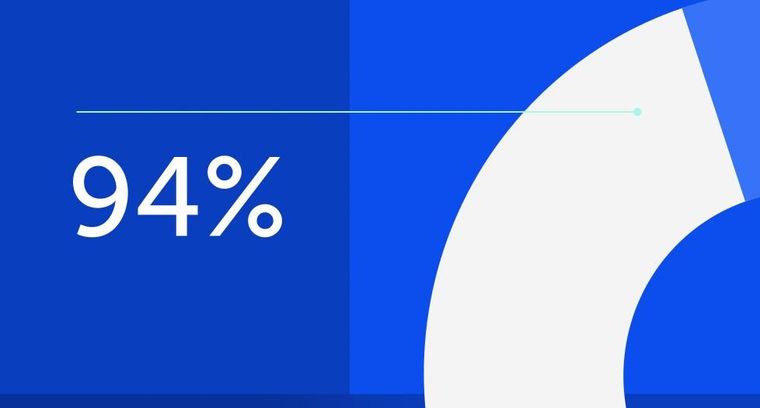
94% of researchers rate our articles as excellent or good
Learn more about the work of our research integrity team to safeguard the quality of each article we publish.
Find out more
SYSTEMATIC REVIEW article
Front. Artif. Intell., 16 February 2024
Sec. AI for Human Learning and Behavior Change
Volume 7 - 2024 | https://doi.org/10.3389/frai.2024.1349668
Introduction: Digital accessibility involves designing digital systems and services to enable access for individuals, including those with disabilities, including visual, auditory, motor, or cognitive impairments. Artificial intelligence (AI) has the potential to enhance accessibility for people with disabilities and improve their overall quality of life.
Methods: This systematic review, covering academic articles from 2018 to 2023, focuses on AI applications for digital accessibility. Initially, 3,706 articles were screened from five scholarly databases—ACM Digital Library, IEEE Xplore, ScienceDirect, Scopus, and Springer.
Results: The analysis narrowed down to 43 articles, presenting a classification framework based on applications, challenges, AI methodologies, and accessibility standards.
Discussion: This research emphasizes the predominant focus on AI-driven digital accessibility for visual impairments, revealing a critical gap in addressing speech and hearing impairments, autism spectrum disorder, neurological disorders, and motor impairments. This highlights the need for a more balanced research distribution to ensure equitable support for all communities with disabilities. The study also pointed out a lack of adherence to accessibility standards in existing systems, stressing the urgency for a fundamental shift in designing solutions for people with disabilities. Overall, this research underscores the vital role of accessible AI in preventing exclusion and discrimination, urging a comprehensive approach to digital accessibility to cater to diverse disability needs.
Digital accessibility is integral to modern times, especially because a significant percentage of the population is with one or multiple disabilities today. According to the World Health Organization (WHO), 16% of the global population (or ~1.3 billion people) currently suffers from significant disability (World Health Organization, 2022). This statistic highlights the need for digital content and services to be accessible to people with disabilities so that they are not excluded from actively participating the digital society today (Dobransky and Hargittai, 2016). Consequently, digital accessibility is not only a social responsibility, but also essential for legal compliance, inclusion, and business benefits. It ensures that everyone, regardless of their abilities or disabilities, has equal access to digital content and services, and is an essential factor of an organization that provides digital content or services. Digital accessibility, as defined by the Web Accessibility Initiative (WAI), implies that people with disabilities should be able to access, navigate, perceive, and interact with content [Initiative (WAI), 2022]. Digital accessibility refers to the practice of designing digital systems and services in a manner that makes them accessible to all individuals, including those with disabilities (Sharma et al., 2020). This includes ensuring that websites, software, and other digital products can be used by people with visual, auditory, motor, or cognitive impairments. Therefore, it is important to ensure that everyone, regardless of their ability, can access and use digital products without facing barriers or discrimination. If this is not done, it may result in people with disabilities being excluded from opportunities, diminishing their degree of independence.
Artificial intelligence (AI) is the capacity of a machine or computer system to simulate and perform tasks that typically require human intelligence, such as logical reasoning, learning, and problem resolution (Hassani et al., 2020). The determination of when applications and services can be classified as intelligent, as opposed to merely emulating intelligent behavior, is a topic of debate (Schank, 1991). Notwithstanding the ongoing philosophical discourse surrounding the extent and limitations of AI, remarkable progress has been made in a vast array of disciplines, such as machine learning, natural language processing, and computer vision. For example, AI-powered voice assistants such as Amazon Alexa, Apple Siri, and Google Assistant have become increasingly popular because of their ability to interpret natural language and offer user-friendly responses (McLean et al., 2021). In addition, AI pattern recognition algorithms have proven beneficial in several applications, including facial recognition, image processing, and object detection (Abiodun et al., 2019; Fu, 2019; Liu et al., 2020). AI has benefited industries by monitoring and detecting defects in various processes, resulting in increased productivity and decreased downtime (Pimenov et al., 2023). AI has also been instrumental in forecasting using intricate data analysis to make predictions. AI has been beneficial for improving treatment processes in the healthcare industry. For instance, tools powered by AI have been used to analyze medical images and identify abnormalities, resulting in more precise diagnosis and treatment recommendations (Liu et al., 2006). Smart assistive technologies, such as wheelchairs designed for people with limited mobility (Leaman and La, 2017) and canes intended for people with visual impairments (Hapsari et al., 2017), leverage advancements in AI to offer enhanced products and services. These advancements demonstrate the immense potential of AI in improving accessibility and user experience, making it an intriguing field of study for both researchers and practitioners.
The importance of digital accessibility in the AI era cannot be overstated. With the increasing use of AI in all spheres of life, it is crucial to ensure that these technologies are accessible to all individuals. This review presents the current state of the application of AI in the digital accessibility sector and proposes a classification system for identifying accessibility standards and frameworks, challenges, AI methodologies, and functionalities of AI in digital accessibility.
The next section provides background information on AI and its applications in various industries. Subsequently, an overview of current applications of AI in the digital accessibility sector is provided. This is followed by the research methodology, which describes the process of systematic review mapping and presents research results based on a classification framework. Subsequently, we discuss the current literature on the four dimensions of accessibility frameworks/standards, challenges, methodologies, and applications of AI for digital accessibility. Finally, future research directions and implications are discussed.
As artificial intelligence continues to permeate various aspects of our lives, it is important to investigate its impact on the digital accessibility of people with disabilities. The inception of the term “Artificial Intelligence” or “AI” dates back to the 1950's when it emerged as a concept for designing machines with the ability to perform tasks that resemble human-like cognitive abilities (Schwendicke et al., 2020). In recent years, AI has made significant advancements in various industries including healthcare, finance, and transportation. With the development of machine learning, natural language processing, and deep learning algorithms, machines can now learn from data and improve their performances over time. This has the potential to significantly improve accessibility for people with disabilities, particularly in the health care industry. For example, AI is already being used to analyze medical images and diagnose diseases, such as cancer (Bi et al., 2019). In the finance industry, AI can also be used for tasks such as fraud detection and credit scoring (Zhou et al., 2018), which could potentially affect the financial accessibility of people with disabilities. However, it is important to monitor the use of AI in finance to ensure that it does not perpetuate discrimination against people with disabilities or other marginalized groups. Overall, although AI has the potential to improve accessibility and inclusion, it is important to approach its development and implementation with caution and focus on inclusivity.
Rapid advancements in technology have led to an increase in the use of digital devices for various purposes, including access to healthcare services. However, not everyone has equal access to these digital resources because of various barriers, such as physical, sensory, and cognitive disabilities. AI-powered technologies such as Automated Speech Recognition (ASR), Google Neural Machine Translation (GNMT), Google Vision API, and DeepMind are increasingly being used to improve accessibility for people with disabilities (Bragg et al., 2019). These technologies have the potential to transform interactions with digital devices and platforms. For example, ASR can provide captions and subtitles for video content (Alonzo et al., 2022), and image and facial recognition technologies can assist people with visual impairments (Feng et al., 2020). The utilization of AI-generated summaries in digital content can provide an advantage for screen reader users by breaking down lengthy texts into more manageable portions (Chen et al., 2023). Furthermore, AI can simulate user behavior to pinpoint and rectify navigation issues, and automate regression testing. Additionally, the implementation of AI-powered chatbots and virtual assistants can offer accessible communication options for people with speech and hearing impairments (Shezi and Ade-Ibijola, 2020; Subashini and Krishnaveni, 2021). However, the potential risks associated with AI in this context must be considered, such as the possibility of perpetuating existing biases and neglecting the needs and experiences of certain groups of people with disabilities. It is crucial to address these potential drawbacks to ensure that these technologies are truly inclusive and provide benefits for all users.
