- 1Department of Electronic Engineering, University of Rome Tor Vergata, Rome, Italy
- 2Interdisciplinary Center of Advanced Study of Organ-on-Chip and Lab-on-Chip Applications (IC-LOC), University of Rome Tor Vergata, Rome, Italy
- 3Department of System Medicine and Centro di Biomedicina Spaziale (CBMS), University of Rome Tor Vergata, Rome, Italy
- 4San Raffaele Rome University, Rome, Italy
- 5Istituto di Ricovero e Cura a Carattere Scientifico (IRCCS) San Raffaele, Rome, Italy
- 6Department of Biomedicine and Prevention, University of Rome Tor Vergata, Rome, Italy
Introduction
One of the most remarkable properties of the brain is the ability to construct representations of the external environment, which can be used to simulate and plan future interactions. In the past 50 years, neuroscientists have devised novel techniques to observe, understand and modulate this capacity, either by visualizing neural activity in vivo or by recording and stimulating the brain via electrodes or electromagnetic fields. This effort has been invaluable also in advancing research on artificial intelligence (AI) and a strong crosstalk has ensued from which both domains have already benefited. Progresses in neural network design have laid the basis for using AI to identify anomalies in brain functioning and modeling neurological disorders but successful computer-assisted therapy as well as a complete understanding of how these diseases arise and evolve are far from acquired (Macpherson et al., 2021). Yet, based on 2016 data, these disorders are the leading cause of disability and second leading cause of death. In the US, approximately one in six children is born with a neurodevelopmental disorder (Mencattini et al., 2018), and 6.5 million individuals aged 65 and older suffer from Alzheimer's disease, a number only destined to increase (Eichmueller, 2022). Hence, to tackle these silent pandemics, we need new, “outside-the-box” research tools and strategies that allow us to devise highly personalized approaches (Kanai and Rees, 2011). In this paper, we first present the possible advantages offered by brain organoids (i.e., engineered cell-based in vitro models of in vivo tissues) and assembloids (i.e., 3D, self-organizing structures that functionally combine two or more organoids, allowing to model interactions between different tissues or regions) as additional tools to track and model neural activity, particularly with reference to complex functions like learning and memory. Next, we approach the methodological problems that still need to be solved before these novel instruments can be successfully implemented, examining the possible limits of an organoid-based approach to neurocognitive research. Finally, we discuss the ethical issues raised by the peculiar nature of brain organoids, briefly summarizing some proactive interventions that should be considered when engaging in this type of experimentation.
Organoids-based models of brain function: what they can do and what they may learn to do
The rapid development of organoids and assembloids—cultures capable of arranging stem cells into specific 3D structures (Zhong et al., 2014; Bartfeld and Clevers, 2015; Bartfeld et al., 2015; Morizane et al., 2015; Wallach and Bayrer, 2017; Pleguezuelos-Manzano et al., 2020; Lawlor et al., 2021)—underscores the need to evaluate their strengths and limitations as innovative methods for studying body functions, developing treatments, and creating new drugs. In contrast to the traditional 2D tissue cultures (D'Orazio et al., 2022), organoids possess an inherent complexity, replicate the intricate cellular compositions and 3D architectures of natural organs (Hoang and Ma, 2021; Shi et al., 2024) and mimic the genetic makeup and physiological characteristics of individual patients. Brain organoids/assembloids (BOA), which originate from the growing, dynamic collaboration between stem-cell biology and bioengineering, are not organs and may not entirely replicate the organ of interest (Jensen and Little, 2023), but they can successfully recapitulate some organ-specific functions. Accordingly, they are possibly the best models available for capturing the early stages of disease development, studying the effects of known risk factors, and testing new therapeutic approaches (Badiola-Mateos et al., 2021) (Figure 1 upper branch). However, as researchers create more complex and intricate models to replicate the structure and functionality of their in-vivo counterparts, our analytical tools must advance in sophistication to match. AI has proven to be fast, efficient, and quantitatively capable of extracting and analyzing the high-dimensional data generated from high-throughput organoid models, making it an ideal tool to deal with this type of data. Combining BOA with AI approaches (through an articulated synergy between AI variations and BOA architecture modeling) may thus lead to powerful hybrid systems (Shi et al., 2024). On the one hand, AI can be used to monitor the functioning of healthy (or diseased) BOAs, with the aim of testing new therapies or elucidating disease mechanisms. On the other hand, AI can also be exploited in hybrid AI/BOAs systems, in order to feed BOAs with appropriate stimuli and “train” them to respond, observing potential healing effects on brain diseases and aging. This scenario represents an unprecedented opportunity for both neuroscience and AI, offering a unique platform to approach cognitive processes objectively and systematically by using reproducible in-vivo human brain physiological systems, which can lead to entirely new types of preclinical studies and better tailored medical interventions. With this in mind, the fitting term Organoid Intelligence (OI), coined by Smirnova et al. (2023) by blending together AI and organoids, now describes a multidisciplinary field that aims at developing “biological computing using 3D cultures of human brain cells and brain-machine interface technologies.” This implies shifting from using machines to simulate brain activities [cf., von Neumann view (HP, 1959; Quirion, 2023) of “making computers brain-like”] to “growing the next supercomputer in a cell culture lab” (Quirion, 2023)—an opportunity that does not come without risks and poses novel methodological and ethical issues.
