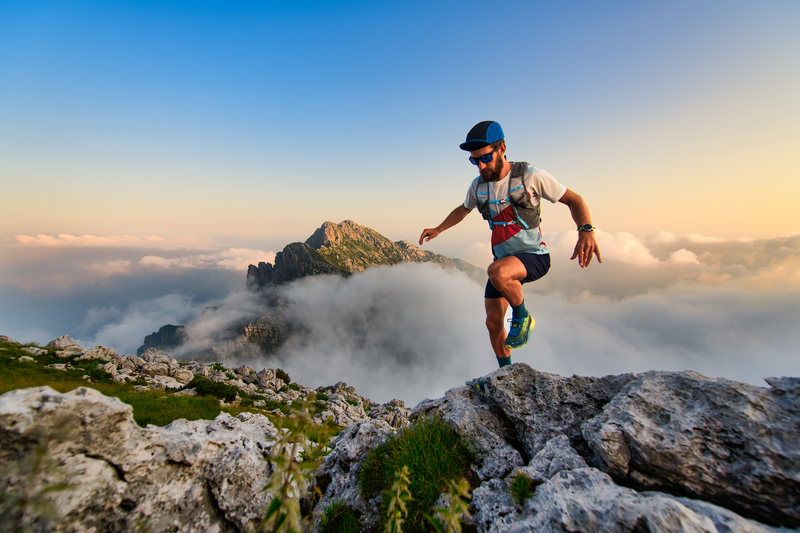
94% of researchers rate our articles as excellent or good
Learn more about the work of our research integrity team to safeguard the quality of each article we publish.
Find out more
ORIGINAL RESEARCH article
Front. Artif. Intell. , 03 September 2024
Sec. Medicine and Public Health
Volume 7 - 2024 | https://doi.org/10.3389/frai.2024.1451963
Background: Artificial intelligence (AI) is reforming healthcare, particularly in respiratory medicine and critical care, by utilizing big and synthetic data to improve diagnostic accuracy and therapeutic benefits. This survey aimed to evaluate the knowledge, perceptions, and practices of respiratory therapists (RTs) regarding AI to effectively incorporate these technologies into the clinical practice.
Methods: The study approved by the institutional review board, aimed at the RTs working in the Kingdom of Saudi Arabia. The validated questionnaire collected reflective insights from 448 RTs in Saudi Arabia. Descriptive statistics, thematic analysis, Fisher’s exact test, and chi-square test were used to evaluate the significance of the data.
Results: The survey revealed a nearly equal distribution of genders (51% female, 49% male). Most respondents were in the 20–25 age group (54%), held bachelor’s degrees (69%), and had 0–5 years of experience (73%). While 28% had some knowledge of AI, only 8.5% had practical experience. Significant gender disparities in AI knowledge were noted (p < 0.001). Key findings included 59% advocating for basics of AI in the curriculum, 51% believing AI would play a vital role in respiratory care, and 41% calling for specialized AI personnel. Major challenges identified included knowledge deficiencies (23%), skill enhancement (23%), and limited access to training (17%).
Conclusion: In conclusion, this study highlights differences in the levels of knowledge and perceptions regarding AI among respiratory care professionals, underlining its recognized significance and futuristic awareness in the field. Tailored education and strategic planning are crucial for enhancing the quality of respiratory care, with the integration of AI. Addressing these gaps is essential for utilizing the full potential of AI in advancing respiratory care practices.
The digitalisation of medical record has resulted in the accumulation of a huge amount of data (Salmon et al., 2021). IBM estimates that approximately one million gigabytes of data over the lifetime of an average patient and with the doubling of healthcare data every few years (IBM) (Carson, 2015). It is crucial to harness the power of artificial intelligence (AI) and computer scientists to make sense of this big data (Nagendran et al., 2020). AI is revolutionizing various industries, including healthcare, with applications like machine learning algorithms transforming medical diagnosis, treatment planning, and patient monitoring (Maleki Varnosfaderani and Forouzanfar, 2024; Alowais et al., 2023; Harry, 2023). In respiratory care, AI has shown promise in interpreting lung function tests, analyzing respiratory sounds, and managing ventilated patients (Al-Anazi et al., 2024; Stivi et al., 2024).