Machine learning algorithms can recognize patterns and identify accessibility barriers to digital content (Abduljabbar et al., 2019). For example, image recognition algorithms can automatically provide alternative text descriptions for images, making them accessible to the visually impaired (Bigham et al., 2006). Similarly, natural language processing algorithms can identify and correct language that may be difficult for people with cognitive impairments to understand (Le Glaz et al., 2021). By creating machine-learning algorithms that specifically address the issues of digital accessibility, we can create technology that benefits all users, regardless of their abilities. However, it is crucial that these algorithms are developed with inputs from people with disabilities to ensure that they are effective and meet their needs. In addition, it is important to consider the potential biases present in the data used to train these algorithms, as they may perpetuate existing inequalities and exclusion. By addressing these issues and prioritizing accessibility in machine-learning algorithms, we can work toward a more inclusive and equitable digital future for all.
Advancements in AI have created new opportunities to enhance digital accessibility for people with disabilities (Hapsari et al., 2017). However, as AI technology progresses, it is crucial to closely examine its impact on accessibility and to ensure that these technologies are developed in an equitable and inclusive manner. Despite the growing interest in the intersection of AI and digital accessibility, a comprehensive systematic review of the current state of knowledge and practices in this field is yet to be conducted. This systematic review aimed to fill this research gap by providing a comprehensive analysis of the current state of knowledge and practices related to AI and digital accessibility. By reviewing the existing literature, this study offers valuable insights into the potential benefits of AI for people with disabilities as well as identifying potential challenges and opportunities. Furthermore, this review can guide future research and development activities toward creating more inclusive and accessible technologies. The results of this systematic review can provide valuable resources for researchers, practitioners, and policymakers working in the fields of digital accessibility and AI by identifying gaps in current knowledge and practices, which can help promote digital accessibility and support the development of inclusive technologies that benefit everyone.
This literature review followed the procedures and processes for conducting literature searches proposed by Watson (2015), and was conducted in three phases: planning, execution, and reporting. The systematic review methodology used in this study incorporated the strategies and guidelines outlined by Kitchenham et al. (2007) and Ali et al. (2018, 2020, 2021, 2023). Figure 1 depicts the steps involved in the systematic review.
In the planning phase, the need for a systematic review was identified, a classification framework was defined, research questions were defined, and a research protocol was developed. During the execution phase, the search query was conducted based on the following keywords: exclusion and inclusion criteria were applied; screening based on title and abstract reading, full-text reading, and quality assessment were conducted. The reporting phase included classification of the chosen articles and discussion of the findings.
To our knowledge, no systematic review has comprehensively outlined these research findings, while providing a profound analysis of the research and practice related to the topic of digital accessibility, with a specific focus on AI applications for people with disabilities. Although limited in number, existing surveys on digital accessibility are often specialized in nature, with many being country-specific studies or tailored to specific fields, such as education and software engineering processes (Bong and Chen, 2021; Chadli et al., 2021; Paiva et al., 2021; Prado et al., 2023). However, despite these valuable efforts, no comprehensive systematic review has yet extensively examined the intersection of digital accessibility and AI applications within the existing literature. This study seeks to address this gap by providing an extensive analysis of this field, revealing potential benefits, challenges, and opportunities.
In this step, the research review protocol used for the literature review was finalized. The classification framework is based on a model initially developed by Ngai and Wat (2002), which is based on how advanced technologies have enhanced various fields. The framework was developed and modified by Ali et al. (2018, 2020, 2021, 2023). The framework used in our study takes into consideration four dimensions of how AI is used in different sectors about accessibility: applications, AI methodology and techniques, design standards and frameworks used, and challenges. Table 1 provides more details on the classification framework used in our study.
Table 1. Classification framework (modified from Ngai and Wat, 2002).
Formulating the research questions for this study is a crucial step in systematic reviews (Paul et al., 2021). The research questions identified for the study are as follows:
RQ 1. What are the AI applications that have been used to enhance digital accessibility?
RQ 2. What are the different AI techniques that have been used to enhance the accessibility of digital systems for people with disabilities?
RQ 3. What are the key challenges and barriers that must be overcome to achieve accessible AI systems for people with disabilities ?
RQ 4. What are the different accessibility standards that have been used to guide the design and implementation of accessible AI systems?
To minimize bias, strategies for article selection were determined at this stage. As a part of this process, a comprehensive search strategy was created to encompass a wide-ranging search of various databases, and a manual review of the selected articles was conducted. The databases chosen for this systematic review included the ACM Digital Library, IEEEXplore, ScienceDirect, Scopus, and Springer. During the research process, filtering tools were employed for every database to minimize duplication. In conducting the manual review, the broad manual review method was utilized, which entailed scanning the title and abstract of each research article (Golder et al., 2014), followed by reading the full content of the selected articles to eliminate any irrelevant ones. Table 2 lists the eligibility criteria used in this study.
The execution stage involved the implementation of the steps outlined in the planning phase. The main steps undertaken in the execution stage are as follows:
• To ensure a thorough search, we utilized key terms extracted from relevant research papers and research questions, including synonyms, acronyms, and spelling variations of these terms. The search terms were then classified into three categories: those related to AI, technology-specific terms, and accessibility in the context of disability. The keywords identified for this study were: ((“Artificial Intelligence” OR “AI”) AND (“Digital” OR “technology” OR “technologies” OR “technological”) AND (“accessibility” OR “inclusion” OR “inclusivity” OR “disability” OR “disabled” OR “special needs” OR “impaired” OR “impairment”)).
• Filtering tools were used to refine the research results while conducting the online database search. Several filters were implemented in this study, including the year of publication (2018–2023), document type (journal articles and conference papers), and language (English). Given the vast quantity of results, additional filters, such as “title,” “abstract,” and “keyword” search, as well as “open access” filters, were utilized to refine the search. Of the 50,655 search results returned by the Springer database, only the first 1,000 were considered for this review.
• The articles were subjected to abstract and keyword screening followed by full-text screening.
• To ensure the validity of the articles included in this study, certain quality assessment criteria were applied to confirm their value. Quality assessment of the candidate papers followed the guidelines outlined in Kitchenham et al. (2007) study. Consequently, we formulated the following questions to evaluate quality.
1. Are the aims clearly stated?
2. Are data collection methods adequately described?
3. Are all of the study questions answered?
4. Is the AI technique and methodology used in this study fully defined?
5. How do these results add to the literature?
6. Are the limitations of this study were adequately addressed.
7. How clear and coherent are reports?
Each QA inquiry was evaluated using a three-point rating system. A response of “yes” received a score of 1, a “partial” response earned 0.5 points, and a “no” response was assigned a score of 0. If the study effectively addressed the QA question, it received a full point, whereas a partial response warranted 0.5 points. Any paper that failed to address the QA question was assigned a score of 0. The quality of the research articles was assessed using the QA questions to determine the overall quality score for each study. An inclusion criterion was established, requiring a minimum overall score of four for research inclusion. Studies with scores below four were excluded.
Data collection for this review was conducted between May 18, 2023, and May 24, 2023. Figure 2 shows the final number of articles selected for this review. The search results based on the keywords and search filters applied identified 3,706 articles. Duplicates and retracted articles were removed prior to the abstract, title, and keyword screening. In total, 2,424 articles were removed at this stage. During the full-text screening stage, 850 articles were excluded, leaving 280 articles. These articles were subjected to quality assessment, and 42 articles were retained for the final analysis.
Figure 3 illustrates the dissemination of articles by the year of publication. The first publication on the utilization of AI in accessibility was published in 2018, whereas the most substantial number of articles (13) were released in 2022. Conversely, only one article was published in 2018.
Figure 4 depicts the distribution of the selected articles by the database source. A total of 41 articles were identified in Scopus, followed by one article each from ScienceDirect and ACM Digital databases. No articles were selected from IEEEXplore or Springer database, as they were either duplicates or had to be excluded as they did not meet the inclusion criteria during the screening process.
The findings of the review were the result of a comprehensive analysis of articles related to AI and digital accessibility that were presented and evaluated. The classification framework was applied by considering four dimensions: accessibility guidelines, frameworks, standards, challenges, methodologies, and applications. Additional information is provided in Tables 3–6.
The reviewed literature emphasizes the development of various applications aimed at enhancing the mobility, safety, and quality of life of the visually impaired. These applications include an array of functionalities such as obstacle detection, navigation, educational support, and social media accessibility.
Several systems have been specifically designed for obstacle detection and spatial information. For example, Balakrishnan et al. (2023) presented a novel synchronization protocol for vision sensors that provides real-time obstacle detection and spatial detail to improve mobility and safety. Building upon this, Royal et al. (2023) went a step further by not only detecting objects but also offering real-time object recognition and text reading within images, thereby improving overall accessibility.