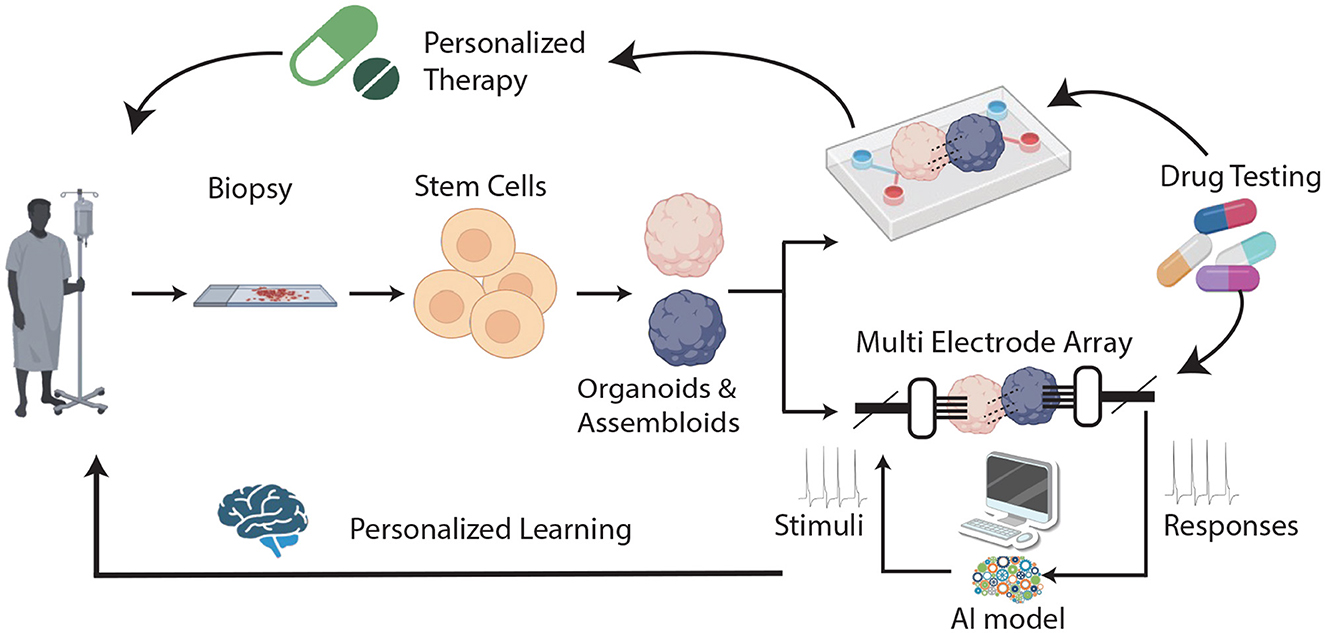
Figure 1. A general scheme of the proposed scenario. In the standard view, BOAs are cultured in an organ-on-chip (OOC) device where drug testing for personalized therapy can be performed (upper branch). In the novel scenario, BOAs are also interfaced with a MEA (lower branch): input and output electrical stimuli are manipulated through artificial intelligence (AI model) and used in learning and memory BOA tasks, leading to the possibility of developing personalized models of learning.