As AI technologies advance, it is essential to consider the viewpoints of healthcare professionals who will be impacted by the use of these tools (Yadav et al., 2024). Respiratory therapists, in particular, are crucial in diagnosing and treating respiratory conditions. Their opinions and perspectives regarding AI in their field can impact how these technologies are adopted and utilized effectively (Ahuja and Nair, 2021; Honkoop et al., 2022; Sreedharan and Varghese, 2020).
Previous research has examined healthcare professionals’ attitudes and perceptions towards AI in various medical specialties, including radiology (Allam et al., 2023), pathology (Berbís et al., 2023), and surgery (Li et al., 2022). However, there is a paucity of research specifically focused on respiratory care professionals (Honkoop et al., 2022). It is crucial to understand their perspectives to improve AI literacy, overcome potential obstacles, and promote the effective implementation of AI tools and algorithms in respiratory care. This study sought to evaluate the knowledge, perception, and practices of respiratory care professionals from the Kingdom of Saudi Arabia, on AI tools and related applications.
This was a cross-sectional survey study conducted online. The survey targeted respiratory therapists and educators across various institutions in Saudi Arabia, Participants were recruited via email invitation and notification, which contained a link to the web version of the questionnaire. The professional society for RTs in the country, along with leaders of the profession, disseminated the online questionnaire among their members and networks. The online version of the questionnaire was set up using the SurveyMonkey® platform (SurveyMonkey Inc., Palo Alto, CA) (Abd Halim et al., 2018). The software was designed to be completely anonymous, and it did not collect respondents’ IP addresses. Participation in the survey was voluntary, and all participants were instructed not to fill out the survey more than once. Weekly reminders were sent to maximize participation rates. The target sample size, determined using the appropriate formula, was 560 (with a range of 535–585). The survey was conducted from February 2023 to July 2023, resulting in a sample of 448 respondents. This sample size was calculated with an assumed response distribution of 50%, a margin of error of ±5%, and a confidence level of 95%.
The survey questionnaire was developed in English. Relevant literature on applications of artificial intelligence (AI) in respiratory therapy and the competencies required for its uses were reviewed. Based on this, two researchers with expertise in medical education, AI in respiratory care, and survey design constructed the questionnaire to assess RTs knowledge and perceptions about AI. Content validation was performed by a five-member expert panel comprising the research group with proficiency in survey analysis and English language. The panel evaluated the core content, language appropriateness, question suitability across domains, scoring patterns, etc. The survey was piloted with an experimental group of 20 judiciously selected participants varying in age, gender, and educational backgrounds. Questions in the knowledge, perception and practice domains underwent content validity and internal consistency testing, with responses analyzed using Cronbach’s alpha (overall α = 0.91, acceptable). The expert panel reviewed observations and recommended modifications before survey launch.
The questionnaire had four sections: socio-demographics (part 1), knowledge (part 2), perception (part 3), and practice (part 4). Socio-demographics collected were gender, age, nationality, location, education, designation, experience, hospital type/bed number, and specialization area. Participants rated importance of AI in respiratory care on a Likert scale with the response options ratings from “Strongly agree” to “Strongly disagree” options, with one 1–5 rating scale question (Jebb et al., 2021). All responses were anonymized and data was securely collected in a password-protected database.
Descriptive statistics were calculated for quantitative survey responses [frequencies and (percentages), or means, ±standard deviations as appropriate]. Responses to open-questions were analyzed thematically. The chi-square test or Fisher’s exact test were used to assess differences in responses between genders and years of experience. A p-value <0.05 was considered statistically significant. All data analyses were conducted using R statistical software version 4.0.2.
The survey was approved by the Institutional Review Board of Prince Sultan Military College of Health Sciences in Dhahran, Saudi Arabia (IRB number: IRB-2022-RC-008). Participants provided informed consent by clicking the survey link, which displayed the consent information before the questionnaire. Those who did not want to participate were given the option to decline. The consent informed participants of the study’s objectives and ensured the anonymity of the survey, protecting their data privacy. This study followed the guidelines set out in the Declaration of Helsinki.
The survey included 230 (51%) females and 218 (49%) males. The majority (54%) were in the 20–25-year age group, and most participants (69%) held a Bachelor’s degree in respiratory care, The majority (77%) graduated from universities in Saudi Arabia, approximately 52% were currently working in hospitals and 73% of them had 0–5 years of experience (Table 1).
