-enabled innovation through employee creativity and self-efficacy.
1 Introduction
Employee well-being is a broad and multidisciplinary concept that encompasses both tangible aspects such as income and social benefits, and intangible aspects related to a sense of belonging, satisfaction, and motivation (Rath and Harter, 2010). These elements significantly influence not only the overall performance of employees but also their personal, family and social fulfillment (Chari et al., 2018). As with any societal change driven by technology, employee well-being is inevitably affected (Nazareno and Schiff, 2021). The shift toward artificial intelligence (AI) is reshaping not just the future of businesses but also the work environments and experiences of employees. The automation of tasks, replacement of the workforce, disappearance of certain jobs, reallocation of skills, and the need to acquire new competencies pose substantial challenges in today’s workplace (Didem and Anke, 2021; Nazareno and Schiff, 2021; Peters, 2017). The impact of AI on professional settings is complex and varied; while it allows some employees to focus on strategic and reflective tasks, freeing them from monotonous and repetitive activities, others view these changes with concern, fearing job loss and instability (Hagel et al., 2018; Stamate et al., 2021).In summary, while AI can enhance employee well-being by improving job satisfaction, overall health, and reducing stress levels, it also presents significant challenges that can adversely affect the workforce. This article explores the dynamic interconnection between artificial intelligence (AI) and employee well-being through a combined methodological approach that includes a bibliometric review and a systematic review. Utilizing data from the Scopus and Web of Science databases, we have selected 92 articles for the bibliometric analysis to identify current trends, recognize influential authors, and outline the main research axes. To enhance this analysis, we conducted a systematic review based on specific inclusion criteria outlined in our methodology section, selecting 25 articles that further our understanding of the applied theories, identify existing theoretical gaps, and highlight potential directions for future research. Our investigation is guided by several crucial questions:
• What is the publication trend of AI & Employee well-being?
• Which countries have contributed to AI & Employee well-being?
• Who are the top-cited authors in the field of AI & Employee well-being?
• Which journals are leading in the field of AI & Employee well-being?
• What are the most used keywords in AI & Employee well-being?
• What are the major theoretical gaps identified in the literature on AI & Employee well-being?
• What future research directions are suggested in the field of AI & Employee well-being?
• What theories have been most frequently utilized to study AI & Employee well-being?
The findings from this study are set to serve as a foundational for future researchers interested in the multifaceted role of AI in professional settings. Additionally, the insights provided will empower decision-makers, especially human resource managers, with a deeper understanding of AI’s dimensions. This knowledge will enable them to use AI not as a disruptor but as a tool to enhance employee performance and well-being.
In the following sections, the article will detail the methodology used for gathering and analyzing data. Subsequently, we will discuss the results of both the bibliometric and content analyses. Finally, the discussion will synthesize these findings, interpreting their implications for both theory and practice, and conclude with a summary of the research contributions and recommendations for future studies.
2 Methods
To address our research questions, we adopted a method combining both a bibliometric review and a systematic review. The bibliometric review enabled a quantitative analysis of the data, focusing particularly on the number of publications per year, the most influential authors, the most relevant journals, and a co-occurrence analysis of keywords. In complement, the systematic review provided an in-depth analysis of the selected articles, allowing us to identify theoretical gaps, suggest future research perspectives, and examine the methodologies employed in the different studies. The data used for this analysis were extracted in May 2024 from Scopus and Web of Science, which are widely recognized for their comprehensive coverage and reliability (Chadegani et al., 2013). To ensure the rigor of the article selection process, we followed the PRISMA method, known for its capacity to enhance transparency and the rigor needed to conduct a literature review (Liberati et al., 2009) (see Figure 1).
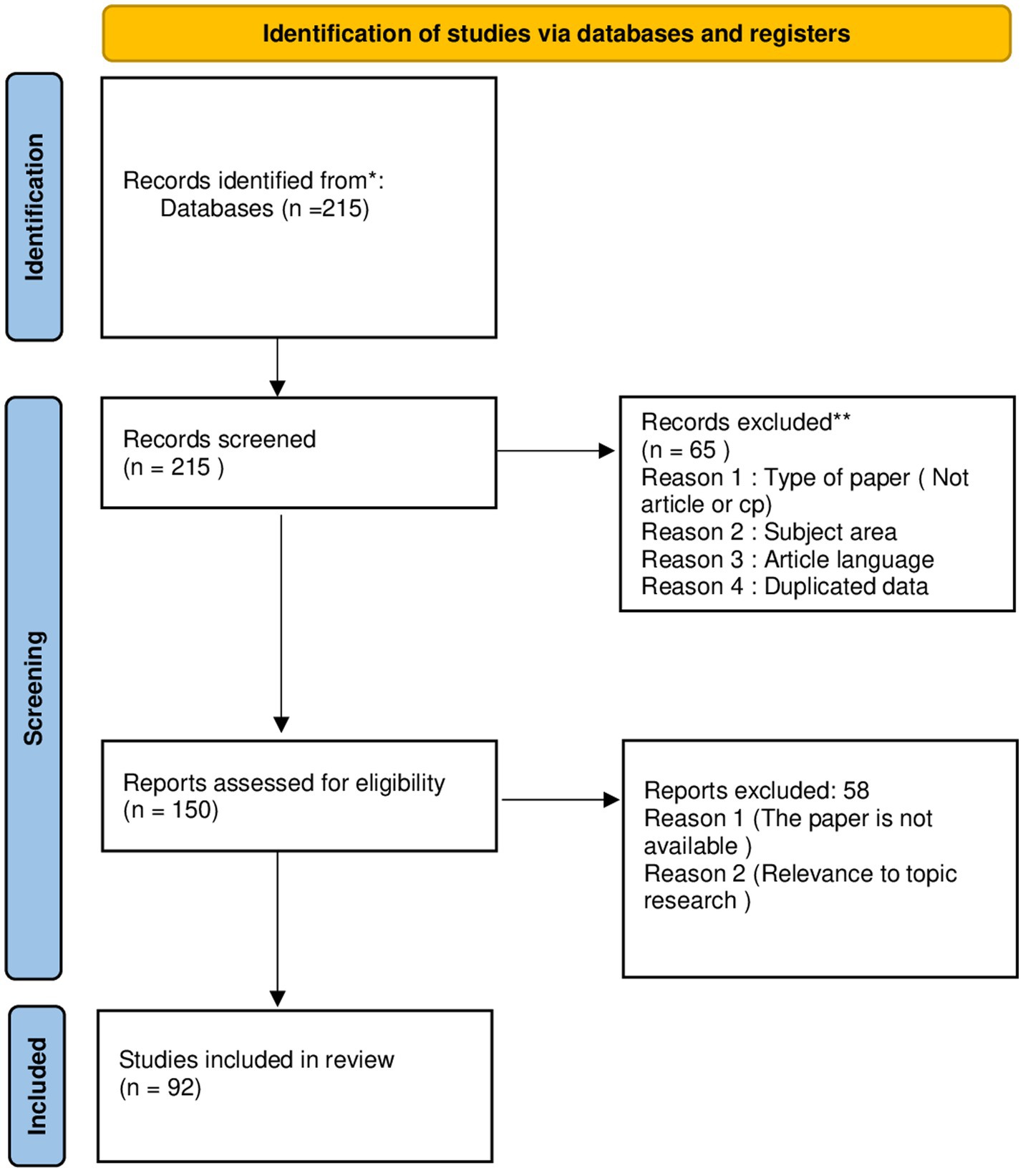
Figure 1. Research steps. The prisma website as a supporting source for the preparation of the diagram. https://www.prisma-statement.org/prisma-2020-flow-diagram.
