- 1AI for Good Lab, Microsoft Corporation, Redmond, WA, United States
- 2Microsoft Research, Microsoft Corporation, Redmond, WA, United States
Background
While there are many examples of how healthcare could create value by emulating processes used in non-healthcare industries (Gawande, 2012), healthcare is fundamentally different from other manufacturing or services industries for three main reasons: (1) healthcare prices are not transparent and are not directly paid for by the consumer; (2) unlike other industries that try to increase per-capita revenue, US healthcare aims to minimize per-capita costs by eliminating waste, improving quality, and achieving better care outcomes; and (3) healthcare cannot standardize its inputs, as patients have unique health profiles, environmental exposures, and genetic predispositions to health states (Weeks and Weinstein, 2015).
The goal of healthcare is to achieve quadruple aim: improving the patient experience, the provider experience, and healthcare quality and outcomes (equitably), while reducing per-capita healthcare expenditures (Bodenheimer and Sinsky, 2014). However, this is challenging because of the impact of social determinants of health, which account for up to 70% of patients' health outcomes (Singh et al., 2017). Further, there is substantial waste in the US healthcare system, some of which is administrative and attributable to the US third party payment system (Gaffney et al., 2023), but much of which is attributable to overuse of unnecessary products and services (Shrank et al., 2019) and excessive provision of low value care (Powers et al., 2020). As a result, the haphazard application of artificial intelligence may amplify inefficiencies and biases in the US healthcare system.
A different approach
In the absence of health insurance reform, technological transformation of healthcare and using AI (artificial intelligence) to achieve the quadruple aim may improve healthcare efficiency in four ways (summarized in the Table 1).
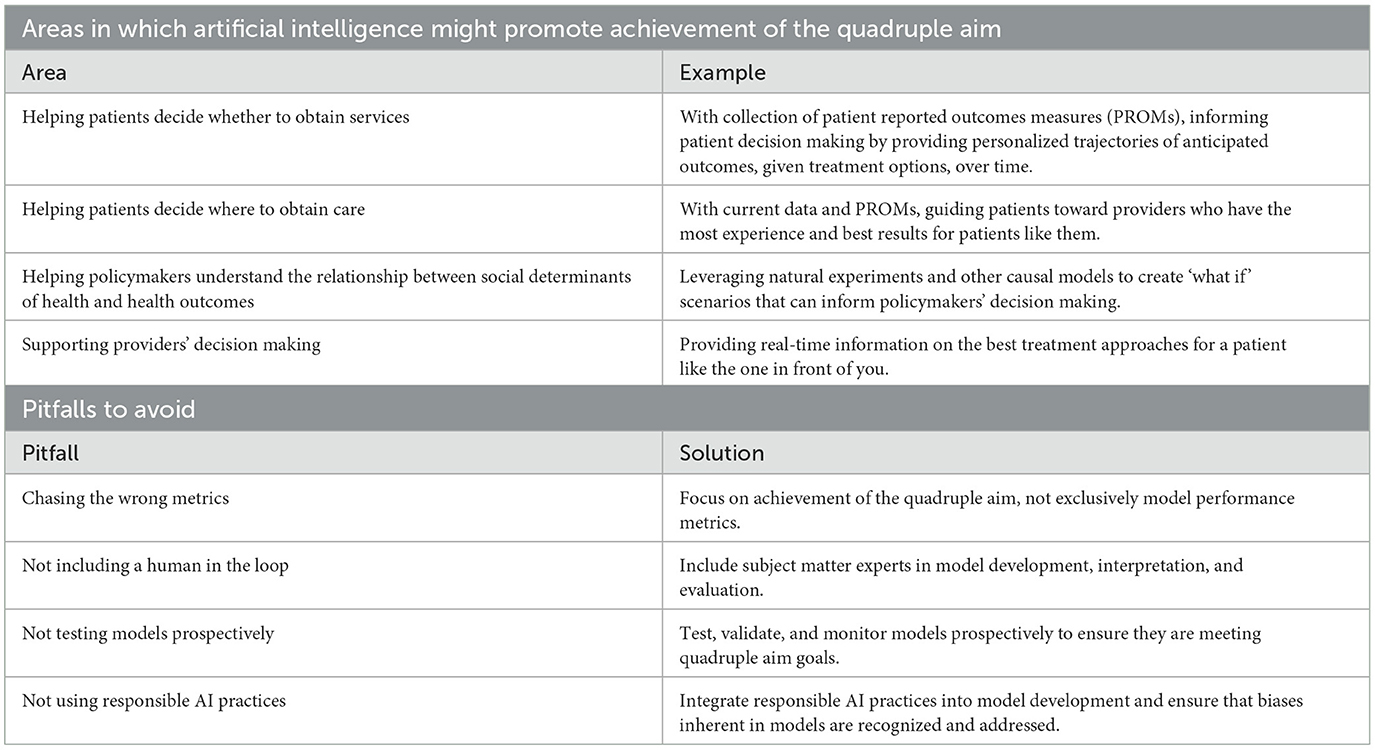
Table 1. Areas in which artificial intelligence might promote achievement of the quadruple aim (top) and pitfalls to avoid in using artificial intelligence in that pursuit (bottom), with examples and solutions, respectively, provided.