The survey revealed varied levels of familiarity with AI among respiratory care professionals. About 28% of respondents were comfortable with the concept of AI but lacked technical knowledge, while 27% were familiar with AI but not confident in applying it to their work. Another 20% had only heard of AI through the news or media, 16% had no understanding of AI, and 8.5% had practical experience working with AI. Despite the varying levels of familiarity, there was strong support for integrating AI into respiratory therapy education. A majority, 59%, agreed or strongly agreed that basic AI knowledge should be included in the curriculum. When examining the sources of AI knowledge, 34% of participants were self-taught, 20% gained knowledge through courses, 20% through work-related activities, while 12% acquired it via postgraduate training, and 14% had no AI knowledge at all.
Looking to the future, 51% of respondents believed that AI would play a significant role in respiratory care, and 55% anticipated that AI would be integrated into many aspects of respiratory care practices. However, perspectives on AI’s impact were mixed, with 29% remaining neutral, 27% disagreeing, and only 18% expressing concern that AI might disrupt or threaten respiratory care practice. Similarly, opinions on AI’s limitations were divided, with 33% neutral, and 20% each agreeing or disagreeing about the absence of AI limitations in their work. Organizational readiness for AI implementation varied widely. While 18% of respondents were in charge of AI at their institutions, 82% were not. Among the respondents, 41% reported having someone responsible for AI in education, research, innovation, and integration within their organization. However, 39% were unaware of their organization’s AI strategy, 30% confirmed that their organization lacked one, 16% noted that a strategy was in development, and 15% reported having an established AI strategy.
The key challenges identified for AI implementation were primarily knowledge gaps and skill development, each cited by 23% of respondents. Other challenges included the lack of AI training in universities (17%), difficulty in finding quality AI education/training courses (15%), and the challenges of integrating AI into work practices (11%) and training current staff (11%). Participants highlighted several AI applications as crucial for respiratory care, including research (19%), critical care management (18%), image interpretation (17%), and quality control (15%). Other areas of interest were dose management (7.8%), communication (7.6%), emergency patient care (5.4%), teaching and mentoring (9.2%), and policy/procedures development (0.2%).
This study reveals significant gender differences in AI knowledge and perceptions among respiratory care professionals (Table 2). Female respondents were predominantly younger, with 66% aged 20–25 compared to 41% of males. However, more males held doctoral degrees (9.6% vs. 2.2% of females) and were graduates of Western universities (17% vs. 8.7% of females). These differences indicate a gender gap in certain areas of AI exposure and education, although both groups generally recognized the importance of AI in respiratory care.
Table 2. Knowledge, perception, and practice about AI in RC domain among the participants based on gender.
Perceptions of AI integration in respiratory care also varied by gender (Figure 1). Males were more likely to express excitement about AI (33% vs. 19% of females), while females were more inclined to acknowledge the challenges (27% vs. 22% of males) or admit to not knowing enough about AI (23% vs. 13% of males). Additionally, a slightly higher percentage of males believed that AI would be integrated into many respiratory care applications (37% vs. 31% of females). However, both genders had similar views on AI’s role, its potential threats to practice, and the need to include AI in the respiratory therapy curriculum. Interestingly, a higher proportion of females (48%) reported having someone responsible for AI education, research, or integration at their organization compared to males (34%) (Figure 2). Despite the differences in excitement levels, both male (46%) and female (41%) participants largely disagreed or strongly disagreed with the notion that AI would threaten or disrupt respiratory care practice in the near future, though a significant portion remained neutral (31% of females and 26% of males) (see Figures 3, 4).
In terms of AI familiarity, more males felt comfortable with the meaning of AI, and a greater percentage of males (14%) were currently working in AI-related fields compared to females (3%). Despite these differences, a majority of both male (58%) and female (60%) participants agreed or strongly agreed that the respiratory therapy curriculum should include basic AI knowledge.