Albeit attractive, observing and measuring the functions of BOAs is not as straightforward as monitoring the activities of kidney or liver organoids. Functions that have an immediate–traceable–output, such as muscle contraction have been successfully replicated in organoids resembling cortex/spinal cord assemblies (Andersen et al., 2020), as are the effects of defective genes (Eichmüller and Knoblich, 2022). On the other hand, measuring BOAs responses linked to cognitive functions, such as learning and memory, is more challenging for both technical and ethical reasons. Yet, BOAs may represent a useful tool in which modeling and monitoring how specific neural assemblies learn and respond to chemicals or other factors that induce plastic changes, particularly because they grant for the possibility to be developed from the cells of a specific individual, leading to the creation of dynamic “personalized” models. Indeed, for living organisms, learning is a powerful instrument, and its impairment is one of the most dramatic consequences of disease. Through learning, systems can make and/or modify future responses based on past experiences: they can save energy by not responding to stimuli that have previously proven to be innocuous (habituation) and they can trigger responses in advance to stimulation by creating links between two or more stimuli that present in association (associative learning). Unsurprisingly, learning is a widespread phenomenon. Not only humans and animals can learn, but so can unicellular organisms (Tang and Marshall, 2018) and machines. By means of algorithms that associate different speech profiles to different labels, vocal assistants like Siri and Alexa learn to recognize the voice of the various speakers in a household and eventually address each member by his/her given name. Although we may view this behavior as if the assistant were “remembering” us (intentional instance; Dennett, 1988), the personalized approach results from a logic gate—not dissimilar from what happens in neural networks when cell discharge favors one of many possible pathways. In either case, learning develops as an implicit process, i.e., does not imply any form of conscious reflection on previous experiences or knowledge, but relies on adaptive modifications of behavior as a function of experience.
Recent studies suggest that this kind of learning can be observed in a 2D neuronal culture linked to a computer and that brain organoids may be trained to play a computer game (Kagan et al., 2022) or control movements of a robot (Schley, 2018). Although these findings do not indicate that cell cultures exhibit any form of sentient behavior (Friston, 2023), they provide a proof of concept that complex cognitive functions may be explored in brain organoids (BOAs), opening an entirely new realm of possibilities for research into neurological diseases, which can be described as “Assembloid learning.” In fact, one could envisage that in a near future, “personalized models” of how neural assemblies learn and undergo plastic changes could be developed, and used to assist brain care, for example by facilitating selection of the best treatment for each patient (e.g., by running in vitro trials first) (Figure 1). For this approach to be extended to functions such as memory, which lack a simple or unique measurable output, BOAs could benefit by hybridization with other approaches, like AI. Indeed, if proper AI approaches can be devised for optimizing the encoding/decoding of temporal-spatial information to and from BOAs (see Figure 1), it could be possible to elucidate how the individual brain responds to external factors and disease. In this scenario, an additional domain likely to benefit from this technique could be that of neurodevelopment, whose stages could be directly monitored, and the effects of potential threats explored at different times.
Current limits hindering BOAs approaches
Though promising, the domain of BOA research is still in its infancy and several challenges remain to be addressed. Here, we will list the most prominent, indicating how current research may provide possible solutions.
First, developing BOAs and exploring how organoid intelligence works requires that these unique 3D cultures are correctly cultivated in vitro and their activities in response to input stimuli are successfully tracked. Neither pre-condition is easily satisfied. Despite the success of various protocols (Miura et al., 2020; Revah et al., 2022), organoids still suffer from low reproducibility, high heterogeneity, low generation throughput and necrosis/hypoxia. While heterogeneity may represent an advantage, allowing for the development of diverse neuronal connections and interactions (Sabate-Soler and Kurniawan, 2024) and promoting highly personalized treatment approaches, it is also a challenging aspect to control. Different culturing protocols, batch-to-batch variations, cell lines and/or gene expression, for example, can reduce coherence in the datasets, increasing the degree of unexplained heterogeneity, and hindering reproducibility and findings' reliability. Development of automated platforms (Jiang et al., 2020), adoption and sharing of standardized protocols, definition of the maturity state of the neural assemblies could certainly improve these limits.