The search query was formulated as follows: (“artificial intelligence” OR “AI”) AND (“employee well-being” OR “employee health” OR “workplace stress” OR “employee satisfaction”) AND (LIMIT TO (DOCTYPE, “ar”)) OR LIMIT-TO (DOCTYPE, “cp”)) AND (LIMIT-TO (LANGUAGE, “English”). This search query allowed us to select 92 articles, which were subsequently subjected to quantitative analysis using VOSviewer (version 1.6.20). VOSviewer is particularly suited for processing bibliographic data and creating graphical representations that help visualize the relationships between the studied concepts (Eck and Waltman, 2009). In the context of our analysis, we used this software to study the evolution of publications over time, identify the most influential authors, analyze the distribution of publications by journal, and perform a co-occurrence analysis of keywords. After importing the bibliographic data in RIS format into VOSviewer, we set the keyword frequency threshold to three occurrences, meaning that only keywords appearing at least three times in the articles were included in the analysis. A data-cleaning process was then carried out to remove redundant or similar keywords that could skew the results, such as “human” and “humans,” or “AI” and “artificial intelligence.” Similarly, overly similar terms like “worker” and “employee” were harmonized to ensure consistency in the analyzed data. The results from this analysis will be discussed in detail in the results section of this study.
Regarding the systematic review, we conducted a thorough and detailed qualitative analysis of the articles related to our research domain. From the 92 articles obtained from the bibliometric review, we refined the selection to retain only 25 articles. The articles selected for our systematic review meet strict inclusion criteria, requiring the presence of terms ‘Artificial Intelligence’ or equivalent expressions, ‘Employee Well-being’, ‘Job Satisfaction’, or similar expressions in the title, abstract, or keywords. Additionally, exclusion criteria were applied to eliminate articles that did not directly address artificial intelligence or aspects related to well-being, satisfaction, or health of employees. To strengthen our selection process, minimize research errors, and ensure a rigorous analysis, the three authors collaborated closely to evaluate each article. The analysis of the articles was carried out using the Nvivo14 software, which allowed us to organize and qualitatively analyze the data. The analysis process followed a five-step protocol, which will be detailed in the “Results” section. First, we generated a word cloud to visualize the most frequently used terms. Next, we created a word tree to explore the hierarchical relationships between the key concepts in the articles. Third, we examined the methodology adopted by each study. Fourth, we focused our analysis on the theories mobilized in the studies. Out of the 25 selected articles, we retained 15 for this step, excluding 10 articles that were limited to literature reviews. Finally, to create a hierarchical diagram in Nvivo, we imported the full text of the 25 articles into the software. During the reading process, we created several nodes (or codes) representing the main axes of research perspectives, such as “exploration of new research questions,” “adoption of alternative methodological approaches,” or “conducting empirical studies in other sectors.” Each node was then subdivided into sub-nodes, where we encoded the relevant parts of the text from each article.
3 Results
In alignment with the research steps previously outlined, this section is divided into two subsections. The first subsection discusses the findings of the bibliometric analysis, covering aspects such as the trends in publication years, the most influential authors, key journals, and clusters for keyword co-occurrence. The second subsection delves into the content analysis results, presenting elements such as word clouds, word trees, the methodologies employed by researchers, the theories utilized, the sectors examined, the themes explored, and the principal findings. This subsection also highlights the theoretical gaps identified and outlines future research directions.
3.1 Bibliometric review
3.1.1 Annual publication
This bar chart (Figure 2) illustrates the number of publications per year from 2015 to 2024, showing a clear increase in activity over time. Initially, the number of publications was relatively low between 2015 and 2018, but it began to rise significantly in 2020. This increase can be attributed to the COVID-19 pandemic (Kniffin et al., 2021), which caused substantial changes in the way businesses operate and had a profound impact on employee well-being. At the same time, the Fourth Industrial Revolution, characterized by the growing adoption of artificial intelligence, led to significant transformations in employment structures and work practices, redefining the future of work (Stamate et al., 2021).
3.1.2 Publications per authors
The third figure illustrates the contributions of the 10 most prolific authors in the field of artificial intelligence and employee well-being. Although the field is still in its early stages, with a maximum of two publications per author, the citation analysis reveals their significant influence within the scientific community. Particularly notable are De Cremer with 12,516 citations, followed by Bromuri with 740 citations, and Byung-Jik Kim with 339 citations (Figure 3).
3.1.3 Publication per countries
The fourth figure illustrates the countries that have contributed the most to research in this field. We notice a predominance of the United States and China, followed by European countries and the United Kingdom. South Africa and Saudi Arabia are also present, and contributions from other countries such as Malaysia, Canada, Hungary, and Morocco are observed. This distribution shows a global interest in research and underscores the need to increase research efforts in other countries where contributions are reduced or non-significant, such as Taiwan, Belgium, Turkey, and Japan (Figure 4).
3.1.4 Keywords clusters
Keywords form the foundation of published articles and illustrate the diversity of research within the studied field (Su and Lee, 2010). Through co-occurrence analysis, it is possible to identify emerging themes and analyze the interdependence between different fields of study (Chiang et al., 2023). Our keyword co-occurrence analysis has identified four main clusters that illustrate the relationship between artificial intelligence (AI) and employee well-being. Figure 5 shows the obtained visualization. The biggest number of keywords was 10, while the lowest was five (see Table 1).
The yellow cluster focuses on artificial intelligence and its various forms such as machine learning and automation highlighting ethical considerations and employability. Artificial intelligence and machine learning have transformed our way of life and our way of working (Ortega-Bolaños et al., 2024) and will continue to bring significant changes in the years to come (Sanderson et al., 2023). Like any powerful technological tool the adoption of artificial intelligence within companies raises important ethical questions (Trotta et al., 2023). These ethical issues touch on various aspects such as transparency fairness data confidentiality and accountability (Ortega-Bolaños et al., 2024; Trotta et al., 2023)
Indeed, the success of an AI-based project first requires transparency; a transparent project is one that is clearly explained, understood by all stakeholders, and motivating (Morley et al., 2020). A project must also be fair, ensuring that all employees have equal opportunities and are not disadvantaged by the deployment of AI technologies (Ortega-Bolaños et al., 2024). Authors (Bollier, 2017; Gibbons, 2021) suggest that widespread internet access and the implementation of a universal basic income could help address issues related to fairness, particularly for employees who perceive the adoption of artificial intelligence in the workplace negatively. Moreover, the use and storage of data present significant ethical challenges. Every individual should have the right to manage and control their data, ensuring privacy and preventing misuse (Khan et al., 2021). Finally, accountability is a crucial pillar in the management of artificial intelligence. Accountability assigns responsibility to the company or individuals for the outcomes produced by AI systems (Benneh, 2023). In sum, these ethical considerations provide a framework for better managing the implementation of artificial intelligence in the future (Sanderson et al., 2023; Benneh, 2023).
Concerning the red cluster, it refers to the psychological and health concerns of employees in the workplace. Indeed, the emergence of artificial intelligence within companies has disrupted the future of work (Fisher et al., 2023). While AI can offer countless benefits to employers and employees, such as improved productivity and performance, its implementation can also have negative impacts. Employees with gaps in digital skills may find themselves at a disadvantage, creating social disparities and increasing the risk of job loss (Landsbergis et al., 2014). According to some studies, the fear of job loss and the stress related to acquiring new skills are considered a public health crisis (Case and Deaton, 2017). To address this and promote equity by creating a stress-free work environment for employees, several tools need to be put in place. These include continuous training for employees to bridge skill gaps, considering the cultural, societal, and environmental aspects by employers, and placing humans at the center of attention (Gibbons, 2021).
The green cluster highlights the role of digital transformation and COVID-19 on employee engagement, performance, and satisfaction. The digital transformation, accelerated by the COVID-19 pandemic, has revolutionized the world of work and business (Schilirò, 2021). The adoption of new technologies, such as artificial intelligence, creates job insecurity and affects employee engagement and performance (Ye et al., 2024; Hizam et al., 2023). To address this, acquiring new skills and improving existing ones among employees is a key element, enabling companies to enhance their competitiveness and equip employees with the necessary tools to support the change (Morandini et al., 2023; Li, 2022). Furthermore, artificial intelligence impacts not only performance and satisfaction but also managerial HR practices (Faye-Schjøll and Høye, 2020). The use of AI in human resources allows for anticipating employee departures and identifying factors contributing to their stress and dissatisfaction (Faye-Schjøll and Høye, 2020). AI also provides platforms that foster employee engagement and a sense of belonging. These tools create an attractive, collaborative work environment and drive business growth (Bhatia, 2018).