The survey included 448 respiratory care professionals, with 292 clinicians and 156 participants from academic institutions (Table 3). The findings revealed that a significant portion of respondents, recognized the potential of AI in respiratory care. Specifically, 34% overall, including 35% of RT clinicians, agreed that AI would play a crucial role in various respiratory care applications. However, awareness of organizational strategies for AI implementation was lacking, with 39% overall and 46% of RT clinicians admitting they had no idea about their organization’s AI plans. The survey also highlighted several key challenges in integrating AI into respiratory care practices. These included the insufficient AI knowledge and skills among graduates (17%), difficulties in training current staff (11%), and the scarcity of quality AI education courses (15%). These challenges underscore the need for targeted educational initiatives. When considering areas where AI could have the most impact, respondents identified critical care unit management (18%), research in respiratory care (19%), and image interpretation (17%) as the most crucial.
Interestingly, academicians demonstrated a higher level of familiarity with AI compared to clinicians. While 44% of academicians reported being familiar with AI, only 19% of clinicians felt the same, though many academicians lacked confidence in applying AI in practice. Additionally, more academicians had attended AI courses (27% vs. 16% of clinicians) and were more aware of the challenges associated with integrating AI into respiratory therapy practices (35% vs. 19% of clinicians). Overall, these findings emphasize the urgent need to incorporate AI education into respiratory care curricula and provide accessible training opportunities. This approach will be vital in preparing the workforce for the growing role of AI in respiratory care.
This group made up 72% of the respondents and seemed generally more familiar with AI concepts (Table 4). However, 18% still had no idea what AI meant. While 22% were excited about integrating AI into respiratory care practice, an equal proportion (22%) remained neutral. Skill development (22%) and knowledge gaps (25%) were seen as the biggest hurdles for AI implementation. The top AI applications needed were research (19%), image interpretation (15%), and critical care management (18%). About 45% reported having someone overseeing AI integration at their institution, but 43% were unaware of any organizational AI strategy.
This group comprised 27% of respondents and generally exhibited more uncertainty about AI. Only 15% worked directly in AI roles. While 38% were excited about AI integration, 21% were aware of the associated challenges. Skill development (27%) and difficulties in implementation (17%) were flagged as major concerns. The applications with highest AI needs were quality control (20%), image interpretation (21%), and research in respiratory care (21%). Only 31% had someone responsible for AI integration at their workplace, and a significant 46% reported no organizational AI strategy. Overall, the results suggest that while AI integration is viewed positively across experience levels, considerable knowledge gaps and strategic planning deficits exist, especially among more experienced professionals.
Our study found that respiratory therapists (RTs) have varying levels of familiarity and perceptions towards AI. While most RTs acknowledged the importance of AI, there were significant gaps in knowledge and challenges in implementing AI, especially in terms of education, skill development, and organizational strategy. Differences in gender and experience also influenced perspectives, underscoring the need for tailored training programs and strategic planning to effectively incorporate AI into respiratory care practice. This study is one of the first to explore this topic among RTs, and the results indicate that while RTs see the potential benefits of AI, they lack formal training opportunities. Therefore, integrating AI education into the curriculum of respiratory therapy programs is crucial.
The disparities in AI knowledge and utility, based on gender and professional roles are consistent with findings from a study by Castagno and Khalifa (2020), which highlighted a notable disparity in AI comprehension and adoption among healthcare professionals in the UK. Despite the recognized benefits of AI in healthcare, their research revealed limited daily utilization of AI tools like speech recognition (5%) and a majority of respondents (63%) who never incorporated these technologies into their practice, underscoring a gap between the potential of AI and its actual implementation.
Abuzaid et al. (2022) also found a significant knowledge gap in AI among nurses in the United Arab Emirates. Their research showed that more than half (51%) of the participants learned about AI through self-teaching, indicating a lack of formal educational opportunities in this area. Only 20% had attended courses, and a mere 8% had engaged in postgraduate AI education, while 9% admitted to having no knowledge of AI at all. Notably, 75% of the respondents believed that AI education should be integrated into the nursing curriculum, underscoring the perceived importance of formal AI training.
Banerjee et al. (2021) conducted a survey of 210 trainee doctors at UK NHS postgraduate centers in London to evaluate their views on the impact of AI technologies on clinical training and education. The study, conducted between October and December 2020, focused on key training domains such as clinical judgment, practical skills, and research and quality improvement skills. The analysis revealed that a majority (58%) of respondents perceived AI technologies as having a positive overall impact on their training and education. Notably, 62% believed that AI would reduce clinical workload, and 68% felt it would enhance research and audit training. However, there was scepticism about AI’s potential to improve clinical judgment (46% agreement, p = 0.12) and practical skills training (32% agreement, p < 0.01). A key concern raised was the lack of AI training in current curricula, with 92% of respondents noting this gap, and 81% supporting the inclusion of more formal AI training. While the benefits of AI in healthcare are evident, it is essential to understand the knowledge, attitudes, and practices of respiratory therapists regarding AI implementation in their daily work. Our study identified various factors influencing RTs’ knowledge, perceptions, and practices related to AI use, including gender and age.