In the domain of safety, Abdusalomov et al. (2022) utilized AI-based fire detection and classification methods to monitor and forecast fire-related scenarios, thereby providing early warnings that are specifically tailored to the needs of blind and visually impaired individuals. Akter et al. (2022) examined shared ethical and privacy considerations relevant to people with visual impairments, making a significant contribution to the ethical development of assistive technologies.
The AviPer system utilizes a visual-tactile multimodal attention network that incorporates a self-developed flexible tactile glove and webcam (Li et al., 2022). This novel approach enables visually impaired individuals to perceive and interact with their surroundings. Furthermore, Chaitra et al. (2022) presented a viable and cost-effective solution for visualizing environments using handheld devices, such as mobile phones.
In urban environments, Montanha et al. (2022) employed context awareness and AI methods to aid visually impaired pedestrians in safely navigating roadways and generating real-time instructions based on traffic conditions.
Duarte et al. (2021) worked to enhance the accessibility of social media content, particularly benefiting people with visual impairments. In addition, Thomas and Meehan (2021) introduced a mobile banknote recognition application and Wadhwa et al. (2021) improved environmental understanding by offering braille-readable captions for digital images.
Lo Valvo et al. (2021) concentrated on the development of indoor and outdoor localization and navigation technologies for visually impaired individuals. Harum et al. (2021) presented a multi-language interactive device that enables the reading and translation of text from various printed materials. Karyono et al. (2020) addressed the issue of lighting discomfort experienced by the visually impaired and elderly, by introducing a specially designed system.
Kose and Vasant (2020) offered effective walking-path support to university students with visual impairments, enhancing their overall campus experience. Joshi et al. (2020) introduced an AI-based fully automated assistive system with real-time perception, obstacle-aware navigation, and auditory feedback. The AI-Vision smart glasses, outlined in Alashkar et al. (2020), utilize machine learning algorithms for facial expression/age/gender recognition and color recognition, providing assistance in daily tasks. Furthermore, See and Advincula (2021) automated the production of tactile educational materials, such as tactile flashcards, tactile maps, and tactile peg puzzles, which incorporate interactive tactile graphics and braille captions, serving as a versatile tool for teaching concepts such as shapes and geography to visually impaired and blind students.
Lucibello and Rotondi (2019), Saha et al. (2019), and Watters et al. (2020) aimed to improve the lives of both blind and visually impaired individuals. Lucibello and Rotondi (2019) focused on supporting blind athletes in the track and field, promoting independent running, and enhancing spatial awareness through the learning of echolocation skills. Saha et al. (2019) has created a system that provides near-real-time information about the surroundings through a smartphone camera, enhancing the mobility skills of people with visual impairments. Finally, Watters et al. (2020) aimed to provide visually impaired students with a virtual assistant in the laboratory that could be controlled through natural language, eliminating the need for specific keywords or phrases.
This section explores innovative technologies developed to cater to people with speech and hearing impairments. These technologies have been categorized into distinct applications that offer a range of benefits. For instance, a graphene-based wearable artificial throat was developed to provide highly accurate speech recognition and voice reproduction capabilities, particularly for those who have undergone laryngectomy (Yang et al., 2023). Additionally, a platform that recognizes complex sign language was designed to offer an effective means of communication for speech-impaired children (Ullah et al., 2023). The automatic recognition of two-handed signs in Indian Sign Language also serves as a teaching assistant, enhancing cognitive abilities and fostering interest in learning for hearing and speech-impaired children (Sreemathy et al., 2022). The real-time interpretation and recognition of American Sign Language contributes to seamless control of smart home components, addressing the specific needs of people who are deaf or hard-of-hearing in their interactions with smart home devices (Rajasekhar and Panday, 2022).
AI Technology has also been utilized to enhance information accessibility through more efficient American Sign Language animations (Al-Khazraji et al., 2021). Real-time video transcripts are provided to improve the accessibility of video content for people with hearing impairments or deafness in Saudi Arabia (Zaid alahmadi and Alsulami, 2020). Finally, the utilization of virtual reality technology in live communication during theatrical performances demonstrates its potential to enhance speech comprehension for people who are deaf or hard of hearing (Teófilo et al., 2018). These insights collectively highlight the diverse applications of AI in addressing the communication and accessibility needs of people with speech and hearing impairments, emphasizing the potential for transformative effects on their daily lives and societal inclusion.
Hughes et al. (2022) concentrated on advancements in virtual AI companion (AIC) and facilitating students with autism spectrum disorder (ASD) in STEM learning environments while fostering their social and communication skills.
Zingoni et al. (2021) aimed to enhance accessibility and inclusion for dyslexic students by creating a supportive platform that utilizes best practices for educators and institutions to predict the most suitable methodologies and digital tools for students with the goal of addressing the challenges they face in their academic journeys.
Cimolino et al. (2021) aimed to tackle the issue of inaccessibility in gaming for people with disabilities, particularly those with spinal cord injuries, by proposing a novel method called “partial automation” that enables an AI partner to manage inaccessible game inputs to improve overall accessibility in gaming.
This section encompasses an array of innovative applications that cater to people with other disabilities. Campomanes-Alvarez and Rosario Campomanes-Alvarez (2021) focused on individuals with profound intellectual and multiple disabilities, devising the INSENSION platform to recognize facial expressions, thereby enabling interaction with digital applications and enhancing their quality of life. Second, Ingavelez-Guerra et al. (2022) implemented a multilevel methodological approach to automatically adapt open educational resources within e-learning environments, considering the diverse needs and preferences of students with various disabilities, including hearing impairments, physical disabilities, intellectual and developmental disabilities, visual impairments, and mental health issues. Third, Guo et al. (2021) presented a virtual human social system that empowered individuals with facial disabilities, deafmutes, and autism to engage in face-to-face video communication. Additionally, Hezam et al. (2023) utilized a hybrid multi-criteria decision-making method to prioritize digital technologies for enhancing transportation accessibility for people with disabilities, addressing barriers, and selecting technologies through an uncertain decision-making framework. Furthermore, Lu et al. (2020) aimed to aid people with disabilities in operating tractors, ensuring safe operation when consciousness and limb movements are inconsistent, whereas Lin et al. (2019) focused on providing wheelchair-accessible bus rides for people with disabilities. The INSENSION Platform for Personalized Assistance of Non-symbolic Interaction for individuals with profound intellectual and multiple disabilities (PIMD), developed by Kosiedowski et al. (2020), supports independence by recognizing non-symbolic behaviors and collecting contextual information through video, audio, and sensor data. Park et al. (2021) proposed an online infrastructure to enable large-scale remote data contributions from disability communities to create inclusive AI systems. These diverse applications underscore the importance of tailored technological solutions in promoting independence, accessibility, and improved quality of life for people with disabilities, emphasizing the need for an inclusive design and comprehensive consideration of user needs in the development of assistive technologies.
The accessibility of digital systems for people with disabilities can be enhanced by employing various AI techniques, which can be classified into several domains including Machine Learning (ML), Deep Learning (DL), Natural Language Processing (NLP), Edge AI, and Computer Vision.
Several studies have demonstrated diverse applications of computer vision in enhancing accessibility. Balakrishnan et al. (2023) utilized object recognition for identifying and predicting object types, employing techniques such as object localization and detection. Jayawardena et al. (2019) used computer vision for object recognition and hand gesture/movement recognition in an educational context. In addition, Lo Valvo et al. (2021) employed Convolutional Neural Networks (CNNs) for recognizing objects or buildings. Royal et al. (2023) developed a real-time object recognition and text extraction from images using deep learning algorithms in combination with the Pytesseract OCR Engine. Chaitra et al. (2022) employed a pre-trained Caffe Object Detection method to facilitate the text-to-signal processing feature summarizing the objects detected in the form of an audio catalog. Abdusalomov et al. (2022) employed AI techniques such as fire, object, and text recognition, along with object mapping. Duarte et al. (2021) employed various AI methodologies including image recognition, text recognition in images, semantic similarity measures between text descriptions and image concepts, and language identification. Saha et al. (2019) utilized AI techniques, including object recognition, within the Landmark AI computer vision system, which employed a smartphone camera to offer real-time information and identify and describe landmarks and signs in the user's surroundings.
NLP plays a critical role in making digital systems more accessible. Ingavelez-Guerra et al. (2022) transformed digital educational resources using NLP for automatic speech recognition (ASR) to extract textual information from videos. In addition, Wadhwa et al. (2021) utilized NLP to preprocess raw image and caption data, incorporating a pretrained CNN for image feature encoding and an LSTM-based RNN for generating natural language descriptions. Google's speech-to-text recognition, which falls under NLP, was integrated to transcribe and process the spoken language into text for analysis (Zaid alahmadi and Alsulami, 2020). Furthermore, Joshi et al. (2020) implemented various NLP techniques including YOLO-v3 object detection, an optical character recognizer, and a text-to-speech module for generating audio prompts. Teófilo et al. (2018) combined AI techniques, including a speech-to-text algorithm for Portuguese, a sentence prediction algorithm for selecting the correct speech based on initial text, and a word correction algorithm to ensure the converted words are valid in the Portuguese language. Harum et al. (2021) leveraged third party apps such as Google Cloud services, including the Cloud Vision API for image-to-text conversion, the Cloud Translation API for translating the converted text, and Google Cloud Text-to-Speech for converting the translated text into speech.