The blue cluster in the network visualization highlights the complex relationship between technology adoption in workplaces and its effects on employee, on autonomy and on health, revealing both opportunities and challenges. The integration of advanced technologies such as AI and automation often shifts employees from routine tasks to more complex and intellectually stimulating roles (Khogali and Mekid, 2023), which can significantly enhance job satisfaction and operational efficiency (Shwedeh et al., 2023; Shwedeh et al., 2023). However, this transition also introduces significant mental health risks, including job security stress and the pressure to quickly acquire new skills (Ghani et al., 2022) To effectively navigate these challenges, organizations must adopt a balanced approach by implementing comprehensive training programs that not only strengthen employees’ skills but also prepare them psychologically for technological changes (Morandini et al., 2023). Additionally, supporting employee well-being through accessible mental health resources, such as counseling and stress management workshops, and promoting policies that encourage work-life balance is crucial for alleviating work-related stress and preventing burnout (Bello et al., 2024). Furthermore, maintaining ethical standards in deploying new technologies by ensuring transparency, fairness, and data confidentiality is essential to build trust and foster an inclusive and supportive work environment (Potter and Doris, 2024). Collectively, these strategies can help organizations leverage technological advancements to improve productivity while simultaneously ensuring that employee autonomy and health are protected and promoted.
3.2 Content analysis
3.2.1 Word cloud and clusters of the most frequently used words
To conduct the word frequency analysis, we used Nvivo software. The full text of the 25 selected articles was imported into the software, and the abstracts and keywords were manually encoded into a node titled “Abstracts and Keywords.” A word frequency query was then executed, and the “word cloud” option was selected to generate a graphical representation of the 100 most frequently used words in the articles. Figure 6 illustrates the most commonly used words in the abstracts and keywords of the 25 selected articles. Words appearing in larger, bold fonts, such as “employee,” were used more frequently compared to smaller words. The term “employee” stands out as a key word in the analysis. Employees are the primary actors in the digital transformation of companies. Their level of motivation, acceptance of change, and engagement are critical factors that can significantly influence the success of a company’s digital transition (Ye et al., 2024).
To create the word cluster analysis, we also used Nvivo software. The full text of the 25 selected articles was integrated, excluding the abstracts and keywords. We then ran a “Word Frequency” query, with the results visualized as clusters to display the connections between frequently occurring words in the articles. Figure 7 illustrates the results, highlighting key associations between specific terms. For instance, the words “psychological” and “need” appeared together in a sentence, as did “career” and “collaboration.” Other notable associations include “experience” with “negative,” “employee” with “performance,” “business” with “engagement,” and “ethical” with “impact.” The pair “innovation” and “creative” is also of interest, appearing after “intelligence” and before “behavior.” Furthermore, the terms “support” and “help” often appeared together, preceded by “organizational,” while “automation” and “factors” were frequently preceded by “satisfaction.”
3.2.2 The methodological approaches used by the authors
In order to identify the methodological approaches used in the articles, we first conducted an in-depth reading of the papers, followed by manually categorizing the articles in an Excel table according to the approaches employed (see Figure 8). This analysis allowed us to identify three main methodological approaches adopted by the authors. First, literature reviews in various forms were undertaken by 10 articles: narrative review (Khogali and Mekid, 2023; Alavi and Habel, 2021; Bankins, 2021; Chang, 2020), systematic review (Cramarenco et al., 2023; Mer and Virdi, 2023; Mera and Srivastavab, 2023; Zhang et al., 2022) and descriptive and exploratory literature review (Gorovei, 2020). Second, the quantitative approach was employed in 12 articles. For instance, some authors (Stamate et al., 2021; Jeong et al., 2024; Jiang et al., 2022; Kinowska and Sienkiewicz, 2022; Yin et al., 2024) conducted research using Structural Equation Models (SEM), while others (Nazareno and Schiff, 2021; Henkel et al., 2020; Kong et al., 2023; Xiao et al., 2023) performed regression analyses. Lastly, the mixed-methods approach was adopted by 3 articles. For example (Brougham and Haar, 2018) analyzed an open-ended question within a quantitative questionnaire, while (Konuk et al., 2023; Correia Loureiro et al., 2023) conducted semi-structured interviews followed by a quantitative study.
3.2.3 Theoretical frameworks mobilized by authors
To create the table presenting the theories utilized in the various articles, we thoroughly read all 15 articles (from the 25 initially selected, we excluded 10 that conducted literature reviews and kept only 15 that carried out empirical studies, whether qualitative, quantitative, or mixed). We manually filled out the table, counting the number of articles in which each theory was applied, and specifying the authors of those articles. We identified 20 theoretical frameworks used across these articles, which we synthesized in Table 2. Interestingly, only the first four theoretical frameworks were discussed in two or more articles, while all other theories were employed by different authors without repetition across other studies.
3.2.4 Business sectors covered by empirical studies
Similarly, for sectoral analysis, we reviewed the 15 empirical studies and created a table showing the percentage of studies focused on various sectors. Of these, 8 conducted research in varied sectors, while 7 targeted specific ones. A bar chart was created to represent the distribution of sectors across the 7 targeted studies (see Figure 9).
3.2.5 Topics covered and main results
In this section, we will outline the primary themes explored and the key findings reported by the authors through narrative descriptions. It’s noteworthy that while a few articles may be categorized thematically in pairs or small groups, the majority of articles introduce unique themes not revisited in subsequent works. This characteristic underscores the subject’s recent emergence and the field’s wide-open terrain, ripe for further investigations aimed at validating or challenging earlier findings. With the exception of a few unique cases, the analyzed articles can be categorized into five thematic groups. Firstly, a set of articles focuses on employees’ attitudes and perceptions regarding the opportunities and threats posed by AI, and how these perceptions impact their workplace well-being. Regarding employees’ attitudes and perceptions of the opportunities and threats posed by AI, the findings are not homogeneous. Some studies highlight that general skepticism toward job displacement from automation is prevalent, with greater awareness of STARA (Smart Technology, Artificial Intelligence, Robotics, and Automation) correlating with reduced organizational commitment and career satisfaction, as well as higher turnover intentions, depression, and cynicism among employees (Brougham and Haar, 2018). Other findings indicate that while job-replacement anxiety does not significantly impact psychological well-being (PWB), AI learning anxiety is associated with decreased PWB (Konuk et al., 2023). Conversely, some results show a positive link between the perception of AI opportunities and workplace well-being (WWB), partially mediated by informal learning in the workplace (ILW), with unemployment risk perception (URP) moderating this relationship (Xu et al., 