Helping patients decide whether to obtain services
Informed choice can change the dynamics of decision making and empowering well-informed consumers with objective information about treatment options, potential risks and benefits of different options, and the degree to which their choices align with their own values (Stacey et al., 2017). Decision aids are particularly helpful in preference-sensitive conditions – like lumbar spinal stenosis, where medical and surgical treatment options offer different short- and long-term risks and benefits (Asthana et al., 2024) – but can also be useful in acute care settings, as when patients are admitted to an Intensive Care Unit where issues of outcomes and futility might arise (Göcking et al., 2023). However, decision aids are under-used (The Commonwealth Fund, 2024). In the short run, for instance, AI could surface treatment options when a patient is using a search engine to seek care for a particular condition, enhance patients' knowledge of those treatment options, and empower them to ask questions of their providers and make informed choices. In the long run, should additional information be collected in the treatment and follow-up process, informed by patient reported outcomes measures collected on patients who did and did not obtain a particular procedure, AI could model outcome trajectories for patients with particular clinical and demographic constellations, helping patients identify treatment pathways consistent with their preferences and values.
Helping patients decide where to obtain desired care
Having decided to obtain care, patients may be uninformed about where to obtain the best care for their healthcare need. There is a long history demonstrating that, for certain procedures, there is a volume-outcomes relationship wherein providers (Birkmeyer et al., 2003; Goodney et al., 2005; Sahni et al., 2016) and hospitals (Flood et al., 1984) that perform more procedures have better outcomes. Despite efforts by the Leapfrog Group (Leapfrog Hospital Survey, 2024) and the Centers for Medicare and Medicaid Services (Centers for Medicare and Medicaid Services, 2024) to provide provider- and procedure-specific information on procedural volumes, those data are not readily interpretable by patients who are making decisions about where to obtain services. Further, while provider- and hospital quality indicators abound, despite finding them useful (Marang-Van De Mheen and Vincent, 2023), patients currently rely much more on word of mouth and interpersonal skills than objective quality metrics when choosing a provider (Yahanda et al., 2016). In the short run, AI could identify high-volume and high-quality providers for particular procedures, surface additional information that patients might want to know (like gender, board certification status, medical school attended, year of graduation, and social media reviews), and aggregate those data to facilitate informed consumer choice. In the long run, emulating matching algorithms used in ecommerce websites, AI might identify the best patient-provider matches based on patients' expectations of encounters and the types of encounters and interactions providers prefer.
Helping policymakers understand the relationship between social determinants of health and healthcare access, quality, and outcomes
The long documented relationships between social determinants of health (SDOH) and health status and outcomes are complex (Marmot et al., 1978; Blane, 1995; Chetty et al., 2016). AI can be used to inform policymakers on the magnitude, timing, and beneficiaries of returns on investment to health following effort to improve SDOH equity (Weeks et al., 2023). To date, these relationships have been associative: in the future, leveraging natural experiments and other causal models, AI might model “what if” scenarios to help policymakers prioritize SDOH interventions to maximize returns to health.
Supporting providers' decision making
The exceedingly high volume of medical knowledge generated makes it impossible for clinicians to keep current with treatment recommendations.AI-informed clinical decision support tools have great potential use in healthcare: (Giordano et al., 2021) such tools can improve care quality, lead to more rapid diagnosis and treatment, and support equity in healthcare delivery. This support can enhance healthcare efficiency – thereby lowering per-capita costs – while improving the provider experience and patients' experiences and health outcomes, as regularly evaluated by regulatory agencies.
Avoiding misapplication of AI in medicine
While AI has tremendous promise in helping to achieve the quadruple aim, as articulated above, we suggest four guidelines for avoiding haphazard applications of AI in medicine.
1. Avoid chasing the wrong metrics. The goal of a model is not to achieve the best area under the curve, but to have measurable positive clinical impact and to achieve the quadruple aim, using metrics defined prior to model implementation. If the model or the technology does not measurably and efficiently promote achievement of the quadruple aim, it should not be implemented.