Moreover, our research uncovered disparities in knowledge and perceptions regarding the integration of AI between clinicians and academicians, that had not been previously documented scientifically. Academicians demonstrated a greater familiarity with AI compared to clinicians, with a higher proportion of academicians having participated in AI-related courses. Additionally, academicians, who predominantly held master’s or doctoral degrees compared to clinicians (45% vs. 9.6%), were more aware of the challenges in integrating AI into respiratory therapy practice. Similarly, age or years of experience also seemed to impact attitudes toward AI application. Participants with less than 5 years of experience [with the majority being under 25 years old (75.1%)] appeared more familiar with AI concepts compared to participants with more than 5 years of experience, who were relatively older, with 73% aged 31 years and above. This low level of experience was a significant limitation of our study and may have introduced bias, as the perceptions and familiarity with AI of less experienced professionals could differ from those of more seasoned practitioners. This bias arised because the respiratory therapy profession in Saudi Arabia has expanded rapidly over the past 5–7 years, resulting in a predominance of newer professionals. To mitigate this bias in future studies, we recommend broadening recruitment efforts to include a larger proportion of experienced professionals, implementing stratified sampling to ensure diverse representation, and conducting longitudinal studies to track how perceptions evolve with experience.
The top three AI applications sought after by the participating respiratory therapists were in the fields of research (19%), critical care units (18%), and image interpretation (17%). It is worth noting that most participants, regardless of gender, age, or workplace, agreed or strongly agreed that the respiratory therapy curriculum should cover basic AI knowledge. However, a major limitation of the study is the subjective nature of self-reported questionnaires, which may introduce a certain degree of bias in the reported responses. In a related discussion on how AI is perceived among patient populations, a study by Fritsch et al. (2022) found that German patients and their companions are generally open to the use of AI in healthcare. Although their knowledge about AI was moderate, the majority viewed AI in healthcare positively. Importantly, patients emphasized the need for physician supervision over AI, insisting that doctors retain ultimate responsibility for diagnosis and therapy.
This study found that there are different levels of knowledge and perception about AI, revealing significant challenges such as knowledge gaps and organizational readiness deficits. The use of AI differs between genders and experience levels, suggesting the importance of customized education and training approaches. Addressing these challenges through foundational to comprehensive AI training programs and strategic planning can help improve the integration of AI in respiratory care services may result in enhanced patient outcome. Given that this study is the first of its kind globally, further research is recommended on a broader scale to assess the formal integration and use of AI in respiratory therapy across diverse populations and healthcare systems. Such studies will be crucial in developing universally applicable strategies for AI integration, ensuring that respiratory care professionals worldwide can fully leverage AI’s potential to advance the field.
The original contributions presented in the study are included in the article/supplementary material, further inquiries can be directed to the corresponding author.
The studies involving humans were approved by IRB-Prince Sultan Military College of Health Sciences, Dhahran, Dammam, Saudi Arabia. The studies were conducted in accordance with the local legislation and institutional requirements. The participants provided their written informed consent to participate in this study.
JS: Conceptualization, Data curation, Formal analysis, Investigation, Methodology, Supervision, Validation, Writing – original draft, Writing – review & editing. AsA: Writing – original draft, Writing – review & editing, Conceptualization, Investigation, Methodology. AmA: Writing – original draft, Writing – review & editing, Conceptualization, Investigation, Methodology. GG: Writing – original draft, Writing – review & editing, Conceptualization, Data curation, Formal analysis, Resources. AAlm: Conceptualization, Data curation, Formal analysis, Writing – original draft, Writing – review & editing. RA: Investigation, Methodology, Writing – original draft, Writing – review & editing. IA: Supervision, Validation, Visualization, Writing – original draft, Writing – review & editing. MuA: Resources, Software, Supervision, Validation, Writing – original draft, Writing – review & editing. MeA: Investigation, Project administration, Resources, Software, Writing – review & editing. AAlq: Investigation, Methodology, Project administration, Writing – review & editing. MoA: Conceptualization, Data curation, Formal analysis, Writing – review & editing. EA: Project administration, Supervision, Writing – review & editing. MK: Writing – original draft, Writing – review & editing.