2023). Trust in AI enhances both employee well-being and supervisor-rated productivity through improved employee-AI collaboration, particularly among those with high protean career orientations (Kong et al., 2023). Overall, general mental ability (GMA) shapes perceptions of AI’s usefulness, which affects attitudes toward the technology and ultimately influences employee satisfaction, the fulfillment of basic psychological needs, and overall psychological health (Stamate et al., 2021). Secondly, another group addresses the effects of AI and automated technologies on employee well-being, particularly emphasizing job stress and its negative impact on physical and mental health, as well as employees’ behavioral responses to this stress. Thus, the adoption of automated technologies at work consistently affects worker well-being in terms of job stress and overall health, with these effects being more pronounced in jobs at higher risk of automation (Nazareno and Schiff, 2021). Also, there is a strong association between AI adoption, heightened job stress, negative impacts on physical health, and the significant moderating effect of coaching leadership in alleviating AI-related stress (Jeong et al., 2024). Benign stress from AI adoption does not directly affect employee happiness but does so indirectly through employee engagement, which positions engagement as a key mediator between AI adoption and overall well-being (Correia Loureiro et al., 2023). Furthermore, employees with higher anxious attachment are more sensitive to AI interaction and may react by maladapting behavior by isolating themselves, experiencing insomnia or increased alcohol consumption due to their need for affiliation and feelings of loneliness (Tang et al., 2023). Providing service employees with AI tools for emotion recognition may initially raise stress levels, but also benefit well-being by raising employees’ sense of goal achievement through enhancing their ability to manage customer emotions (Henkel et al., 2020). A third group examines the contentious impact of AI adoption on job autonomy and its subsequent effects on employee well-being. The findings indicate that AI adoption promotes workplace flexibility, employee confidence, and job autonomy, all of which positively contribute to Employee Mental Health and Well-Being (EMHWB) (Shaikh et al., 2023). Conversely, the application of automated and algorithmic management practices significantly affects job autonomy, leading to indirect and potentially undesirable effects on well-being (Kinowska and Sienkiewicz, 2022). Some other articles investigate the positive influence of AI adoption on employee well-being through enhanced self-efficacy. Smart technology plays a crucial role in enhancing employee self-efficacy, which in turn has a positive influence on their overall well-being (Jiang et al., 2022). AI assistants with high intelligence not only indirectly boost employees’ AI-enabled innovation behaviors by enhancing creative self-efficacy—an effect that is amplified in organizations with greater AI readiness—but they can also have a negative impact on these behaviors through increased awareness of Smart Technology, Artificial Intelligence, Robotics, and Algorithms (STARA), particularly when organizational readiness for AI is lower (Yin et al., 2024). Lastly, a final group explores how AI contributes to tailoring workstations to meet employees’ needs, thereby improving their well-being. The evolution of the Internet-of-Things (IoT) and artificial intelligence has significantly contributed to the development of smart offices capable of understanding employees’ contexts and adapting to their needs (Zhang et al., 2022). Furthermore, job crafting mediates the positive impact of AI-enabled HR analytics on enhancing employee resilience, which, as we know, leads to employee well-being, while there is a positive moderating effect of HRM system strength on the link between AI-enabled HR analytics and job crafting (Xiao et al., 2023). The results discussed above primarily focus on the impact of AI use on employee well-being, as this constitutes the central research question of the paper. However, it is worth extending this inquiry to explore the potential effects of AI on employee productivity and performance. Consequently, in the following paragraph, we will briefly outline the key findings from the articles analyzed that address these additional dimensions, providing a more comprehensive understanding of AI’s broader organizational implications. Indeed, the question of how AI affects employee performance or productivity adds another important dimension to the discussion of well-being. For example, AI adoption promotes workplace flexibility, employee trust and autonomy, all of which contribute positively to EMHWB and, consequently, employee productivity (Shaikh et al., 2023). In addition, trust in AI positively influences supervisor-assessed productivity through improved collaboration between employees and AI (Kong et al., 2023). In terms of employee performance, smart technologies have a significant impact on company trust, self-efficacy, employee well-being and learning performance (Jiang et al., 2022). At the same time, the application of automated and algorithmic management practices significantly affects professional autonomy, with indirect and potentially undesirable effects on well-being and performance (Kinowska and Sienkiewicz, 2022).
3.2.6 Research perspectives
Through an in-depth analysis of existing literature and using the Nvivo14 software, we have identified several research perspectives that can provide avenues for future studies on employee well-being and artificial intelligence. Figure 10 depicts a hierarchical diagram illustrating research perspectives identified through thematic analysis conducted with Nvivo software, we examined the “discussion of results and research perspectives” sections from all 25 articles. Major research perspectives were encoded into nodes (e.g., “asking other research questions” “adopting other methodological approaches“). Within each node, sub-nodes were created to capture more detailed suggestions. Using Nvivo’s ability to count and visualize references (articles encoded in each node), we identified similarities across the articles in terms of suggested research perspectives. Several articles mentioned the same research perspectives, such as the need for empirical studies in other countries (8 articles) or studies targeting other groups (7 articles). Once the encoding was complete, we performed a “Hierarchical Diagram” query, which visually mapped the distribution of research perspectives across the articles. The size of each rectangle in the diagram represented both the number of articles addressing a particular perspective and the volume of text devoted to it. Larger rectangles indicated greater emphasis on those perspectives. For instance, the need for posing additional research questions emerged as a key focus, as seen by the prominence of its corresponding rectangle in the diagram. This visual representation allowed for an intuitive understanding of which research perspectives were prioritized across the literature. While Nvivo does offer the ability to generate diagrams that include both nodes and sub-nodes, the result was overly complex and hard to interpret. To address this, we refined the diagram manually using Word, applying graphic elements to incorporate key sub-nodes (or sub-themes) into the corresponding rectangles. This approach helped to clarify, for example, which additional research questions were proposed by the authors or which new target groups were suggested for future empirical studies. The goal was to create a clear, concise visual summary, allowing readers to quickly grasp the main points before engaging with the detailed text that followed. In the upcoming text, we will elaborate on the various research perspectives referenced by the authors of the 25 selected articles.
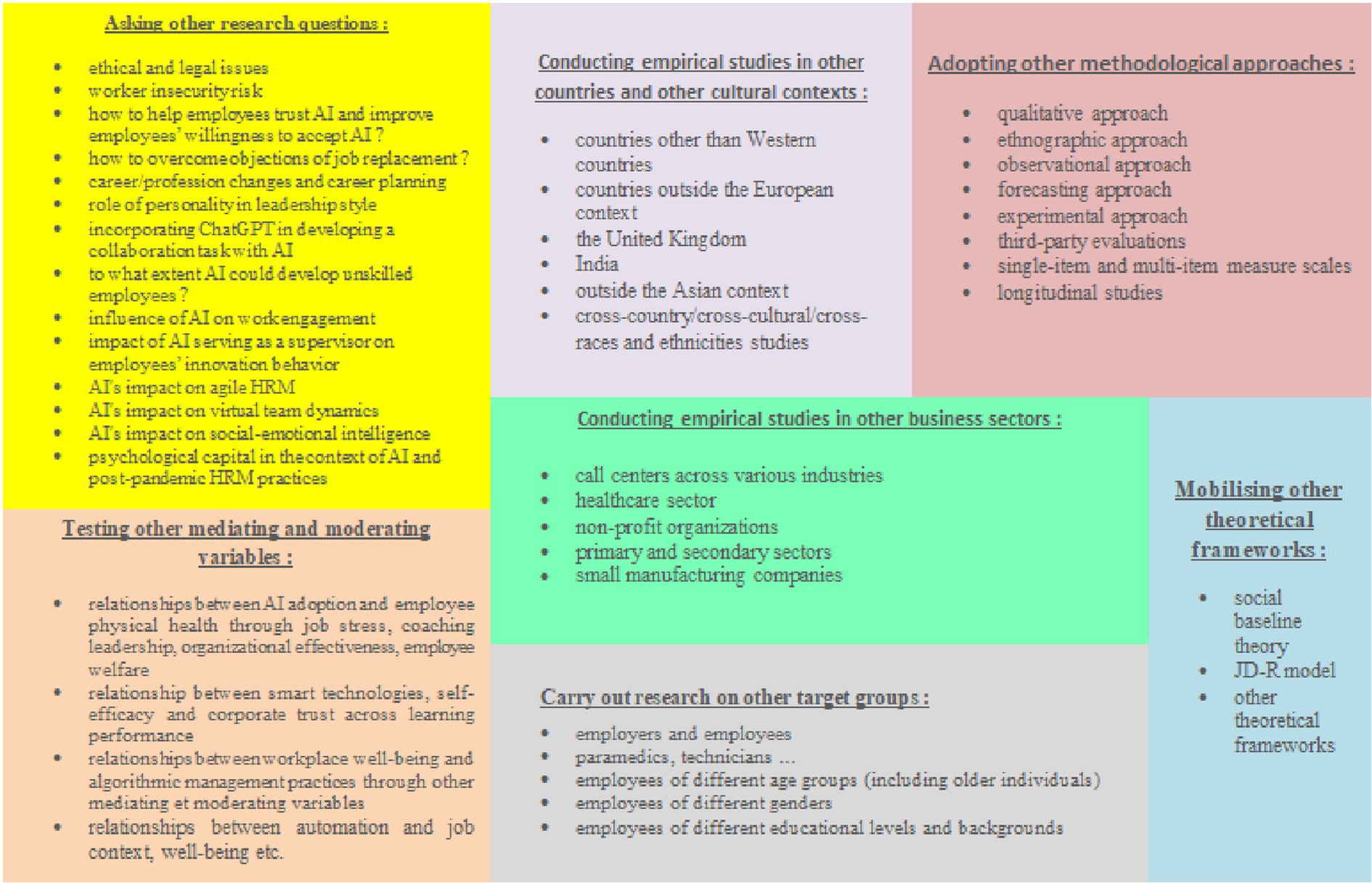
Figure 10. Hierarchical diagram of research perspectives based on thematic analysis. Source: Output of Nvivo 14 Software revised by us.