2. Always include a human subject matter expert in the loop. Models increasingly use a ‘human in the loop' process to ensure that the model is operating as intended; in healthcare, it is critical to include a healthcare subject matter expert in the loop. Models must make sense to providers, so interpretable models and tools can allow subject matter experts to evaluate a model's utility in clinical practice, using metrics defined prior to model implementation.
3. Test, validate, and monitor models. AI models are invariably developed, tested, and refined retrospectively. While AI models have an advantage of testing results on a randomly selected held-out dataset, just as with any quality improvement effort all AI models should be prospectively tested and validated on the target population before widespread implementation, using pre-defined validation thresholds. Further, models should be monitored over time: if models are effective, they may lead to behavior change; that behavior change may change key relationships, and those changes will require new model development. Those determining whether to develop and implement AI models should consider the cumulative long-term costs of monitoring and re-developing models.
4. Use responsible AI practices Model effectiveness is intrinsically tied to the quality of data used in their training; ensuring that those data free from bias is crucial. When the data itself are not biased, subsequent decisions derived from its analysis – for instance, misapplication of models to populations that are not represented in the unbiased data - might be unfair. Particularly with health-related AI models, where stakes are significantly higher and impacts more profound, adherence to responsible AI practices is imperative.
Conclusion
Current uses of AI applications can improve the efficiency of healthcare operations, such as scheduling, letter writing, provider in-box email responses, patient triage, and coding optimization. These uses can improve patient and provider experiences, reduce per-capita healthcare costs, and promote achievement of the quadruple aim.
However, given healthcare's many inefficiencies, the unconsidered application of AI may increase healthcare costs without advancing the quadruple aim. AI models can be expensive to develop, test, implement, and monitor: a modest increase in accuracy may not warrant the expense if the impact on patients and clinical care is not significant. An unwavering focus on and objective evaluation of how technological implementation helps achieve the quadruple aim is essential for improving healthcare efficiency and effectiveness.
Author contributions
WW: Conceptualization, Writing – original draft. JL: Writing – review & editing. JW: Writing – review & editing.
Funding
The author(s) declare that no financial support was received for the research, authorship, and/or publication of this article.
Conflict of interest
WW, JL, and JW were employed by the Microsoft Corporation.
Publisher's note
All claims expressed in this article are solely those of the authors and do not necessarily represent those of their affiliated organizations, or those of the publisher, the editors and the reviewers. Any product that may be evaluated in this article, or claim that may be made by its manufacturer, is not guaranteed or endorsed by the publisher.
References
Asthana, S., Walker, J., Staub, J., Bajaj, P., Reyes, S., Shlobin, N. A., et al. (2024). Preference sensitive care and shared-decision making in lumbar spinal stenosis: a scoping review. Spine 2024:4952. doi: 10.1097/BRS.0000000000004952
Birkmeyer, J. D., Stukel, T. A., Siewers, A. E., Goodney, P. P., Wennberg, D. E., Lucas, F. L., et al. (2003). Surgeon volume and operative mortality in the United States. N. Engl. J. Med. 349, 2117–2127. doi: 10.1056/NEJMsa035205
Blane, D. (1995). Social determinants of health–socioeconomic status, social class, and ethnicity. Am. J. Public Health 85, 903–905. doi: 10.2105/AJPH.85.7.903
Bodenheimer, T., and Sinsky, C. (2014). From triple to quadruple aim: care of the patient requires care of the provider. Ann. Fam. Med. 12, 573–576. doi: 10.1370/afm.1713
Centers for Medicare and Medicaid Services (2024). Find and Compare Providers Near You. Available online at: https://www.medicare.gov/care-compare/?redirect=trueandproviderType=Physician (accessed March 3, 2024).
