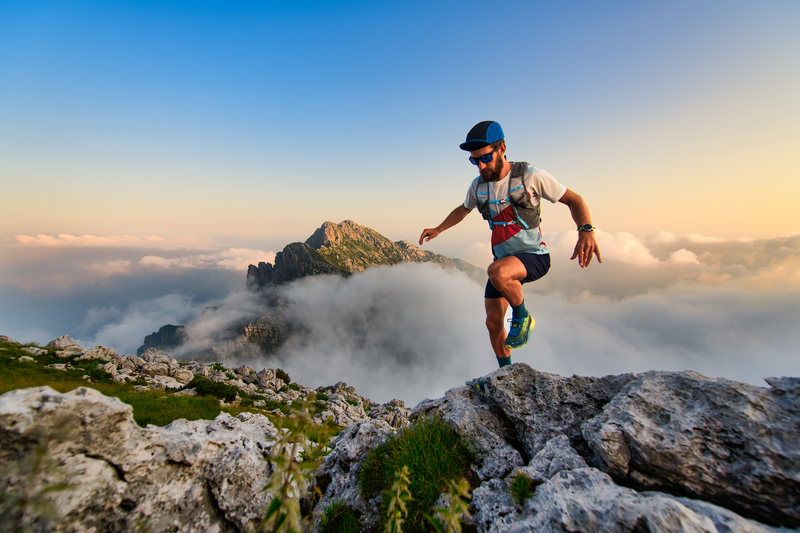
94% of researchers rate our articles as excellent or good
Learn more about the work of our research integrity team to safeguard the quality of each article we publish.
Find out more
ORIGINAL RESEARCH article
Front. Artif. Intell.
Sec. Natural Language Processing
Volume 7 - 2024 | doi: 10.3389/frai.2024.1308206
The final, formatted version of the article will be published soon.
You have multiple emails registered with Frontiers:
Please enter your email address:
If you already have an account, please login
You don't have a Frontiers account ? You can register here
Multi-round Q&A based on background text needs to infer the answer to the question through the current question, historical Q&A pairs, and background text. The pre-trained model has proved its effectiveness in this task, however, the existing model has many problems such as too many parameters and high resource consumption. We propose a knowledge transfer method that combines knowledge distillation, co-learning of similar datasets, and fine-tuning of similar tasks.Through multi-knowledge cooperative training from large model to small model, between different data sets, and between different tasks, the performance of the small model with low resource consumption can match or surpass that of the large model.
Keywords: Multi-round Q&A, knowledge transfer, Background understanding, Knowledge distillation, Model compression
Received: 09 Oct 2023; Accepted: 29 Oct 2024.
Copyright: © 2024 Huang, Song and Lu. This is an open-access article distributed under the terms of the Creative Commons Attribution License (CC BY). The use, distribution or reproduction in other forums is permitted, provided the original author(s) or licensor are credited and that the original publication in this journal is cited, in accordance with accepted academic practice. No use, distribution or reproduction is permitted which does not comply with these terms.
* Correspondence:
Xin Huang, Jiangxi Normal University, Nanchang, China
Disclaimer: All claims expressed in this article are solely those of the authors and do not necessarily represent those of their affiliated organizations, or those of the publisher, the editors and the reviewers. Any product that may be evaluated in this article or claim that may be made by its manufacturer is not guaranteed or endorsed by the publisher.
Research integrity at Frontiers
Learn more about the work of our research integrity team to safeguard the quality of each article we publish.