3.2.6.1 Testing other moderating and mediating variables
Most quantitative empirical studies suggest further research to test additional mediating and moderating variables within their proposed conceptual models, such as job crafting, cognitive reappraisal, self-esteem (Xu et al., 2023) or psychosomatic well-being, cognitive and professional elements (Cramarenco et al., 2023).
3.2.6.2 Conducting empirical studies in other countries and other cultural contexts
Indeed, there is a need for more comprehensive research to encompass other countries, especially beyond the European context (Stamate et al., 2021; Henkel et al., 2020). Similarly, it is important to conduct studies in cultural settings beyond China and other Asian countries, particularly focusing on regions such as the United Kingdom and India (Kong et al., 2023). Furthermore, there is a necessity for cross-country and cross-cultural studies (Kong et al., 2023; Correia Loureiro et al., 2023), as well as research examining different races and ethnicities (Nazareno and Schiff, 2021).
3.2.6.3 Mobilizing other theories and theoretical frameworks
From the perspective of social baseline theory, interactions with AI in the workplace may be seen as a form of “relationship disruption” which can trigger a social-regulatory process marked by heightened uncertainty and perceived risk, leading individuals to actively work toward preserving social relationships (Tang et al., 2023). Future research on the impact of AI on work engagement, as well as the investigation of the dual effects of AI-assisted intelligence on employees’ innovation behaviors, could benefit from using the JD-R model as a foundational framework (Mera and Srivastavab, 2023); (Yin et al., 2024). Additionally, future studies might gain from integrating additional theoretical frameworks that account for the myriad factors influencing employee productivity, such as organizational culture and leadership styles (Shaikh et al., 2023).
3.2.6.4 Carry out research on other target groups
Research on workplace well-being should integrate perspectives from both employers and employees, as well as other relevant stakeholders (Kinowska and Sienkiewicz, 2022). Additionally, the inclusion of healthcare employees, alongside patients, is crucial to fully understanding well-being in the healthcare sector (Mera and Srivastavab, 2023). To generalize findings, it is important to expand studies beyond doctors to include paramedics, technicians, and other support staff (Shaikh et al., 2023). Given the prevailing emphasis on younger populations in existing research, it is essential to explore the perceptions of older participants and compare behaviors across various age groups (Stamate et al., 2021; Correia Loureiro et al., 2023). Considering the complex socio-technical dynamics associated with automation and AI, studies should also examine gender differences (Nazareno and Schiff, 2021). Moreover, individuals with a background in technology or engineering, along with higher levels of knowledge and skills, may experience enhanced collaboration with AI (Ahn et al., 2022; Oksanen et al., 2020). Therefore, it is vital to diversify research samples to include individuals with lower educational backgrounds, moving beyond the predominant focus on well-educated groups (Yin et al., 2024; Xu et al., 2023).
3.2.6.5 Adopting other methodological approaches
Comprehensive research employing a diverse range of qualitative and quantitative methods—such ethnographic, survey, observational, forecasting, and experimental approaches—is essential (Nazareno and Schiff, 2021). Furthermore, to mitigate common method bias and enhance reliability, researchers should incorporate third-party evaluations alongside self-report measures (Xu et al., 2023). Moreover, to reduce response biases, it is crucial to utilize robust measurement techniques with higher content validity, including multi-item scales in addition to single-item measures (Stamate et al., 2021). Longitudinal studies are also necessary to capture changes over time (Henkel et al., 2020; Xiao et al., 2023; Brougham and Haar, 2018; Konuk et al., 2023).
3.2.6.6 Conducting empirical studies in other business sectors
Studying the effects of AI in various industries and service settings is crucial (Nazareno and Schiff, 2021). The healthcare sector emerges as a particularly promising area for AI adoption, especially considering the vulnerabilities highlighted during the COVID-19 pandemic (Mera and Srivastavab, 2023). Comparative analyses between public and private hospitals could deepen our understanding of AI’s role in healthcare (Shaikh et al., 2023). Additionally, research in call centers, including non-profit organizations like municipalities, is vital due to differing operational dynamics (Konuk et al., 2023). Future studies should also include employees from both primary and secondary sectors (Brougham and Haar, 2018). Lastly, examining small manufacturing companies is essential, as workers in these settings may face unique challenges associated with AI, often stemming from lower education levels and a lack of necessary skills and resources (Xiao et al., 2023).
3.2.6.7 Asking other research questions
Future research on AI-enabled HR analytics should not only focus on its positive impacts but also address potential risks and challenges, including ethical and legal issues as well as negative employee experiences (Xiao et al., 2023). Investigating ethical norms in the workplace context of AI use is essential, particularly regarding the implications of job losses and gains amid rapid technological advancements (Khogali and Mekid, 2023). The unresolved issue of worker insecurity due to automation warrants further investigation, as it could significantly affect employee well-being (Nazareno and Schiff, 2021).
Moreover, while concerns about job displacement are prevalent, AI is becoming an integral part of future workplaces. Research should emphasize educating and training employees on AI applications to build trust and alleviate fears of job loss (Correia and Matos, 2021). Strategies that enhance employee trust in AI and facilitate acceptance of its presence in the workplace could also be explored, particularly regarding how AI technologies impact work-life balance (Kong et al., 2023). Additional research questions may include examining the effects of AI on cognitively demanding jobs and identifying management strategies to empower employees in their interactions with AI rather than fostering feelings of threat (Chang, 2020). Although there is a concern about job displacement due to Smart Technology and Advanced Robotics Applications (STARA), it is likely that new job categories will emerge, necessitating studies on career planning and the potential for workforce transitions (Brougham and Haar, 2018). In the healthcare sector, effective management of AI through technological leadership remains a challenge, highlighting the need for studies on the influence of leadership personality on management styles (Shaikh et al., 2023). Scholars could also consider integrating open AI systems, such as ChatGPT, into collaborative tasks (Tang et al., 2023). Future investigations should assess how AI, automation, and digitalization can enhance the skills of unskilled workers in advanced economies (Mer and Virdi, 2023). Furthermore, exploring AI’s impact on agile HRM, virtual team dynamics, social–emotional intelligence, and psychological capital within post-pandemic HRM practices is crucial (Mer and Virdi, 2023). Finally, research should delve into AI’s role in enhancing work engagement (Mera and Srivastavab, 2023) and examine the effects of AI as a supervisory entity on employees’ innovation behaviors (Yin et al., 2024).
4 Discussion
This article investigates how integrating artificial intelligence (AI) in businesses affects employee well-being. The integration of AI into professional environments has both positive and negative effects on employees as well as on the future of employment (Mera and Srivastavab, 2023). Our study has shown that the positive impacts of AI include the automation of repetitive and stressful tasks, along with improvements in flexibility, confidence, autonomy, and collaboration among employees (Shaikh et al., 2023). However, some employees view these changes negatively, fearing potential job loss and mental health issues, such as depression (Brougham and Haar, 2018).