The system proposed by Yang et al. (2022) showcased the utilization of Edge AI in a cost-effective manner. The combination of Edge AI and computer vision enabled the system to employ one-stage detectors and 2-D human pose estimation for non-motorized users, emphasizing the importance of edge computing in efficient real-time processing of data. Additionally, the SINAPSI device in Lucibello and Rotondi (2019) employed Edge AI, utilizing ultrasonic sensors and machine learning algorithms for 3D environmental awareness, demonstrating its application in innovative environmental awareness solutions.
Various studies have employed machine learning techniques to address accessibility challenges. For instance, Ullah et al. (2023) utilized KNN, decision trees, random forest, and neural networks to decipher sign languages, underscoring the versatility of machine learning in linguistic contexts. A range of machine learning techniques, including Naïve Bayes, K-Nearest Neighbor, Stochastic Gradient Descent, Logistic Regression, Neural Networks, and Random Forest, have been used to classify facial expressions and jaw movements (Campomanes-Alvarez and Rosario Campomanes-Alvarez, 2021). Zingoni et al. (2021) primarily employed machine learning techniques, starting with supervised ML algorithms, to predict appropriate supporting materials for dyslexic students based on questionnaire and clinical report data, with a potential transition to deep learning methods for more complex data processing. The Speech Recognition Engine in Jayawardena et al. (2019) utilized ML to recognize a user's voice commands, demonstrating the adaptability of machine learning across diverse applications.
The utilization of deep-learning methodologies has been prevalent in several studies, including Royal et al. (2023), which facilitated real-time object recognition and text extraction using deep-learning algorithms. Li et al. (2022) used a visual-tactile fusion classification model, which is a multimodal deep learning model that combines visual and tactile information to classify objects, and three attention mechanisms, namely temporal, channel-wise, and spatial attention, which are used to improve the accuracy of the classification model. See and Advincula (2021) utilized a model based on the Mask RCNN model trained using the common objects with context (COCO) dataset with a backbone of ResNet-50.
Rajasekhar and Panday (2022) implemented a 1D CNN as the deep learning model in an ASL gesture interpreter, while Thomas and Meehan (2021) employed a CNN to implement object detection in a banknote recognition system.
Zingoni et al. (2021) primarily employed machine learning techniques, starting with supervised ML algorithms with a potential transition to deep learning methods for more complex data processing. Kosiedowski et al. (2020) harnessed video and audio analysis, pattern recognition, and deep-learning techniques to recognize various aspects of users with Profound and Multiple Intellectual Disabilities. The integration of YOLOv3, a real-time image recognition model, by Lin et al. (2019) highlighted the effective use of deep learning for image recognition and notification. Yang et al. (2023) utilized spectrogram-based feature extraction with pre-trained neural networks, k-fold cross-validation, and an ensemble model involving AlexNet, ReliefF, and an SVM classifier to enhance speech recognition accuracy. Abdusalomov et al. (2022) utilized the YOLOv5m model for real-time monitoring and enhanced the detection accuracy of indoor fire disasters. In their study, Sreemathy et al. (2022) utilized four distinct deep learning models, namely, AlexNet, GoogleNet, VGG-16, and VGG-19 and Histogram Oriented Gradient (HOG) features for feature extraction for the automatic recognition of two-handed signs of Indian Sign Language. Montanha et al. (2022) employed a signal trilateration technique complemented by deep learning (DL) for image processing. Montanha et al. (2022) also leveraged a pre-trained deep neural network model from the open-source toolkit OpenVINO2 (Open Visual Inference and Neural Network Optimization) by Intel. Campomanes-Alvarez and Rosario Campomanes-Alvarez (2021) employed Long-Short Term Memory (LSTM) Neural Networks for jaw movement classification. Wadhwa et al. (2021) employed AI techniques involving preprocessing of raw image and caption data, using a pretrained Convolutional Neural Network (CNN) for encoding image features into high-dimensional vectors, and subsequently utilizing a Long Short-Term Memory (LSTM) based Recurrent Neural Network (RNN) for decoding and generating natural language descriptions. Lo Valvo et al. (2021) utilized Convolutional Neural Networks (CNNs) that have been trained to recognize objects or buildings. Guo et al. (2021) leveraged deep-learning technology for facial restoration, incorporated affective computing for emotion recognition, and employed these elements in the design of virtual avatars. Karyono et al. (2020) utilized artificial neural networks in their adaptive lighting system which considers behavioral adaptation aspects for visually impaired people. Lu et al. (2020) employed a tractor driving control method that combined EEG-based input with a recurrent neural network with transfer learning (RNN-TL) deep learning algorithm.
Ingavelez-Guerra et al. (2022) utilized various AI techniques to adapt digital educational resources for the diverse needs of learners. It employs automatic speech recognition (ASR) to extract textual information from videos, thereby making the text more readable. In addition, the system leverages convolutional neural networks (CNNs) and recurrent neural networks (RNNs) for image classification and description. Furthermore, it utilized long short-term memory neural networks (LSTM NNs) from the Tesseract OCR library for text recognition. Natural language processing (NLP) has also been applied to aid in describing images using nearby text. Li et al. (2022) introduced a visual-tactile fusion classification model, a multimodal deep learning approach that combined visual and tactile information for improved object classification. Cimolino et al. (2021) applied AI techniques utilizing the Unity game engine along with the Behavior Designer plugin. The system developed by Kose and Vasant (2020) employs various AI techniques, including optimization-based AI techniques, such as the Dijkstra algorithm, ant colony optimization, intelligent water drop algorithm, and speech recognition interface. Watters et al. (2020) integrated an Alexa smart speaker and custom Alexa Skill for natural language interaction, a Talking LabQuest with AI for data collection and analysis, and a Raspberry Pi for coordination, effectively combining AI techniques with components to create a virtual AI lab assistant for enhanced laboratory assistance. In a study by Lin et al. (2019), machine learning and deep learning techniques, particularly neural networks, were harnessed for image recognition using YOLOv3, a real-time image recognition model, for object recognition and notification. In addition, chatbot technology was implemented using the LINE platform, demonstrating the integration of AI methodologies in both image recognition and chatbot development. Alashkar et al. (2020) employed a modern Convolutional Neural Network (CNN) named Mini Xception for facial features recognition, utilized the K-Nearest Neighbors (K-NN) algorithm for color recognition, and provided sound feedback using the IBM Watson Text to Speech API. These studies demonstrated the potential of combining deep learning with other techniques to develop more robust and adaptive systems that cater to diverse user requirements.
A multitude of challenges confront efforts to enhance accessibility for people with disabilities by introducing barriers that may lead to exclusion and marginalization. Understanding and categorizing the various factors that influence the adoption of AI can aid stakeholders in devising specialized approaches to overcome the multifaceted challenges associated with its implementation. These challenges span various aspects, each of which requires careful consideration for successful implementation of accessible technologies.
System complexity and lack of user friendliness can create difficulties for people with various disabilities, hindering their ability to use and benefit from technology. Implementation barriers, such as cost, lack of technical support, and insufficient awareness and training among users and caregivers, affect the overall accessibility and usability of AI systems (Lu et al., 2020; Cimolino et al., 2021; Theodorou et al., 2021).
The incorporation of AI into digital accessibility systems has resulted in several data challenges. These challenges encompass matters pertaining to the inadequacy and questionable quality of data, along with apprehensions regarding data privacy, surveillance, administration, and the potential for bias and discrimination in the utilization of data within AI systems. The limited availability of training data, with people with disability comprising only 0.5–3% of the total user population, presents a significant challenge in achieving high levels of accuracy in speech recognition technology (Yang et al., 2022). Li et al. (2022) faced challenges in accurate tactile data acquisition for wearable devices, handling heterogeneous data from tactile and visual sensors. In their investigation, Zaid alahmadi and Alsulami (2020) encountered biases in the data, as the evaluation results of the prototype may have been influenced by the participation of only female participants, which overlooked the gender diversity present in Saudi Arabian universities. Additionally, Lu et al. (2020) encountered limitations in obtaining a comprehensive dataset because of constraints such as site-specific conditions, environmental variables, and tractor operating factors. Furthermore, maintaining consistency in virtual datasets is problematic, requiring ongoing algorithm adjustments (Lu et al., 2020). Acosta-Vargas et al. (2022) emphasized the importance of inclusive data collection processes, acknowledging the varying challenges faced by participants of different types and prominence of disabilities. This includes physical disabilities affecting phone usage, blindness posing challenges in photography tasks, considerations for cultural aspects and sign language fluency for deaf participants. These challenges highlight the need for innovative solutions and strategies to address the issue of data scarcity for specific user groups.