The author(s) declare that no financial support was received for the research, authorship, and/or publication of this article.
The authors are grateful to the Saudi Society for Respiratory Care (SSRC) for their valuable support in distributing the survey. The authors also want to thank all the respiratory therapy colleagues in Saudi Arabia who enthusiastically participated in the survey to reflect their knowledge aiming to incorporate new technology into their practice. Furthermore, the authors appreciate the visionary leaders in the profession who have played a crucial role in advancing respiratory therapy in Saudi Arabia and fostering its development in recent years.
The authors declare that the research was conducted in the absence of any commercial or financial relationships that could be construed as a potential conflict of interest.
All claims expressed in this article are solely those of the authors and do not necessarily represent those of their affiliated organizations, or those of the publisher, the editors and the reviewers. Any product that may be evaluated in this article, or claim that may be made by its manufacturer, is not guaranteed or endorsed by the publisher.
Abd Halim, M., Foozy, C. F. M., Rahmi, I., and Mustapha, A. (2018). A review of live survey application: SurveyMonkey and SurveyGizmo. Int. J. Inform. Vis. 2, 309–312. doi: 10.30630/joiv.2.4-2.170
Abuzaid, M. M., Elshami, W., and Fadden, S. M. (2022). Integration of artificial intelligence into nursing practice. Health Technol. 12, 1109–1115. doi: 10.1007/s12553-022-00697-0
Ahuja, V., and Nair, L. V. (2021). Artificial intelligence and technology in COVID era: a narrative review. J. Anaesthesiol. Clin. Pharmacol. 37, 28–34. doi: 10.4103/joacp.JOACP_558_20
Al-Anazi, S., Al-Omari, A., Alanazi, S., Marar, A., Asad, M., Alawaji, F., et al. (2024). Artificial intelligence in respiratory care: current scenario and future perspective. Ann. Thorac. Med. 19, 117–130. doi: 10.4103/atm.atm_192_23
Allam, A. H., Eltewacy, N. K., Alabdallat, Y. J., Owais, T. A., Salman, S., and Ebada, M. A. (2023). Knowledge, attitude, and perception of Arab medical students towards artificial intelligence in medicine and radiology: a multi-national cross-sectional study. Eur. Radiol. 34, 1–14. doi: 10.1007/s00330-023-10509-2
Alowais, S. A., Alghamdi, S. S., Alsuhebany, N., Alqahtani, T., Alshaya, A. I., Almohareb, S. N., et al. (2023). Revolutionizing healthcare: the role of artificial intelligence in clinical practice. BMC Med. Educ. 23:689. doi: 10.1186/s12909-023-04698-z
Banerjee, M., Chiew, D., Patel, K. T., Johns, I., Chappell, D., Linton, N., et al. (2021). The impact of artificial intelligence on clinical education: perceptions of postgraduate trainee doctors in London (UK) and recommendations for trainers. BMC Med. Educ. 21:429. doi: 10.1186/s12909-021-02870-x
Berbís, M. A., McClintock, D. S., Bychkov, A., Van der Laak, J., Pantanowitz, L., Lennerz, J. K., et al. (2023). Computational pathology in 2030: a Delphi study forecasting the role of AI in pathology within the next decade. EBioMedicine 88:104427. doi: 10.1016/j.ebiom.2022.104427
Carson, E. (2015). IBM Watson Health computes a pair of new solutions to improve healthcare data and security: TechRepublic. Available at: https://www.techrepublic.com/article/ibm-watson-health-computes-a-pair-of-new-solutions-to-improve-healthcare-data-and-security/ (Accessed August 22, 2023).