To ensure successful AI integration, it is crucial to address several aspects. The ethical dimension is vital, encompassing transparency, fairness, data confidentiality, and accountability (Khogali and Mekid, 2023; Bankins, 2021). Moreover, ongoing training and coaching are necessary to reduce stress and alleviate fears of job loss among employees (Bello et al., 2024). These measures will help mitigate the negative effects of AI and enhance its management within the company.
Our systematic analysis has revealed several gaps in the current literature, which has led us to suggest new avenues for future research. We have identified the need to broaden the geographic scope of AI studies beyond the United States, Asia, and Europe, with a particular focus on less-researched sectors such as education and call centers. To gain a deeper understanding of AI’s impact on employee well-being, our findings indicate the necessity of employing diverse methodologies, including qualitative approaches and longitudinal studies. Our analysis also highlights the importance of exploring how various demographic groups, taking into account age, sex, gender, and culture, perceive the adoption of AI. It is crucial to pay special attention to the ethical implications of AI in professional environments, issues related to job security, and the significant role that training can play in boosting employees’ confidence and adaptability to AI. Additionally, our study suggests that an examination of leadership and management strategies in AI integration could yield important insights. These perspectives are directly derived from our analysis, underscoring a critical need to address these identified gaps in future research.
To conclude, while our study initially aimed to analyze the relationship between artificial intelligence (AI) and employee well-being, our review of the literature revealed that the impact of AI extends beyond well-being to encompass employee engagement, productivity, performance, and satisfaction (Tang et al., 2023).
5 Conclusion
This article has explored the complex interplay between artificial intelligence (AI) and employee well-being through a detailed bibliometric review and contextual analysis. Our findings highlight the dual nature of AI’s impact: enhancing efficiency and engagement on one hand, while potentially increasing job stress and insecurity on the other (Morandini et al., 2023). Significant gaps were identified in the existing literature, particularly concerning the sectors studied, methodologies applied, and theories employed. The adoption of AI in professional settings presents substantial ethical and organizational challenges that necessitate careful management to maximize benefits while minimizing risks to employee well-being (Olatoye et al., 2024).
These results corroborate the findings of Stamate et al. (2021), who suggest that employees’ attitudes toward AI significantly influence their job satisfaction and psychological well-being. Moreover Calvo et al. (2020) and (Nazareno and Schiff, 2021) indicates that while AI can offer opportunities, it also poses potential threats of instability and stress for workers undergoing rapid technological transitions.
Future research in this domain is essential to develop a nuanced understanding of AI’s interaction with employee well-being. This necessity for further investigation is underscored by Ortega-Bolaños et al. (2024), who call for a deeper exploration of the ethical issues associated with AI in the workplace. Additionally, Fisher et al. (2023) emphasize the need to examine AI’s impacts on occupational health and safety equity. Indeed, good mental health among employees can significantly enhance an organization’s competitiveness. An organization whose employees suffer from mental health issues may face detrimental impacts on its financial and operational health. This can lead to problems such as decreased productivity and concentration, as well as an increase in absenteeism and turnover rates (Dimoff et al., 2014; Dimoff and Kelloway, 2018). To mitigate these risks, organizations looking to maintain their performance and the well-being of their employees should consider implementing artificial intelligence solutions that address the psychological needs of employees. It is crucial to use AI cautiously, tailoring its application to the specific needs, age, and educational level of each employee (Wei and Li, 2022).
Future research should also incorporate the theoretical perspectives mentioned, particularly those concerning data privacy and transparency in AI processing. Additionally, as emphasized by Gibbons (2021), ongoing education for employees on AI technologies and support for their adaptation are essential to minimize potential negative impacts and ensure a technological integration that improves workplace well-being rather than detracting from it. The findings of our study contribute to the existing literature on artificial intelligence (AI) and employee well-being at three levels: theoretical, practical, and methodological. Theoretically, this research paves the way for new perspectives by analyzing the intersection of AI and employee well-being through theoretical frameworks such as the “Social Baseline Theory” and the “JD-R Model.” The results have also highlighted the need to explore these themes in various research contexts, including in small manufacturing companies, call centers, and the non-profit sector, as well as through diverse targets such as comparative studies between employees and employers, between different age groups, and those with lower education levels. Practically, although our study is theoretical in nature, it has revealed key points that allow organizations to fully benefit from the potential of AI to improve performance and employee well-being. Indeed, successful adoption of AI requires organizations to implement certain ethical standards such as transparency and fairness, as well as effective AI management based on ongoing training of employees to enhance their skills, placing them at the center of attention to preserve their well-being and mental health. Methodologically, our study has developed a solid theoretical framework for studying artificial intelligence and well-being, allowing future researchers to further explore this field. We have also identified several methodological approaches to adopt in future research, such as ethnographic or longitudinal studies, to assess long-term impacts. In summary, in an era marked by rapid digital transformation, successful adoption of AI requires an approach that promotes the integration of all stakeholders and relies on close collaboration between them, in order to develop strategies that combine employee well-being and organizational performance (Dikshit et al., 2024).
Data availability statement
The raw data supporting the conclusions of this article will be made available by the authors, without undue reservation.
Author contributions
MS: Conceptualization, Methodology, Project administration, Supervision, Validation, Visualization, Writing – original draft, Writing – review & editing. SB: Conceptualization, Data curation, Formal analysis, Investigation, Methodology, Software, Validation, Writing – original draft, Writing – review & editing. AG: Conceptualization, Data curation, Formal analysis, Investigation, Methodology, Validation, Writing – original draft, Writing – review & editing.
Funding
The author(s) declare that no financial support was received for the research, authorship, and/or publication of this article.
Acknowledgments
DeepL and Chatgpt were used to assist with the language editing of this article.
Conflict of interest
The authors declare that the research was conducted in the absence of any commercial or financial relationships that could be construed as a potential conflict of interest.
Publisher’s note
All claims expressed in this article are solely those of the authors and do not necessarily represent those of their affiliated organizations, or those of the publisher, the editors and the reviewers. Any product that may be evaluated in this article, or claim that may be made by its manufacturer, is not guaranteed or endorsed by the publisher.
References
Ahn, J., Kim, J., and Sung, Y. (2022). The effect of gender stereotypes on artificial intelligence recommendations. J. Bus. Res. 141, 50–59. doi: 10.1016/j.jbusres.2021.12.007
Alavi, S., and Habel, J. (2021). The human side of digital transformation in sales: review & future paths. J. Pers. Selling Sales Manag. 41, 83–86. doi: 10.1080/08853134.2021.1920969
Bankins, S. (2021). The ethical use of artificial intelligence in human resource management: a decision-making framework. Ethics Inf. Technol. 23, 841–854. doi: 10.1007/s10676-021-09619-6
Bello, B., Tula, S., Omotoye, G., Kess-Momoh, A., and Daraojimba, A. (2024). Work-life balance and its impact in modern organizations: an HR review. World J. Adv. Res. Rev. 21, 1162–1173. doi: 10.30574/wjarr.2024.21.1.0106
Benneh, Mensah G. Artificial intelligence and ethics: A comprehensive review of Bias mitigation, transparency, and accountability in AI systems. (2023).
Bhatia, T. Artificial intelligence in HR. (2018). Available at: https://www.proquest.com/docview/2158022576/abstract/EB8FD4371A?sourcetype=Magazines (Accessed July 13, 2024).
Bollier, D. The Aspen Institute. (2017). Artificial intelligence comes of age. Available at: https://www.aspeninstitute.org/publications/artificial-intelligence-comes-age/ (Accessed July 13, 2024).
Brougham, D., and Haar, J. (2018). Smart technology, artificial intelligence, robotics, and algorithms (STARA): employees’ perceptions of our future workplace. J. Manag. Organ. 24, 239–257. doi: 10.1017/jmo.2016.55
Calvo, R. A., Peters, D., Vold, K., and Ryan, R. M. (2020). “Supporting human autonomy in AI systems: A framework for ethical enquiry” in Ethics of digital well-being: A multidisciplinary approach. eds. C. Burr and L. Floridi (Cham: Springer International Publishing), 31–54.