Considering the privacy and security challenges associated with integrating assistive technologies, several issues have been noted in various studies. The accurate acquisition of tactile data for wearable devices raises privacy and security concerns, particularly related to webcam usage (Li et al., 2022). Similarly, the development of digitally accessible games introduced ethical dilemmas, encompassing issues of player autonomy, privacy, and potential unintended consequences tied to partial automation. Privacy and security concerns were also integral to the challenges faced in designing an indoor navigation system (Lo Valvo et al., 2021) and developing the avatar-to-person system (Guo et al., 2021), emphasizing the necessity for technical expertise in AI and 3D modeling. The study conducted by Zaid alahmadi and Alsulami (2020) faced challenges linked to the need to navigate privacy concerns, especially given the impact of including only female participants. Involving people with disabilities in data collection processes underscores the significance of inclusive practices, recognizing diverse accessibility challenges and implicitly emphasizing the importance of safeguarding privacy (Acosta-Vargas et al., 2022). These challenges collectively highlight the multifaceted nature of integrating assistive technologies and the imperative to address privacy and security across various domains.
Karyono et al. (2020) drew attention to the deficiency of a dependable predictive lighting model for visually impaired and elderly individuals, the scarcity of implementation of lighting standards, and potential barriers such as system cost and insufficient awareness. Similarly, Jayawardena et al. (2019) emphasized the challenges in enhancing the lives of visually impaired children, including the dearth of research studies, limited resources and funding, and the requirement for user-friendly, affordable systems in developing countries.
The challenges faced by Teófilo et al. (2018) include technical setup issues affecting user experience, accuracy of captioning for users with hearing impairments, and usability factors such as head and eye strain, brightness, and device weight.
Li et al. (2022) presented several challenges in the acquisition of accurate tactile data for wearable devices, including the handling of heterogeneous data and concerns related to privacy and security, which can impact users with varying motor abilities. Additionally, Cimolino et al. (2021) highlighted challenges in the development of digitally accessible games, particularly in interpreting the intentions of players with diverse motor abilities and designing interfaces for partial automation.
Park et al. (2021) involved people with disabilities and highlighted the challenges faced by those with cognitive disabilities, such as difficulties with reading and typing tasks, including people with attention deficit hyperactivity disorder (ADHD) and dyslexia.
Other challenges include the lack of resources and funding for the research and development of systems that enhance accessibility (Jayawardena et al., 2019). Lo Valvo et al. (2021) faced challenges related to physical infrastructure adaptation, 3D object registration, and accessibility design. Guo et al. (2021) identified challenges related to psychological barriers, accessibility issues, the need for technical expertise in relevant areas, and the importance of user acceptance and adoption influenced by factors such as ease of use and perceived usefulness.
Various frameworks and accessibility standards have been employed in AI-based research endeavors aimed at enhancing digital accessibility for people with disabilities. One notable framework is the “Framework for value co-design and co-creation” (Vieira et al., 2022), which centers on the impact of Virtual Assistants (VAs) on the wellbeing of people with disabilities. This framework emphasizes the co-creation of value through interactions between people with disabilities and VA technology within their home environments.
Additionally, addressing the adaptability and accessibility of Open Educational Resources (OER), the need for new metadata aligned with Universal Design for Learning guidelines is highlighted (Ingavelez-Guerra et al., 2022). Cimolino et al. (2021) explored the improvement in game accessibility by utilizing multiple frameworks, including universal design, player balancing, and interface adaptation. Within this context, universal design focuses on the creation of products and environments that cater to a broad spectrum of individuals irrespective of their abilities.
In the domain of e-commerce website accessibility, Acosta-Vargas et al. (2022) predominantly relied on the Web Content Accessibility Guidelines (WCAG) 2.1, employing a modified approach to the Website Accessibility Conformance Evaluation Methodology (WCAG-EM) 1.0, for automated evaluations using the Web Accessibility Evaluation Tool (WAVE) to identify potential WCAG 2.1-related accessibility concerns. Furthermore, See and Advincula (2021) evaluated system usability through the ISO/IEC 25010:2011 SQuaRE quality models, which encompass factors such as appropriateness, recognizability, learnability, operability, user error protection, user interface aesthetics, and accessibility.
The Digital Accessible Information System (DAISY) standard allows for utmost flexibility in integrating text and audio, accommodating various combinations ranging from pure audio, text-only, full text, to full audio integration.
Karyono et al. (2020) employed various accessibility standards, including CIE 1,231:1997 for addressing lighting needs of partially sighted individuals, CIE 196:2011 for enhancing accessibility in lighting, and CIE 227:2017 for lighting considerations in buildings for older individuals and those with visual impairments.
In their study, Hezam et al. (2023) harnessed a decision-making framework grounded in Fermatean fuzzy information, incorporating AI methodologies from the field of expert systems, to comprehensively evaluate the suitability of digital technologies within the realm of sustainable transportation for people with disabilities.
Yeratziotis and Van Greunen (2013) outlined guidelines for deaf and hard of hearing-friendly mobile app design, drawing from sources like telecom accessibility guidelines and industry practices (e.g., Apple, Samsung, and Google) which were used to guide the system developed in Zaid alahmadi and Alsulami (2020).
Table 7 shows the results of the classification framework.
Most research in the field of AI-driven digital accessibility has primarily focused on addressing the needs of people with visual impairment. This emphasis on AI solutions related to visual impairment is evident from the multitude of innovations and systems designed to enhance the lives of the visually impaired. These include a wide range of applications, from real-time obstacle detection and object recognition to tactile educational materials, and even AI-driven smart glasses. The impact of AI on digital accessibility for the visually impaired has been profound, leading to improved mobility, safety, and educational opportunities (Lo Valvo et al., 2021; See and Advincula, 2021; Abdusalomov et al., 2022). However, this concentration of visual impairments has highlighted a significant gap in the research landscape. There is a paucity of comprehensive AI systems tailored to address the unique challenges faced by people with other disabilities such as speech and hearing impairments, autism spectrum disorder (ASD), neurological disorders, and motor impairments. While there are some noteworthy AI solutions for these other disability types (Zingoni et al., 2021; Ullah et al., 2023), the sheer volume of research and innovation predominantly dedicated to visual impairment underscores the need for a more equitable distribution of research efforts. It is crucial to expand the scope of AI-driven digital accessibility to bridge this gap and provide people with various disabilities with the same level of support, independence, and accessibility that the visually impaired enjoy. This will require concerted effort to foster innovation and research in AI systems tailored to the specific needs of these communities. Our research highlights the urgent need for a fundamental shift in the design and development of systems catering to people with disabilities.
To address these shortcomings, the research agenda must prioritize a 2-fold approach. First, researchers must realign their efforts toward a more comprehensive examination of disabilities, ensuring that the unique challenges faced by people with diverse impairments are adequately considered. Second, it is imperative to augment data-collection efforts involving people with disabilities to capture a broader range of experiences and requirements. This inclusive approach not only enhances the inclusivity of technological solutions but also provides a more robust and informed foundation for future research and development in the field. A more focused effort is necessary to comprehend how the information requirements of people with disabilities can vary across different contexts, cultures, and audiences and how their needs are context-dependent (Akter et al., 2022). Future research should explore this aspect to enhance the AI-driven accessibility. Park et al. (2021) suggested that motivating people with disabilities for AI data collection should involve fair monetary compensation, non-monetary incentives, and transparent communication regarding data use and privacy. To ensure accessibility, the data collection process should be streamlined and consider the diverse range of abilities within disability categories, avoid punitive measures, and acknowledge potential performance anxiety among people with disabilities. Future research should prioritize algorithmic accountability, transparency, and explainability in the development of assistive technologies (Akter et al., 2022). This approach ensures that users can better understand and trust the functioning of AI-driven systems, thereby contributing to their overall effectiveness and acceptance. Maintaining the confidentiality and privacy of the collected data is crucial, especially when the data are publicly available to promote large-scale machine learning advancements (Li et al., 2022). It is essential to extend the reach of the infrastructure to individuals beyond WEIRD societies, who may use different platforms, devices with lower specifications, or have limited access to broadband, and to support cultural adaptations in the data collection process (Li et al., 2022).
Although every effort has been made to ensure the comprehensiveness of the present research, it is possible that some significant studies may have been overlooked because of the vast number of results retrieved from one of the databases.