Second, detecting BOAs activity is another critical issue. Fluorescence imaging methods, such as those using calcium indicators (Zhang et al., 2023) and voltage sensors (Evans et al., 2023), widely used to measure activity in neuronal populations, have limited temporal resolution and may suffer from photobleaching or phototoxicity. On the other hand, electrophysiological recordings can monitor neural activity over long intervals with high temporal resolution but may interfere with organoids cytoarchitecture and development due to direct contact with the neural substrate. Multielectrode arrays (MEAs) are a reasonable compromise, being versatile, and coming in different configurations (Sharf et al., 2022). A further promising opportunity comes from the innovative “Kirigami electronics” (KiriE) approach (Yang et al., 2023). Current platforms such as Brainoware (Cai et al., 2023) use flat and rigid MEA electrodes for interfacing with organoids but can only stimulate/record from a small number of neurons on the surface. In this respect, advantages could arise from further miniaturization of electrodes as well as re-arrangement of their topology. Alternatively, the way BOAs are grown could be changed, creating flat architectures that maintain the internal BOA network structure but can be accessed with standard MEA technology. Multimodal techniques for BOA interfacing should also be envisaged, exploring the possibility of integrating microfluidics, optical microscopy, optogenetics, and microfabrication to stimulate and read feedback from BOAs grown in a single platform.
Third, these stimulating opportunities, however, raise additional challenges. BOAs are living cells that require nutrition in more than one sense. Not only vascularization must be ensured but also power consumption of the infrastructure interfacing BOAs should be considered. Though intrinsically low, the addition of any complementary peripheral equipment (e.g., CO2 incubator) requires power, and technological solutions to decrease consumption should be developed, especially in virtue of a massive usage.
Fourth, novel and efficient modalities for data interpretation and visualization should be envisaged to deal with the large amount of information that can be extracted from these specimens. Graph theory-based methods (GTM) have recently proved reliable in partially understanding brain architecture, allowing for example to discriminate different forms of connectivity in fMRI studies (Farahani and Karwowski, 2019). However, cognitive, emotional, and behavioral responses are highly dynamic phenomena, both during development and in adult life. Presently, measures obtained via brain graph only display a snapshot of the disease over time, preventing immediate observation of the dynamics of brain networks (Fleischer et al., 2019). By extending the findings of fMRI studies (Madhyastha et al., 2018) and integrating GTM approach into the BOA environment, it would be possible to track the development of pathological conditions, as well as temporal correlations with topological alterations in the brain network.
Finally, but importantly, it is still a matter of debate how faithfully brain organoids replicate neural assemblies. A recent study for example (Bhaduri et al., 2020), reported that compared to primary cortical cells, single cells derived from organoids present differences in spatial organization and glial specificity—possibly due to ectopically activated cellular stress pathways. These observations suggest a word of caution, especially for studies on neurodevelopment, while, at the same time, indicate novel frameworks for improving the accuracy of cortical organoids (e.g., by acting on metabolic stress, transplantation), which may further benefit from the development of assembloids that may better capture the complexity of neural circuits (Miura et al., 2020, 2022).