Case, A., and Deaton, A. (2017). Mortality and morbidity in the 21st century. Brook. Pap. Econ. Act. 2017, 397–476. doi: 10.1353/eca.2017.0005
Chadegani, A. A., Salehi, H., Yunus, M. M., Farhadi, H., Fooladi, M., Farhadi, M., et al. (2013). A comparison between two Main academic literature collections: web of science and Scopus databases. Asian Soc. Sci. 9:p18. doi: 10.5539/ass.v9n5p18
Chang, K. (2020). Artificial intelligence in personnel management: the development of APM model. Bottom Line 33, 377–388. doi: 10.1108/BL-08-2020-0055
Chari, R., Chang, C. C., Sauter, S. L., Petrun Sayers, E. L., Cerully, J. L., Schulte, P., et al. (2018). Expanding the paradigm of occupational safety and health: A new framework for worker well-being. J. Occup. Environ. Med. 60, 589–593. doi: 10.1097/JOM.0000000000001330
Chiang, D. C. I., Antwi-Afari, M. F., Anwer, S., Mohandes, S. R., and Li, X. (2023). Occupational stress in the construction industry: a bibliometric-qualitative analysis of literature and future research directions. Int. J. Build Pathol. Adapt. doi: 10.1108/IJBPA-08-2023-0114 [Epub ahead of print].
Correia Loureiro, S. M., Bilro, R. G., and Neto, D. (2023). Working with AI: can stress bring happiness? Serv. Bus. 17, 233–255. doi: 10.1007/s11628-022-00514-8
Correia, MJ, and Matos, F. The impact of artificial intelligence on innovation management: A literature review. In: F Matos, MD Ferreiro, A Rosa, and I Salavisa, editors. Proceedings of the 16th european conference on innovation and entrepreneurship (ECIE 2021), 1. NR Reading: Acad Conferences Ltd; (2021). 222–230. (Proceedings of the European Conference on Entrepreneurship and Innovation). Available at: https://www.webofscience.com/wos/woscc/full-record/WOS:000759344600027 (Accessed May 13, 2024).
Cramarenco, R. E., Burca-Voicu, M. I., and Dabija, D. C. (2023). The impact of artificial intelligence (AI) on employees’ skills and well-being in global labor markets: A systematic review. Oecon Copernic. 14, 731–767. doi: 10.24136/oc.2023.022
Didem, O., and Anke, H. (2021). Artificial Intelligence at Work: An Overview of the Literature. Available from: https://www.researchgate.net/publication/349854588_Artificial_Intelligence_at_Work_An_Overview_of_the_Literature
Dikshit, S, Grover, Y, Shukla, P, Mishra, A, Sahu, Y, Kumar, C, et al., Empowering employee wellness and building resilience in demanding work settings through predictive analytics. EAI Endorsed Transactions on Internet of Things. (2024). 10. Available at: https://publications.eai.eu/index.php/IoT/article/view/4644 (Accessed July 17, 2024).
Dimoff, K. J., and Kelloway, E. K. (2018). Signs of struggle (SOS): The development and validation of a behavioural mental health checklist for the workplace. Work & stress 33. doi: 10.1080/02678373.2018.1503359
Dimoff, K. J., Kevin Kelloway, E., and Maclellan A, M. (2014). Health and performance: science or advocacy? J. Organiz. Effect. 1, 316–334. doi: 10.1108/JOEPP-07-2014-0031
Eck, N. v., and Waltman, L. (2009). Software survey: VOSviewer, a computer program for bibliometric mapping. Available from: https://akjournals.com/view/journals/11192/84/2/article-p523.xml
Faye-Schjøll, I, and Høye, JC. A study on employee well-being: Investigating the effects of implementing artificial intelligence in human resource management practices. (2020). Available at: https://biopen.bi.no/bi-xmlui/handle/11250/2687363 (Accessed July 13, 2024).
Fisher, E., Flynn, M. A., Pratap, P., and Vietas, J. A. (2023). Occupational safety and health equity impacts of artificial intelligence: A scoping review. Int. J. Environ. Res. Public Health 20:6221. doi: 10.3390/ijerph20136221
Ghani, B., Memon, K. R., Han, H., Ariza-Montes, A., and Arjona-Fuentes, J. M. (2022). Work stress, technological changes, and job insecurity in the retail organization context. Front. Psychol. 13:918065. doi: 10.3389/fpsyg.2022.918065
Gibbons, E. D. (2021). Toward a more equal world: The human rights approach to extending the benefits of artificial intelligence. IEEE Technol. Soc. Mag. 40, 25–30. doi: 10.1109/MTS.2021.3056295
Gorovei, A. A. (2020). “Internet of Things and employee happiness in the digital era” in Strategica: preparing for tomorrow, today. eds. C. Bratianu, A. Zbuchea, F. Anghel, and B. Hrib (Bucharest: Tritonic Publ House), 486–494.
Hagel, J., Schwartz, J., and Bersin, J. (2018). Navigating the future of work: Can we point businesses, workers, and social institutions in the same direction?
Henkel, A., Bromuri, S., Iren, D., and Urovi, V. (2020). Half human, half machine - augmenting service employees with AI for interpersonal emotion regulation. J. Serv. Manag. 31, 247–265. doi: 10.1108/JOSM-05-2019-0160
Hizam, S. M., Akter, H., Sentosa, I., Ahmed, W., Masrek, M. N., and Ali, J. (2023). Predicting workforce engagement towards digital transformation through a multi-analytical approach. Sustain. For. 15:6835. doi: 10.3390/su15086835
Jeong, J., Kim, B. J., and Lee, J. (2024). Navigating AI transitions: how coaching leadership buffers against job stress and protects employee physical health. Front. Public Health 12:1343932. doi: 10.3389/fpubh.2024.1343932
Jiang, F., Wang, L., Li, J. X., and Liu, J. (2022). How smart technology affects the well-being and supportive learning performance of logistics employees? Front. Psychol. 12:768440. doi: 10.3389/fpsyg.2021.768440
Khan, AA, Badshah, S, Liang, P, Khan, B, Waseem, M, Niazi, M, et al. Ethics of AI: A systematic literature review of principles and challenges. arXiv. (2021). Available at: http://arxiv.org/abs/2109.07906 (Accessed July 13, 2024).
Khogali, H. O., and Mekid, S. (2023). The blended future of automation and AI: examining some long-term societal and ethical impact features. Technol. Soc. 73. doi: 10.1016/j.techsoc.2023.102232
Kinowska, H., and Sienkiewicz, L. J. (2022). Influence of algorithmic management practices on workplace well-being - evidence from European organisations. Inf. Technol. People 36, 21–42. doi: 10.1108/ITP-02-2022-0079
Kniffin, K. M., Narayanan, J., Anseel, F., Antonakis, J., Ashford, S. P., Bakker, A. B., et al. (2021). COVID-19 and the workplace: implications, issues, and insights for future research and action. Am. Psychol. 76, 63–77. doi: 10.1037/amp0000716
Kong, H., Yin, Z., Baruch, Y., and Yuan, Y. (2023). The impact of trust in AI on career sustainability: The role of employee-AI collaboration and protean career orientation. J. Vocat. Behav. 146:103928. doi: 10.1016/j.jvb.2023.103928
Konuk, H., Ataman, G., and Kambur, E. (2023). The effect of digitalized workplace on employees’ psychological well-being: digital Taylorism approach. Technol. Soc. 74:102302. doi: 10.1016/j.techsoc.2023.102302
Landsbergis, P. A., Grzywacz, J. G., and LaMontagne, A. D. (2014). Work organization, job insecurity, and occupational health disparities. Am. J. Ind. Med. 57, 495–515. doi: 10.1002/ajim.22126
Liberati, A., Altman, D. G., Tetzlaff, J., Mulrow, C., Gøtzsche, P. C., Ioannidis, J. P. A., et al. (2009). The PRISMA statement for reporting systematic reviews and Meta-analyses of studies that evaluate health care interventions: explanation and elaboration. Ann. Intern. Med. 151:W. doi: 10.7326/0003-4819-151-4-200908180-00136
Li, L. Reskilling and upskilling the future-ready workforce for industry 4.0 and beyond. (2022). Available at: https://www.researchgate.net/publication/361980796_Reskilling_and_Upskilling_the_Future-ready_Workforce_for_Industry_40_and_Beyond (Accessed July 13, 2024).