Chetty, R., Stepner, M., Abraham, S., Lin, S., Scuderi, B., Turner, N., et al. (2016). The association between income and life expectancy in the United States, 2001-2014. JAMA 315, 1750–1766. doi: 10.1001/jama.2016.4226
Flood, A. B., Scott, W. R., and Ewy, W. (1984). Does practice make perfect? Part I: The relation between hospital volume and outcomes for selected diagnostic categories. Med Care 22, 98–114. doi: 10.1097/00005650-198402000-00002
Gaffney, A. W., Himmelstein, D. U., Woolhandler, S., and Kahn, J. G. (2023). Hospital expenditures under global budgeting and single-payer financing: an economic analysis, 2021-2030. Int. J. Soc. Determinants Health Health Serv. 53, 548–556. doi: 10.1177/27551938231152750
Giordano, C., Brennan, M., Mohamed, B., Rashidi, P., Modave, F., Tighe, P., et al. (2021). Accessing artificial intelligence for clinical decision-making. Front. Digit Health 3:645232. doi: 10.3389/fdgth.2021.645232
Göcking, B., Gloeckler, S., Ferrario, A., Brandi, G., Glässel, A., Biller-Andorno, N. A., et al. (2023). case for preference-sensitive decision timelines to aid shared decision-making in intensive care: need and possible application. Front. Digit. Health 5:1274717. doi: 10.3389/fdgth.2023.1274717
Goodney, P. P., Lucas, F. L., Stukel, T. A., and Birkmeyer, J. D. (2005). Surgeon specialty and operative mortality with lung resection. Ann Surg. 241, 179–184. doi: 10.1097/01.sla.0000149428.17238.03
Leapfrog Hospital Survey (2024). Safety in Numbers: Hospital Performance on Leapfrog's Surgical Volume Standard Based on Results of the 2019 Leapfrog Hospital Survey. Available online at https://www.leapfroggroup.org/sites/default/files/Files/Leapfrog%20Report%20on%20Safe%20Surgical%20Volumes%202020.pdf (accessed March 3, 2024).
Marang-Van De Mheen, P. J., and Vincent, C. (2023). Measuring what matters: refining our approach to quality indicators. BMJ Qual. Saf. 32, 305–308. doi: 10.1136/bmjqs-2022-015221
Marmot, M. G., Rose, G., Shipley, M., and Hamilton, P. J. (1978). Employment grade and coronary heart disease in British civil servants. J. Epidemiol. Commun. Health 2, 244–249. doi: 10.1136/jech.32.4.244
Powers, B. W., Jain, S. H., and Shrank, W. H. (2020). De-adopting low-value care: evidence, eminence, and economics. JAMA 324, 1603–1604. doi: 10.1001/jama.2020.17534
Sahni, N. R., Dalton, M., Cutler, D. M., Birkmeyer, J. D., and Chandra, A. (2016). Surgeon specialization and operative mortality in United States: retrospective analysis. BMJ 354:i3571. doi: 10.1136/bmj.i3571
Shrank, W. H., Rogstad, T. L., and Parekh, N. (2019). Waste in the US health care system: estimated costs and potential for savings. JAMA 322, 1501–1509. doi: 10.1001/jama.2019.13978
Singh, G. K., Daus, G. P., Allender, M., Ramey, C. T., Martin, E. K., Perry, C., et al. (2017). Social determinants of health in the United States: addressing major health inequality trends for the nation, 1935-2016. Int. J. MCH AIDS 6, 139–164. doi: 10.21106/ijma.236
Stacey, D., Légar,é, F., Lewis, K., Barry, M. J., Bennett, C. L., Eden, K. B., et al. (2017). Decision aids for people facing health treatment or screening decisions. Cochrane Database Syst. Rev. 4, Cd001431. doi: 10.1002/14651858.CD001431.pub5
The Commonwealth Fund (2024). Helping Patients Make Better Treatment Choices With Decision Aids. Available online at: https://www.commonwealthfund.org/publications/newsletter-article/helping-patients-make-better-treatment-choices-decision-aids (accessed March 11, 2024).
Weeks, W. B., and Weinstein, J. N. (2015). How healthcare is different. Am. J. Med. 128, 107–108. doi: 10.1016/j.amjmed.2014.09.015
Weeks, W. B., Weinstein, J. N., and Lavista, J. M. (2023). All sustainable development goals support good health and well-being. Int. J. Public Health 68:1606901. doi: 10.3389/ijph.2023.1606901
Keywords: artificial intelligence, healthcare, shared decision making, social determinants of heath, population health, healthcare delivery
Citation: Weeks WB, Lavista Ferres JM and Weinstein JN (2024) Artificial intelligence: promise and peril in achieving the quadruple aim in healthcare. Front. Artif. Intell. 7:1430756. doi: 10.3389/frai.2024.1430756
Received: 10 May 2024; Accepted: 05 June 2024;
Published: 19 June 2024.
Edited by:
Christos A. Frantzidis, University of Lincoln, United KingdomReviewed by:
Panteleimon Chriskos, Aristotle University of Thessaloniki, GreeceCopyright © 2024 Weeks, Lavista Ferres and Weinstein. This is an open-access article distributed under the terms of the Creative Commons Attribution License (CC BY). The use, distribution or reproduction in other forums is permitted, provided the original author(s) and the copyright owner(s) are credited and that the original publication in this journal is cited, in accordance with accepted academic practice. No use, distribution or reproduction is permitted which does not comply with these terms.
*Correspondence: William B. Weeks, wiweeks@microsoft.com