Discussion
Inevitably, the opportunity of developing brain models that can receive/process inputs and acquire implicit learning capabilities raises important ethical questions related to how these entities should be considered (Koplin and Savulescu, 2019; Lavazza, 2021; Jeziorski et al., 2023; Kagan et al., 2023a). Maturation of BOAs is accelerated by growth factors so that organoids at 10 weeks of culture show features (such as myelination) that in fetuses are viewed only after 20 weeks of gestation (Jakovcevski et al., 2009), instilling doubts as to whether they could potentially exhibit consciousness (Sawai et al., 2019) or experience rudimentary forms of pain. In fact, while developing brain organoids for modeling disease and/or testing novel drug appears both appealing and justified, the possible moral implications of novel technologies that may stem from this approach should also be proactively considered: for example, 2D-cell cultures have been already successfully connected to computers (Dennett, 1988) and organoids to muscle tissue (Andersen et al., 2020), opening to a plethora of enticing—but also ethically concerning possibilities. Recently, a debate ensued from mention in a paper that “in vitro, neurons learn and exhibit sentience” (Balci et al., 2023; Kagan et al., 2023b), suggesting that at least one major question should be promptly addressed. Although the nature and physiological mechanisms supporting consciousness is a puzzle that will not be rapidly solved, the forming OI community should consider exploring which definitions apply to culture models, qualifying and “translating” what is meant by “consciousness,” “sentience,” (Friston, 2023) or “awareness to stimuli” when BOAs are considered, and/or what terms should be used to describe more basic capacities, such as responding to sensory inputs (Friston, 2002; American Psychological Association, 2023). On this respect, a recent paper (Boyd, 2024) has proposed a series of qualities that should be considered when reasoning on the moral status of non-conventional entities—and that could represent a useful benchmark for dealing with neuroethical issues pertaining to brain organoids and assembloids.
Addressing issues related to the brain relies on a delicate equilibrium between enthusiasm for the potential benefits offered by biological computing in terms of improvements for public health, and a general fear of the unknown that can lead to skepticism and misinformation. Legal limitations, considerations based on ethics and moral, as well as evidence-based research, should provide the basis for establishing effective, ethical frameworks and principles to guide this vital work at the intersection of science, society, and policy, sidestepping the unfortunate challenges encountered with technologies like genetically modified organisms.
Author contributions
AM: Writing—original draft, Writing—review & editing. ED: Writing—original draft, Writing—review & editing. DD-M: Writing—original draft, Writing—review & editing. FG: Writing—original draft, Writing—review & editing. FS: Writing—original draft, Writing—review & editing. EM: Writing—original draft, Writing—review & editing.
Funding
The author(s) declare financial support was received for the research, authorship, and/or publication of this article. FG's contribution was partially funded by the Italian Ministry of Health (Ricerca Corrente # 10/1701).
Conflict of interest
The authors declare that the research was conducted in the absence of any commercial or financial relationships that could be construed as a potential conflict of interest.
The author(s) declared that they were an editorial board member of Frontiers, at the time of submission. This had no impact on the peer review process and the final decision.
Publisher's note
All claims expressed in this article are solely those of the authors and do not necessarily represent those of their affiliated organizations, or those of the publisher, the editors and the reviewers. Any product that may be evaluated in this article, or claim that may be made by its manufacturer, is not guaranteed or endorsed by the publisher.
References
American Psychological Association (2023). APA dictionary of psychology website. Available online at: https://dictionary.apa.org/ (accessed April 11, 2024).
Andersen, J., Revah, O., Miura, Y., Thom, N., Amin, N. D., Kelley, K. W., et al. (2020). Generation of functional human 3D cortico-motor assembloids. Cell 183, 1913–1929.e26. doi: 10.1016/j.cell.2020.11.017
Badiola-Mateos, M., Di Giuseppe, D., Paoli, R., Lopez-Martinez, M. J., Mencattini, A., Samitier, J., et al. (2021). A novel multi-frequency trans-endothelial electrical resistance (MTEER) sensor array to monitor blood-brain barrier integrity. Sensors Actuat. B 334:129599. doi: 10.1016/j.snb.2021.129599
Balci, F., Hamed, S. B., Boraud, T., Bouret, S., Brochier, T., Brun, C., et al. (2023). A response to claims of emergent intelligence and sentience in a dish. Neuron 111, 604–605. doi: 10.1016/j.neuron.2023.02.009
Bartfeld, S., Bayram, T., Van De Wetering, M., Huch, M., Begthel, H., Kujala, P., et al. (2015). In vitro expansion of human gastric epithelial stem cells and their responses to bacterial infection. Gastroenterology 148, 126–136.e6. doi: 10.1053/j.gastro.2014.09.042
Bartfeld, S., and Clevers, H. (2015). Organoids as model for infectious diseases: culture of human and murine stomach organoids and microinjection of Helicobacter pylori. JoVE 2015:e53359. doi: 10.3791/53359
Bhaduri, A., Andrews, M. G., Mancia Leon, W., Jung, D., Shin, D., Allen, D., et al. (2020). Cell stress in cortical organoids impairs molecular subtype specification. Nature 578, 142–148. doi: 10.1038/s41586-020-1962-0
Boyd, J. L. (2024). Dimensions of consciousness and the moral status of brain organoids. Neuroethics 17:5. doi: 10.1007/s12152-023-09538-x
Cai, H., Ao, Z., Tian, C., Wu, Z., Liu, H., Tchieu, J., et al. (2023). Brain organoid reservoir computing for artificial intelligence. Nat. Electr. 6, 1032–1039. doi: 10.1038/s41928-023-01069-w
Dennett, D. C. (1988). “The intentional stance in theory and practice,” in Machiavellian intelligence: Social expertise and the evolution of intellect in monkeys, apes, and humans, eds. R. W. Byrne, and A. Whiten (Oxford: Clarendon Press/Oxford University Press), 180–202.