Castagno, S., and Khalifa, M. (2020). Perceptions of artificial intelligence among healthcare staff: a qualitative survey study. Front. Artif. Intell. 3:578983. doi: 10.3389/frai.2020.578983
Fritsch, S. J., Blankenheim, A., Wahl, A., Hetfeld, P., Maassen, O., Deffge, S., et al. (2022). Attitudes and perception of artificial intelligence in healthcare: a cross-sectional survey among patients. Digit. Health 8:205520762211167. doi: 10.1177/20552076221116772
Harry, A. (2023). The future of medicine: harnessing the power of AI for revolutionizing healthcare. Int. J. Multidiscip. Sci. Arts 2, 36–47. doi: 10.47709/ijmdsa.v2i1.2395
Honkoop, P., Usmani, O., and Bonini, M. (2022). The current and future role of technology in respiratory care. Pulm. Ther. 8, 167–179. doi: 10.1007/s41030-022-00191-y
Jebb, A. T., Ng, V., and Tay, L. (2021). A review of key Likert scale development advances: 1995–2019. Front. Psychol. 12:637547. doi: 10.3389/fpsyg.2021.637547
Li, B., de Mestral, C., Mamdani, M., and Al-Omran, M. (2022). Perceptions of Canadian vascular surgeons toward artificial intelligence and machine learning. J. Vasc. Surg. Cases Innov. Tech. 8, 466–472. doi: 10.1016/j.jvscit.2022.06.018
Maleki Varnosfaderani, S., and Forouzanfar, M. (2024). The role of AI in hospitals and clinics: transforming healthcare in the 21st century. Bioengineering 11:337. doi: 10.3390/bioengineering11040337
Nagendran, M., Chen, Y., Lovejoy, C. A., Gordon, A. C., Komorowski, M., Harvey, H., et al. (2020). Artificial intelligence versus clinicians: systematic review of design, reporting standards, and claims of deep learning studies. BMJ 368:m689. doi: 10.1136/bmj.m689
Salmon, J. W., Thompson, S. L., Salmon, J. W., and Thompson, S. L. (2021). “Big data: information technology as control over the profession of medicine” in The corporatization of American Health Care (Cham: Springer), 181–254.
Sreedharan, J. K., and Varghese, S. (2020). Twenty-five years of excellence; respiratory therapy in India-past, present, and future. Indian J. Respir. Care 9, 5–11. doi: 10.4103/ijrc.ijrc_62_19
Stivi, T., Padawer, D., Dirini, N., Nachshon, A., Batzofin, B. M., and Ledot, S. (2024). Using artificial intelligence to predict mechanical ventilation weaning success in patients with respiratory failure, including those with acute respiratory distress syndrome. J. Clin. Med. 13:1505. doi: 10.3390/jcm13051505
Keywords: artificial intelligence, AI, respiratory care, respiratory therapy, professionals, challenges, integration artificial intelligence, integration
Citation: Sreedharan JK, Alharbi A, Alsomali A, Gopalakrishnan GK, Almojaibel A, Alajmi R, Albalawi I, Alnasser M, Alenezi M, Alqahtani A, Alahmari M, Alzahrani E and Karthika M (2024) Artificial intelligence in respiratory care: knowledge, perceptions, and practices—a cross-sectional study. Front. Artif. Intell. 7:1451963. doi: 10.3389/frai.2024.1451963
Received: 20 June 2024; Accepted: 22 August 2024;
Published: 03 September 2024.
Edited by:
L. J. Muhammad, Bayero University Kano, NigeriaReviewed by:
Massimo Salvi, Polytechnic University of Turin, ItalyCopyright © 2024 Sreedharan, Alharbi, Alsomali, Gopalakrishnan, Almojaibel, Alajmi, Albalawi, Alnasser, Alenezi, Alqahtani, Alahmari, Alzahrani and Karthika. This is an open-access article distributed under the terms of the Creative Commons Attribution License (CC BY). The use, distribution or reproduction in other forums is permitted, provided the original author(s) and the copyright owner(s) are credited and that the original publication in this journal is cited, in accordance with accepted academic practice. No use, distribution or reproduction is permitted which does not comply with these terms.
*Correspondence: Jithin K. Sreedharan, aml0aGlua3NyZWVAZ21haWwuY29t; aml0aGluLnNyZWVkaGFyYW5AdWRzdC5lZHUucWE=
Disclaimer: All claims expressed in this article are solely those of the authors and do not necessarily represent those of their affiliated organizations, or those of the publisher, the editors and the reviewers. Any product that may be evaluated in this article or claim that may be made by its manufacturer is not guaranteed or endorsed by the publisher.
Research integrity at Frontiers
Learn more about the work of our research integrity team to safeguard the quality of each article we publish.