Mera, A., and Srivastavab, A. (2023). “Employee engagement in the new Normal: artificial intelligence as a buzzword or a game changer?” in The adoption and effect of artificial intelligence on hum resources management, part A (Emerald Publishing), 15–46.
Mer, A., and Virdi, A. S. (2023). “Navigating the paradigm shift in HRM practices through the Lens of artificial intelligence: A post-pandemic perspective” in The adoption and effect of artificial intelligence on hum resources management, part A (Emerald Publishing), 123–154.
Morandini, S, Fraboni, F, De Angelis, M, Giusino, D, Puzzo, G, and Pietrantoni, L. (PDF) The impact of artificial intelligence on workers’ skills: Upskilling and reskilling in Organisations. (2023). Available at: https://www.researchgate.net/publication/368721087_The_impact_of_artificial_intelligence_on_workers'_skills_Upskilling_and_reskilling_in_organisations (Accessed July 13, 2024).
Morandini, S., Fraboni, F., De Angelis, M., Puzzo, G., Giusino, D., and Pietrantoni, L. (2023). The impact of artificial intelligence on workers’ skills: upskilling and reskilling in Organisations. Informing Sci. 26, 039–068. doi: 10.28945/5078
Morley, J., Machado, C. C. V., Burr, C., Cowls, J., Joshi, I., Taddeo, M., et al. (2020). The ethics of AI in health care: a mapping review. Rochester, NY: Elsevier in the journal Social Science & Medicine. Vol. 260.
Nazareno, L., and Schiff, D. S. (2021). The impact of automation and artificial intelligence on worker well-being. Technol. Soc. 67:101679. doi: 10.1016/j.techsoc.2021.101679
Oksanen, A, Savela, N, Latikka, R, and Koivula, A. Trust toward robots and artificial intelligence: An experimental approach to human–technology interactions online. (2020). Available at: https://www.frontiersin.org/journals/psychology/articles/10.3389/fpsyg.2020.568256/full (Accessed July 14, 2024).
Olatoye, F., Awonuga, K., Mhlongo, N., Ibeh, C., Elufioye, O., and Ndubuisi, N. (2024). AI and ethics in business: A comprehensive review of responsible AI practices and corporate responsibility. Int. J. Sci. Res. Arch. 11, 1433–1443. doi: 10.30574/ijsra.2024.11.1.0235
Ortega-Bolaños, R., Bernal-Salcedo, J., Germán Ortiz, M., Galeano Sarmiento, J., Ruz, G. A., and Tabares-Soto, R. (2024). Applying the ethics of AI: a systematic review of tools for developing and assessing AI-based systems. Artif. Intell. Rev. 57:110. doi: 10.1007/s10462-024-10740-3
Peters, M. A. (2017). Technological unemployment: Educating for the fourth industrial revolution. Educ. Philos. Theory 49, 1–6.
Potter, K., and Doris, L. (2024). Ethical considerations in the development and deployment of artificial intelligence systems. Artif. Intell. [Epub ahead of print].
Rath, T., and Harter, J. K. (2010). Wellbeing: The five essential elements : Simon and Schuster, 240.
Sanderson, C., Douglas, D., Lu, Q., Schleiger, E., Whittle, J., Lacey, J., et al. (2023). AI ethics principles in practice: perspectives of designers and developers. IEEE Trans. Technol. Soci. 4, 171–187. doi: 10.1109/TTS.2023.3257303
Schilirò, D. Digital transformation, COVID-19, and the future of work. 12. (2021). 1945–1952. Available at: https://mpra.ub.uni-muenchen.de/108817/ (Accessed July 13, 2024).
Shaikh, F., Afshan, G., Anwar, R. S., Abbas, Z., and Chana, K. A. (2023). Analyzing the impact of artificial intelligence on employee productivity: the mediating effect of knowledge sharing and well-being. Asia Pac. J. Hum. Resour. 61, 794–820. doi: 10.1111/1744-7941.12385
Shwedeh, F., Aburayya, A., and Mansour, M. (2023). The impact of organizational digital transformation on employee performance: A study in the UAE. Migration Letters 20, 83–108. doi: 10.59670/ml.v20iS12.5843
Stamate, A., Sauvé, G., and Denis, P. (2021). The rise of the machines and how they impact workers’ psychological health: an empirical study. Human Behav. Emerg. Technol. 3, 942–955. doi: 10.1002/hbe2.315
Su, H. N., and Lee, P. C. (2010). Mapping knowledge structure by keyword co-occurrence: A first look at journal papers in technology foresight. Scientometrics 85, 65–79. doi: 10.1007/s11192-010-0259-8
Tang, P., Koopman, J., Mai, K., De Cremer, B. J. K., Zhang, J., Reynders, P., et al. (2023). No person is an island: unpacking the work and after-work consequences of interacting with artificial intelligence. J. Appl. Psychol. 108, 1766–1789. doi: 10.1037/apl0001103
Trotta, A., Ziosi, M., and Lomonaco, V. (2023). The future of ethics in AI: challenges and opportunities. AI & Soc. 38, 439–441. doi: 10.1007/s00146-023-01644-x
Wei, W., and Li, L. (2022). The impact of artificial intelligence on the mental health of manufacturing workers: The mediating role of overtime work and the work environment. Front. Public Health. 10:862407. doi: 10.3389/fpubh.2022.862407
Xiao, Q., Yan, J., and Bamber, G. J. (2023). How does AI-enabled HR analytics influence employee resilience: job crafting as a mediator and HRM system strength as a moderator. Pers. Rev. 20:1974. doi: 10.1108/PR-03-2023-0198 [Epub ahead of print].
Xiao, Q, Yan, J, and Bamber, GJ. How does AI-enabled HR analytics influence employee resilience: job crafting as a mediator and HRM system strength as a moderator. PR. (2023). Available at: https://www.emerald.com/insight/content/doi/10.1108/PR-03-2023-0198/full/html (Accessed June 16, 2024).
Xu, G., Xue, M., and Zhao, J. (2023). The relationship of artificial intelligence opportunity perception and employee workplace well-being: A moderated mediation model. Int. J. Environ. Res. Public Health 20. doi: 10.3390/ijerph20031974
Ye, D., Xu, B., Wei, B., Zheng, L., and Wu, Y. J. (2024). Employee work engagement in the digital transformation of enterprises: a fuzzy-set qualitative comparative analysis. Human. Soc. Sci. Commun. 11:35. doi: 10.1057/s41599-023-02418-y
Yin, M., Jiang, S., and Niu, X. (2024). Can AI really help? The double-edged sword effect of AI assistant on employees’ innovation behavior. Comput. Hum. Behav. 150:107987. doi: 10.1016/j.chb.2023.107987
Keywords: artificial intelligence, bibliometric review, employee well-being, systematic review, workplace stress
Citation: Soulami M, Benchekroun S and Galiulina A (2024) Exploring how AI adoption in the workplace affects employees: a bibliometric and systematic review. Front. Artif. Intell. 7:1473872. doi: 10.3389/frai.2024.1473872
Edited by:
Hanqi Zhuang, Florida Atlantic University, United StatesReviewed by:
Alok Bihari Singh, Malaviya National Institute of Technology, Jaipur, IndiaRajesh Tiwari, Graphic Era University, India
Copyright © 2024 Soulami, Benchekroun and Galiulina. This is an open-access article distributed under the terms of the Creative Commons Attribution License (CC BY). The use, distribution or reproduction in other forums is permitted, provided the original author(s) and the copyright owner(s) are credited and that the original publication in this journal is cited, in accordance with accepted academic practice. No use, distribution or reproduction is permitted which does not comply with these terms.
*Correspondence: Malika Soulami, Malikasoulami96@gmail.com