D'Orazio, M., Murdocca, M., Mencattini, A., Casti, P., Filippi, J., Antonelli, G., et al. (2022). Machine learning phenomics (MLP) combining deep learning with time-lapse-microscopy for monitoring colorectal adenocarcinoma cells gene expression and drug-response. Sci. Rep. 12:8545. doi: 10.1038/s41598-022-12364-5
Eichmueller, O. L. (2022). Human cerebral organoids—A new tool for clinical neurology research. Nature Reviews Neurology 18, 661–680. doi: 10.1038/s41582-022-00723-9
Eichmüller, O. L., and Knoblich, J. A. (2022). Human cerebral organoids - a new tool for clinical neurology research. Nat. Rev. Neurol. 18, 661–680.
Evans, S. W., Shi, D. Q., Chavarha, M., Plitt, M. H., Taxidis, J., Madruga, B., et al. (2023). A positively tuned voltage indicator for extended electrical recordings in the brain. Nat. Methods 20, 1104–1113. doi: 10.1038/s41592-023-01913-z
Farahani, F. V., and Karwowski, W. (2019). Application of graph theory for identifying connectivity patterns in human brain networks: a systematic review. Front. Neurosci. 13:585. doi: 10.3389/fnins.2019.00585
Fleischer, V., Radetz, A., Ciolac, D., Muthuraman, M., Gonzalez-Escamilla, G., Zipp, F., et al. (2019). Graph theoretical framework of brain networks in multiple sclerosis: a review of concepts. Neuroscience 403, 35–53. doi: 10.1016/j.neuroscience.2017.10.033
Friston, K. (2002). Functional integration and inference in the brain. Prog. Neurobiol. 68, 113–143. doi: 10.1016/S0301-0082(02)00076-X
Hoang, P., and Ma, Z. (2021). Biomaterial-guided stem cell organoid engineering for modelling development and diseases. Acta Biomater. 132, 23–36. doi: 10.1016/j.actbio.2021.01.026
Jakovcevski, I., Filipovic, R., Mo, Z., Rakic, S., and Zecevic, N. (2009). Oligodendrocyte development and the onset of myelination in the human fetal brain. Front. Neuroanat. 3:5. doi: 10.3389/neuro.05.005.2009
Jensen, K. B., and Little, M. H. (2023). Organoids are not organs: Sources of variation and misinformation in organoid biology. Stem Cell Rep. 18, 1255–1270. doi: 10.1016/j.stemcr.2023.05.009
Jeziorski, J., Brandt, R., Evans, J. H., Campana, W., Kalichman, M., Thompson, E., et al. (2023). Brain organoids, consciousness, ethics and moral status. Semin. Cell Dev. Biol. 144, 97–102. doi: 10.1016/j.semcdb.2022.03.020
Jiang, S., Zhao, H., Zhang, W., Wang, J., Liu, Y., Cao, Y., et al. (2020). An automated organoid platform with inter-organoid homogeneity and inter-patient heterogeneity. Cell Rep. Med. 1:100161. doi: 10.1016/j.xcrm.2020.100161
Kagan, B. J., Gyngell, C., Lysaght, T., Cole, V. M., Sawai, T., Savulescu, J., et al. (2023a). The technology, opportunities, and challenges of synthetic biological intelligence. Biotechnol. Adv. 68:108233. doi: 10.1016/j.biotechadv.2023.108233
Kagan, B. J., Kitchen, A. C., Tran, N. T., Habibollahi, F., Khajehnejad, M., Parker, B. J., et al. (2022). In vitro neurons learn and exhibit sentience when embodied in a simulated game-world. Neuron 110, 3952–3969.e8. doi: 10.1016/j.neuron.2022.09.001
Kagan, B. J., Razi, A., Bhat, A., Kitchen, A. C., Tran, N. T., Habibollahi, F., et al. (2023b). Scientific communication and the semantics of sentience. Neuron 111, 606–607. doi: 10.1016/j.neuron.2023.02.008
Kanai, R., and Rees, G. (2011). The structural basis of inter-individual differences in human behaviour and cognition. Nat. Rev. Neurosci. 12, 231–242. doi: 10.1038/nrn3000