The significance of access to the Internet and digital content has intensified in recent times; however, not all individuals, particularly those with disabilities, have equitable access to this technology. By employing a thorough review and evaluation using a classification framework, this research specifically focuses on the impact of AI on digital accessibility in the context of disability. The most prevalent AI methodologies utilized are edge AI, NLP, Computer Vision, machine learning, and deep learning. The most common challenges encountered were related to data, technical difficulties, security and privacy concerns, and operational difficulties. The findings reveal a prevalent focus on AI-driven digital accessibility for people with visual impairments, indicating a substantial gap in addressing other disabilities. This research underscores the imperative need to realign efforts toward a more comprehensive examination of disabilities, urging researchers to broaden their scope and enhance data collection efforts involving people with various disabilities. The shortcomings of existing systems regarding adherence to accessibility standards highlight the pressing need for a fundamental shift in the design of solutions that prioritize the needs of people with disabilities. The study underscores the critical role of accessible AI in preventing exclusion and discrimination and emphasizes the urgency for a comprehensive approach to digital accessibility that accommodates diverse disability needs. As we move forward into the digital age, where Internet access is increasingly integral to education, entertainment, and communication, organizations are encouraged to prioritize and invest in digital accessibility. By adhering to established guidelines and standards, organizations can bridge the digital divide and ensure a fair and enjoyable online experience for all users regardless of their abilities. This not only promotes equal opportunities for individuals with disabilities, but also enhances overall usability and satisfaction for all users. Ultimately, this research calls for a concerted effort to make digital accessibility a cornerstone of our digital landscape, fostering an inclusive environment that benefits the entire user spectrum.
The raw data supporting the conclusions of this article will be made available by the authors, without undue reservation.
KC: Data curation, Investigation, Methodology, Resources, Software, Visualization, Writing – original draft, Writing – review & editing. AO: Conceptualization, Methodology, Project administration, Supervision, Validation, Writing – original draft, Writing – review & editing.
The author(s) declare that no financial support was received for the research, authorship, and/or publication of this article.
The authors declare that the research was conducted in the absence of any commercial or financial relationships that could be construed as a potential conflict of interest.
All claims expressed in this article are solely those of the authors and do not necessarily represent those of their affiliated organizations, or those of the publisher, the editors and the reviewers. Any product that may be evaluated in this article, or claim that may be made by its manufacturer, is not guaranteed or endorsed by the publisher.
Abduljabbar, R., Dia, H., Liyanage, S., and Bagloee, S. A. (2019). Applications of artificial intelligence in transport: an overview. Sustainability 11:189. doi: 10.3390/su11010189
Abdusalomov, A. B., Mukhiddinov, M., Kutlimuratov, A., and Whangbo, T. K. (2022). Improved real-time fire warning system based on advanced technologies for visually impaired people. Sensors 22:197305. doi: 10.3390/s22197305
Abiodun, O. I., Jantan, A., Omolara, A. E., Dada, K. V., Umar, A. M., Linus, O. U., et al. (2019). Comprehensive review of artificial neural network applications to pattern recognition. IEEE Access 7, 158820–158846. doi: 10.1109/ACCESS.2019.2945545
Acosta-Vargas, P., Salvador-Acosta, B., Salvador-Ullauri, L., and Jadán-Guerrero, J. (2022). Accessibility challenges of e-commerce websites. PeerJ Comput. Sci. 8:891. doi: 10.7717/peerj-cs.891
Akter, T., Ahmed, T., Kapadia, A., and Swaminathan, M. (2022). Shared privacy concerns of the visually impaired and sighted bystanders with camera-based assistive technologies. ACM Trans. Access. Comput. 15:3506857. doi: 10.1145/3506857
Alashkar, R., Elsabbahy, M., Sabha, A., Abdelghany, M., Tlili, B., and Mounsef, J. (2020). “AI-vision towards an improved social inclusion,” in IEEE/ITU Int. Conf. Artif. Intell. Good, AI4G (Institute of Electrical and Electronics Engineers Inc.) (Geneva), 204–209.
Ali, O., Abdelbaki, W., Shrestha, A., Elbasi, E., Alryalat, M. A. A., and Dwivedi, Y. K. (2023). A systematic literature review of artificial intelligence in the healthcare sector: benefits, challenges, methodologies, and functionalities. J. Innov. Knowl. 8:100333. doi: 10.1016/j.jik.2023.100333
Ali, O., Ally, M., Dwivedi, Y., and others (2020). The state of play of blockchain technology in the financial services sector: a systematic literature review. Int. J. Inf. Manag. 54:102199. doi: 10.1016/j.ijinfomgt.2020.102199
Ali, O., Jaradat, A., Kulakli, A., and Abuhalimeh, A. (2021). A comparative study: blockchain technology utilization benefits, challenges and functionalities. IEEE Access 9, 12730–12749. doi: 10.1109/ACCESS.2021.3050241
Ali, O., Shrestha, A., Soar, J., and Wamba, S. F. (2018). Cloud computing-enabled healthcare opportunities, issues, and applications: a systematic review. Int. J. Inf. Manag. 43, 146–158. doi: 10.1016/j.ijinfomgt.2018.07.009
Al-Khazraji, S., Dingman, B., Lee, S., and Huenerfauth, M. (2021). “At a different pace: evaluating whether users prefer timing parameters in American sign language animations to differ from human signers' timing,” in ASSETS—Int. ACM SIGACCESS Conf. Comput. Access. (Association for Computing Machinery, Inc).
Alonzo, O., Shin, H. V., and Li, D. (2022). “Beyond subtitles: captioning and visualizing non-speech sounds to improve accessibility of user-generated videos,” in Proceedings of the 24th International ACM SIGACCESS Conference on Computers and Accessibility (New York, NY), 1–12.
Balakrishnan, A., Ramana, K., Ashok, G., Viriyasitavat, W., Ahmad, S., and Gadekallu, T. R. (2023). Sonar glass—artificial vision: comprehensive design aspects of a synchronization protocol for vision based sensors. Meas. J. Int. Meas. Confed. 211:2636. doi: 10.1016/j.measurement.2023.112636
Bi, W. L., Hosny, A., Schabath, M. B., Giger, M. L., Birkbak, N. J., Mehrtash, A., et al. (2019). Artificial intelligence in cancer imaging: clinical challenges and applications. Cancer J. Clin. 69, 127–157. doi: 10.3322/caac.21552
Bigham, J. P., Kaminsky, R. S., Ladner, R. E., Danielsson, O. M., and Hempton, G. L. (2006). “WebInSight: making web images accessible,” in Proceedings of the 8th International ACM SIGACCESS Conference on Computers and Accessibility (New York, NY), 181–188.
Bong, W. K., and Chen, W. (2021). Increasing faculty's competence in digital accessibility for inclusive education: a systematic literature review. Int. J. Incl. Educ. 2021, 1–17. doi: 10.1080/13603116.2021.1937344
Bragg, D., Koller, O., Bellard, M., Berke, L., Boudreault, P., Braffort, A., et al. (2019). “Sign language recognition, generation, and translation: an interdisciplinary perspective,” in The 21st International ACM SIGACCESS Conference on Computers and Accessibility (Pittsburgh, PA). Available online at: https://hal.science/hal-02394580 (accessed November 19, 2023).
Campomanes-Alvarez, C., and Rosario Campomanes-Alvarez, B. (2021). “Automatic facial expression recognition for the interaction of individuals with multiple disabilities,” in Int. Conf. Appl. Artif. Intell., ICAPAI (Institute of Electrical and Electronics Engineers Inc.) (Halden).
Chadli, F. E., Gretete, D., and Moumen, A. (2021). Digital accessibility: a systematic literature review. SHS Web. Conf. 119:e06005. doi: 10.1051/shsconf/202111906005
Chaitra, C., Vethanayagi, R., Manoj Kumar, M. V., Prashanth, B. S., Snehah, H. R., Thomas, L., et al. (2022). “Image/video summarization in text/speech for visually impaired people,” in MysuruCon—IEEE Mysore Sub Sect. Int. Conf. (Institute of Electrical and Electronics Engineers Inc.) (Mysuru).
Chen, X., Wu, C.-S., Murakhovs' ka, L., Laban, P., Niu, T., Liu, W., et al. (2023). Marvista: exploring the design of a human-AI collaborative news reading tool. ACM Trans. Comput.-Hum. Interact. 30, 1–27. doi: 10.1145/3609331
Cimolino, G., Askari, S., and Graham, T. C. N. (2021). The role of partial automation in increasing the accessibility of digital games. Proc. ACM Hum. Comput. Interact. 5:3474693. doi: 10.1145/3474693
Dobransky, K., and Hargittai, E. (2016). Unrealized potential: exploring the digital disability divide. Poetics 58, 18–28. doi: 10.1016/j.poetic.2016.08.003
Duarte, C., Pereira, L. S., Santos, A., Vicente, J., Rodrigues, A., Guerreiro, J., et al. (2021). “Nipping inaccessibility in the bud: opportunities and challenges of accessible media content authoring,” in ACM Int. Conf. Proc. Ser. (Association for Computing Machinery), 3–9.