Koplin, J. J., and Savulescu, J. (2019). Moral limits of brain organoid research. J. Law Med. Ethics. 47, 760–767. doi: 10.1177/1073110519897789
Lavazza, A. (2021). Potential ethical problems with human cerebral organoids: consciousness and moral status of future brains in a dish. Brain Res. 1750:147146. doi: 10.1016/j.brainres.2020.147146
Lawlor, K. T., Vanslambrouck, J. M., Higgins, J. W., Chambon, A., Bishard, K., Arndt, D., et al. (2021). Cellular extrusion bioprinting improves kidney organoid reproducibility and conformation. Nat. Mater. 20, 260–271. doi: 10.1038/s41563-020-00853-9
Macpherson, T., Churchland, A., Sejnowski, T., DiCarlo, J., Kamitani, Y., Takahashi, H., et al. (2021). Natural and Artificial Intelligence: A brief introduction to the interplay between AI and neuroscience research. Neural. Netw. 144, 603–613. doi: 10.1016/j.neunet.2021.09.018
Madhyastha, T., Peverill, M., Koh, N., McCabe, C., Flournoy, J., Mills, K., et al. (2018). Current methods and limitations for longitudinal fMRI analysis across development. Dev. Cogn. Neurosci. 33, 118–128. doi: 10.1016/j.dcn.2017.11.006
Mencattini, A., Mosciano, F., Comes, M. C., Di Gregorio, T., Raguso, G., Daprati, E., et al. (2018). An emotional modulation model as signature for the identification of children developmental disorders. Sci. Rep. 8:14487. doi: 10.1038/s41598-018-32454-7
Miura, Y., Li, M. Y., Birey, F., Ikeda, K., Revah, O., Thete, M. V., et al. (2020). Generation of human striatal organoids and cortico-striatal assembloids from human pluripotent stem cells. Nat. Biotechnol. 38, 1421–1430. doi: 10.1038/s41587-020-00763-w
Miura, Y., Li, M. Y., Revah, O., Yoon, S. J., Narazaki, G., Paşca, S. P., et al. (2022). Engineering brain assembloids to interrogate human neural circuits. Nat. Protoc. 17, 15–35. doi: 10.1038/s41596-021-00632-z
Morizane, R., Lam, A. Q., Freedman, B. S., Kishi, S., Valerius, M. T., Bonventre, J. V., et al. (2015). Nephron organoids derived from human pluripotent stem cells model kidney development and injury. Nat. Biotechnol. 33, 1193–1200. doi: 10.1038/nbt.3392
Pleguezuelos-Manzano, C., Puschhof, J., van den Brink, S., Geurts, V., Beumer, J., Clevers, H., et al. (2020). Establishment and culture of human intestinal organoids derived from adult stem cells. Curr. Protoc. Im. 130:e106. doi: 10.1002/cpim.106
Quirion, R. (2023). Brain organoids: are they for real? Front. Sci. 1:1148127. doi: 10.3389/fsci.2023.1148127
Revah, O., Gore, F., Kelley, K. W., Andersen, J., Sakai, N., Chen, X., et al. (2022). Maturation and circuit integration of transplanted human cortical organoids. Nature 610, 319–326. doi: 10.1038/s41586-022-05277-w
Sabate-Soler, S., and Kurniawan, H. (2024). Advanced brain organoids for neuroinflammation disease modeling. Neural Regener. Res. 19, 154–155. doi: 10.4103/1673-5374.375321
Sawai, T., Sakaguchi, H., Thomas, E., Takahashi, J., and Fujita, M. (2019). The ethics of cerebral organoid research: being conscious of consciousness. Stem Cell Rep. 13, 440–447. doi: 10.1016/j.stemcr.2019.08.003
Schley, L. (2018). Meet the scientists connecting lab-grown “mini brains” to robots. Available at: https://www.discovermagazine.com/mind/meet-the-scientists-connecting-lab-grown-mini-brains-to-robots (accessed April 11, 2024).