Efanov, D., Aleksandrov, P., and Karapetyants, N. (2022). “The BiLSTM-based synthesized speech recognition,” in Procedia Comput. Sci., eds. F. F. Ramos Corchado and A. V. Samsonovich (Elsevier B.V.), 415–421.
Feng, C.-H., Hsieh, J.-Y., Hung, Y.-H., Chen, C.-J., and Chen, C.-H. (2020). “Research on the visually impaired individuals shopping with artificial intelligence image recognition assistance,” in Lect. Notes Comput. Sci. eds. M. Antona and C. Stephanidis (Berlin: Springer), 518–531.
Golder, S., Loke, Y. K., and Zorzela, L. (2014). Comparison of search strategies in systematic reviews of adverse effects to other systematic reviews. Health Inf. Libr. J. 31, 92–105. doi: 10.1111/hir.12041
Guo, Z., Wang, Z., and Jin, X. (2021). “Avatar To Person” (ATP) virtual human social ability enhanced system for disabled people. Wirel. Commun. Mob. Comput. 2021:5098992. doi: 10.1155/2021/5098992
Hapsari, G. I., Mutiara, G. A., and Kusumah, D. T. (2017). “Smart cane location guide for blind using GPS,” in 2017 5th International Conference on Information and Communication Technology (ICoIC7) (Melaka), 1–6.
Harum, N., Izzati, M. S. K. N., Emran, N. A., Abdullah, N., Zakaria, N. A., Hamid, E., et al. (2021). A development of multi-language interactive device using artificial intelligence technology for visual impairment person. Int. J. Interact. Mob. Technol. 15, 79–92. doi: 10.3991/ijim.v15i19.24139
Hassani, H., Silva, E. S., Unger, S., TajMazinani, M., and Mac Feely, S. (2020). Artificial intelligence (AI) or intelligence augmentation (ia): what is the future? AI 1, 143–155. doi: 10.3390/ai1020008
Hezam, I. M., Mishra, A. R., Rani, P., and Alshamrani, A. (2023). Assessing the barriers of digitally sustainable transportation system for persons with disabilities using Fermatean fuzzy double normalization-based multiple aggregation method. Appl. Soft Comput. 133:109910. doi: 10.1016/j.asoc.2022.109910
Hughes, C. E., Dieker, L. A., Glavey, E. M., Hines, R. A., Wilkins, I., Ingraham, K., et al. (2022). RAISE: robotics & AI to improve STEM and social skills for elementary school students. Front. Virtual Real. 3:968312. doi: 10.3389/frvir.2022.968312
Ingavelez-Guerra, P., Robles-Bykbaev, V. E., Perez-Munoz, A., Hilera-Gonzalez, J., and Oton-Tortosa, S. (2022). Automatic adaptation of open educational resources: an approach from a multilevel methodology based on students' preferences, educational special needs, artificial intelligence and accessibility metadata. IEEE Access 10, 9703–9716. doi: 10.1109/ACCESS.2021.3139537
Initiative (WAI), W. W. A. (2022). Introduction to Web Accessibility. Web Access. Initiat. WAI. Available online at: https://www.w3.org/WAI/fundamentals/accessibility-intro/ (accessed April 12, 2023).
Jayawardena, C., Balasuriya, B. K., Lokuhettiarachchi, N. P., and Ranasinghe, A. R. M. D. N. (2019). “Intelligent platform for visually impaired children for learning indoor and outdoor objects,” in IEEE Reg 10 Annu Int Conf Proc TENCON (Institute of Electrical and Electronics Engineers Inc.), 2572–2577.
Joshi, R. C., Yadav, S., Dutta, M. K., and Travieso-Gonzalez, C. M. (2020). Efficient multi-object detection and smart navigation using artificial intelligence for visually impaired people. Entropy 22:e22090941. doi: 10.3390/e22090941
Karyono, K., Abdullah, B., Cotgrave, A., and Bras, A. (2020). A novel adaptive lighting system which considers behavioral adaptation aspects for visually impaired people. Buildings 10:90168. doi: 10.3390/buildings10090168
Kitchenham, B., Charters, S., and others (2007). Guidelines for Performing Systematic Literature Reviews in Software Engineering.
Kose, U., and Vasant, P. (2020). Better campus life for visually impaired University students: intelligent social walking system with beacon and assistive technologies. Wirel. Netw. 26, 4789–4803. doi: 10.1007/s11276-018-1868-z
Kosiedowski, M., Radziuk, A., Szymaniak, P., Kapsa, W., Rajtar, T., Stroinski, M., et al. (2020). “On applying ambient intelligence to assist people with profound intellectual and multiple disabilities,” in Adv. Intell. Sys. Comput. eds. Y. Bi, R. Bhatia, and S. Kapoor (Berlin: Springer Verlag), 895–914.
Le Glaz, A., Haralambous, Y., Kim-Dufor, D.-H., Lenca, P., Billot, R., Ryan, T. C., et al. (2021). Machine learning and natural language processing in mental health: systematic review. J. Med. Internet Res. 23:e15708. doi: 10.2196/15708
Leaman, J., and La, H. M. (2017). A comprehensive review of smart wheelchairs: past, present, and future. IEEE Trans. Hum.-Mach. Syst. 47, 486–499. doi: 10.1109/THMS.2017.2706727
Li, X., Huang, M., Xu, Y., Cao, Y., Lu, Y., Wang, P., et al. (2022). AviPer: assisting visually impaired people to perceive the world with visual-tactile multimodal attention network. CCF Trans. Pervasive Comput. Interact. 4, 219–239. doi: 10.1007/s42486-022-00108-3
Lin, P.-J., Hung, S., Lam, S. F. S., and Chen, B. C. (2019). “Object recognition with machine learning: case study of demand-responsive service,” in Proc.—IEEE Int. Conf. Internet Things Intell. Syst., IoTaIS (Institute of Electrical and Electronics Engineers Inc.) (Bali), 129–134.
Liu, L., Ouyang, W., Wang, X., Fieguth, P., Chen, J., Liu, X., et al. (2020). Deep learning for generic object detection: a survey. Int. J. Comput. Vis. 128, 261–318. doi: 10.1007/s11263-019-01247-4
Lo Valvo, A., Croce, D., Garlisi, D., Giuliano, F., Giarré, L., and Tinnirello, I. (2021). A navigation and augmented reality system for visually impaired people. Sensors 21:93061. doi: 10.3390/s21093061
Lu, W., Wei, Y., Yuan, J., Deng, Y., and Song, A. (2020). Tractor assistant driving control method based on eeg combined with rnn-Tl deep learning algorithm. IEEE Access 8, 163269–163279. doi: 10.1109/ACCESS.2020.3021051
Lucibello, S., and Rotondi, C. (2019). The biological encoding of design and the premises for a new generation of ‘living' products: the example of sinapsi. Temes Disseny 2019, 116–139. doi: 10.46467/TdD35.2019.116-139
McLean, G., Osei-Frimpong, K., and Barhorst, J. (2021). Alexa, do voice assistants influence consumer brand engagement?—examining the role of AI powered voice assistants in influencing consumer brand engagement. J. Bus. Res. 124, 312–328. doi: 10.1016/j.jbusres.2020.11.045
Montanha, A., Oprescu, A. M., and Romero-Ternero, M. (2022). A context-aware artificial intelligence-based system to support street crossings for pedestrians with visual impairments. Appl. Artif. Intell. 36:2062818. doi: 10.1080/08839514.2022.2062818
Ngai, E. W., and Wat, F. (2002). A literature review and classification of electronic commerce research. Inf. Manage. 39, 415–429. doi: 10.1016/S0378-7206(01)00107-0
Paiva, D. M. B., Freire, A. P., and de Mattos Fortes, R. P. (2021). Accessibility and software engineering processes: a systematic literature review. J. Syst. Softw. 171:110819. doi: 10.1016/j.jss.2020.110819
Park, J. S., Bragg, D., Kamar, E., and Morris, M. R. (2021). “Designing an online infrastructure for collecting AI data from people with disabilities,” in FAccT—Proc. ACM Conf. Fairness, Account., Transpar. (Association for Computing Machinery, Inc), 52–63.
Paul, J., Lim, W. M., O'Cass, A., Hao, A. W., and Bresciani, S. (2021). Scientific procedures and rationales for systematic literature reviews (SPAR-4-SLR). Int. J. Consum. Stud. 45, O1–O16. doi: 10.1111/ijcs.12695
Pimenov, D. Y., Bustillo, A., Wojciechowski, S., Sharma, V. S., Gupta, M. K., and Kuntoglu, M. (2023). Artificial intelligence systems for tool condition monitoring in machining: analysis and critical review. J. Intell. Manuf. 34, 2079–2121. doi: 10.1007/s10845-022-01923-2
Prado, B., Gobbo Junior, J. A., and Bezerra, B. S. (2023). Emerging themes for digital accessibility in education. Sustainability 15:11392. doi: 10.3390/su151411392
Rajasekhar, N., and Panday, S. (2022). “SiBo-the Sign Bot, connected world for disabled,” in WINTECHCON—IEEE Women Technol. Conf. (Institute of Electrical and Electronics Engineers Inc.) (Bangalore).