Sharf, T., van der Molen, T., Glasauer, S. M., Guzman, E., Buccino, A. P., Luna, G., et al. (2022). Functional neuronal circuitry and oscillatory dynamics in human brain organoids. Nat. Commun. 13:4403. doi: 10.1038/s41467-022-32115-4
Shi, H., Kowalczewski, A., Vu, D., Liu, X., Salekin, A., Yang, H., et al. (2024). Organoid intelligence: integration of organoid technology and artificial intelligence in the new era of in vitro models. Med. Novel Technol. Dev. 21:100276. doi: 10.1016/j.medntd.2023.100276
Smirnova, L., Caffo, B. S., Gracias, D. H., Huang, Q., Morales Pantoja, I. E., Tang, B., et al. (2023). Organoid intelligence (OI): the new frontier in biocomputing and intelligence-in-a-dish. Front. Sci. 1:1017235. doi: 10.3389/fsci.2023.1017235
Tang, S. K. Y., and Marshall, W. F. (2018). The Physarum cells (a form of amoeba) habituate and learn to anticipate periodic stimuli, while the Paramecium (an animal-like protist) might even be capable of associative learning Cell learning. Curr. Biol. 28, R1180–R1184. doi: 10.1016/j.cub.2018.09.015
Wallach, T. E., and Bayrer, J. R. (2017). Intestinal organoids: new frontiers the study of intestinal disease and physiology. J. Pediatr. Gastroenterol. Nutr. 64, 180–185. doi: 10.1097/MPG.0000000000001411
Yang, X., Forro, C., Li, T. L., Miura, Y., Zaluska, T., Tsai, C. T., et al. (2023). Kirigami electronics for long-term electrophysiological recording of human neural organoids and assembloids. bioRxiv 2023–09. doi: 10.1101/2023.09.22.559050
Zhang, Y., Rózsa, M., Liang, Y., Bushey, D., Wei, Z., Zheng, J., et al. (2023). Fast and sensitive GCaMP calcium indicators for imaging neural populations. Nature 615, 884–891. doi: 10.1038/s41586-023-05828-9
Keywords: organoid intelligence, assembloid learning, brain organoids (BOs), machine learning (ML), personalized medicine, neurodegenerative diseases
Citation: Mencattini A, Daprati E, Della-Morte D, Guadagni F, Sangiuolo F and Martinelli E (2024) Assembloid learning: opportunities and challenges for personalized approaches to brain functioning in health and disease. Front. Artif. Intell. 7:1385871. doi: 10.3389/frai.2024.1385871
Received: 13 February 2024; Accepted: 08 April 2024;
Published: 19 April 2024.
Edited by:
Brett J. Kagan, Cortical Labs, AustraliaReviewed by:
Chris Gyngell, The University of Melbourne, AustraliaLomax Boyd, Johns Hopkins University, United States
Copyright © 2024 Mencattini, Daprati, Della-Morte, Guadagni, Sangiuolo and Martinelli. This is an open-access article distributed under the terms of the Creative Commons Attribution License (CC BY). The use, distribution or reproduction in other forums is permitted, provided the original author(s) and the copyright owner(s) are credited and that the original publication in this journal is cited, in accordance with accepted academic practice. No use, distribution or reproduction is permitted which does not comply with these terms.
*Correspondence: Eugenio Martinelli, bWFydGluZWxsaUBpbmcudW5pcm9tYTIuaXQ=