Royal, A. B., Sandeep, B. G., Das, B. M., Bharath Raj Nayaka, A. M., and Joshi, S. (2023). “VisionX—a virtual assistant for the visually impaired using deep learning models,” in Lect. Notes Electr. Eng., eds. N. R. Shetty, N. H. Prasad, and L. M. Patnaik (Berlin: Springer Science and Business Media Deutschland GmbH), 891–901. doi: 10.1007/978-981-19-5482-5_75
Saha, M., Fiannaca, A. J., Kneisel, M., Cutrell, E., and Morris, M. R. (2019). “Closing the gap: designing for the last-few-meters wayfinding problem for people with visual impairments,” in ASSETS—Int. ACM SIGACCESS Conf. Comput. Access. (Association for Computing Machinery, Inc), 222–235.
Schwendicke, F., Samek, W., and Krois, J. (2020). Artificial intelligence in dentistry: chances and challenges. J. Dent. Res. 99, 769–774. doi: 10.1177/0022034520915714
See, A. R., and Advincula, W. D. (2021). Creating tactile educational materials for the visually impaired and blind students using ai cloud computing. Appl. Sci. Switz. 11:167552. doi: 10.3390/app11167552
Sharma, T., Legarda, R., and Sharma, S. (2020). “Assessing trends of digital divide within digital services in New York City,” in Human Interaction and Emerging Technologies Advances in Intelligent Systems and Computing, eds. T. Ahram, R. Taiar, S. Colson, and A. Choplin (Cham: Springer International Publishing), 682–687.
Shezi, M., and Ade-Ibijola, A. (2020). Deaf chat: a speech-to-text communication aid for hearing deficiency. Adv. Sci. Technol. Eng. Syst. 5, 826–833. doi: 10.25046/aj0505100
Sreemathy, R., Turuk, M., Kulkarni, I., and Khurana, S. (2022). Sign language recognition using artificial intelligence. Educ. Inf. Technol. 22:11391. doi: 10.1007/s10639-022-11391-z
Subashini, P., and Krishnaveni, M. (2021). “Artificial intelligence-based assistive technology,” in Artificial Intelligence Theory, Models, and Applications (Boca Raton, FL: Auerbach Publications), 217–240.
Teófilo, M., Lourenço, A., Postal, J., and Lucena, V. F. (2018). “Exploring virtual reality to enable deaf or hard of hearing accessibility in live theaters: a case study,” in Lect. Notes Comput. Sci. eds. M. Antona and C. Stephanidis (Berlin: Springer Verlag), 132–148.
Theodorou, L., Massiceti, D., Zintgraf, L., Stumpf, S., Morrison, C., Cutrell, E., et al. (2021). “Disability-first dataset creation: lessons from constructing a dataset for teachable object recognition with blind and low vision data collectors,” in ASSETS—Int. ACM SIGACCESS Conf. Comput. Access. (Association for Computing Machinery, Inc).
Thomas, M., and Meehan, K. (2021). “Banknote object detection for the visually impaired using a CNN,” in 2021 32nd Irish Signals and Systems Conference (ISSC) (Athlone: IEEE), 1–6.
Ullah, F., AbuAli, N. A., Ullah, A., Ullah, R., Siddiqui, U. A., and Siddiqui, A. A. (2023). Fusion-based body-worn IoT sensor platform for gesture recognition of autism spectrum disorder children. Sensors 23:1672. doi: 10.3390/s23031672
Vieira, A. D., Leite, H., and Volochtchuk, A. V. L. (2022). The impact of voice assistant home devices on people with disabilities: a longitudinal study. Technol. Forecast. Soc. Change 184:121961. doi: 10.1016/j.techfore.2022.121961
Wadhwa, V., Gupta, B., and Gupta, S. (2021). “AI based automated image caption tool implementation for visually impaired,” in ICIERA—Int. Conf. Ind. Electron. Res. Appl., Proc. (Institute of Electrical and Electronics Engineers Inc.) (New Delhi).
Watson, R. T. (2015). Beyond being systematic in literature reviews in IS. J. Inf. Technol. 30, 185–187. doi: 10.1057/jit.2015.12
Watters, J., Liu, C., Hill, A., and Jiang, F. (2020). “An artificial intelligence tool for accessible science education,” in IMCIC - Int. Multi-Conf. Complex., Informatics Cybern., Proc., eds. R. M. A. Baracho, N. C. Callaos, S. K. Lunsford, B. Sanchez, and M. Savoie (International Institute of Informatics and Systemics, IIIS), 147–150. Available online at: https://www.scopus.com/inward/record.uri?eid=2-s2.0-85085951599&partnerID=40&md5=8ff1be6533d855bc4f3338a78ba516db (accessed May 18, 2023).
World Health Organization (2022). Global Report on Health Equity for Persons With Disabilities. Geneva: World Health Organization.
Yang, H. F., Ling, Y., Kopca, C., Ricord, S., and Wang, Y. (2022). Cooperative traffic signal assistance system for non-motorized users and disabilities empowered by computer vision and edge artificial intelligence. Transp. Res. C Emerg. Technol. 145:103896. doi: 10.1016/j.trc.2022.103896
Yang, Q., Jin, W., Zhang, Q., Wei, Y., Guo, Z., Li, X., et al. (2023). Mixed-modality speech recognition and interaction using a wearable artificial throat. Nat. Mach. Intell. 5, 169–180. doi: 10.1038/s42256-023-00616-6
Yeratziotis, G., and Van Greunen, D. (2013). “Making ICT accessible for the deaf,” in 2013 IST-Africa Conference and Exhibition (IEEE), 1–9. Available online at: https://ieeexplore.ieee.org/abstract/document/6701722/ (accessed November 15, 2023).
Yue, W. S., and Zin, N. A. M. (2013). Voice recognition and visualization mobile apps game for training and teaching hearing handicaps children. Proce. Technol. 11, 479–486.
Zaid alahmadi, S., and Alsulami, R. A. (2020). ““Asmeany”: an andriod application for deaf and hearing-impaired people,” in Proc. Int. Conf. Ind. Eng. Oper. Manage. (IEOM Society), 434–435. Available online at: https://www.scopus.com/inward/record.uri?eid=2-s2.0-85088992536&partnerID=40&md5=caf9751c4881d56383dddfc8abb3d60b (accessed May 18, 2023).
Zhou, X., Cheng, S., Zhu, M., Guo, C., Zhou, S., Xu, P., et al. (2018). “A state of the art survey of data mining-based fraud detection and credit scoring,” in MATEC Web of Conferences (EDP Sciences) (Beijing), e03002.
Zingoni, A., Taborri, J., Panetti, V., Bonechi, S., Aparicio-Martínez, P., Pinzi, S., et al. (2021). Investigating issues and needs of dyslexic students at university: proof of concept of an artificial intelligence and virtual reality-based supporting platform and preliminary results. Appl. Sci. Switz. 11:104624. doi: 10.3390/app11104624
Keywords: digital accessibility, artificial intelligence (AI), research analysis, systematic review, persons with disabilities
Citation: Chemnad K and Othman A (2024) Digital accessibility in the era of artificial intelligence—Bibliometric analysis and systematic review. Front. Artif. Intell. 7:1349668. doi: 10.3389/frai.2024.1349668
Received: 05 December 2023; Accepted: 29 January 2024;
Published: 16 February 2024.
Edited by:
Timothy Arndt, Cleveland State University, United StatesReviewed by:
Amadeo José Argüelles, National Polytechnic Institute (IPN), MexicoCopyright © 2024 Chemnad and Othman. This is an open-access article distributed under the terms of the Creative Commons Attribution License (CC BY). The use, distribution or reproduction in other forums is permitted, provided the original author(s) and the copyright owner(s) are credited and that the original publication in this journal is cited, in accordance with accepted academic practice. No use, distribution or reproduction is permitted which does not comply with these terms.
*Correspondence: Achraf Othman, YW90aG1hbkBtYWRhLm9yZy5xYQ==
†ORCID: Khansa Chemnad orcid.org/0000-0001-5052-9285
Achraf Othman orcid.org/0000-0003-1290-2098
Disclaimer: All claims expressed in this article are solely those of the authors and do not necessarily represent those of their affiliated organizations, or those of the publisher, the editors and the reviewers. Any product that may be evaluated in this article or claim that may be made by its manufacturer is not guaranteed or endorsed by the publisher.
Research integrity at Frontiers
Learn more about the work of our research integrity team to safeguard the quality of each article we publish.